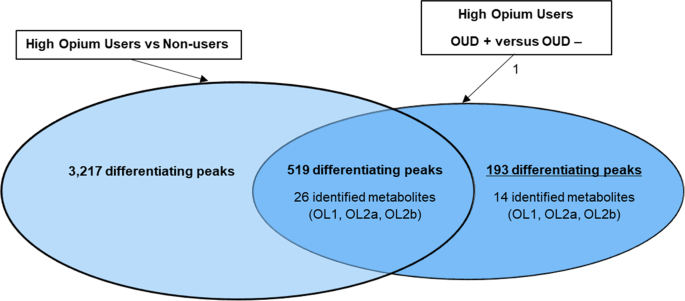
Metabolomics reveals biomarkers of opioid use disorder
- Select a language for the TTS:
- UK English Female
- UK English Male
- US English Female
- US English Male
- Australian Female
- Australian Male
- Language selected: (auto detect) - EN
Play all audios:

ABSTRACT Opioid use disorder (OUD) is diagnosed using the qualitative criteria defined by the Diagnostic and Statistical Manual of Mental Disorders, Fifth Edition (DSM-5). Diagnostic
biomarkers for OUD do not currently exist. Our study focused on developing objective biological markers to differentiate chronic opiate users with OUD from chronic opiate users without OUD.
Using biospecimens from the Golestan Cohort Study, we compared the metabolomics profiles of high opium users who were diagnosed as OUD positive with high opium users who were diagnosed as
OUD negative. High opium use was defined as maximum weekly opium usage greater than or equal to the median usage (2.4 g per week), and OUD was defined as having 2 or more DSM-5 criteria in
any 12-month period. Among the 218 high opium users in this study, 80 were diagnosed as OUD negative, while 138 were diagnosed as OUD positive. Seven hundred and twelve peaks differentiated
high opium users diagnosed as OUD positive from high opium users diagnosed as OUD negative. Stepwise logistic regression modeling of subject characteristics data together with the 712
differentiating peaks revealed a signature that is 95% predictive of an OUD positive diagnosis, a significant (_p_ < 0.0001) improvement over a 63% accurate prediction based on subject
characteristic data for these samples. These results suggest that a metabolic profile can be used to predict an OUD positive diagnosis. SIMILAR CONTENT BEING VIEWED BY OTHERS DRUG
REPURPOSING FOR OPIOID USE DISORDERS: INTEGRATION OF COMPUTATIONAL PREDICTION, CLINICAL CORROBORATION, AND MECHANISM OF ACTION ANALYSES Article 11 January 2021 SERUM CYTOKINES AND
INFLAMMATORY PROTEINS IN INDIVIDUALS WITH HEROIN USE DISORDER: POTENTIAL MECHANISTICALLY BASED BIOMARKERS FOR DIAGNOSIS Article Open access 03 October 2024 OPIOID AGONIST AND ANTAGONIST USE
AND THE GUT MICROBIOTA: ASSOCIATIONS AMONG PEOPLE IN ADDICTION TREATMENT Article Open access 10 November 2020 INTRODUCTION More than fifty years have passed since Dole and Nyswander
described opioid addiction as a metabolic disease, suggesting that opioids disrupt homeostasis to produce drug-seeking behavior in the face of adverse consequences1. An important issue in
the addiction is that people exposed to opioids may develop dependence, but not Opioid Use Disorder (OUD)2. OUD is a chronic recurrent disorder that increasingly causes undesirable emotional
states by involving the brain’s reward system and could include impaired social functioning3,4. Despite significant advances in the genetics and neurobiology of addiction as a brain
disease, and preliminary studies to discover biomarkers of OUD, validated systemic biomarkers for OUD do not exist5. Differential diagnosis of OUD is obtained through interview or
questionnaire to determine if the patients meet the DSM-5 diagnostic criteria. These criteria include impaired control, social impairment, risky use, tolerance, withdrawal, craving, and
continued use despite problems. Having at least two of the 11 criteria meets the diagnoses of OUD with the number of criteria met as an indicator of the severity of the OUD6. Iran is a
country with a high rate of opiate use. Opium is the main opiate used7. Our study focused on a random sample of opium users in the Golestan Cohort Study (GCS) in Iran, where more than 8400
individuals (about 17% of the participants) reported chronic opiate use with a median duration of use of 19 years8. 75% of the opium users in the full cohort used a combination of teriak and
shireh with only 4 people reporting heroin use8. We investigated urinary metabolomic profiles to reveal biomarkers that could differentiate high opium users who were diagnosed as OUD
positive from high opium users who were diagnosed as OUD negative. Our investigation is important because optimized treatment relies on accurate diagnosis of OUD. The DSM-5, the predominant
diagnostic instrument in psychiatry, has known limitations for diagnosis of substance abuse disorders9. Objective biological markers can improve the diagnosis that is currently based on
subjective DSM-5 questionnaire. In addition metabolites that are increased or decreased in opium users diagnosed with OUD (compared with opium users not diagnosed with OUD) can be used to
determine pathway perturbations, and lead to the identification of druggable or nutritional targets. MATERIALS AND METHODS STUDY POPULATION The details of the GCS (a cohort of over 50,000
adults aged 40–75 living in Golestan Province, Northeast Iran) have been previously published10. The GCS was approved by appropriate ethics committees at Tehran University of Medical
Sciences, the US National Cancer Institute (NCI, IRB# 07-C-N120), and the International Agency for Research on Cancer (IARC). In 2018, a random sample of 451 GCS participants who reported
long-term opium use and 92 never-users were recalled. They underwent a detailed interview using modified Persian and Turkman versions of the Section L of WHO Composite International
Diagnostic Interview (CIDI, version 2.1) to diagnose lifetime OUD7, based on the Diagnostic and Statistical Manual of Mental Disorders, 5th edition (DSM‐5). The presence of 2 or more of the
11 criteria during any 12-month period of life was defined as lifetime OUD. Variables considered for adjustment of the logistic regression models (see below) included age at enrollment,
gender, tobacco use (current/former/never), BMI, and route of opium use (ingestion/inhalation). OUD diagnosis was the outcome for the models. Alcohol use was not included in the analysis,
because it was rare in this population, and only 3.5% of participants reported ever using alcohol. No subjects participated in drug-related treatment for addiction as part of the GCS. Cohort
participants gave non-fasted spot urine samples which were stored at −20 °C until 2015 when they were transferred on dry ice to the NCI Biorepository and stored at −80 °C. Aliquots were
then shipped to UNC Chapel Hill. SAMPLE SELECTION In this sample selection, we excluded individuals who had discordant reports of their opiate use compared with baseline (baseline users who
reported no lifetime opium use at the recall visit and vice versa, _n_ = 24), and those without a urine sample available (_n_ = 8). We also restricted the current analysis to high opium
users reporting equal to or more than the median intake (2.4 g per week), to reduce the chance of misclassification. The final sample used in the current study included 138 urine samples
from high opium users who were diagnosed as OUD positive, and 80 urine samples from high opium users diagnosed as OUD negative. Urine samples were selected from an additional 80 subjects who
reported that they had never used opium. UNTARGETED METABOLOMICS VIA ULTRA-PERFORMANCE LIQUID CHROMATOGRAPHY (UPLC) HIGH-RESOLUTION MASS SPECTROMETRY Details of the sample preparation, data
acquisition, data preprocessing and metabolite identification and annotation are provided in the Supplementary Material Section. In brief, urine samples were prepared according to published
methods11, and untargeted metabolomics data were acquired on a Vanquish UHPLC systems coupled with a Q Exactive™ HF-X Hybrid Quadrupole-Orbitrap™ Mass Spectrometer (UPLC-HR-MS; Thermo
Fisher Scientific). Data were processed using Progenesis QI (Waters Corporation). Peaks detected by UPLC-HR-MS were identified or annotated. Signals detected on our untargeted platform are
matched to an in-house physical standards library that was developed by acquiring data for over 2000 chemical standards run under the same conditions to the study samples. The evidence basis
for metabolite identifications and annotations are based on matching to our in-house library physical standards library (Ontology Level, OL), as well as to Public Databases (PD), and are
detailed in the supplementary material. HYPOTHESIS TESTING Statistical tests for the normalized peaks in the metabolomics profiles were conducted using a two-tailed _t_-test with the
Satterthwaite correction for unequal variances or the chi-square test. Statistical analyses were conducted using SAS 9.4 (SAS Institute Inc., Cary, NC). In this exploratory metabolomics
study, _p_-values were not adjusted for multiple testing12,13. The nominal _p_-values are reported for the following comparisons 80 high opium users diagnosed as OUD negative versus 138 high
opium users diagnosed as OUD positive. LOGISTIC REGRESSION MODELING Logistic regression was used to model which peaks/metabolites were predictive of a positive OUD diagnosis. Several
modeling approaches were used that included all normalized metabolomics peaks, or included subsets of peaks. Stepwise logistic regression procedures (criteria: model entry _p_ < 0.1 and
model removal _p_ > 0.05) with standardization of continuous variables, was used for model selection. The Hosmer-Lemeshow goodness-of-fit test was used to assess the final model for
adequacy. Receiver operating characteristics (ROC) curve and the area under the curve (AUC) were used to evaluate metabolites as predictors of OUD. Stepwise models were conducted, with and
without subject characteristics as potential covariates, using: * (a) 712 peaks which differentiated high opium users who were diagnosed as OUD positive from high opium users who were
diagnosed as OUD negative. * (b) 40 identified/annotated metabolites that differentiated the high opium users who were diagnosed as OUD positive from high opium users who were diagnosed as
OUD negative (26 of these 40 metabolites also differentiated opium users from non-opium users). * (c) 14 identified/annotated metabolites that were unique to differentiation of high opium
users who were diagnosed as OUD positive from high opium users who were diagnosed as OUD negative (but did not also differentiate opium users from non-opium users). PATHWAY ENRICHMENT: HIGH
OPIUM USERS WHO WERE DIAGNOSED AS OUD POSITIVE FROM THE HIGH OPIUM USERS WHO WERE DIAGNOSED OUD AS NEGATIVE Pathway enrichment was conducted using the Mummichog software in Metaboanalyst
4.014. All features (_m_/_z_) remaining after filtering data were entered together with the _p_-value that was calculated for the comparison of high opium users who were diagnosed as OUD
positive and high opium users who were diagnosed OUD as negative. A _p_-value cut-off of 0.01 was used to determine the size of the permutation group that the algorithm used for selecting
significant features to match for all possible metabolites. A mass accuracy of 3 ppm was used as the threshold for annotations used in identifying candidate pathways. All possible
metabolites which were matched by _m_/_z_ were searched in the human reference metabolic network (hsa_m__fn_), and the null distribution of module activities was estimated by using 100
permutations of random lists drawn from the experimental reference feature list. The candidate pathways were based on the similarity of _m_/_z_. RESULTS SAMPLE CHARACTERISTICS The subject
characteristics for the 218 high Opium users who were diagnosed as OUD positive (138 subjects) or OUD negative (80 subjects) are provided in Table 1. For these study samples, the OUD
diagnosis was associated at _p_ < 0.1 with age at the time of enrollment (_p_ = 0.029, OUD positive were 2 years younger than OUD negative), and route of opium exposure (_p_ = 0.054,
higher by inhalation than by ingestion), but was not associated with BMI, gender, or tobacco use. METABOLIC PROFILES OF HIGH OPIUM USER DIAGNOSED AS OUD POSITIVE VERSUS HIGH OPIUM USER
DIAGNOSED AS OUD NEGATIVE Over 7714 UPLC-HRMS signals were obtained after data preprocessing. Hypothesis testing and fold change were determined for the normalized peaks in the metabolomics
data set for the comparison of (a) high opium users diagnosed as OUD positive vs high opium users diagnosed as OUD negative, and (b) opium users vs non-opium users. Over 700 peaks (712)
tested different by _t_-test (_p_ < 0.10) between high opium users diagnosed as OUD positive versus high opium users diagnosed as OUD negative (Fig. 1). Forty of the 712 peaks were
identified or annotated through matching to the in-house physical standards library (Table 2), while additional peaks were annotated using big data analytics (Table S1). PATHWAY ENRICHMENT
Pathway enrichment was conducted in Metaboanalyst14 using all 7714 peaks. A cut-off for pathway significance (_p_ < 0.01) was used to determine the size of the permutation group that the
algorithm used to determine the enrichment between high opium users diagnosed as OUD positive versus high opium users diagnosed as OUD negative. The candidate pathways based on the match of
exact mass (<3 ppm) of key metabolites that are included in the known pathway map are provided in Table S2. The distribution plot of the Enrichment Factor versus −log10 (_P_) is shown in
Fig. 2. High opium users diagnosed as OUD positive versus those diagnosed as OUD negative had an enrichment for pathways involving biotin (vitamin B7), folate (vitamin B9), cytochrome P450
metabolism, purine metabolism, keratan sulfate degradation, N-glycan degradation, and R group synthesis. _Vitamin_ absorption, bioavailability, and utilization are known to be impacted by
drug addiction15. _Cytochrome P450s_ are involved in the metabolism of opium, and the slow versus fast metabolism has been associated with addiction16. Opioid use has been shown to alter
_purine_ metabolism17. _Keratan sulfate_ is a glycosaminoglycan that is at significant levels in central and peripheral nervous systems18. _N-glycan_ is required to express the correctly
folded form of the delta-opioid receptor19. R group synthesis is associated with the FAD/FADH2 conversion of fatty acids. MODELING APPROACH 1 Stepwise logistic regression was used to
determine which of the 712 peaks were predictive of an OUD positive diagnosis. First, the area under the ROC curve (AUC) was calculated using the subject characteristics (Table 1) of age at
the time of enrollment and route of opium use. This base model resulted in an AUC of 0.625 (Figure S1a). Second, all 712 peaks that differentiated (_p_ < 0.10) the high opium users
diagnosed as OUD positive from high opium users diagnosed as OUD negative was modeled without including subject characteristics. This resulted in an AUC of 0.720 which was significantly
different (_p_ = 0.042) from the base model. Third, all 712 peaks that differentiated (_p_ < 0.10) the high opium users diagnosed as OUD positive from high opium users diagnosed as OUD
negative was modeled with age at the time of enrollment and opium use as covariates. This resulted in an AUC of 0.946, which was significantly increased (_p_ < 0.0001) over the base
model. Using this modeling approach, only 16 peaks were selected that were predictive of an OUD positive diagnosis (Table 3). Two of the 16 peaks matched to pterin (OL1) and tryptophan
(OL2b) using the in-house physical standards library. Annotations using public databases are provided for 6 additional peaks, while 8 of the peaks remained unknown unknowns. MODELING
APPROACH 2 Forty of the 712 peaks that differentiated (_p_ < 0.10) high opium users diagnosed as OUD positive from high opium users diagnosed as OUD negative could be matched to the
in-house physical standards library (Table 2, Fig. 1). Major differentiators included metabolites derived from opium use, tobacco use, involved in biopterins and vitamin B9, tryptophan
metabolism, acetylation of amino acids, bile acids, fatty acids, and carnitine metabolism. In addition, _N_-Acetyl-S-(3,4-dihydroxybutyl)-L-cysteine (_p_ = 0.023) and
_N_-Acetyl-S-(2-carbamoylethyl)-L-cysteine (_p_ = 0.083) were lower in urine of high opium users diagnosed as OUD positive vs high opium users diagnosed as OUD negative.
N-Acetyl-S-(3,4-dihydroxybutyl)-L-cysteine and N-Acetyl-S-(2-carbamoylethyl)-L-cysteine (_p_ = 0.083) are metabolic products of butadiene20 (BD) and acrylamide21 (AM), respectively. These
metabolites have previously been detected in biospecimens from tobacco users at significantly higher levels than non-tobacco users. They are attributed to the metabolism of the parent
compounds (AM and BD) that form during the curation process, or on combustion of tobacco22. They could also be formed in the curing and combustion of opium or other plant material. Stepwise
logistic regression using these 40 metabolites resulted in an AUC of 0.76, which was significantly increased (_p_ = 0.0049) over the base model (Figure S1b). Including subject
characteristics of age at the time of enrollment and route of opium exposure resulted in an AUC of 0.80, also significantly (_p_ < 0.0001) increased from the base model. Metabolites that
were predictive of an OUD positive diagnosis (Table 3) included tryptophan, pterine, sarcosine, N-acetylproline, azelate, octopamine, serine, and nicotine. METABOLIC PROFILES UNIQUE TO THE
OUD POSITIVE VERSUS OUD NEGATIVE DIAGNOSIS Five hundred and nineteen of the 712 peaks that tested different between OUD positive high opium users versus high OUD negative high opium users,
also differentiated the opium users from non-opium users (Fig. 1). To provide a focus on only metabolites that are important to the diagnosis of OUD, the 519 signals that were also important
to differentiation of opium users from non-opium users were excluded for this analysis. This resulted in 193 peaks unique to the differentiation (_p_ < 0.10) of subjects diagnoses as OUD
positive versus those diagnoses as OUD negative. Of these 193 peaks, only 14 peaks that defined the OUD diagnosis matched to the in-house physical standards library. These 14 metabolites
are listed in Table 2, while the additional annotated peaks through public databases are provided in Table S1. Eleven of the 14 peaks that matched to the in-house library that were most
important to defining OUD included the following endogenous metabolites: pterine (_p_ = 0.0011), 2,4-dihydroxypterine (_p_ = 0.0695), sarcosine (_p_ = 0.0263), phosphorylcholine (_p_ =
0.0962), 6-carboxyhexonate (_p_ = 0.021), lauroylcarnitine (0.0574), glycocholate (_p_ = 0.0816), 3-methylhistamine (0.087), azelate (_p_ = 0.0713), n-methyl-D-aspartic acid (_p_ = 0.0488),
and tryptophan (0.0378). The biological significance of these 11 endogenous metabolites is summarized: * (a) _Pterin_ is part of biopterin and folate. Biopterins are cofactors for aromatic
amino acid hydroxylases, which are involved in the synthesis of dopamine, norepinephrine, epinephrine, and serotonin, and trace amines23. The active form of folate (vitamin B9) is
tetrahydrofolate which accepts and donates one carbon unit (methyl group). _Dihydroxypteridine_ is involved in folate and riboflavin pathways24. * (b) _Phosphorylcholine_ is derived from
phosphorylation of choline25, and _sarcosine_ is an intermediate in the metabolism of choline to glycine26. * (c) _N-Methyl-d-aspartic acid_ (NMDA) is an agonist at the NMDA receptor and
mimics the action of glutamate27, and _tryptophan_ is in the neurotransmitter pathway28. _Azelaic acid_ (AZA) is a competitive inhibitor of tyrosinase in vitro29. * (d) _Lauroylcarnitine_ is
associated with fatty oxidation disorders involving acyl CoA dehydrogenase deficiency, and carnitine palmitoyltransferase I and II deficiency30. _6-Carboxyhexanoic acid_ is a medium-chain
fatty acid derived from heptanedioic acid and is involved in the gut microbial biosynthesis of biotin31. * (e) _Glycocholate_ is a secondary bile acid, produced in the microbial flora of the
colonic environment by bacteria32, and is absorbed and recirculated. Bile acids are important for absorption of hydrophobic nutrients, dietary fats and vitamins, and the regulation enzymes
involved in cholesterol homeostasis. * (f) _3-Methylhistamine_ is a prominent metabolite of histamine, which has a role in allergy, inflammation, gastric acid secretion, and
neurotransmission33. Three metabolites (Table 2) derived from exogenous exposures were also important to the differentiation of the high opium users who were diagnosed as OUD positive from
high opium users who were diagnosed as OUD negative. These included mono-isobutyl phthalate (_p_ = 0.0485, +) and mono ethyl hexyl phthalate (_p_ = 0.0852, +), which could arise as metabolic
products following ingestion of phthalates that leach from plastics used in inhalation of opium. N-Acetyl-S-(3,4-dihydroxybutyl)-L-cysteine was also a differentiator (_p_ = 0.0228, −), and
is presumably derived as a metabolic product of BD intake associated with the curing or combustion of plant matter. MODELING APPROACH 3 Stepwise logistic regression using the 14 metabolites
unique to the differentiation of OUD positive versus OUD negative, together with covariates of age at the time of enrollment and route of opium use resulted in an AUC of 0.751, which was
significantly increased (_p_ < 0.0005) over the base model (Figure S1c). Stepwise logistic regression using only the 14 metabolites (with no subject characteristics) resulted in an ACU of
0.706, which was not significantly increased (_p_ = 0.127) over the base model. Results from Model 3 (with or without the covariates of age at time of enrollment and route of opium use)
show 5 of the 14 metabolites (pterine, sarcosine, tryptophan, azelate, and N-Acetyl-S-(3,4-dihydroxybutyl)-L-cysteine) as predictive of a positive OUD (Table 3). DISCUSSION Our study
revealed metabolomics signatures of OUD in a predominantly Turkmen population of chronic high opium users. We provide metabolite identifications and annotations for 712 features detected
using untargeted mass spectrometry that are important to the differentiation of high opium users diagnosed as OUD positive from high opium users diagnosed as OUD negative. None of these
identifications are known metabolites derived from other drugs of abuse. Pathway enrichment analysis points to a general disruption in vitamin B9 (folate), vitamin B7 (biotin), cytochrome
P450, purine, and glycan metabolism, and FAD/FADH2 conversion of fatty acids. Stepwise logistic regression analysis of these 712 peaks, together with subject characteristics, resulted in 16
candidate peaks that predict 95% of the high opium users who were diagnosed as OUD positive. Forty of the 712 features which differentiate high opium users who were diagnosed as OUD positive
from high opium users who were diagnosed as OUD negative matched to an in-house physical standards library. Models constructed with only these 40 metabolites predicted 80% of the subjects
diagnosed as OUD positive, selecting 8 metabolites as predictors. Predictors of an OUD diagnosis in these high opium users included an increase in three endogenous compounds (pterine,
sarcosine, and octopamine), a decrease in four endogenous compounds (tryptophan, azelate, N-acetylproline, and serine), and an increase in nicotine. Fourteen metabolites were determined to
be unique to OUD diagnosis, after subtracting analytes known to overlap with opium use. Models using these 14 metabolites predicted 75% of the subjects testing OUD positive and replicated
pterine, sarcosine, tryptophan, and azelate as metabolite predictors. Many identified or annotated metabolites that differentiated high opium users who were OUD positive from high opium
users who were OUD negative play a significant role in neurotransmitter synthesis and signal transduction34. Tryptophan is the major amino acid precursor of serotonin (5HT). 5HT deficits
have been implicated in physical symptoms and emotional dysphoria following withdrawal from opioids35. Alterations in sarcosine, serine, kyneurate, NMDA found in this study are consistent
with the observations that glutamatergic signaling is disrupted by opioids36. Sarcosine (methyl-glycine) acts as an NMDA receptor agonist and a glycine receptor agonist37. Serine is
converted to D-serine by serine racemace. D-serine acts as co-agonist with glutamate to activate NMDA receptors38. Kyneurate a metabolite of tryptophan metabolized to quinolinic acid acts as
a NMDA receptor agonist39. Octopamine is a trace amine that is an agonist of TAAR1 receptors implicated in mediating the actions of drugs of abuse40. Methylhistamine is a histamine receptor
(H3) agonist that inhibits the firing of cholinergic neurons in the ventral striatum and decreases dopamine release41. Limitations of the current study include the (a) use of self-report
for the amount of opium consumed, (b) assumption that symptoms over any 12-month period-of-time are accurately recalled, (c) estimated amount/grams of opium may vary within or among regions,
and be underestimated42, (d) analyses were not stratified by the route of administration or type of opiate used due to the sample size, and because this paper focuses on a marker of OUD
independent of route, (e) that the current study was not powered for multiple testing, and (f) that the metabolomic profiles are not quantitated, or replicated in this cohort or across
cohorts. The year that the individuals met a DSM-5 OUD diagnosis during their history of opium use is unknown because the DSM-5 interview was not conducted at the time of baseline urine
collection. These urinary baseline metabolomic profiles presented herein could result from chronic opium use, and/or from inherent individual metabolic differences present prior to the
acquisition of OUD. Chronic use of opiates and opioids without meeting the criteria for DSM-5 OUD is not unique to the Turkman population for opiate use. Some chronic pain patients treated
with prescription opioids and chronic users of illicit opioids do not meet the criteria for DSM-5 OUD. This suggests that the approaches used in this study are likely to be generalizable to
other cohorts. This is also consistent with other substance use disorders where heavy use does not necessarily imply a substance used disorder43. Research on biomarkers for OUD and other
substance use disorders has focused on neuroimaging (MRI, fMRI, and PET) and EEG studies44. While these biomarkers may eventually be clinically validated in other populations, they will be
costly to implement. In contrast, validation of biomarkers in other populations in accessible biological fluids (e.g., urine, blood, saliva) will be less costly, and easier to implement in
general medical practice. In addition, these non-invasive biomarkers will be important complements to results from neuroimaging studies. In conclusion, if the current results are replicated,
the identification of peripheral biomarkers for OUD would represent a significant advancement in defining and managing the disease. It would further validate the Dole and Nyswander
hypothesis that OUD is a brain disease in which metabolism is disrupted, and would provide biomarkers for OUD that could be used to optimize treatment. In addition, validation of the
discovered metabolic perturbations related to vitamins and fatty acids could lead to the development of a nutrient cocktail to test in clinical settings for efficacy to mitigate symptoms
that lead to the diagnosis of OUD. REFERENCES * Dole, V. P. & Nyswander, M. E. Heroin addiction—a metabolic disease. _Arch. Intern. Med._ 120, 19–24 (1967). Article CAS PubMed Google
Scholar * Volkow, N. D. & Blanco, C. Medications for opioid use disorders: clinical and pharmacological considerations. _J. Clin. Invest._ 130, 10–13 (2020). Article CAS PubMed
Google Scholar * Strang, J. et al. Opioid use disorder. _Nat. Rev. Dis. Prim._ 6, 1–28 (2020). Google Scholar * Ruffle, J. K. Molecular neurobiology of addiction: what’s all the (Δ) FosB
about? _Am. J. Drug Alcohol Abus._ 40, 428–437 (2014). Article Google Scholar * Bough, K. J. & Pollock, J. D. Defining substance use disorders: the need for peripheral biomarkers.
_Trends Mol. Med._ 24, 109–120 (2018). Article PubMed Google Scholar * Hasin, D. S. et al. DSM-5 criteria for substance use disorders: recommendations and rationale. _Am. J. Psychiatry_
170, 834–851 (2013). Article PubMed PubMed Central Google Scholar * Amin-Esmaeili, M. et al. Epidemiology of illicit drug use disorders in Iran: prevalence, correlates, comorbidity and
service utilization results from the Iranian Mental Health Survey. _Addiction_ 111, 1836–1847 (2016). Article PubMed Google Scholar * Khademi, H. et al. Opium use and mortality in
Golestan Cohort Study: prospective cohort study of 50,000 adults in Iran. _BMJ_ 344, e2502 (2012). Article PubMed PubMed Central Google Scholar * Gorfinkel, L., Voon, P., Wood, E. &
Klimas, J. Diagnosing opioid addiction in people with chronic pain. _BMJ_ 362, k3949. https://doi.org/10.1136/bmj.k3949 (2018). * Pourshams, A. et al. Cohort Profile: The Golestan Cohort
Study-a prospective study of oesophageal cancer in northern Iran. _Int. J. Epidemiol._ 39, 52–59 (2010). Article PubMed Google Scholar * Want, E. J. et al. Global metabolic profiling
procedures for urine using UPLC–MS. _Nat. Protoc._ 5, 1005–1018 (2010). Article CAS PubMed Google Scholar * Xi, B., Gu, H. & Baniasadi, H. & Raftery, D. in _Mass Spectrometry in
Metabolomics_ 333–353 (Springer, 2014). * Bender, R. & Lange, S. Adjusting for multiple testing—when and how? _J. Clin. Epidemiol._ 54, 343–349 (2001). Article CAS PubMed Google
Scholar * Chong, J. et al. MetaboAnalyst 4.0: towards more transparent and integrative metabolomics analysis. _Nucleic Acids Res._ 46, W486–W494 (2018). Article CAS PubMed PubMed Central
Google Scholar * Grotzkyj-Giorgi, M. Nutrition and addiction-can dietary changes assist with recovery? _Drugs Alcohol Today_ 9, 24 (2009). Article Google Scholar * Kramlinger, V. M.,
Rojas, M. A., Kanamori, T. & Guengerich, F. P. Cytochrome P450 3A enzymes catalyze the O6-demethylation of thebaine, a key step in endogenous mammalian morphine biosynthesis. _J. Biol.
Chem._ 290, 20200–20210 (2015). Article CAS PubMed PubMed Central Google Scholar * Mannelli, P. et al. Opioid use affects antioxidant activity and purine metabolism: preliminary
results. _Hum. Psychopharmacol._ 24, 666–675 (2009). Article CAS PubMed PubMed Central Google Scholar * Caterson, B. & Melrose, J. Keratan sulfate, a complex glycosaminoglycan with
unique functional capability. _Glycobiology_ 28, 182–206 (2018). Article CAS PubMed PubMed Central Google Scholar * Markkanen, P. M. & Petäjä-Repo, U. E. N-glycan-mediated quality
control in the endoplasmic reticulum is required for the expression of correctly folded δ-opioid receptors at the cell surface. _J. Biol. Chem._ 283, 29086–29098 (2008). Article CAS PubMed
PubMed Central Google Scholar * Carmella, S. G. et al. Effects of smoking cessation on eight urinary tobacco carcinogen and toxicant biomarkers. _Chem. Res. Toxicol._ 22, 734–741 (2009).
Article CAS PubMed PubMed Central Google Scholar * Bjellaas, T., Janak, K., Lundanes, E., Kronberg, L. & Becher, G. Determination and quantification of urinary metabolites after
dietary exposure to acrylamide. _Xenobiotica_ 35, 1003–1018 (2005). Article CAS PubMed Google Scholar * Chang, C. M. et al. Biomarkers of tobacco exposure: summary of an FDA-sponsored
public workshop. _Cancer Epidemiol. Biomark. Prev._ 26, 291–302 (2017). Article CAS Google Scholar * Nagatsu, T. & Ichinose, H. Regulation of pteridine-requiring enzymes by the
cofactor tetrahydrobiopterin. _Mol. Neurobiol._ 19, 79–96 (1999). Article CAS PubMed Google Scholar * Brown, E. G. _Ring Nitrogen and Key Biomolecules: The Biochemistry of
N-heterocycles_ (Springer Science & Business Media, 2012). * Jimenez, B., del Peso, L., Montaner, S., Esteve, P. & Lacal, J. C. Generation of phosphorylcholine as an essential event
in the activation of Raf-1 and MAP-kinases in growth factors-induced mitogenic stimulation. _J. Cell. Biochem._ 57, 141–149 (1995). Article CAS PubMed Google Scholar * Ducker, G. S.
& Rabinowitz, J. D. One-carbon metabolism in health and disease. _Cell Metab._ 25, 27–42 (2017). Article CAS PubMed Google Scholar * Paoletti, P. & Neyton, J. NMDA receptor
subunits: function and pharmacology. _Curr. Opin. Pharm._ 7, 39–47 (2007). Article CAS Google Scholar * Gheorghe, C. E. et al. Focus on the essentials: tryptophan metabolism and the
microbiome-gut-brain axis. _Curr. Opin. Pharm._ 48, 137–145 (2019). Article CAS Google Scholar * Passi, S., Picardo, M., Mingrone, G., Breathnach, A. S. & Nazzaro-Porro, M. Azelaic
acid-biochemistry and metabolism. _Acta Derm.-venereologica. Supplementum_ 143, 8–13 (1989). CAS Google Scholar * Yamada, K. & Taketani, T. Management and diagnosis of mitochondrial
fatty acid oxidation disorders: focus on very-long-chain acyl-CoA dehydrogenase deficiency. _J. Hum. Genet._ 64, 73–85 (2019). Article CAS PubMed Google Scholar * Estrada, P. et al. The
pimeloyl-CoA synthetase BioW defines a new fold for adenylate-forming enzymes. _Nat. Chem. Biol._ 13, 668–674 (2017). Article CAS PubMed PubMed Central Google Scholar * Tanaka, H.,
Doesburg, K., Iwasaki, T. & Mierau, I. Screening of lactic acid bacteria for bile salt hydrolase activity. _J. Dairy Sci._ 82, 2530–2535 (1999). Article CAS PubMed Google Scholar *
Lieberman, P. The basics of histamine biology. _Ann. Allergy, Asthma Immunol._ 106, S2–5 (2011). Article Google Scholar * Dinis-Oliveira, R. J. Metabolism and metabolomics of opiates: a
long way of forensic implications to unravel. _J. Forensic Leg. Med._ 61, 128–140 (2019). Article PubMed Google Scholar * Welsch, L., Bailly, J., Darcq, E. & Kieffer, B. L. The
negative affect of protracted opioid abstinence: progress and perspectives from rodent models. _Biol. Psychiatry_ 87, 54–63 (2020). Article CAS PubMed Google Scholar * Hearing, M.,
Graziane, N., Dong, Y. & Thomas, M. J. Opioid and psychostimulant plasticity: targeting overlap in nucleus accumbens glutamate signaling. _Trends Pharm. Sci._ 39, 276–294 (2018). Article
CAS PubMed Google Scholar * Zhang, H. X., Lyons-Warren, A. & Thio, L. L. The glycine transport inhibitor sarcosine is an inhibitory glycine receptor agonist. _Neuropharmacology_ 57,
551–555 (2009). Article CAS PubMed PubMed Central Google Scholar * Wolosker, H. The neurobiology of d-serine signaling. _Adv. Pharmacol._ 82, 325–348 (2018). Article CAS PubMed
Google Scholar * Schwarcz, R., Bruno, J. P., Muchowski, P. J. & Wu, H. Q. Kynurenines in the mammalian brain: when physiology meets pathology. _Nat. Rev. Neurosci._ 13, 465–477 (2012).
Article CAS PubMed PubMed Central Google Scholar * Liu, J. F. & Li, J. X. TAAR1 in addiction: looking beyond the tip of the iceberg. _Front Pharm._ 9, 279 (2018). Article CAS
Google Scholar * Varaschin, R. K. et al. Histamine H3 receptors decrease dopamine release in the ventral striatum by reducing the activity of striatal cholinergic interneurons.
_Neuroscience_ 376, 188–203 (2018). Article CAS PubMed Google Scholar * Mohebbi, E. et al. An exploratory study of units of reporting opium usein Iran: implications for epidemiologic
studies. _Arch. Iran. Med._ 22, 541–545 (2019). PubMed Google Scholar * Degenhardt, L. et al. Agreement between definitions of pharmaceutical opioid use disorders and dependence in people
taking opioids for chronic non-cancer pain (POINT): a cohort study. _Lancet Psychiatry_ 2, 314–322 (2015). Article PubMed Google Scholar * Moningka, H. et al. Can neuroimaging help combat
the opioid epidemic? A systematic review of clinical and pharmacological challenge fMRI studies with recommendations for future research. _Neuropsychopharmacology_ 44, 259–273 (2019).
Article PubMed Google Scholar Download references ACKNOWLEDGEMENTS Dr. Blake Rushing and Dr. Krissy Kay contributed to quality control review of figures and tables. Mr. Justin Chandler
assisted with literature review, and editorial review. The metabolomics platform was developed with funding in part by the NIH Common Fund Phase 1 Program (1U24DK097193, Sumner PI), and the
NIEHS CHEAR program (U2CES026544, Fennell PI). This metabolomics investigation was funded in part through the NIDA Invest Fellowship (Dr. Reza Ghanbari), and the Sumner-Lab. The Golestan
Cohort Study was supported in part by Tehran University of Medical Sciences (grant no. 81/15); Cancer Research UK (grant no. C20/A5860); the Intramural Research Program of the NCI, NIH; and
various collaborative research agreements with IARC. AUTHOR INFORMATION Author notes * These authors contributed equally: Reza Ghanbari, Yuanyuan Li AUTHORS AND AFFILIATIONS * Department of
Nutrition, Nutrition Research Institute, University of North Carolina at Chapel Hill, Chapel Hill, NC, USA Reza Ghanbari, Yuanyuan Li, Wimal Pathmasiri, Susan McRitchie & Susan C. J.
Sumner * Digestive Oncology Research Center, Digestive Diseases Research Institute, Tehran University of Medical Science, Tehran, Iran Reza Ghanbari, Hossein Poustchi, Amaneh Shayanrad,
Behrouz Abaei & Reza Malekzadeh * Division of Cancer Epidemiology and Genetics, National Cancer Institute (NCI), Bethesda, MD, USA Arash Etemadi * Genetics, Epigenetics, and
Developmental Neuroscience Branch, National Institute on Drug Abuse (NIDA), Bethesda, MD, USA Jonathan D. Pollock * Iranian National Center for Addiction Studies (INCAS), Tehran University
of Medical Sciences (TUMS), Tehran, Iran Afarin Rahimi-Movaghar & Masoumeh Amin-Esmaeili * Department of Mental Health, Bloomberg School of Public Health, Johns Hopkins University,
Baltimore, MD, USA Masoumeh Amin-Esmaeili * Golestan Research Center of Gastroenterology and Hepatology, Golestan University of Medical Sciences, Gorgan, Iran Gholamreza Roshandel Authors *
Reza Ghanbari View author publications You can also search for this author inPubMed Google Scholar * Yuanyuan Li View author publications You can also search for this author inPubMed Google
Scholar * Wimal Pathmasiri View author publications You can also search for this author inPubMed Google Scholar * Susan McRitchie View author publications You can also search for this author
inPubMed Google Scholar * Arash Etemadi View author publications You can also search for this author inPubMed Google Scholar * Jonathan D. Pollock View author publications You can also
search for this author inPubMed Google Scholar * Hossein Poustchi View author publications You can also search for this author inPubMed Google Scholar * Afarin Rahimi-Movaghar View author
publications You can also search for this author inPubMed Google Scholar * Masoumeh Amin-Esmaeili View author publications You can also search for this author inPubMed Google Scholar *
Gholamreza Roshandel View author publications You can also search for this author inPubMed Google Scholar * Amaneh Shayanrad View author publications You can also search for this author
inPubMed Google Scholar * Behrouz Abaei View author publications You can also search for this author inPubMed Google Scholar * Reza Malekzadeh View author publications You can also search
for this author inPubMed Google Scholar * Susan C. J. Sumner View author publications You can also search for this author inPubMed Google Scholar CORRESPONDING AUTHORS Correspondence to Reza
Malekzadeh or Susan C. J. Sumner. ETHICS DECLARATIONS CONFLICT OF INTEREST The authors declare that they have no conflict of interest. DISCLOSURE The views and opinions expressed in this
manuscript are those of the authors only and do not necessarily represent the views, official policy or position of the U.S. Department of Health and Human Services or any of its affiliated
institutions or agencies. ADDITIONAL INFORMATION PUBLISHER’S NOTE Springer Nature remains neutral with regard to jurisdictional claims in published maps and institutional affiliations.
SUPPLEMENTARY INFORMATION SUPPLEMENTARY MATERIAL FIGURE S1 RIGHTS AND PERMISSIONS OPEN ACCESS This article is licensed under a Creative Commons Attribution 4.0 International License, which
permits use, sharing, adaptation, distribution and reproduction in any medium or format, as long as you give appropriate credit to the original author(s) and the source, provide a link to
the Creative Commons license, and indicate if changes were made. The images or other third party material in this article are included in the article’s Creative Commons license, unless
indicated otherwise in a credit line to the material. If material is not included in the article’s Creative Commons license and your intended use is not permitted by statutory regulation or
exceeds the permitted use, you will need to obtain permission directly from the copyright holder. To view a copy of this license, visit http://creativecommons.org/licenses/by/4.0/. Reprints
and permissions ABOUT THIS ARTICLE CITE THIS ARTICLE Ghanbari, R., Li, Y., Pathmasiri, W. _et al._ Metabolomics reveals biomarkers of opioid use disorder. _Transl Psychiatry_ 11, 103 (2021).
https://doi.org/10.1038/s41398-021-01228-7 Download citation * Received: 12 August 2020 * Revised: 08 January 2021 * Accepted: 18 January 2021 * Published: 04 February 2021 * DOI:
https://doi.org/10.1038/s41398-021-01228-7 SHARE THIS ARTICLE Anyone you share the following link with will be able to read this content: Get shareable link Sorry, a shareable link is not
currently available for this article. Copy to clipboard Provided by the Springer Nature SharedIt content-sharing initiative