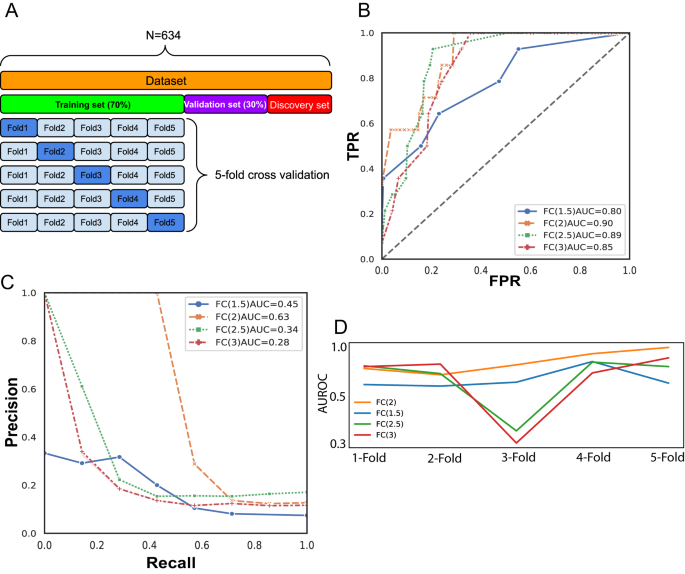
Identifying novel mechanisms of biallelic tp53 loss refines poor outcome for patients with multiple myeloma
- Select a language for the TTS:
- UK English Female
- UK English Male
- US English Female
- US English Male
- Australian Female
- Australian Male
- Language selected: (auto detect) - EN
Play all audios:

ABSTRACT Biallelic _TP53_ inactivation is the most important high-risk factor associated with poor survival in multiple myeloma. Classical biallelic _TP53_ inactivation has been defined as
simultaneous mutation and copy number loss in most studies; however, numerous studies have demonstrated that other factors could lead to the inactivation of _TP53_. Here, we hypothesized
that novel biallelic _TP53_ inactivated samples existed in the multiple myeloma population. A random forest regression model that exploited an expression signature of 16 differentially
expressed genes between classical biallelic _TP53_ and _TP53_ wild-type samples was subsequently established and used to identify novel biallelic _TP53_ samples from monoallelic _TP53_
groups. The model reflected high accuracy and robust performance in newly diagnosed relapsed and refractory populations. Patient survival of classical and novel biallelic _TP53_ samples was
consistently much worse than those with mono-allelic or wild-type _TP53_ status. We also demonstrated that some predicted biallelic _TP53_ samples simultaneously had copy number loss and
aberrant splicing, resulting in overexpression of high-risk transcript variants, leading to biallelic inactivation. We discovered that splice site mutation and overexpression of the splicing
factor _MED18_ were reasons for aberrant splicing. Taken together, our study unveiled the complex transcriptome of _TP53_, some of which might benefit future studies targeting abnormal
_TP53_. SIMILAR CONTENT BEING VIEWED BY OTHERS HOMOGENOUS TP53MUT-ASSOCIATED TUMOR BIOLOGY ACROSS MUTATION AND CANCER TYPES REVEALED BY TRANSCRIPTOME ANALYSIS Article Open access 14 April
2023 CLONAL AND SUBCLONAL TP53 MOLECULAR IMPAIRMENT IS ASSOCIATED WITH PROGNOSIS AND PROGRESSION IN MULTIPLE MYELOMA Article Open access 26 January 2022 COMPREHENSIVE MOLECULAR PROFILING OF
MULTIPLE MYELOMA IDENTIFIES REFINED COPY NUMBER AND EXPRESSION SUBTYPES Article Open access 19 August 2024 INTRODUCTION Multiple myeloma is a heterogeneous disease characterized by genomic
markers that are used to delineate high-risk disease. There are many algorithms to determine how to define high-risk myeloma, and the genomic markers include t(4;14), t(14;16), gain 1q21
(_CKS1B_), del 1p32 (_CDKN2C_), and del 17p13 (_TP53_) [1,2,3,4]. Of these markers, _TP53_ is probably the most important and is associated with rapid progression and poor overall survival.
Deletion of 17p has been seen as a poor prognostic marker since its discovery in MM [5]. Del 17p has been detected using fluorescence in situ hybridization, and although detection of
deletion in as low as 10% of cells is associated with poor outcome [6], the larger the proportion of cells with loss of 17p, the stronger the effect on outcome [7, 8]. More recently, the use
of molecular technologies has highlighted the importance of multi-hit or biallelic _TP53_ abnormalities in MM. We have previously shown that biallelic abnormalities of _TP53_, comprising
deletion and/or mutation of both alleles, are associated with outcome, whereas deletion alone is not [9]. The frequency of biallelic loss of _TP53_ in MM increases with disease progression,
being rare in smoldering myeloma (1.2%) and increasing through relapse (20%), indicating that it is a key mechanism in the pathogenesis of the disease [10, 11]. Biallelic loss of _TP53_ has
also been shown to be responsible for poor outcomes in myelodysplastic syndromes, myelofibrosis, and acute myeloid leukemia, pointing to a consistent abnormality in hematological
malignancies [12, 13]. In addition to mutation and deletion of _TP53_, other mechanisms are at play that result in loss of cellular function of p53, including alternative splicing, promoter
methylation, protein isoform usage, and changes in expression of gene regulators [14, 15]. These distinct mechanisms that result in the loss of functional p53 are currently impossible to
determine solely at the DNA level but could be assessed by modeling the downstream transcriptomic signature of biallelic _TP53_. Here, we utilized 634 newly diagnosed (NDMM) and 66
relapsed/refractory multiple myeloma (RRMM) samples from the MMRF CoMMpass dataset. By training a random forest regression model with transcriptomic features from known biallelic and
wild-type samples, we predicted potential biallelic _TP53_ samples from known monoallelic populations. Moreover, we demonstrated that predicted biallelic samples underwent expression of
high-risk transcript variants and aberrant splicing but also investigated the reasons that led to them. METHODS DEFINING ‘KNOWN’ BIALLELIC TP53 SAMPLES Mutation and copy number variation
calls were obtained from the MMRF CoMMpass dataset web portal (version IA18). Identified somatic mutations had agreements from at least three out of four mutation callers: Mutect2 [16],
Strelka2 [17], Octopus [18], and LANCET [19]. Copy number variations (CNVs) were identified by GATK [20], where copy number amplification (log2-fold change (Log2FC) ≥ 0.8), gain (0.2–0.8),
deletion (−0.2 to −2.0), and deep deletion (<−2.0) were defined. B-allele frequency was estimated by GATK [20] and normalized to scale (0 to 0.5), with 0.5 corresponding to the balanced
copy number and 0 corresponding to the complete loss of heterozygosity (LOH) between the major and minor alleles. We subsequently used <0.25 as the threshold to determine LOH status.
‘Known’ biallelic inactivation of _TP53_ (‘known’ biallelic) samples were defined as samples with either deep deletion, mutation plus deletion, or mutation plus LOH. Monoallelic _TP53_
(‘known’ monoallelic) samples were defined as samples with a _TP53_ mono-allelic mutation/CNV or LOH. In total, 634 newly diagnosed multiple myeloma (NDMM) samples with mutation and CNV
annotations were utilized, including 23 (3.6%), 62 (9.8%), and 549 (86.5%) samples with known biallelic, monoallelic, and wild-type status of _TP53_, respectively. Relapsed and refractory MM
(RRMM) patients who developed plasma cell leukemia at any relapsed stage were excluded. This resulted in 66 RRMM samples, including 4, 15, and 47 samples with known biallelic, monoallelic,
and wild-type status of _TP53_, respectively. DIFFERENTIALLY EXPRESSED GENE ANALYSIS Gene expression of NDMM, RRMM, and 5 normal bone marrow plasma cell (BMPC) samples was quantified by
transcript-per-million (TPM) [21] using Salmon [22] (Quasi-mode mapping, validate map mode) and Gencode V35 hg38 Gentrome (a combination of HG38 genome and Gencode HG38 transcriptome V35) as
a reference. Expression profiles were rescaled with Log2(TPM + 1) transformation. Differentially expressed genes (DEGs) were identified between known biallelic and wild-type _TP53_ samples
using LIMMA [23]. Significant DEGs were defined as FC > 1.4 or FC < 0.71, FDR < 0.05, and Log2(TPM + 1) > 1 in either group. DETERMINING PROLIFERATION (PR) GROUP FROM GENE
EXPRESSION The PR expression subgroup was previously described [24] (Supplementary Fig. 1E), and in this dataset, a PR group of 26 samples was identified. TRANSCRIPT VARIANT AND PROTEIN
DOMAIN INFERENCE The Scallop pipeline (https://github.com/Kingsford-Group/scallop) was used to identify and quantify novel transcript variants. Transcriptomes were assembled by Scallop [25],
while novel transcripts were identified by Gffread and quantified by Salmon [22]. Corresponding transcripts were extracted from the genome and translated to peptide sequences using the
Expasy database [26]. Conserved domains were identified from predicted peptide sequences using the NCBI conserved domain database [27]. PATHWAY ANALYSIS Gene overrepresentation analysis
(ORA) was conducted using WebGestalt [28]. Pathway enrichment analysis was conducted using GSEA [29]. The pathway knowledge bases ‘Kyoto Encyclopedia of Genes and Genomes’ (KEGG) [30] and
Wikipathways [31] were used. Single-sample level pathway analysis was conducted using GSVA [32] with a GSEA-defined hallmark pathway set. Only significantly dysregulated (_p_ < 0.05)
pathways were reported and plotted. MODEL PERFORMANCE METRICS True positive rate, false positive rate, precision, and recall were calculated and used to generate receiver operating
characteristic (ROC) curves and precision-recall curves (PRC). The area under the ROC curve (AUROC) and area under the PRC (AUPRC) were subsequently generated to measure the overall
performance of the models. HYPERPARAMETER TUNING To obtain the optimal hyperparameters that resulted in the model with the best performance, an exhaustive ‘grid search’ in the ‘scikit-learn’
[33] package was conducted for the number of trees (_n_ ∈ (1, Number of Genes)), the minimum number of samples required to be a leaf node (_n_ ∈ (0, 1)) and minimum weight fraction of the
sum total of weights required to be at a leaf node (_n_ \(\in\) (0, 1)) with an offset of 0.05. The model with the highest AUPRC and AUROC > 0.8 was selected. SURVIVAL ANALYSIS The MMRF
CoMMpass IA18 clinical annotation for 634 NDMM samples and 66 RRMM samples was utilized. The log-rank test and Cox regression tests were used to examine the survival difference between
groups. Kaplan‒Meier curves were drawn to describe progression-free survival (PFS), overall survival (OS), and after-relapse survival (ARS). In this study, ARS was defined as the survival
duration of patients after _TP53_ abnormality was detected/predicted for the first time. ALTERNATIVE SPLICING ANALYSIS rMATS [34] was used to identify AS events in _TP53_ regions between
each MM patient and 5 normal bone marrow plasma cell (BMPC) samples. Percent-of-Spliced-In (PSI) was used to measure the splicing level per sample, while deltaPSI (dPSI) was used to measure
average splicing differences between the two groups. The retention intron (RI) events were observed from Sashimi plots, and PSI and dPSI were calculated as follows:
$${\rm{PSI}}=\frac{{\rm{reads}}\,{\rm{on}}\,{\rm{the}}\,{\rm{intron}}}{{\rm{Junction}}\,{\rm{reads}}\,+\,{\rm{reads}}\,{\rm{on}}\,{\rm{the}}\,{\rm{intron}}}$$
$${\rm{dPSI}}={\rm{PSI}}_{\rm{normal}}^{-}-{\rm{PSI}}_{\rm{tumor}}^{-}$$ RESULTS MODEL TRAINING AND VALIDATION Although biallelic _TP53_ is currently defined by DNA methodologies, which
identify mutation and copy number loss, other mechanisms of inactivation can occur, including loss of expression, alternative splicing, and generation of rare protein isoforms. These
additional mechanisms could be difficult to identify but may be inferred from modeling downstream expression signatures. Using 634 NDMM samples with mutation and copy number annotations, we
determined biallelic, monoallelic, and wild-type _TP53_ status. Differentially expressed gene (DEG) analysis was conducted between existing known biallelic _TP53_ (_n_ = 23) and WT samples
(_n_ = 549) to identify a biallelic _TP53_ expression signature, and samples were split into training and validation sets in a 7:3 ratio (Fig. 1A). DEGs that were either significantly (FDR
< 0.05) up- or downregulated in biallelic _TP53_ were defined based on the fold-change (FC), namely, ‘FC1.5’, ‘FC2’, ‘FC2.5’ and ‘FC3’ (Supplementary Fig. 1A). Random forest regression
models were proposed to predict biallelic _TP53_ samples from _TP53_ wild-type (WT) samples using their expression profiles, and fivefold cross-validation was subsequently performed to
measure the robustness of the established models (Fig. 1A). The random forest regression models were established using each set of DEGs as features and compared by their area under
precision-recall curves (AUPRCs) and area under receiver operative characteristics curves (AUROCs). A hyperparameter tuning method was conducted to identify the parameter set corresponding
to the optimal performance. Given that the dataset is highly imbalanced (4.1% biallelic _TP53_ samples in the population), precision-recall curves are more informative and accurate when
measuring model performance [35, 36]. Hence, models with different parameters were prioritized by AUPRC first and subsequently by a high AUROC threshold (>0.8). Curves indicated models
with the best performance after hyperparameter tuning (Fig. 1B, C). The optimal model was derived from the FC > 2 DEG set and reflected not only the highest AUPRC among all but also
consistently high performance in 5-fold cross-validation (AUROC ∈ (0.82,1)). This model was subsequently selected for further analysis (Fig. 1D). The final model consisted of 25 trees,
comprising 16 genes (Fig. 2A) out of 100 DEGs in the ‘FC2’ set (Supplementary Fig. 1B). Among the 16 genes, 14 were connected in a protein‒protein interaction (PPI) network (Supplementary
Fig. 1C). Five genes were directly involved in the cell cycle, and related pathways (Fig. 2B). Of the other two genes, _NDC80_ (previously known as Hec1) is a key regulator of G2/M phase
[37] and is often overexpressed in human cancers, including MM [38]. High expression of _PHF19_ has been reported [39] to be associated with high-risk disease in myeloma. Most of the
significantly dysregulated pathways from 16 genes were related to the cell cycle (Fig. 2B), which is in line with the previously reported roles of _TP53_ [40]. Individual-level pathway
analysis was also conducted for all samples using GSVA and hallmark cancer pathway sets defined by the GSEA database. The top dysregulated pathways between known biallelic and WT samples
included _MYC_ targets, cell cycle, DNA repair, and oxidative phosphorylation, and these pathways were consistently upregulated between known and predicted biallelic samples (Supplementary
Fig. 1D). CHARACTERISTICS OF PREDICTED NOVEL BIALLELIC SAMPLES IN NDMM Samples in the validation dataset were given a predicted score (Fig. 2A). To reach the maximum sensitivity, a cutoff of
the predicted score was set until the last known biallelic sample was included, resulting in 100% sensitivity and 71.4% specificity. We noted the enrichment of several genomic markers in
the predicted biallelic _TP53_ samples, including biallelic _TENT5C_ (_N_ = 6; _p_ = 0.03, chi-square test), suggesting a similar regulation of gene expression between biallelic _TENT5C_ and
_TP53_ alterations. There was also enrichment for the PR subgroup expression signature [24] (_N_ = 6; _p_ = 0.002, chi-square test) in the predicted biallelic _TP53_ samples, indicating a
similarity between the PR signature and the biallelic _TP53_ signature, suggesting similar mechanisms at action. NOVEL BIALLELIC _TP53_ SAMPLES HAVE A SIMILAR EXPRESSION PROFILE AND ARE
ASSOCIATED WITH A POOR OUTCOME We hypothesize that there will be more novel biallelic _TP53_ samples in those that are already monoallelic defined by mutation or deletion. The established
model was therefore used to predict novel biallelic samples from known monoallelic samples, referred to as the discovery set (Table 1). The same cutoff score was applied to the discovery set
of known monoallelic _TP53_ samples (_N_ = 62), leading to the identification of 26 (42%) predicted biallelic samples. At the gene level, 26 samples were expressing _TP53_ (Log2(TPM + 1)
> 1). Differential expression analysis between the 26 predicted and 23 known biallelic samples identified no significantly dysregulated genes, indicating parity between the two sets. In
contrast, 939 significant DEGs were identified between the 26 predicted biallelic and 36 confirmed monoallelic samples from the discovery set. Subsequent GSEA analysis identified
significantly upregulated KEGG pathways covering the cell cycle/DNA replication, DNA damage repair, and p53 signaling (Supplementary Fig. 2A). These upregulated categories were consistently
observed from the same GSEA between the 23 known biallelic and 36 predicted monoallelic samples (Supplementary Fig. 2B). Additionally, we compared the number of SV events in known biallelic,
predicted biallelic, predicted monoallelic, and WT groups (Supplementary Fig. 2C). No significant difference was found between the known and predicted groups (median 48 vs. 31, _p_ = 0.23,
Mann‒Whitney U test), while both groups contained significantly more events than the WT group (median 48 vs. 31 vs. 22, _p_ = 0.0002 and _p_ = 0.04). This fact still held when both groups
were compared against the predicted monoallelic group (median 48 vs. 31 vs. 28, _p_ = 0.01 and _p_ = 0.07). Patient survival was compared between the 23 known biallelic, 26 predicted
biallelic, 36 confirmed monoallelic, and 549 wild-type samples. As expected, the previously defined monoallelic group (_N_ = 62) was not associated with a different outcome compared to WT
samples, while known biallelic samples (_N_ = 23) were associated with significantly worse survival than both groups in PFS (median survival (days): 478 vs. 900 vs. 1176, _p_ = 0.17 and _p_
= 0.02, log-rank test) and OS (median survival (days): 1094 vs. 2256 vs. 2859, _p_ = 0.02 and _p_ = 0.003, Supplementary Fig. 2D, E). However, using the new categorization, the predicted
biallelic samples were associated with a significantly worse outcome than the predicted monoallelic and WT samples with PFS (median survival (days): 623 vs. 1832 vs. 1176, _p_ = 0.002 and
_p_ = 0.0006) and OS (median survival (days): 1794 vs. not reached vs. 2859, _p_ = 0.09 and _p_ = 0.04) (Fig. 2C, D). Moreover, the survival difference between the confirmed monoallelic
group and WT was not significant for PFS (_p_ = 0.2) and OS (_p_ = 0.6) (Fig. 2C, D). Conversely, the predicted biallelic group showed no significant survival difference compared to the
known biallelic group for PFS and OS, indicating a similar patient outcome between known and predicted biallelic samples. When known biallelic and predicted biallelic samples were combined
in one group (_N_ = 49), their survival remained significantly worse than the confirmed monoallelic and WT samples for PFS (median survival (days): 610 vs. 1176 vs. 1832, _p_ = 0.002 and _p_
= 0.0001) and OS (median survival (days): 1340 vs. not-reached vs. 2859, _p_ = 0.01 and _p_ = 0.0002, Supplementary Fig. 2F, G). THE MODEL PREDICTS NOVEL BIALLELIC _TP53_ SAMPLES FROM
RELAPSED OR REFRACTORY MULTIPLE MYELOMA (RRMM) To explore the prediction power further, the model was applied to 66 RRMM samples (Table 1). Four known biallelic samples existed in the
validation set (Fig. 3A), and their corresponding NDMM samples from the same patients were also biallelic. The model resulted in a 76% AUROC and 42% AUPRC from the validation set of four
known biallelic and 47 wild-type samples, indicating consistently good performance. With the same threshold applied as in the NDMM population, 75% sensitivity (3/4) and 47% specificity
(22/47) were achieved. The model predicted seven biallelic samples that corresponded to 5 patients from 15 monoallelic samples in the discovery set (Fig. 3A). Among them, four monoallelic
samples from two patients were consistently predicted as biallelic samples. All seven samples were expressing _TP53_ (Log2(TPM + 1) > 1). After-relapse survival analysis conducted between
the five predicted biallelic and the eight remaining monoallelic patients in the discovery set showed that the patients with predicted biallelic _TP53_ had a significantly inferior
after-relapse survival (_p_ = 0.04, log-rank test) compared to the other eight monoallelic _TP53_ patients (Fig. 3B). Meanwhile, the duration before such relapse was not significantly
different (Supplementary Fig. 3). Taken together, this observation is in line with the inferior survival of patients once biallelic _TP53_ inactivation was detected. ABERRANT SPLICING AND
TRANSCRIPT VARIANT EXPRESSION WERE FOUND IN PREDICTED BIALLELIC _TP53_ SAMPLES _TP53_ has numerous transcripts, some of which encode isoforms that deviate from the original tumor suppressor
role and offer unique functions under different contexts [41]. Previous studies identified three major _TP53_ protein isoforms (_α_, _β_, and _γ_), each of which had four different lengths
(full length (TA), ∆40, ∆133, and ∆160) [15, 41]. In MM, patients with high expression of \({\rm{TAp53}}\beta \,{\rm{and}}\,{\rm{TAp}}53\gamma\) isoforms were associated with significantly
worse survival than patients without [15]. Conversely, high expression of ∆133 and ∆160 was associated with a good prognosis [15]. We speculated that there was an expression of adverse
_TP53_ transcripts that, along with mutations or copy number alterations, led to its complete loss of function and would identify additional biallelic _TP53_ samples that would not be
detected by DNA techniques alone. Based on established studies [15] and databases [42], we annotated all protein-coding transcript variants of _TP53_ listed in the Gencode [43] hg38 V35
genome annotation (Fig. 4A). In cancers, the expression of \(\alpha ,\beta \,{\rm{and}}\,\gamma\) isoforms is frequently switched [44]. Inclusion of the cryptic exon(s) between exons 9 and
10 results in a switch to the \(\beta \,{\rm{and}}\,\gamma\) isoforms (Fig. 4A). Among all predicted biallelic samples, we observed several that underwent dramatic splicing changes of the
cryptic exon (Fig. 4B), leading to the dominant expression of \(\beta /\gamma\) over \(\alpha\). For instance, inclusion of the cryptic exon (_dPSI_ = −0.54, versus normal) was observed in
sample MMRF_1922 (Fig. 4C), indicating the dominant expression of \(\beta /\gamma\) transcripts over \(\alpha\) (Fig. 4B). We further measured the expression levels of different transcript
variants (_α_, _β_ and _γ_) and transcript variants with different lengths (full length (TA), ∆40, ∆133 and ∆160) (Supplementary Fig. 4A-C). We confirmed that aberrant inclusion of the
cryptic exon resulted in the predominant expression of \({\rm{TAp}}53\beta\), which further led to the imbalanced expression of \({\rm{TAp}}53\beta /\gamma\) over \({\rm{TAp}}53\alpha\).
Such an imbalance was reported as a major predictor of poor prognosis in MM [15]. Given that MMRF_1922 had copy number-neutral LOH and a high level of imbalanced expression of
\({\rm{TAp}}53\beta /\gamma\) over \({\rm{TAp}}53\alpha\) (ratio = 1.95), it could be reasonably speculated that both alleles underwent cryptic exon inclusion, which further led to dominant
expression of high-risk transcripts, which was equivalent to complete inactivation of _TP53_. Similarly, a few predicted biallelic samples with copy number loss also underwent aberrant
cryptic exon inclusion. MMRF_1085_3 had copy number loss (CNV = −0.9) on one allele and cryptic exon inclusion on the other allele (_dPSI_ = −0.29, vs. normal, Fig. 4B, C). Some other
predicted biallelic samples had a mutation or copy number loss while having a very low expression of all _TP53_ transcripts. For instance, MMRF_1450, which was a predicted biallelic sample
with copy number loss, had low _TP53_ total gene expression (Log2(TPM + 1) = 1.01, log2FC = −0.89, vs. normal) as well as low \(\alpha\) transcript expression (Log2(TPM + 1) = 0.41, log2FC =
−1.59, vs. normal, Fig. 4B, C). This indicated that biallelic _TP53_ inactivation could also be defined by copy number loss on one allele and insufficient expression on the remaining
allele. Novel aberrant splicing sites other than the cryptic exon were also observed in predicted biallelic samples. For instance, in sample MMRF_2816 with copy number loss, we observed two
novel retained introns between exons 6 and 8 (Fig. 4D). Since the intron retention event was novel, a novel transcript variant assembly and subsequent functional analysis were conducted.
Three novel transcript variants with coding potential were inferred (Fig. 4D). Among them, ‘_TP53_-v4’ was predicted to have direct readthrough of exons 6–8, and the resulting protein would
terminate within intron 6, resulting in a truncated DNA-binding domain and loss of the tetramerization domain (Fig. 4D and Supplementary Fig. 4D) and protein function [45]. We found that
this transcript was expressed in MMRF_2816 (Log2(TPM + 1) = 0.92) and minimally expressed in normal BMPCs (Supplementary Fig. 4E). SPLICE SITE MUTATIONS RESULT IN ABERRANT INTRON SPLICING
After identifying aberrant splicing as a possible source that contributes to biallelic inactivation, we next tried to identify the reasons that lead to such abnormality. Previous research
indicated that splice site mutations could lead to alternative splicing [46]. We subsequently examined samples with splice site mutations in the population. Out of six samples with _TP53_
splice site mutations, three were found to have aberrant splicing (Fig. 5A–C), while the other three were not due to low VAF of the splice site mutations (average VAF = 0.1), and VAF was
positively correlated with aberrant splicing levels (Supplementary Fig. 5A–C). A C > T substitution (rs1131691042, g.7675052C>T) was found at the 3’ splice site of exon 5 in MMRF_1641
(Fig. 5A). Another C > T substitution (rs1555525367, g.7673838C>T) was found at the 5’ splice site of exon 8 in MMRF_2194 (Fig. 5B). Both single nucleotide variations had high variant
allele frequencies (VAF = 0.83 and 0.72), leading to aberrant intron retention (Fig. 5A, B). A T > C substitution (rs1555526335, g.7675235T>C, VAF = 0.26) was found at the 5’ end of
exon 10 in MMRF_1915, resulting in a novel alternative 3’ splice site event (Fig. 5C). This novel splice site shared a similar splicing pattern with the previously reported TP53\(\Psi\), of
which the aberrant splice site was on the 3’ between exon 6 and 7 [47]. ADDITIONAL MECHANISMS CONTROLLING CRYPTIC SPLICING OF _TP53_ Interestingly, the inclusion of the cryptic exons
described above was not related to splice site mutations and was likely governed by various factors, such as splicing factors [48] and miRNAs [49], each of which may be involved in
regulating the exclusion/inclusion of exons and introns. Most likely, the inclusion of cryptic exons is regulated by the combinational effects of these factors. To potentially identify a
master regulator that further controls exon inclusion in MM, we conducted a correlation analysis between gene expression and cryptic exon splicing levels. _MED18_ expression had the highest
correlation (Fig. 5D) with dPSI in _TP53_ cryptic splicing (\(\rho =-0.57\), Spearman correlation). Among 411 MM samples with more retained cryptic exons (dPSI < 0), _MED18_ expression
was significantly higher than that in normal BMPCs (FC = 1.3, _p_ = 0.04, Mann‒Whitney _U_ test, Fig. 5E). _MED18_ encodes a component of the mediator complex that binds to DNA, activating
transcription via RNA polymerase II (RNAPII) [50], of which the carboxy-terminal domain (CTD) regulates exon in-/exclusion via transcription elongation [51]. Inhibition of RNAPII elongation
has been shown to result in more exon inclusion in vitro and vice versa [52]. Our observation suggested that the downregulation of _MED18_ may lead to more cryptic exon inclusion, possibly
via the downregulation of RNAPII activity. DISCUSSION Historically, deletion of the short arm of chromosome 17p, as detected by cytogenetics and FISH, has been associated with poor outcomes
in MM, and the gene of interest on 17p is _TP53_ [7, 53]. As technologies have evolved, we have learned that deletion of _TP53_ alone may not be associated with poor outcomes. Instead,
biallelic inactivation of both copies, through deletion or mutation, is truly associated with poor outcome, and deletion alone is not [9]. This situation has been identified not only in MM
but also in myelodysplastic syndromes, myelofibrosis, and acute myeloid leukemia [12]. Given that biallelic inactivation of genes can arise not only through deletion and mutation but also by
a variety of other means, it stands to reason that there may be additional patients with biallelic inactivation of _TP53_ who are not identified with current DNA tests. However, the
downstream signature of biallelic inactivation may be detectable through expression profiling, which can give a more complete picture of the cellular response. In this study, we demonstrated
that biallelic _TP53_ samples in MM could be accurately predicted by the transcriptomic signature. This signature predicted biallelic samples from newly diagnosed and relapsed populations
with consistently high accuracy. As a result, 26 newly diagnosed and 5 relapsed samples with confirmed monoallelic _TP53_ status were predicted as biallelic _TP53_ patients. Their survival
showed no significant difference from known biallelic patients but was significantly worse than that of confirmed monoallelic or wild-type patients. This is in line with previous reports in
which patients with monoallelic _TP53_ copy number loss or mutation were also associated with inferior survival [54], which could be partly accounted for by an underestimation of the
population of biallelic inactivation. From the predicted subgroup, we identified the enrichment of samples with overexpressed high-risk _TP53_ transcript variants, which is in line with
previous reports [15] and may work in concert with existing monoallelic abnormalities such as copy number loss, resulting in loss of function of both alleles of _TP53_. Such high-risk _TP53_
transcript variants were derived from aberrant exon inclusion or intron retention. Moreover, different mechanisms of biallelic inactivation were observed. For instance, low expression of
_TP53_ was found in a few predicted biallelic samples with existing copy number loss, even though the reason for such low expression requires further investigation. These samples could be
potentially biallelic, while the second hit might not be detected due to various reasons, including DNA methylation of the _TP53_ promoter, cryptic rearrangements that are difficult to
resolve with short-read sequencing, or germline variants that are masked by somatic analysis. However, the second hit was unlikely to be caused by germline _TP53_ pathogenic mutations due to
its ultra-low frequency (<0.2%) in the CoMMpass MM population [55]. Previous studies indicated that splice site mutations in _TP53_ resulted in aberrant splicing in colorectal cancer
[46]. Here, we confirmed that such mutations not only led to aberrant splicing but also generated high-risk transcript variants, some of which were not previously documented. This indicated
that even though _TP53_ has been extensively studied, the complete _TP53_ landscape is highly heterogeneous among MM patients, illustrating a need for further investigation. Moreover,
aberrant splicing may work in concert with genomic variations to cause biallelic status, indicating a future need to combine genomic and transcriptomic features to confirm the biallelic
status of _TP53_ in the clinical setting. _TP53_ splicing is a complex process and possibly regulated by multiple factors simultaneously [48]. Most likely, the splicing level is determined
by a combination of factors. Nonetheless, we demonstrated that _MED18_ may serve as a master regulator to control the cryptic exon splicing level of _TP53_. Numerous reports have suggested
regulatory roles of mediator complex members in RNA splicing [56]. The strong correlation between _MED18_ expression and cryptic exon splicing levels indicated that by targeting the mediator
complex, the ‘hazardous’ _TP53_ isoforms could potentially be turned into tumor-suppressing isoforms, which may offer an alternative approach for targeting aberrant _TP53_ in MM. However,
as an essential component in the mediator complex, _MED18_ most likely controls numerous splicing events other than _TP53_ cryptic exons. DATA AVAILABILITY All sequencing datasets have been
previously published and can be accessed through dbGAP Study Accession number phs000748 (MMRF CoMMpass dataset) and GEO GSE110486 (normal plasma cell dataset). REFERENCES * Chng WJ,
Dispenzieri A, Chim CS, Fonseca R, Goldschmidt H, Lentzsch S, et al. IMWG consensus on risk stratification in multiple myeloma. Leukemia 2014;28:269–77. Article PubMed CAS Google Scholar
* Palumbo A, Avet-Loiseau H, Oliva S, Lokhorst HM, Goldschmidt H, Rosinol L, et al. Revised international staging system for multiple myeloma: a report from international myeloma working
group. J Clin Oncol 2015;33:2863–9. Article PubMed PubMed Central CAS Google Scholar * Caers J, Garderet L, Kortum KM, O’Dwyer ME, van de Donk N, Binder M, et al. European Myeloma
Network recommendations on tools for the diagnosis and monitoring of multiple myeloma: what to use and when. Haematologica 2018;103:1772–84. Article PubMed PubMed Central CAS Google
Scholar * D’Agostino M, Cairns DA, Lahuerta JJ, Wester R, Bertsch U, Waage A, et al. Second Revision of the International Staging System (R2-ISS) for Overall Survival in Multiple Myeloma: a
European Myeloma Network (EMN) Report Within the HARMONY Project. J Clin Oncol. 2022;40:3406–18. Article PubMed Google Scholar * Drach J, Ackermann J, Fritz E, Kromer E, Schuster R,
Gisslinger H, et al. Presence of a p53 gene deletion in patients with multiple myeloma predicts for short survival after conventional-dose chemotherapy. Blood 1998;92:802–9. Article PubMed
CAS Google Scholar * Walker BA, Leone PE, Chiecchio L, Dickens NJ, Jenner MW, Boyd KD, et al. A compendium of myeloma-associated chromosomal copy number abnormalities and their
prognostic value. Blood 2010;116:e56–65. Article PubMed CAS Google Scholar * Thanendrarajan S, Tian E, Qu P, Mathur P, Schinke C, van Rhee F, et al. The level of deletion 17p and
bi-allelic inactivation of TP53 has a significant impact on clinical outcome in multiple myeloma. Haematologica 2017;102:e364–e7. Article PubMed PubMed Central CAS Google Scholar *
Thakurta A, Ortiz M, Blecua P, Towfic F, Corre J, Serbina NV, et al. High subclonal fraction of 17p deletion is associated with poor prognosis in multiple myeloma. Blood 2019;133:1217–21.
Article PubMed PubMed Central CAS Google Scholar * Walker BA, Mavrommatis K, Wardell CP, Ashby TC, Bauer M, Davies F, et al. A high-risk, double-hit, group of newly diagnosed myeloma
identified by genomic analysis. Leukemia 2019;33:159–70. Article PubMed CAS Google Scholar * Boyle EM, Deshpande S, Tytarenko R, Ashby C, Wang Y, Bauer MA, et al. The molecular make up
of smoldering myeloma highlights the evolutionary pathways leading to multiple myeloma. Nat Commun. 2021;12:293. Article PubMed PubMed Central CAS Google Scholar * Weinhold N, Ashby C,
Rasche L, Chavan SS, Stein C, Stephens OW, et al. Clonal selection and double-hit events involving tumor suppressor genes underlie relapse in myeloma. Blood 2016;128:1735–44. Article PubMed
PubMed Central CAS Google Scholar * Bernard E, Nannya Y, Hasserjian RP, Devlin SM, Tuechler H, Medina-Martinez JS, et al. Implications of TP53 allelic state for genome stability,
clinical presentation and outcomes in myelodysplastic syndromes. Nat Med. 2020;26:1549–56. Article PubMed PubMed Central CAS Google Scholar * Gagelmann N, Badbaran A, Salit RB,
Schroeder T, Gurnari C, Pagliuca S, et al. Impact of TP53 on outcome of patients with myelofibrosis undergoing hematopoietic stem cell transplantation. Blood 2023;141:2901–11. PubMed CAS
Google Scholar * Teoh PJ, Chung TH, Sebastian S, Choo SN, Yan J, Ng SB, et al. p53 haploinsufficiency and functional abnormalities in multiple myeloma. Leukemia 2014;28:2066–74. Article
PubMed CAS Google Scholar * Rojas EA, Corchete LA, De Ramon C, Krzeminski P, Quwaider D, Garcia-Sanz R, et al. Expression of p53 protein isoforms predicts survival in patients with
multiple myeloma. Am J Hematol. 2022;97:700–10. Article PubMed PubMed Central CAS Google Scholar * Cibulskis K, Lawrence MS, Carter SL, Sivachenko A, Jaffe D, Sougnez C, et al.
Sensitive detection of somatic point mutations in impure and heterogeneous cancer samples. Nat biotechnology. 2013;31:213–9. Article CAS Google Scholar * Kim S, Scheffler K, Halpern AL,
Bekritsky MA, Noh E, Källberg M, et al. Strelka2: fast and accurate calling of germline and somatic variants. Nat Methods. 2018;15:591–4. Article PubMed CAS Google Scholar * Cooke DP,
Wedge DC, Lunter G. A unified haplotype-based method for accurate and comprehensive variant calling. Nat Biotechnol. 2021;39:885–92. Article PubMed PubMed Central CAS Google Scholar *
Narzisi G, Corvelo A, Arora K, Bergmann EA, Shah M, Musunuri R, et al. Genome-wide somatic variant calling using localized colored de Bruijn graphs. Commun Biol. 2018;1:1–9. Article CAS
Google Scholar * Van der Auwera GA, Carneiro MO, Hartl C, Poplin R, Del Angel G, Levy‐Moonshine A, et al. From FastQ data to high‐confidence variant calls: the genome analysis toolkit best
practices pipeline. Curr Protoc Bioinforma. 2013;43:11. Google Scholar * Wagner GP, Kin K, Lynch VJ. Measurement of mRNA abundance using RNA-seq data: RPKM measure is inconsistent among
samples. Theory Biosci. 2012;131:281–5. Article PubMed CAS Google Scholar * Patro R, Duggal G, Love MI, Irizarry RA, Kingsford C. Salmon provides fast and bias-aware quantification of
transcript expression. Nat methods. 2017;14:417–9. Article PubMed PubMed Central CAS Google Scholar * Ritchie ME, Phipson B, Wu DI, Hu Y, Law CW, Shi W, et al. limma powers differential
expression analyses for RNA-sequencing and microarray studies. Nucleic acids Res. 2015;43:e47. Article PubMed PubMed Central Google Scholar * Zhan F, Huang Y, Colla S, Stewart JP,
Hanamura I, Gupta S, et al. The molecular classification of multiple myeloma. Blood 2006;108:2020–8. Article PubMed PubMed Central CAS Google Scholar * Shao M, Kingsford C. Accurate
assembly of transcripts through phase-preserving graph decomposition. Nat Biotechnol. 2017;35:1167–9. Article PubMed PubMed Central CAS Google Scholar * Gasteiger E, Gattiker A,
Hoogland C, Ivanyi I, Appel RD, Bairoch A. ExPASy: the proteomics server for in-depth protein knowledge and analysis. Nucleic acids Res. 2003;31:3784–8. Article PubMed PubMed Central CAS
Google Scholar * Lu S, Wang J, Chitsaz F, Derbyshire MK, Geer RC, Gonzales NR, et al. CDD/SPARCLE: the conserved domain database in 2020. Nucleic Acids Res. 2020;48:D265–D8. Article
PubMed CAS Google Scholar * Wang J, Vasaikar S, Shi Z, Greer M, Zhang B. WebGestalt 2017: a more comprehensive, powerful, flexible and interactive gene set enrichment analysis toolkit.
Nucleic acids Res. 2017;45:W130–W7. Article PubMed PubMed Central CAS Google Scholar * Subramanian A, Tamayo P, Mootha VK, Mukherjee S, Ebert BL, Gillette MA, et al. Gene set enrichment
analysis: a knowledge-based approach for interpreting genome-wide expression profiles. Proc Natl Acad Sci USA. 2005;102:15545–50. Article PubMed PubMed Central CAS Google Scholar *
Kanehisa M, Goto S. KEGG: Kyoto encyclopedia of genes and genomes. Nucleic Acids Res. 2000;28:27–30. Article PubMed PubMed Central CAS Google Scholar * Slenter DN, Kutmon M, Hanspers K,
Riutta A, Windsor J, Nunes N, et al. WikiPathways: a multifaceted pathway database bridging metabolomics to other omics research. Nucleic Acids Res. 2018;46:D661–D7. Article PubMed CAS
Google Scholar * Hänzelmann S, Castelo R, Guinney J. GSVA: gene set variation analysis for microarray and RNA-seq data. BMC Bioinforma. 2013;14:1–15. Article Google Scholar * Pedregosa F,
Varoquaux G, Gramfort A, Michel V, Thirion B, Grisel O, et al. Scikit-learn: Machine learning in Python. J Mach Learn Res. 2011;12:2825–30. Google Scholar * Shen S, Park JW, Lu Z-X, Lin L,
Henry MD, Wu YN, et al. rMATS: robust and flexible detection of differential alternative splicing from replicate RNA-Seq data. Proc Natl Acad Sci USA. 2014;111:E5593–E601. Article PubMed
PubMed Central CAS Google Scholar * Saito T, Rehmsmeier M. The precision-recall plot is more informative than the ROC plot when evaluating binary classifiers on imbalanced datasets. PLoS
One. 2015;10:e0118432. Article PubMed PubMed Central Google Scholar * Jeni LA, Cohn JF, De La Torre F, editors. Facing imbalanced data--recommendations for the use of performance
metrics. (IEEE, 2013). * Qu Y, Li J, Cai Q, Liu B. Hec1/Ndc80 is overexpressed in human gastric cancer and regulates cell growth. J Gastroenterol. 2014;49:408–18. Article PubMed CAS
Google Scholar * Wu P, Walker BA, Brewer D, Gregory WM, Ashcroft J, Ross FM, et al. A gene expression–based predictor for myeloma patients at high risk of developing bone disease on
bisphosphonate treatment. Clin Cancer Res. 2011;17:6347–55. Article PubMed CAS Google Scholar * Mason MJ, Schinke C, Eng CLP, Towfic F, Gruber F, Dervan A, et al. Multiple Myeloma DREAM
Challenge reveals epigenetic regulator PHF19 as marker of aggressive disease. Leukemia 2020;34:1866–74. Article PubMed PubMed Central Google Scholar * Chen J. The cell-cycle arrest and
apoptotic functions of p53 in tumor initiation and progression. Cold Spring Harb Perspect Med. 2016;6:a026104. Article PubMed PubMed Central Google Scholar * Anbarasan T, Bourdon J-C.
The emerging landscape of p53 isoforms in physiology, cancer and degenerative diseases. Int J Mol Sci. 2019;20:6257. Article PubMed PubMed Central CAS Google Scholar * Zhou X, Edmonson
MN, Wilkinson MR, Patel A, Wu G, Liu Y, et al. Exploring genomic alteration in pediatric cancer using ProteinPaint. Nat Genet. 2016;48:4–6. Article PubMed PubMed Central CAS Google
Scholar * Frankish A, Diekhans M, Ferreira A-M, Johnson R, Jungreis I, Loveland J, et al. GENCODE reference annotation for the human and mouse genomes. Nucleic acids Res. 2019;47:D766–D73.
Article PubMed CAS Google Scholar * Bourdon JC. p53 isoforms change p53 paradigm. Mol Cell Oncol. 2014;1:e969136. Article PubMed PubMed Central CAS Google Scholar * Baugh EH, Ke H,
Levine AJ, Bonneau RA, Chan CS. Why are there hotspot mutations in the TP53 gene in human cancers? Cell Death Differ. 2018;25:154–60. Article PubMed CAS Google Scholar * Smeby J, Sveen
A, Eilertsen IA, Danielsen SA, Hoff AM, Eide PW, et al. Transcriptional and functional consequences of TP53 splice mutations in colorectal cancer. Oncogenesis 2019;8:1–8. Article CAS
Google Scholar * Senturk S, Yao Z, Camiolo M, Stiles B, Rathod T, Walsh AM, et al. p53Ψ is a transcriptionally inactive p53 isoform able to reprogram cells toward a metastatic-like state.
Proc Natl Acad Sci USA. 2014;111:E3287–E96. Article PubMed PubMed Central CAS Google Scholar * Kędzierska H, Piekiełko-Witkowska A. Splicing factors of SR and hnRNP families as
regulators of apoptosis in cancer. Cancer Lett. 2017;396:53–65. Article PubMed Google Scholar * Jones MF, Lal A. MicroRNAs, wild-type and mutant p53: more questions than answers. RNA
Biol. 2012;9:781–91. Article PubMed PubMed Central CAS Google Scholar * Sato S, Tomomori-Sato C, Banks CAS, Sorokina I, Parmely TJ, Kong SE, et al. Identification of Mammalian Mediator
Subunits with Similarities to Yeast Mediator Subunits Srb5, Srb6, Med11, and Rox3* 210. J Biol Chem. 2003;278:15123–7. Article PubMed CAS Google Scholar * Muñoz MJ, Santangelo MSP,
Paronetto MP, de la Mata M, Pelisch F, Boireau S, et al. DNA damage regulates alternative splicing through inhibition of RNA polymerase II elongation. Cell 2009;137:708–20. Article PubMed
Google Scholar * Ip JY, Schmidt D, Pan Q, Ramani AK, Fraser AG, Odom DT, et al. Global impact of RNA polymerase II elongation inhibition on alternative splicing regulation. Genome Res.
2011;21:390–401. Article PubMed PubMed Central CAS Google Scholar * Boyd KD, Ross FM, Tapper WJ, Chiecchio L, Dagrada G, Konn ZJ, et al. The clinical impact and molecular biology of del
(17p) in multiple myeloma treated with conventional or thalidomide‐based therapy. Genes Chromosomes Cancer. 2011;50:765–74. Article PubMed CAS Google Scholar * Corre J, Perrot A,
Caillot D, Belhadj K, Hulin C, Leleu X, et al. del (17p) without TP53 mutation confers a poor prognosis in intensively treated newly diagnosed patients with multiple myeloma. Blood.
2021;137:1192–5. Article PubMed PubMed Central CAS Google Scholar * Thibaud S, Etra A, Subaran R, Soens Z, Newman S, Chen R, et al. Pathogenic germline variants in multiple myeloma.
Blood 2021;138:399. Article Google Scholar * Huang Y, Li W, Yao X, Lin Q-J, Yin J-W, Liang Y, et al. Mediator complex regulates alternative mRNA processing via the MED23 subunit. Mol Cell.
2012;45:459–69. Article PubMed PubMed Central CAS Google Scholar Download references ACKNOWLEDGEMENTS B.A.W. is partially supported by NIH grant R01CA249981 and the Leukemia &
Lymphoma Society. This study used the Multiple Myeloma Research Foundation (MMRF) CoMMpass Dataset. The authors acknowledge the efforts of the MMRF research consortium to provide the
fundamental resource for our study. AUTHOR INFORMATION AUTHORS AND AFFILIATIONS * Melvin and Bren Simon Comprehensive Cancer Center, Division of Hematology and Oncology, School of Medicine,
Indiana University, Indianapolis, IN, USA Enze Liu, Parvathi Sudha, Nathan Becker, Oumaima Jaouadi, Attaya Suvannasankha, Kelvin Lee, Rafat Abonour, Mohammad Abu Zaid & Brian A. Walker *
Center for Computational Biology and Bioinformatics, School of Medicine, Indiana University, Indianapolis, IN, USA Brian A. Walker Authors * Enze Liu View author publications You can also
search for this author inPubMed Google Scholar * Parvathi Sudha View author publications You can also search for this author inPubMed Google Scholar * Nathan Becker View author publications
You can also search for this author inPubMed Google Scholar * Oumaima Jaouadi View author publications You can also search for this author inPubMed Google Scholar * Attaya Suvannasankha View
author publications You can also search for this author inPubMed Google Scholar * Kelvin Lee View author publications You can also search for this author inPubMed Google Scholar * Rafat
Abonour View author publications You can also search for this author inPubMed Google Scholar * Mohammad Abu Zaid View author publications You can also search for this author inPubMed Google
Scholar * Brian A. Walker View author publications You can also search for this author inPubMed Google Scholar CONTRIBUTIONS E.L. performed data analysis and wrote the paper. B.A.W.
conceived the project and wrote the paper. All authors have read and approved the paper. CORRESPONDING AUTHOR Correspondence to Brian A. Walker. ETHICS DECLARATIONS COMPETING INTERESTS
B.A.W. received unrelated research support from Bristol–Myers Squibb and Genentech and has received consulting fees from Genentech and Sanofi. ADDITIONAL INFORMATION PUBLISHER’S NOTE
Springer Nature remains neutral with regard to jurisdictional claims in published maps and institutional affiliations. SUPPLEMENTARY INFORMATION SUPPLEMENTARY MATERIAL RIGHTS AND PERMISSIONS
OPEN ACCESS This article is licensed under a Creative Commons Attribution 4.0 International License, which permits use, sharing, adaptation, distribution and reproduction in any medium or
format, as long as you give appropriate credit to the original author(s) and the source, provide a link to the Creative Commons license, and indicate if changes were made. The images or
other third party material in this article are included in the article’s Creative Commons license, unless indicated otherwise in a credit line to the material. If material is not included in
the article’s Creative Commons license and your intended use is not permitted by statutory regulation or exceeds the permitted use, you will need to obtain permission directly from the
copyright holder. To view a copy of this license, visit http://creativecommons.org/licenses/by/4.0/. Reprints and permissions ABOUT THIS ARTICLE CITE THIS ARTICLE Liu, E., Sudha, P., Becker,
N. _et al._ Identifying novel mechanisms of biallelic _TP53_ loss refines poor outcome for patients with multiple myeloma. _Blood Cancer J._ 13, 144 (2023).
https://doi.org/10.1038/s41408-023-00919-2 Download citation * Received: 13 June 2023 * Revised: 27 August 2023 * Accepted: 30 August 2023 * Published: 11 September 2023 * DOI:
https://doi.org/10.1038/s41408-023-00919-2 SHARE THIS ARTICLE Anyone you share the following link with will be able to read this content: Get shareable link Sorry, a shareable link is not
currently available for this article. Copy to clipboard Provided by the Springer Nature SharedIt content-sharing initiative