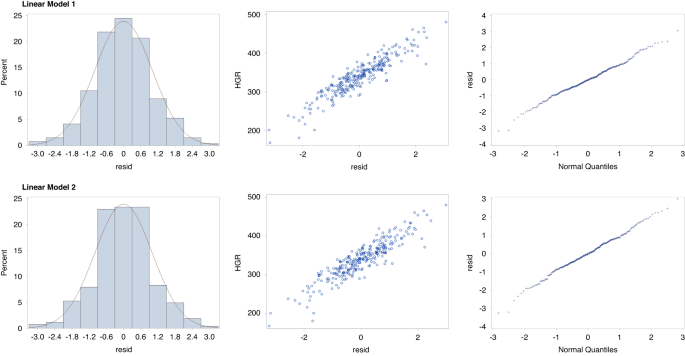
Linear hair growth rates in preschool children
- Select a language for the TTS:
- UK English Female
- UK English Male
- US English Female
- US English Male
- Australian Female
- Australian Male
- Language selected: (auto detect) - EN
Play all audios:

ABSTRACT BACKGROUND Human scalp hair is a validated bio-substrate for monitoring various exposures in childhood including contextual stressors, environmental toxins, prescription or
non-prescription drugs. Linear hair growth rates (HGR) are required to accurately interpret hair biomarker concentrations. METHODS We measured HGR in a prospective cohort of preschool
children (_N_ = 266) aged 9–72 months and assessed demographic factors, anthropometrics, and hair protein content (HPC). We examined HGR differences by age, sex, race, height, hair pigment,
and season, and used univariable and multivariable linear regression models to identify HGR-related factors. RESULTS Infants below 1 year (288 ± 61 μm/day) had slower HGR than children aged
2–5 years (_p_ = 0.0073). Dark-haired children (352 ± 52 μm/day) had higher HGR than light-haired children (325 ± 50 μm/day; _p_ = 0.0019). Asian subjects had the highest HGR overall (_p_ =
0.016). Younger children had higher HPC (_p_ = 0.0014) and their HPC-adjusted HGRs were slower than older children (_p_ = 0.0073). Age, height, hair pigmentation, and HPC were related to HGR
in multivariable regression models. CONCLUSIONS We identified age, height, hair pigment, and hair protein concentration as significant determinants of linear HGRs. These findings help
explain the known hair biomarker differences between children and adults and aid accurate interpretation of hair biomarker results in preschool children. IMPACT * Discovery of hair
biomarkers in the past few decades has transformed scientific disciplines like toxicology, pharmacology, epidemiology, forensics, healthcare, and developmental psychology. * Identifying
determinants of hair growth in children is essential for accurate interpretation of hair biomarker results in pediatric clinical studies. * Childhood hair growth rates define the
time-periods of biomarker incorporation into growing hair, essential for interpreting the biomarkers associated with environmental exposures and the mind-brain-body connectome. * Our study
describes age-, sex-, and height-based distributions of linear hair growth rates and provides determinants of linear hair growth rates in a large population of children. * Age, height, hair
pigmentation, and hair protein content are determinants of hair growth rates and should be accounted for in child hair biomarkers studies. * Our findings on hair protein content and linear
hair growth rates may provide physiological explanations for differences in hair growth rates and biomarkers in preschool children as compared to adults. You have full access to this article
via your institution. Download PDF SIMILAR CONTENT BEING VIEWED BY OTHERS SEGMENTAL ANALYSIS OF HUMAN HAIR REVEALS INTRA-ANNUAL VARIATION IN 25(OH)D3 CONCENTRATIONS IN MODERN AND
ARCHAEOLOGICAL INDIVIDUALS Article Open access 24 January 2025 SEGMENTAL HAIR ANALYSIS AS A RETROSPECTIVE TESTOSTERONE DIARY: POSSIBILITIES AND PITFALLS Article Open access 25 September 2023
STABILITY AND INTER-FAMILY ASSOCIATIONS OF HAIR ENDOCANNABINOID AND _N_-ACYLETHANOLAMINES ACROSS THE PERINATAL PERIOD IN MOTHERS, FATHERS, AND CHILDREN Article Open access 24 April 2024
INTRODUCTION Discovery of hair biomarkers in the past few decades has transformed scientific disciplines like toxicology, pharmacology, epidemiology, forensics, healthcare, and developmental
psychology.1,2,3,4 Moreover, the frequent use of hair in large population cohorts to measure environmental exposures in children has emerged from the discovery of hair biomarkers.5,6,7,8,9
Cortisol in human scalp hair is a validated biomarker for monitoring time-averaged acute/chronic responses to various familial, health, psychosocial, and economic conditions across the
lifespan.10,11,12,13,14 Hair biomarkers capture these ecological exposures and can serve as organic record logs of various exposures.11,15,16,17 To identify the time-periods for these
exposures, it is important to understand the determinants of hair growth and adjust for confounding factors.18,19,20,21 Scalp hair is characterized by growth cycles of active growth
(anagen), transition (catagen), and resting (telogen) phases; hair density, diameter, or linear length; and even according to its texture (straight or curly), shape, pigmentation, or
elasticity.22,23,24,25 These characteristics may reflect nutritional status, mitochondrial metabolism, puberty, pregnancy, or seasonal changes.18,25,26,27,28,29 Furthermore, a few hair
studies demonstrate the diversity in hair quality and character across individual factors, such as race, ethnicity, age, sex, and disease.2,11,30,31,32 Studies of hair growth have been
reported on exceptionally few children and focused on keratinization, medullation, or diameter/cross-sectional growth patterns.22,33,34,35,36 Human scalp hair, derived from the neuroectoderm
and mesoderm,34,37 evolves via prenatal lanugo, postnatal vellus, intermediate medullary, and post-pubertal terminal hair stages.24,25,38 These studies also revealed that: (a) scalp hair
medullation increases diameter with the greatest increases occurring at 1–3 years of age and no sex differences in hair diameter; (b) males have greater hair density (follicles/cm2) and
faster linear hair growth rates at 3–9 years.22,24,36 Linear hair growth rates in adults are correlated with hair diameter, medullation, and loss of pigmentation; microscopically correlated
with the hair follicle size, and interscale distances within the hair shaft.27,39,40 While these are important observations, linear hair growth rates (HGRs) are required to accurately
interpret the time frame during which biomarkers are incorporated into the growing hair shaft, and linear HGRs remain undetermined in children. Current hair biomarker research practices
involve the application of adult HGRs to the interpretation of biomarker levels in children.11,41 Rapid physical growth and development in childhood may alter HGRs; thus, child HGRs may
differ from HGRs reported in adults.27 For example, differing biomarker (cortisol) levels in adults vs. children demonstrated by Neumann et al. and de Kruijff et al. may be due to
age-dependent differences in HGRs.42,43 Race-dependent differences in hair growth linked to hair color, texture, biochemical composition, and hair growth cycles are determined in adults
(specifically in African, Asian, European/Caucasian groups), but remain undetermined in children.44 Child hair biomarker studies have described analogous race-dependent differences linked to
hair qualities.11,38 Furthermore, Barth and Pecoraro et al. reported higher HGRs in male vs. female children (5–15% faster hair growth; 340 vs. 302 μm/day, respectively), but both examined
small sample sizes and only performed descriptive statistical analyses.38,45 On the whole, adult hair studies provide biomarker research with more sophisticated findings (more studies,
larger sample sizes, rigorous methodologies, and statistical analyses) than child hair studies. For example, while the composition of adult hair is known (0.25–0.95% trace elements,1–9%
lipids, 3–5% endogenous water, and 65–95% protein),46,47,48,49,50 the composition of children’s hair largely remains unknown. We designed this study to understand hair growth physiology in
early childhood, specifically investigating anthropometric measures and demographic factors that determine linear HGRs in preschool children. Results from this study will enable better
interpretation of the timeframe in which biomarkers are incorporated into children’s growing hair so that appropriate adjustments can be made to hair biomarker levels associated with
environmental exposures and the mind-brain-body connectome. MATERIALS AND METHODS STUDY COHORT Following approval from the Stanford University IRB, parents or guardians gave informed consent
for participation in the Hair Biomarkers Study (https://childwellness.stanford.edu/hair-biomarkers-study). Healthy preschool children aged 9–72 months (_N_ = 266) from Santa Clara County,
CA were enrolled in a 3-week longitudinal observational study to evaluate HGRs during the period of November 2017 to February 2020. Subjects with tinea capitis, alopecia areata, eczema, or
other scalp conditions, or those receiving any prescription or other drugs (except daily vitamins) were excluded. Subjects receiving systemic steroid therapy, or those with a history of
chronic medical conditions such as cystic fibrosis, sickle cell disease, congenital heart disease, or other disorders, and subjects with chemical exposures (e.g., dying, bleaching, chemical
straightening, perming) to their hair in the 3 months prior to study entry were also excluded. Qualitative assessment of social and demographic factors confirmed that our study sample only
included healthy children from nuclear families free from adversity. The COVID-19 pandemic limited the enrollment and in-person contact with several participants for serial HGR measurements.
DETERMINANTS OF HAIR GROWTH Hair samples, demographic information, and anthropometric measures were collected at seven participating sites. Parents were asked to classify their child’s race
into racial categories recognized by the Federal government (White, Asian, African American, Alaska Native, or Pacific Islander). Ethnicity was assigned in accordance with the Department of
Homeland Security’s code of ethnicities (based on the geographic location of birth, namely, Africa, Asia, Caribbean, Central America, Europe, North America, Oceania, and South America).
Subjects were designated as “Mixed” if maternal and paternal race or ethnicity differed, assigned to the “Other” race group if they were identified as African American, Native Alaskan, or
Pacific Islander, and into the “Other” ethnicity group if they were identified as African, Caribbean, or Oceanian. To assess nutritional status, we measured the child’s height, head, and
waist circumferences. We classified children into three hair pigment groups (light, medium, and dark hair) using a modification of Loussouarn’s methodology, which involved matching hair
color to a reference scale compromised of a 10-unit gradient ranging from black (1) to pale blonde (10).27 Dark pigmentation included black/brown, black and dark black hair colors (pigments
1–3). Medium pigmentation included brown/red, light brown, brown and dark brown hair colors (pigments 4–7). Light pigmentation included gradients from pale blonde, blonde/brown and
blonde/red hair colors (pigments 8–10). Hair sampling seasons (Spring, Summer, Autumn, Winter) were identified by dates of the two solstices and two equinoxes to assess seasonal changes in
hair growth. HAIR SAMPLING Researchers were trained to trim a 1 cm2 area near the posterior vertex using a Philips Norelco Multigroom 3000 trimmer®, which cuts hair at 0.1 mm from the scalp.
Fine digital calipers (Fisher Scientific) were used to measure the length of new hair at weekly follow-up visits. The same researcher who obtained hair measured the hair growth at weekly
appointments across 7–21 days. Hair growth was averaged based on serial measurements of 3–5 hairs. The difference in hair length from the previous measurement was divided by the number of
days between measurements to calculate the averaged hair growth rate (HGR) (μm/day). HAIR PROTEIN EXTRACTION AND MEASUREMENT The distal end of hair was taped and the proximal (scalp) end was
indicated by an arrow on a folded paper containing the sample. The hair sample was placed into a zip-lock bag, labeled, and stored at 4 °C until processing.12 The proximal 0–3 cm length of
hair was weighed (10–50 mg) in a glass vial, finely cut to a powdery consistency, and processed with a four-step extraction procedure, with alternating two cycles of hair extraction in 1 ml
of methanol (15 h, at 52 °C, rotating 200 rpm) followed by 1 ml acetone (5 min, at room temperature, rotating 200 rpm). Samples were centrifuged, supernatants removed and air dried at 4 °C.
Dried residue was reconstituted with phosphate buffered saline (70 μl/10 mg hair) with transfer to microcentrifuge tubes. Samples were cleaned by cold (4 °C) centrifugation, 12,000 rpm for
15 min, and the supernatant was transferred to fresh tubes. The soluble hair protein content (HPC) was measured by spectrophotometric absorption at 260/280 (Take-3, Epoch plate reader,
Gen5.5 software, BioTek Instruments). STATISTICAL METHODS Descriptive statistics were used to summarize demographic characteristics and anthropometric measures for children enrolled. These
variables were summarized using means with standard deviations or medians with interquartile ranges for continuous variables, frequencies and percentages for categorical variables. HGRs for
each follow-up visit were averaged to obtain an overall mean HGR and then summarized by age group, sex, race, height tertiles, age-adjusted height tertiles, hair pigmentation, and hair
sampling season. ANOVA and _post hoc_ two-stage linear step-up procedure of Benjamini, Krieger and Yekutieli was performed to assess whether HGRs differed by age, sex, race, height,
age-adjusted height, hair pigment, or season. To examine associations between HGR and factors of interest, we performed several simple and multiple linear regression models. Simple linear
regression was used to model the bivariate relationship between HGR and each factor. Multiple linear regression was used to examine the association between HGR and all factors
simultaneously. Data were fitted to a linear regression model that included age, sex, race, height, hair pigmentation, and hair sampling season (Model 1) and HPC was added in Model 2.
Multiple imputations using fully conditional specification were used to impute any missing independent covariates used in these models. We created ten imputed datasets, and estimates were
combined using Rubin’s rules. The ‘type3_MI_glm’ macro developed by Wang et al. was used to generate p-values from type-III analyses.51 All analyses were conducted using SAS 9.4 (SAS
Institute, Inc., Cary, NC) and R (version 4.1.2.; R Foundation for Statistical Computing, Vienna, Austria). All tests were two-sided and evaluated using an alpha-error of 0.05. RESULTS Table
1 shows child demographics and measurements for the study sample (_N_ = 266). Participants had an average age 3.5 ± 1.3 years, height 101.2 ± 12.2 cm, head circumference 49.7 ± 3.0 cm, and
waist circumference 51.2 ± 4.5 cm. Nearly half of the subjects (44.7%) were White, while the remaining subjects were Asian (25.2%), Mixed (16.5%), and Other (13.5%, including African
American, Pacific Islander, and Alaska Native). Most subjects belonged to non-Hispanic (88.7%) ethnicity. We studied similar numbers of male (54.1%) and female (45.9%) subjects and subjects
with light (27.8%), medium (39.5%), and dark (32.7%) hair. Table 2 shows the distribution of sex, race, hair pigment, and anthropometric measures for children within each age group
(<1-year-old, 1-, 2-, 3-, 4-, and 5-year-old children). HAIR GROWTH RATES The average HGR ranged from 287.9 ± 60.7 μm/day in infants less than 1 year old to 345.1 ± 47.2 μm/day in
3-year-old children. HGR rates differed among age groups, with infants less than 1 year old showing slower HGRs than 2-, 3-, 4-, and 5-year-old children (all _p_ < 0.05, Table 3). We
found no sex differences in HGRs (female 338.4 ± 47.2 μm/day vs. male 340.7 ± 50.4 μm/day; _p_ = 0.71). While HGR was not associated with waist or head or arm circumferences, subjects in the
top tertiles for height and age-adjusted height had higher HGRs than those in the bottom tertile (_p_ = 0.0025 and _p_ = 0.04, respectively, Table 3). Subjects with dark hair pigment (352.4
± 51.6 μm/day) had higher HGRs compared to subjects with light hair pigment (325.3 ± 50.0 μm/day; _p_ = 0.0019, Table 3). Asian subjects had the highest HGRs among all races and
significantly higher HGR compared to White subjects (355.6 ± 50.3 μm/day vs. 332.1 ± 48.5 μm/day, respectively, _p_ = 0.016, Table 3). HGRs differed across the age groups for White children
(_p_ = 0.0026) but not Asian children (_p_ = 0.19), and were inconclusive for “Mixed” and “Other” race groups. No HGR differences were observed between the four seasons. Table 4 presents the
results of linear regression analyses. Consistent with the findings from ANOVA in Table 3, simple linear regression showed significant relationships between HGR and age (_p_ = 0.0073),
height (_p_ = 0.0025), race (_p_ = 0.016) and hair pigmentation (_p_ = 0.0019). Age (_p_ = 0.0066) and height (_p_ = 0.005) remained significant when other factors were adjusted in the
linear regression model (Model 1). Model 1 explained 15% of the variation in children’s HGR. With addition of hair protein content (HPC) to the model (Model 2), significant differences in
HGR were seen for age (_p_ = 0.018), height (_p_ = 0.0073) and HPC (_p_ = 0.013), whereas hair pigmentation had marginal effects (_p_ = 0.062). Model 2 explained 17% of the variation in HGR
and fit the data better (_p_ = 0.01) as compared to Model 1. An R-squared of 0.17 corresponds to Cohen’s effect size F-squared of 0.20, which based on Cohen’s 1988 criteria, indicates a
medium effect size for HGR variability in children. We also tested the assumptions for both linear models. As shown in Fig. 1, the density plots and scatterplots of studentized residuals for
hair growth rates followed a linear pattern for both Models 1 and 2, showing that the linearity and the equal variance assumptions were being met.52 In addition, the residuals for both
models were not skewed, because all points in the Quantile-Quantile plots (Q-Q plots) were distributed along the 45-degree diagonal reference line, further substantiating that the normality
assumption was satisfied (Fig. 1).52 HPC was negatively correlated to HGR (_r_ = –0.18, _p_ = 0.001) and significantly altered by age, showing higher values in infants below 1 year compared
to children at older ages (_p_ = 0.0014, Fig. 2a). There were no differences in HPC between children grouped by race (_p_ = 0.2261). HGRs were lower in the youngest children and
significantly higher at 2–5 years (_p_ = 0.0073, Fig. 2b). Given these findings and improvement in the HGR regression model after adding HPC, we adjusted each child’s HGR by their measured
hair protein content. The ratio of HPC to HGR revealed the highest levels of hair protein synthesis below age 1 year with steady decline through to age 5 (_p_ = 0.0001, Fig. 2c). DISCUSSION
This is the first study to determine potential covariates of linear hair growth in preschool children. Linear HGRs in preschool children are age-dependent with overall increases in the HGR
as children age; HGRs are lowest in children under 1 year of age and highest in children aged 3–5 years. These findings are consistent with the findings of Barth and de Kruijff et al., who
reported lower HGRs in children below 2 years of age.38,42 Furthermore, age explains a moderate degree of HGR variance when comparing adult vs. child HGRs from Asian, European, and North
American groups (30, 39, and 55% variance, respectively; see Supplementary). There are several plausible explanations for the age-dependent differences in HGRs. First, newborn hair has much
less medulla than infant hair, and the degree of medullation, which is associated with the anagen phase of hair growth, increases throughout childhood.22 Furthermore, it is hypothesized that
the four types of infant hair (i.e., lanugo in newborns, vellus hair, an intermediate form of hair, followed by terminal hair) grow at different rates.53 Second, around the first year of
life the hair growth cycle transitions from a state of synchronous to asynchronous activity in the hair follicles.38,54 This transition results in variable hair loss and significant
differences in the percentage of terminal hair follicles; subsequently, there is a transient decrease in HGR around 1 year of age. Once hair growth has transitioned from synchronous to
asynchronous cycles, hairs are uniformly distributed to all stages of the growth cycle, which results in a relative net increase in HGR (when compared to the transition period) in older
children—this is maintained unless disrupted by ageing, hormonal changes, or illness.19,20,38,55,56,57 When comparing within ethnicities, child HGRs observed in our study differ from
published adult HGR ranges.27 The discrepancy between adult and child HGRs may promote inaccurate interpretations of biomarker levels; thus, we caution investigators applying adult linear
HGRs for defining the time-period of biomarker incorporation into children’s hair. For example, variations in linear HGRs (child vs. adult) may partially account for the higher hair cortisol
levels observed in young children as compared to adults.11,42,43 Our study also determined that race, hair pigmentation, height, and age-adjusted height are covariates of HGR in preschool
children. Specifically, HGRs were higher in Asian vs. White children, those with dark vs. light pigmented hair, and in the taller children. Examination of the age-dependent inverse
relationship between HGR and HPC (i.e., younger children have lower HGR and higher HPC) via the HPC to HGR ratio revealed that hair protein synthesis is highest in children below 1 year of
age and decreases as children get older. One plausible explanation for these findings may be that the protein utilization required for increased height growth velocity may lead to lower HPC
in older/taller children, although further research directly comparing the rates of total body protein synthesis with hair protein synthesis will be required to assess this relationship. In
adults, decreases in hair pigmentation are associated with decreases in telogen (resting phase) density, with likely increases in the proportion of anagen hair follicles in adults with
lighter color hair.27 Longitudinal analyses indicate that cycling of hair follicles is independent of linear HGRs in adults.56 Therefore, even if children with light-colored hair had a
higher proportion of anagen hairs, that does not contradict our finding of lower HGRs in these children. There is limited data on the effect of malnutrition on hair growth rates in preschool
children.58 Our findings did not indicate an association between waist circumference and HGR. However, our study protocol included a healthy population at low risk of malnutrition. No other
published data on anthropometric measures and HGRs was identified, therefore, future studies enrolling at-risk populations may provide insight on the undetermined association of HGR and
other anthropometric measures in preschool children. Strengths of this study include a larger and more diverse sample than all prior studies on hair growth. We also studied a broad range of
potential covariates and identified several HGR-related factors in preschool children. Thus, our study provides determinants of linear HGRs for previously understudied preschool children.
Weaknesses of this study include the uneven distribution of participants from different racial/ethnic groups. Very few subjects identified as Pacific Islander, Alaska Native, or African
American; these subjects were collectively assigned to the “Other” group. Uneven distribution of boys and girls also occurred in the <1-year age group, though HGRs did not differ across
sex in this or other age groups. Lack of hair quality assessment (given the established racial and ethnic differences in hair qualities, such as texture, form, and thickness) was another
weakness of our study.27,35,38,59,60 We advise that future reference studies must include diverse populations and incorporate objective metrics for evaluating hair qualities, so that
meaningful associations between hair growth and race/ethnicity can be investigated to facilitate the interpretation of hair biomarkers in children. CONCLUSIONS Compared to the existing
literature on linear hair growth in children, our data provides potential covariates of hair growth in a racially and ethnically diverse population of preschool children. Determinants of
linear HGR in preschool children will be essential to implement biomarker testing and to accurately interpret their results in pediatric research and clinical practice. Our study reveals
age, race, hair pigment (color), and height as covariates of HGRs in preschool children; studies measuring hair biomarkers should account for these determinants. Moreover, our initial
observations on hair protein content and HGRs may provide physiological explanations for differences in HGRs and biomarkers in preschool children as compared to adults. DATA AVAILABILITY The
datasets generated during and/or analyzed during the current study are available from the corresponding author on request. REFERENCES * Niebel, A., Pragst, F., Krumbiegel, F. & Hartwig,
S. Prevalence of cathinones and other new psychoactive substances in hair of parents and children of families with known or suspected parental abuse of conventional illegal drugs. _Forensic
Sci. Int._ 331, 111148 (2022). Article CAS PubMed Google Scholar * Bhattarai, D. et al. Hair microscopy: an easy adjunct to diagnosis of systemic diseases in children. _Appl. Microsc._
51, 18 (2021). Article CAS PubMed PubMed Central Google Scholar * Plott, T. J. et al. Age-related changes in hair shaft protein profiling and genetically variant peptides. _Forensic
Sci. Int. Genet._ 47, 102309 (2020). Article CAS PubMed PubMed Central Google Scholar * Leonhard, M. J., Chang, E. T., Loccisano, A. E. & Garry, M. R. A systematic literature review
of epidemiologic studies of developmental manganese exposure and neurodevelopmental outcomes. _Toxicology_ 420, 46–65 (2019). Article CAS PubMed Google Scholar * Fabelova, L. et al.
Hair concentration of trace elements and growth in homeless children aged <6years: results from the enfams study. _Environ. Int._ 114, 318–325 (2018). Article CAS PubMed Google Scholar
* Pineiro, X. F. et al. Heavy metal contamination in Peru: implications on children’s health. _Sci. Rep._ 11, 22729 (2021). Article CAS PubMed PubMed Central Google Scholar *
Takeuchi, H. et al. Lead exposure is associated with functional and microstructural changes in the healthy human brain. _Commun. Biol._ 4, 912 (2021). Article CAS PubMed PubMed Central
Google Scholar * Lyon-Caen, S. et al. Deciphering the impact of early-life exposures to highly variable environmental factors on foetal and child health: design of sepages couple-child
cohort. _Int. J. Environ. Res. Public Health_ 16, 1–29 (2019). * Perng, W. et al. Early life exposure in Mexico to environmental toxicants (element) project. _BMJ Open_ 9, e030427 (2019).
Article PubMed PubMed Central Google Scholar * DePasquale, C. E. et al. Lifetime stressors, hair cortisol, and executive function: age-related associations in childhood. _Dev.
Psychobiol._ 63, 1043–1052 (2021). Article CAS PubMed Google Scholar * Gray, N. A. et al. Determinants of hair cortisol concentration in children: a systematic review.
_Psychoneuroendocrinology_ 87, 204–214 (2018). Article CAS PubMed Google Scholar * Slominski, R., Rovnaghi, C. R. & Anand, K. J. S. Methodological considerations for hair cortisol
measurements in children. _Ther. Drug Monit._ 37, 812–820 (2015). Article CAS PubMed PubMed Central Google Scholar * Anand, K. J. S. et al. Measuring socioeconomic adversity in early
life. _Acta Paediatr._ 108, 1267–1277 (2019). Article PubMed PubMed Central Google Scholar * Anand, K. J. S. et al. Demographic and psychosocial factors associated with hair cortisol
concentrations in preschool children. _Pediatr. Res._ 87, 1119–1127 (2020). Article PubMed Google Scholar * Lakshmi Priya, M. D. & Geetha, A. A biochemical study on the level of
proteins and their percentage of nitration in the hair and nail of autistic children. _Clin. Chim. Acta_ 412, 1036–1042 (2011). Article CAS PubMed Google Scholar * Jursa, T., Stein, C.
R. & Smith, D. R. Determinants of hair manganese, lead, cadmium and arsenic levels in environmentally exposed children. _Toxics_ 6, 1–15 (2018). * Gareri, J. & Koren, G. Prenatal
hair development: implications for drug exposure determination. _Forensic Sci. Int_. 196, 27–31 (2010). Article CAS PubMed Google Scholar * Courtois, M., Loussouarn, G., Hourseau, S.
& Grollier, J. F. Periodicity in the growth and shedding of hair. _Br. J. Dermatol._ 134, 47–54 (1996). Article CAS PubMed Google Scholar * Grymowicz, M. et al. Hormonal effects on
hair follicles. _Int. J. Mol. Sci._ 21, 5342 (2020). Article CAS PubMed PubMed Central Google Scholar * Pierard-Franchimont, C., Petit, L., Loussouarn, G., Saint-Leger, D. &
Pierard, G. E. The hair eclipse phenomenon: sharpening the focus on the hair cycle chronobiology. _Int J. Cosmet. Sci._ 25, 295–299 (2003). Article CAS PubMed Google Scholar * Van Neste,
D. J., de Brouwer, B. & De Coster, W. The phototrichogram: analysis of some technical factors of variation. _Ski. Pharm._ 7, 67–72 (1994). Article Google Scholar * Duggins, O. H.
& Trotter, M. Age changes in head hair from birth to maturity. Ii. Medullation in hair of children. _Am. J. Phys. Anthropol._ 8, 399–415 (1950). Article CAS PubMed Google Scholar *
Duggins, O. H. & Trotter, M. Changes in morphology of hair during childhood. _Ann. N. Y Acad. Sci._ 53, 569–575 (1951). Article CAS PubMed Google Scholar * Trotter, M. & Duggins,
O. H. Age changes in head hair from birth to maturity; index and size of hair of children. _Am. J. Phys. Anthropol._ 6, 489–506 (1948). Article CAS PubMed Google Scholar * Trotter, M.
& Duggins, O. H. Age changes in head hair from birth to maturity. _Am. J. Phys. Anthropol._ 8, 467–484 (1950). Article CAS PubMed Google Scholar * Liu, C. et al. Changes in Chinese
hair growth along a full year. _Int. J. Cosmet. Sci._ 36, 531–536 (2014). Article CAS PubMed Google Scholar * Loussouarn, G. et al. Diversity in human hair growth, diameter, colour and
shape. an in vivo study on young adults from 24 different ethnic groups observed in the five continents. _Eur. J. Dermatol_. 26, 144–154 (2016). Article CAS PubMed Google Scholar *
Randall, V. A. & Ebling, F. J. Seasonal changes in human hair growth. _Br. J. Dermatol_. 124, 146–151 (1991). Article CAS PubMed Google Scholar * Eerkens, J. W., Ryder, A., Evoy, A.
& Hull, B. Hydrogen isotopes in serial hair samples record season of death in a mummified child from 19th century San Francisco, Ca. _Am. J. Phys. Anthropol._ 173, 606–614 (2020).
Article PubMed Google Scholar * Commo, S. et al. Age-dependent changes in eumelanin composition in hairs of various ethnic origins. _Int J. Cosmet. Sci._ 34, 102–107 (2012). Article CAS
PubMed Google Scholar * Sarifakioglu, E., Yilmaz, A. E., Gorpelioglu, C. & Orun, E. Prevalence of scalp disorders and hair loss in children. _Cutis_. 90, 225–229 (2012). PubMed
Google Scholar * Khumalo, N. P. & Gumedze, F. African hair length in a school population: a clue to disease pathogenesis? _J. Cosmet. Dermatol_. 6, 144–151 (2007). Article CAS PubMed
Google Scholar * Alves, R. & Grimalt, R. Hair loss in children. _Curr. Probl. Dermatol_. 47, 55–66 (2015). Article PubMed Google Scholar * Dawber, R. P. The embryology and
development of human scalp hair. _Clin. Dermatol_. 6, 1–6 (1988). Article CAS PubMed Google Scholar * Das-Chaudhuri, A. B. & Chopra, V. P. Variation in hair histological variables:
medulla and diameter. _Hum. Hered._ 34, 217–221 (1984). Article CAS PubMed Google Scholar * Wynkoop, E. M. A study of the age correlations of the cuticular scales, medullas, and shaft
diameters of human head hair. _Am. J. Phys. Anthropol._ 13, 177–188 (1929). Article Google Scholar * Smith, D. W. & Gong, B. T. Scalp hair patterning as a clue to early fetal brain
development. _J. Pediatr._ 83, 374–380 (1973). Article CAS PubMed Google Scholar * Barth, J. H. Normal hair growth in children. _Pediatr. Dermatol_. 4, 173–184 (1987). Article CAS
PubMed Google Scholar * Baque, C. S. et al. Relationships between hair growth rate and morphological parameters of human straight hair: a same law above ethnical origins? _Int J. Cosmet.
Sci._ 34, 111–116 (2012). Article PubMed Google Scholar * Van Neste, D. Thickness, medullation and growth rate of female scalp hair are subject to significant variation according to
pigmentation and scalp location during ageing. _Eur. J. Dermatol_. 14, 28–32 (2004). PubMed Google Scholar * Kirschbaum, C., Tietze, A., Skoluda, N. & Dettenborn, L. Hair as a
retrospective calendar of cortisol production-increased cortisol incorporation into hair in the third trimester of pregnancy. _Psychoneuroendocrinology_ 34, 32–37 (2009). Article CAS
PubMed Google Scholar * de Kruijff, I. et al. Lc-Ms/Ms-based reference intervals for hair cortisol in healthy children. _Psychoneuroendocrinology_ 112, 104539 (2020). Article PubMed
Google Scholar * Neumann, A. et al. Predicting hair cortisol levels with hair pigmentation genes: a possible hair pigmentation bias. _Sci. Rep._ 7, 8529 (2017). Article PubMed PubMed
Central Google Scholar * Buffoli, B. et al. The human hair: from anatomy to physiology. _Int J. Dermatol_. 53, 331–341 (2014). Article PubMed Google Scholar * Pecoraro, V., Astore, I.,
Barman, J. & Ignacioaraujo, C. The normal trichogram in the child before the age of puberty. _J. Invest Dermatol_. 42, 427–430 (1964). Article CAS PubMed Google Scholar * Nicolaides,
N. & Rothman, S. Studies on the chemical composition of human hair fat. I. The squalene-cholesterol relationship in children and adults. _J. Invest Dermatol_. 19, 389–391 (1952).
Article CAS PubMed Google Scholar * Adav, S. S. et al. Studies on the proteome of human hair—identification of histones and deamidated keratins. _Sci. Rep._ 8, 1599 (2018). Article
PubMed PubMed Central Google Scholar * Cruz, C. F. et al. Keratins and lipids in ethnic hair. _Int J. Cosmet. Sci._ 35, 244–249 (2013). Article CAS PubMed Google Scholar * Nicolaides,
N. & Rothman, S. Studies on the chemical composition of human hair fat. II. The overall composition with regard to age, sex and race. _J. Invest Dermatol_. 21, 9–14 (1953). Article CAS
PubMed Google Scholar * Robbins, C. R. _Chemical and Physical Behavior of Human Hair: Chapter 2. Chemical Composition of Different Hair Types_ 4th edn, 105–176 (Springer, 2002). * Wang,
B., Fang, Y. & Jin, M. Combining type-III analyses from multiple imputations. _SAS J._ 14, 1543 https://Support.Sas.Com/Resources/Papers/Proceedings14/1543-2014.Pdf (2015). Google
Scholar * Mishra, P. et al. Descriptive statistics and normality tests for statistical data. _Ann. Card. Anaesth._ 22, 67–72 (2019). Article PubMed PubMed Central Google Scholar *
Janniger, C. K. & Bryngil, J. M. Hair in infancy and childhood. _Cutis_ 51, 336–338 (1993). CAS PubMed Google Scholar * Furdon, S. A. & Clark, D. A. Scalp hair characteristics in
the newborn infant. _Adv. Neonatal Care_ 3, 286–296 (2003). Article PubMed Google Scholar * Courtois, M., Loussouarn, G., Hourseau, C. & Grollier, J. F. Ageing and hair cycles. _Br.
J. Dermatol_. 132, 86–93 (1995). Article CAS PubMed Google Scholar * Halloy, J., Bernard, B. A., Loussouarn, G. & Goldbeter, A. Modeling the dynamics of human hair cycles by a
follicular automaton. _Proc. Natl Acad. Sci. USA_ 97, 8328–8333 (2000). Article CAS PubMed PubMed Central Google Scholar * Thom, E. Stress and the hair growth cycle: cortisol-induced
hair growth disruption. _J. Drugs Dermatol_. 15, 1001–1004 (2016). CAS PubMed Google Scholar * Neuberger, F. M., Jopp, E., Graw, M., Puschel, K. & Grupe, G. Signs of malnutrition and
starvation–reconstruction of nutritional life histories by serial isotopic analyses of hair. _Forensic Sci. Int._ 226, 22–32 (2013). Article CAS PubMed Google Scholar * Morgan, M. D. et
al. Genome-wide study of hair colour in Uk Biobank explains most of the SNP heritability. _Nat. Commun._ 9, 5271 (2018). Article PubMed PubMed Central Google Scholar * De la Mettrie, R.
et al. Shape variability and classification of human hair: a worldwide approach. _Hum. Biol._ 79, 265–281 (2007). Article PubMed Google Scholar Download references ACKNOWLEDGEMENTS We
thank Grace K-Y. Tam, Clinical Research Coordinator, Pain/Stress Neurobiology Laboratory, and Dr. Sukyung Chung, Quantitative Sciences Unit, Stanford University School of Medicine, Stanford,
CA for their contributions to this study and to earlier versions of this manuscript. FUNDING Grants from the _Eunice Kennedy Shriver_ National Institute for Child Health & Human
Development (R01 HD099296) and the Maternal & Child Health Research Institute to KJSA supported this study. Study sponsors had no role in the design and conduct of the study; the
collection, management, analysis, or interpretation of the data; the preparation, review, approval, or decision to publish this manuscript. AUTHOR INFORMATION Author notes * Mónica O. Ruiz
Present address: Department of Pediatrics, Brown University School of Medicine, Rhode Island Hospital & Hasbro Children’s Hospital, Providence, RI, USA AUTHORS AND AFFILIATIONS *
Department of Pediatrics, Stanford University School of Medicine, Stanford, CA, USA Mónica O. Ruiz, Cynthia R. Rovnaghi & Kanwaljeet J. S. Anand * Stanford Child Wellness Lab, Maternal
& Child Health Research Institute, Stanford, CA, USA Mónica O. Ruiz, Cynthia R. Rovnaghi, Sahil Tembulkar, Leni Truong & Kanwaljeet J. S. Anand * Quantitative Sciences Unit, Stanford
University School of Medicine, Stanford, CA, USA FeiFei Qin & Sa Shen * Department of Anesthesiology, Perioperative, and Pain Medicine, Stanford University School of Medicine, Stanford,
CA, USA Kanwaljeet J. S. Anand Authors * Mónica O. Ruiz View author publications You can also search for this author inPubMed Google Scholar * Cynthia R. Rovnaghi View author publications
You can also search for this author inPubMed Google Scholar * Sahil Tembulkar View author publications You can also search for this author inPubMed Google Scholar * FeiFei Qin View author
publications You can also search for this author inPubMed Google Scholar * Leni Truong View author publications You can also search for this author inPubMed Google Scholar * Sa Shen View
author publications You can also search for this author inPubMed Google Scholar * Kanwaljeet J. S. Anand View author publications You can also search for this author inPubMed Google Scholar
CONTRIBUTIONS M.O.R.: substantial contributions to data analysis and interpretation; drafting the article and revising it critically for important intellectual content; and final approval of
the version to be published. C.R.R.: substantial contributions to conception and design; data acquisition, analysis, and interpretation; drafting the article and revising it critically for
important intellectual content; and final approval of the version to be published. S.T.: substantial contributions to conception and design; acquisition of data; and final approval of the
version to be published. F.Q.: substantial contributions to data analysis and interpretation; and final approval of the version to be published. S.S.: substantial contributions to data
analysis and interpretation; and final approval of the version to be published. L.T.: substantial contributions to data acquisition and analysis; and final approval of the version to be
published. K.J.S.A.: Substantial contributions to conception and design; data acquisition, analysis and interpretation; drafting the article and revising it critically for important
intellectual content; securing grant funding for the project; and final approval of the version to be published. CORRESPONDING AUTHOR Correspondence to Mónica O. Ruiz. ETHICS DECLARATIONS
COMPETING INTERESTS The authors declare no competing interests. ETHICS APPROVAL AND CONSENT TO PARTICIPATE Parents/Guardians of child participants gave written consent to trained research
coordinators at the IRB-approved recruitment sites. ADDITIONAL INFORMATION PUBLISHER’S NOTE Springer Nature remains neutral with regard to jurisdictional claims in published maps and
institutional affiliations. SUPPLEMENTARY INFORMATION SUPPLEMENTARY MATERIAL RIGHTS AND PERMISSIONS Springer Nature or its licensor (e.g. a society or other partner) holds exclusive rights
to this article under a publishing agreement with the author(s) or other rightsholder(s); author self-archiving of the accepted manuscript version of this article is solely governed by the
terms of such publishing agreement and applicable law. Reprints and permissions ABOUT THIS ARTICLE CITE THIS ARTICLE Ruiz, M.O., Rovnaghi, C.R., Tembulkar, S. _et al._ Linear hair growth
rates in preschool children. _Pediatr Res_ 95, 359–366 (2024). https://doi.org/10.1038/s41390-023-02791-z Download citation * Received: 13 September 2022 * Revised: 13 July 2023 * Accepted:
15 August 2023 * Published: 04 September 2023 * Issue Date: January 2024 * DOI: https://doi.org/10.1038/s41390-023-02791-z SHARE THIS ARTICLE Anyone you share the following link with will be
able to read this content: Get shareable link Sorry, a shareable link is not currently available for this article. Copy to clipboard Provided by the Springer Nature SharedIt content-sharing
initiative