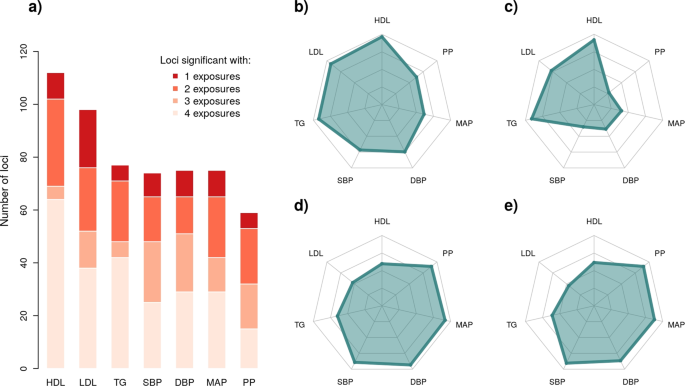
Gene-lifestyle interactions in the genomics of human complex traits
- Select a language for the TTS:
- UK English Female
- UK English Male
- US English Female
- US English Male
- Australian Female
- Australian Male
- Language selected: (auto detect) - EN
Play all audios:

ABSTRACT The role and biological significance of gene-environment interactions in human traits and diseases remain poorly understood. To address these questions, the _CHARGE Gene-Lifestyle
Interactions Working Group_ conducted series of genome-wide interaction studies (GWIS) involving up to 610,475 individuals across four ancestries for three lipids and four blood pressure
traits, while accounting for interaction effects with drinking and smoking exposures. Here we used GWIS summary statistics from these studies to decipher potential differences in genetic
associations and G×E interactions across phenotype-exposure-ancestry combinations, and to derive insights on the potential mechanistic underlying G×E through in-silico functional analyses.
Our analyses show first that interaction effects likely contribute to the commonly reported ancestry-specific genetic effect in complex traits, and second, that some phenotype-exposures
pairs are more likely to benefit from a greater detection power when accounting for interactions. It also highlighted modest correlation between marginal and interaction effects, providing
material for future methodological development and biological discussions. We also estimated contributions to phenotypic variance, including in particular the genetic heritability
conditional on the exposure, and heritability partitioned across a range of functional annotations and cell types. In these analyses, we found multiple instances of potential heterogeneity
of functional partitions between exposed and unexposed individuals, providing new evidence for likely exposure-specific genetic pathways. Finally, along this work, we identified potential
biases in methods used to jointly meta-analyze genetic and interaction effects. We performed simulations to characterize these limitations and to provide the community with guidelines for
future G×E studies. SIMILAR CONTENT BEING VIEWED BY OTHERS AN APPROACH TO IDENTIFY GENE-ENVIRONMENT INTERACTIONS AND REVEAL NEW BIOLOGICAL INSIGHT IN COMPLEX TRAITS Article Open access 22
April 2024 VARIANCE-QUANTITATIVE TRAIT LOCI ENABLE SYSTEMATIC DISCOVERY OF GENE-ENVIRONMENT INTERACTIONS FOR CARDIOMETABOLIC SERUM BIOMARKERS Article Open access 09 July 2022 GENOTYPE ×
ENVIRONMENT INTERACTIONS IN GENE REGULATION AND COMPLEX TRAITS Article 10 June 2024 INTRODUCTION The precise role of gene-environment interactions (G×E) in complex human traits and disease
traits remains unclear. Although genome-wide G×E studies have been conducted for many phenotypes, the number of identified G×E is very small relative to the large number of genetic variants
identified in traditional genome-wide association studies (GWAS). A number of issues related to the identification of G×E have been well described in the literature [1,2,3], including in
particular very low power [4]. As a result, the required sample size needed to detect G×E is substantially larger than for marginal genetic effect (i.e., genetic effect estimated from a
model not accounting for G×E). Moreover, few studies have explored potential differences in G×E across ancestry, assessed the contribution of G×E to the variance of human phenotypes, or
explored enrichment of G×E for specific functional mechanisms. The Gene-Lifestyle Interactions Working Group [5] within the Cohorts for Heart and Aging Research in Genetic Epidemiology
(CHARGE) is an international initiative that has the potential to address some of these challenges. It is a large-scale, multi-ancestry consortium that aims at systematically evaluating
genome-wide gene-lifestyle interactions on cardiovascular disease-related traits using genotypic data from up to 610,475 individuals. The consortium published a series of genome-wide single
nucleotide polymorphism (SNP) by smoking and drinking interaction screenings focusing on four blood pressure phenotypes: diastolic blood pressure (DBP), systolic blood pressure (SBP), pulse
pressure (PP), mean arterial pressure (MAP), and three lipid levels: triglycerides (TG), high-density lipoprotein cholesterol (HDL), and low-density lipoprotein cholesterol (LDL). For each
pair of a phenotype and an exposure, a genome-wide interaction studies (GWIS) using the 1 degree of freedom (df) test for G×E interaction and the 2df joint test of main genetic effect
(_i.e_. the estimate of genetic effect from the interaction model) and G×E interaction effects [6] has been conducted. The results from these analyses have been published in five papers:
SNP-by-alcohol interaction [7] and SNP-by-smoking interaction [8, 9] on blood pressure, and SNP-by-alcohol interaction [10] and SNP-by-smoking interaction on lipids [11]. Here we first
synthesize the GWIS results for all phenotype-exposure combinations. We highlight the importance of our large-scale initiative, providing evidence that interacting variants might differ by
genetic ancestry, and show that accounting for G×E can help to discover new loci, especially for certain phenotype-exposure pairs. We then performed a series of analyses comparing
interaction effects against marginal genetic effects derived from both our studies and from previous GWAS. Contrary to a commonly assumed hypothesis [12], we found only modest correlation
between interaction effect and marginal effect, highlighting additional challenges for future G×E interactions studies. Estimated variance explained by main and interaction effect for the
outcomes under study also showed that in general, interactions explain a very small amount of phenotypic variance on top of the marginal genetic effect for these traits. However, these
limitations were balanced by stratified heritability analyses. Partitioning the genetic variance in exposed and unexposed individuals separately, using both functional and cell-type
annotations, we observed differential enrichment patterns between the two groups in multiple instances. This suggests G×E might still play an important role in these phenotypes, with some
exposures potentially triggering new molecular mechanisms or reducing the contribution of pathways involved in unexposed individuals. METHODS DATA AND PROCESSING We considered four blood
pressure phenotypes (DBP, SBP, PP, MAP), and three lipids levels (TG, HDL, LDL). Two binary smoking exposures, _current smoking_ and _ever smoking_, were considered and measured similarly
across all smoking GWIS. The _current smoking_ variable was coded as 1 if the subject smoked regularly in past year and as 0 otherwise. _Ever smoking_ status was coded as 1 if the subject
smoked at least 100 cigarettes during his/her lifetime and 0 otherwise. For alcohol consumption, two binary variables were considered, referred further as _current drinking_ and _drinking
habit_. All studies conducted a two-stage approach. In stage 1 (referred to as _Discovery_), a standard GWIS was performed using up to 18 million genetic variants. In stage 2 (referred to as
_Replication_), only a subset of variants with a _p_ value for either 1df or the 2df test below a certain threshold (_P_ < 10−6 or _P_ < 10−5) at stage 1 were further considered. For
each outcome exposure, we had access to complete meta-analysis summary statistics of both the discovery and the replication stages for four different ancestries (European, African, Asian,
and Hispanic) after quality control filtering. To ensure a fair comparison, we re-processed all results for each outcome-exposure-ancestry combination using the same pipeline. More details
are provided on the data and pre-processing are available in the supplementary notes and in the corresponding publications [7,8,9,10,11]. IDENTIFICATION OF INDEPENDENT SIGNALS AND ASSOCIATED
LOCI We defined two levels of association when reporting genome-wide significant variants in the combined meta-analyses (_P_ < 5 × 10−8): independent signal (lead SNPs after clumping)
and associated locus (genetic regions of 1 Mb with at least one independent signal). _Independent signals_ represent independent SNPs associated at genome-wide significance level.
Independent signals were defined using the clumping framework from the PLINK software [13], using a linkage disequilibrium (LD) threshold of 0.2 and a maximum physical distance from the lead
SNP (_i.e_., the most associated variant) of ±500 kb. The LD was derived using 1000 Genomes Project [14] individuals as a reference panel while accounting for ancestry. We used the EUR,
AFR, combined EAS-SAS, and AMR samples as proxies for the individuals from European ancestry (EA), African ancestry (AA), Asian ancestry (ASA), and Hispanic ancestry (HA), respectively. For
the trans-ancestry analyses, we built our reference panel by merging all those reference populations. _Associated loci_ are genetic regions of 1 Mb or more harboring at least one genome-wide
associated SNPs. To define associated loci, we first derived region 500 kb upstream and downstream of each and every independent signal (as defined above). All overlapping regions were then
merged to form the loci. Further details are provided in the Supplementary Note INTERACTION EFFECT CONDITIONAL ON MARGINAL EFFECT We assessed potential enrichment for interactions effects
for SNPs displaying marginal genetic association. To increase independence between our interaction effect GWIS and the marginal GWAS, we used summary statistics from previous studies on
blood pressure traits [15,16,17] and lipid traits [18,19,20,21]. However, note that there might be a small overlap of samples between some of these previously published marginal GWAS and the
1df and 2df GWIS from the CHARGE consortium. For this analysis, we considered only individuals of EA, in order to maximize the sample size while limiting potential issues due to genetic
heterogeneity, where the top variants might differ across populations. In practice we used the 1df interaction test from the combined analysis derived in European sample in CHARGE, and for
external studies, we used only the GWAS conducted in European populations. Moreover, to avoid enrichment driven by a single locus, we performed a clumping of the previously published GWAS of
marginal genetic effect with PLINK [13], so that all lead SNPs considered are independent from each other. We first derived the proportion of interaction effect nominally significant at
type I error rate (alpha) threshold of 0.05 among bins of SNPs grouped based on their marginal association (i.e., we derive the proportion of SNPs with interaction _p_ value below 0.05 and
_p_ value for marginal effect in bins [1, 0.1], [0.1, 0.01], [0.01, 0.001], etc). Note that the aforementioned clumping of SNPs avoids any biased enrichment due to pairwise SNP correlation.
For the last bin, including only SNPs previously identified at genome-wide significance level (5 × 10−8) in marginal effect GWAS, we also performed three complementary association tests [4]
to assess interaction effects that would have been missed by single SNP G×E interaction: an omnibus test, an unweighted genetic risk score (uGRS) test, and a weighted genetic risk score
(wGRS) (see Supplementary Note). VARIANCE EXPLAINED AND HERITABILITY For each ancestry and each phenotype-exposure combination, we derived from the combined (stage 1 and stage 2) results the
fraction of phenotypic variance explained by top SNPs was decomposed into main effects, interaction effects and those effects jointly using the R package _VarExp_ [22]. The significance of
the variance explained by interaction effects was derived using an approximation of the joint test of all interaction effects. For EA samples, we further assessed potential differences in
heritability across exposure-specific strata using stage 1 genome-wide association results using the _LDSC_ approach [23]. We used the pre-computed _LDscore_ relative to EA samples provided
with the software. When unavailable from the original studies, stratified results were derived from the interaction model using J2S [24]. For each exposure stratum, genetic heritability was
further partitioned by both cell-type-specific and general annotations [25] using two distinct sets of annotations: _baseline_ and _GenoSkyline_+. The significance of the annotation
enrichment was assessed using a Bonferroni corrected significance threshold of _P_ < 0.000277. Tissue-specific heritability was also derived following Finucane et al. [26]. Except when
specified otherwise, enrichment analyses compared median enrichment between exposure strata. We avoided comparison of significance level here, which would be biased by differences in sample
size. Additional details of these analyses are provided in the Supplementary Note. RESULTS OVERVIEW We investigated results from 28 GWIS on three lipid and four blood pressure phenotypes,
each examining G×E interaction with two smoking and two alcohol exposures (Table 1). All outcome-exposure pairs were analyzed using a two-stage approach involving up to 610,475 individuals.
In stage 1, a GWIS was performed in up to 29 cohorts with a total of up to 149,684 individuals from four ancestries: EA, AA, ASA, and HA. In stage 2, involving up to 71 additional cohorts
including 460,791 individuals, also from multiple ancestries, studies focused on the replication of a subset of variants from stage 1. The total sample size (discovery and replication)
varied substantially across the trait analyzed, with an average of 311 K for lipids and 457 K for blood pressure traits. Moreover, our analyses explored not only the 28 primary
trans-ancestry GWIS, but also the 112 corresponding ancestry-specific GWIS. To ensure a fair comparison across all analyses, we re-processed all GWIS summary results using the same pipeline.
Stage 1 quantile-quantile (QQ) plots for both the 1df and the 2df test are presented in Fig. S1, and frequency of the exposure are presented in Fig. S2 and Table S1. Finally, note that the
primary association results from the original studies and our analyses are highly concordant, but minor differences might exist because of slight differences in the analysis pipeline.
IDENTIFYING SINGLE SNP G×E IS CHALLENGING BUT ACCOUNTING FOR G×E STILL BOOST POWER Despite the reasonably large sample size available in our studies, there was only one significant
interaction signal with the 1df interaction test across the 28 trans-ancestry GWIS when combining discovery and replication (rs1626071 on chr10 near gene _LINC01517_, interaction with
current smoking on MAP, _P__1df_ = 3.19 × 10−8). For the ancestry-specific meta-analysis, the 1df interaction test identified 8 loci reaching genome-wide significance, all observed in the
African ancestry population (Table S2). They involved the smoking exposure only, and are associated with both lipids and blood pressure traits. Four of those loci were also detected at
genome-wide significance with the 2df test in the African ancestry population, while the remaining four associations achieve suggestive significance with that test (_P__2df_ between 1.9 ×
10−6 and 6.3 × 10−8). In sharp contrast with the 1df interaction test, the 2df joint test identified a large number of variants in both the trans-ancestry (Table 1) and ancestry-specific
(Tables S3 and S4) meta-analyses. Altogether, the 2df trans-ancestry analyses identified a total of 1698 loci-phenotype associations (see “Methods” for the definition of loci), when summing
results over all phenotypes and all exposures. Among those, a total of 54% of the loci (_N_ = 926) harbored a single independent association signal, while others display multiple independent
signals (Fig. S3). Many loci overlapped across the exposures tested. For example, there were 108 and 103 loci identified for HDL when including interaction between current drinking and
drinking habits, respectively. However, 92 of those loci were identified in both analyses. Merging all overlapping loci unraveled by different exposure scans, the 2df scans found a total of
112, 98, 77 loci for HDL, LDL, TG, and 74, 75, 75, and 59 loci for SBP, DBP, MAP, and PP respectively. On average, 13% of the loci were identified by a single exposure scan, while 41% were
identified by all four exposure association studies for each phenotype (Fig. 1a). We compared the trans-ancestry 2df results against 599 significant marginal genetic effect association on
the two primary blood pressure traits (DBP and SBP) [15,16,17] and the three lipid traits [20, 27] retrieved from previous studies (Tables S5, S6, Fig. S4, and “Methods”). Among those, 294
were also found genome-wide significant in our studies, and 305 associations did not reach this significance threshold. Conversely, the trans-ancestry 2df screenings identified 119 novel
loci-outcome associations. Most of the new association results for lipids were identified when accounting for interaction with drinking exposures, while the majority of new blood pressure
associations were identified when accounting for interaction with smoking exposures (Table 2). Part of these observed differences might be explained by heterogeneity in sample size (e.g. _N_
was substantially smaller for BP and drinking habits as compared to BP and other exposure). However, sample size for all other phenotype-exposure pairs were fairly very similar (<14%)
and unlikely to explain difference in number of replicated signal (e.g. for HDL, _N_drinking habits = 379 K, _N_current smoking = 384 K, and number of replicated signals equals 17 and 11,
respectively). We also derived the association signal from the combined stage 1 and 2 SNPs that would have been obtained using a standard marginal genetic effect in the CHARGE data, while
adjusting for the effect of the exposure, but not modeling the interaction. The marginal model replicated only at a similar proportion of signal, 48% (_N_ = 289) as compared to 49% (_N_ =
294) of the 599 previously reported associations. Among the 119 new associations detected by the 2df test, 29% (_N_ = 35) did not passed the genome-wide significance level with the marginal
model, highlighting the importance of accounting for G×E to detect new associated variants. G×E EFFECTS MIGHT VARY BY EXPOSURE AND ANCESTRY When stratifying the 2df joint test results by
exposure, accounting for interaction with drinking tended to identify more lipids associations, while accounting for interaction with smoking identified more associations for blood pressure
phenotypes (Fig. 1b–e). Looking at cross-phenotypes results, GWIS accounting for current drinking and drinking habits captured 81% and 61% of all loci, respectively, and current smoking and
ever smoking scans identified 75% and 72% of all loci respectively. Note that the lower number of signals for drinking habits is likely partly explained by the smaller sample size used for
that exposure (307 K on average versus 440 K for the other exposures), especially for the BP GWIS that used a different definition for drinking habits (see “Methods”). To understand the
differences observed across other exposures, we used the HDL results as a case study. First, we noticed that the chi-squared from the 2df joint test from overlapping loci across the four
exposure scans were highly correlated (Fig. S5a). This is expected, as most studies have approximately the same sample size at discovery and replication stages, and contribution of the
interaction effect is assumed to be limited. Nevertheless, we noticed a larger mean interaction effect chi-square at those same loci for the drinking exposures (\(\overline {\chi ^2}\)=
1.57, _P_ = 8.7 × 10−5 and 1.58, _P_ = 1.2 × 10−4) as compared to the smoking exposures (\(\overline {\chi ^2}\) = 1.07, _P_ = 0.32 and 1.09, _P_ = 0.28), suggesting a potential contribution
of SNP-by-drinking interaction effect (Fig. S5b). Over the two stages, 63% of the individuals (_N_ = 380,612) were of European, 27% (_N_ = 162,370) of Asian, 6% (_N_ = 34,901) of African
and 4% (_N_ = 22,334) of HAs. For the 2df joint test, the total number of significant associations per ancestry was, as expected, significantly positively correlated to the available sample
size (_r_2 = 0.42, 95% CI = [0.25, 0.57], _P_ = 1.1 × 10−5) (Table S3). When merging results from all phenotype-exposure pairs, there were 1,285, 383, 135, and 148 phenotype-variants
associations identified after clumping by this approach in EA, ASA, AA, and HA ancestries, respectively. The vast majority of the loci found in the ASA (95%) and HA (99%) ancestries were
also identified in the larger EA studies (Fig. 2a). Conversely, 32% (43 out of 135) of the associations identified in AA were exclusively identified in this ancestry and mostly involved
variants not present in all ancestries except in AA (in non-AA ancestry populations, 60% of those variants were filtered out at stage 1 because of low frequency). The trans-ancestry analysis
identified 1276 (94%) of all ancestry-specific associations, while uncovering an additional 148 associations. All associations missed in the trans-ancestry analyses were found in a single
ancestry from ASA (_N_ = 6), AA (_N_ = 36), EA (_N_ = 41), and HA (_N_ = 1). To account for sample size differences and assess whether top variants were consistent across ancestries, we
extracted the top variants for each ancestry-specific association and checked for nominal significance (_P_ < 0.05) in other ancestries screenings from stage 1. Figure 2b shows that the
overlap across all phenotypes and per phenotype is modest. These results, along the aforementioned 1df significant signals unique to the AA samples, suggest the presence of ancestry-specific
variants and G×E s, and in AA in particular. CORRELATION BETWEEN MARGINAL GENETIC AND INTERACTION EFFECT ARE NEGLIGIBLE To understand further the contribution of G×E to significant 2df
results, we derived for each phenotype-exposure-ancestry combination (_N_ = 12,302, see Table 1 and Table S3) the number of SNPs inducing an enhanced genetic effect in exposed individuals
(when main genetic effect and interaction effect have the same direction) and those inducing a reduced genetic effect (when main and interaction have opposite signs). In practice, we used
the marginal genetic effect as a proxy for the main effect, as the former parameter has better properties for such an analysis (i.e., the marginal effect is expected to be independent of the
interaction effect under the null [12], while the main effect does not [4]). Note that this subtlety has almost no impact on the results as the main and marginal are highly correlated (_ρ_
= 0.99) among the significant 2df variants. Overall, the direction of marginal and interaction effects estimated using the 1df test tended to be randomly distributed among those SNPs,
although we observed a slight enrichment, with 13 out of 91 trios showing disequilibrium for either concordant or discordant effects (one sided binomial test _P_ = 6 × 10−4, Fig. S6). Four
of them, all in the trans-ancestry analyses, displayed discordant marginal and interaction effect that remained significant after correcting for multiple testing (_P_ < 5.5 × 10−4): LDL
showed larger genetic effects in both current (_P_ = 4.3 × 10−4) and ever smokers (_P_ = 3.7 × 10−4), DBP showed larger genetic effects in current drinkers (_P_ = 1.6 × 10−4), and SBP showed
smaller genetic effects among ever smokers (_P_ = 9.9 × 10−5). Among sets of variants displaying interaction effects discordant with marginal genetic effects, we also searched for those
inducing an opposite effect between exposed and unexposed individuals. Although the 2df joint test is supposed to outperform substantially the marginal test in this scenario [4], there were
only 66 such associations (0.6% of all associations), suggesting this pattern is quite rare in these data. We next assessed potential enrichment for interaction effects across variants
previously identified in marginal effect GWAS [15,16,17, 20, 27] (Tables S5 and S6) and available in the trans-ancestry stage 1 analyses. Among those variants, the smallest single SNP 1df
interaction _p_ value was observed for rs1260326 (for G×Drinking habits on TG, _P__1df_ = 3.3e−6), a missense variant in _GCKR_ previously found associated with alcohol consumption [28, 29].
However, besides this particular signal, the distribution of interaction effects at those variants did not indicate any clear trend (Fig. S7) and the joint test of all single SNP [30] did
not find any significant enrichment for interaction effect among these variants (see Supplementary Notes, Table S7). We also explored potential enrichment for interaction at non-significant
SNPs. Such enrichment would be of particular interest to increase power of G×E test through 2-step approaches [12, 31, 32] (see for example Fig. S8). The most common 2-step approach consists
of filtering out SNPs displaying a marginal genetic _p_ value larger than a given α1 significance threshold. To assess the potential of this strategy in our data, we quantified the
enrichment of nominally significant variants (i.e. _P_ < 0.05) for G×E interaction effect while varying α1 between 0.1 and 10−6 applied to the aforementioned previous marginal GWAS
summary statistics. Some phenotype-exposure pairs show a slight increase in the proportion of significant G×E interactions, including in particular TG and drinking habits (11% of the SNPs
against the 5% expected for α1 = 10−5). However, as illutrated in Fig. 3 which display the enrichment along 0.05 and 1 × 10−4 confidence interval, no enrichment remains significant after
correction for multiple testing in our data. We also considered using marginal genetic effect derived from the stage 1 in CHARGE (Fig. S9). This analysis displayed a modest enrichment for
interaction with drinking exposure for lipids and with current smoking for TG, with enrichment for some bins falling outside the stringent confidence interval (i.e., _P_ < 1 × 10−4).
SMALL CONTRIBUTION OF G×E AT TOP VARIANTS BUT DIFFERENCES IN HERITABILITY STRATIFIED BY EXPOSURE We first used _VarExp_, a tool we recently developed [22], to estimate the variance explained
by marginal genetic effects, the joint genetic and G×E interaction effects, and the interaction effects only, at the top genome-wide significant variants in each locus for each
phenotype-exposure-ancestry analysis (Table S8). Marginal genetic effects explained between 0.09% and 8.72% of the total phenotypic variance with an average of 3.59%. The fraction of
variance explained by the interaction effects only were substantially smaller, varying between 0% and 0.41%, but were statistically significant for many analyses. The largest amount of
variance explained was observed for lipids traits, (average of 4.47% for the 2df, as compared to 0.81% for blood pressure phenotypes). Looking at ancestry-specific results, we noted a larger
fraction of variance explained in the European ancestry samples than in other ancestries, with greater differences observed in lipids phenotypes and drinking exposures (7.11% of explained
variance in individuals from European ancestry versus 5.11% in other ancestries on average). We also noted a slightly higher contribution of G×E in the African ancestry population (0.15%)
than in other ancestries (around 0.04%), in agreement with the higher number of significant interactions identified for this ancestry. Second, we estimated potential changes in the
heritability of the three lipids and two blood pressures (DBP and SBP) traits across all individuals and in strata defined by exposure, using the _LDSC_ approach [23] applied to summary
statistics from the stage 1 analyses performed in the European ancestry population (Fig. 4, Table S9). Because of potentially biased heritability estimates, we performed a sensitivity
analysis, re-deriving the heritability after filtering out SNPs based on their _p_ value for heterogeneity in the meta-analysis and selected the most reliable estimate (see Fig. S10 and
Supplementary Notes). Based on those estimates, we observed that heritability among exposed individuals was on average smaller than among non-exposed individuals for current smoking
(\(\overline {h^2}\) = 0.06 and \(\overline {h^2}\) = 0.11, respectively) and for drinking habits (\(\overline {h^2}\) = 0.12 and \(\overline {h^2}\) = 0.15, respectively). Conversely,
heritability was on average larger for current drinkers than non-current drinkers (\(\overline {h^2}\) = 0.15 and \(\overline {h^2}\) = 0.11, respectively). However, only one
outcome-phenotype pair showed borderline nominal significance (HDL and drinking habits, with \(h^2\) = 0.19, _P_ = 7.0 × 10−17, and _h_2 = 0.13, _P_ = 3.1 × 10−10 for unexposed and exposed,
respectively, _P_ = 0.052), and this difference did not remain statistically significant after correction for multiple testing. DIFFERENTIAL PATHWAYS ACROSS EXPOSURES To explore further
differences in genetic effect between exposure strata, we partitioned the genetic heritability estimated in individuals from European ancestry across different functional annotations [25,
26]. We first considered baseline annotations provided with the _LDSC_ package and the _GenoSkyline_ [33] annotation set, a cell-type-specific annotation database derived mainly from the
Roadmap Epigenomics [34] (Figs. S11–15). Because of the relatively modest sample size in some strata (_N_ = 12,578 in the smallest strata, see Table S1), we focused on the distribution of
the estimated enrichment coefficient between exposed and unexposed. The majority of phenotype-exposure pairs exhibited a similar enrichment pattern (Fig. S16). For example, the enrichment
estimates were significantly correlated for drinking habits exposure and lipids (correlations equal 0.76 (_P_ = 9.5 × 10−24), 0.60 (_P_ = 5.8 × 10−13) and 0.40 (_P_ = 7.7 × 10−6) for HDL,
LDL and TG, respectively), suggesting that potential G×E interactions for those phenotypes do not involve new pathways. Conversely, LDL shows substantial variability in enrichment for the
three other exposures (correlations equal 0.10 (_P_ = 0.25), 0.22 (_P_ = 0.01), and 0.17 (_P_ = 0.07), for current drinking, current smoking, and ever smoking, respectively), suggesting
those exposures might activate new genetic pathways while reducing the effect of genetic variants involved in unexposed individuals. We also noted substantial variability for the
phenotypes-exposure pairs showing the largest differences in heritability (lipids and current smoking, and BP and drinking habits, see Fig. 4). However, part of that variability might be due
to the reduced sample size in one of the two strata, thus making interpretation challenging. We next investigated whether exposures tended to display systematic enrichment in specific
tissues [26]. For each phenotype, heritability was stratified based on annotation from 205 cell types linked to 9 tissues (adipose, blood/immune, cardiovascular, central nervous system,
digestive, endocrine, liver, musculoskeletal/connective, and other), in unexposed and exposed individuals separately (Fig. 5, Figs. S17–S21). Because of unbalanced sample size between
strata, we focused on the relative differences in median enrichment between exposed and unexposed by tissue, and reported the proportion of cell types nominally significant for enrichment in
each tissue. Overall, liver and adipose were the most enriched and most significant tissues for lipids traits, while showing variability between exposed and unexposed individuals. LDL also
showed some significance and variability for cell types mapped to digestive tissue for the drinking exposures and current smoking (Fig. 5a). There was less significant enrichment and a less
marked difference for BP traits, although we noticed a substantially larger enrichment in liver tissue among heavy drinkers versus low-drinkers for DBP (Fig. 5d). GENETIC HETEROGENEITY,
POWER, AND RISK OF BIAS FOR THE TEST OF INTERACTION IN TRANS-ANCESTRY ANALYSIS Throughout this work, we used G×E interaction effect estimates and _p_ values derived using the standard 1df
inverse-variance meta-analysis scheme as described in Willer et al. [35], and applied to the 1df interaction effect from each contributing cohort similarly to the original GWIS papers. On
the other hand, we used the main genetic effect estimates and _p_ values derived from the 2df framework as described in Manning et al. [36]. Although, the 2df framework provides a joint
estimation of the main and interaction effect coefficients along with standard errors, we did not use the interaction effect parameters from that model as we identified potential biases in
those estimates through several simulation studies (see Supplementary notes for more details and Table S10). Heterogeneity for both the main genetic effect and the proportion of exposed
individuals between the two cohorts (e.g., higher genetic effect in one cohort combined to a higher level of exposure) can bias the interaction effect estimates in the 2df framework and
result in a severe type I error rate inflation, inducing false-positive associations (Fig. S22). However, main genetic effects estimates are not biased in the 2df framework in the case of a
binary exposure (Fig. S23) but can be noisy in the case of a continuous exposure (Fig. S24). These simulation studies highlighted the special care required to interpret results from the 2df
framework. DISCUSSION In this study, we assembled and synthesized the results from 28 G×E interaction GWIS on lipid and blood pressure phenotypes performed across four ancestries. Overall,
we found the trans-ancestry 2df test to be efficient for SNP discovery, with the vast majority of associations identified in ancestry-specific analyses being confirmed in the trans-ancestry
analysis, while allowing for a 10% increase in detection. However, our data also pointed toward ancestry-specific patterns for interaction effects, especially for African ancestry
populations. Differences were also observed when comparing results across exposures. We noted a greater increase in detection for lipid-associated variants when accounting for interaction
with drinking, and a greater increase in detection for blood pressure-associated variants when accounting for interaction with smoking. When leveraging marginal genetic effect reported from
previous studies to select potential candidates for interaction effects, we did not observe any significant enrichment for interaction effects whatever the significance level used. This is
in agreement with our in-depth comparison of main genetic and interaction effects using the consortium data, which found only modest correlation between the interaction and main effects
coefficients. Finally, our assessment of variance explained by interaction effects suggests that, even if small, accounting for interaction can help push signals above the stringent
genome-wide significance threshold. Furthermore, the stratification of heritability by functional annotations highlighted that exposures can induce divergent mechanisms of phenotype
production with modification in the associated genetic pathway and cell type involved. Our estimation of the phenotypic variance explained by marginal genetic effect and interaction shows,
in agreement with previous studies, that the contribution of G×E terms on top of marginal genetic effect is relatively modest. It confirms the likely limited impact of discovering G×E for
prediction purposes in the general population [37]. The variability between non-smokers and drinkers observed in the exposure-specific heritability is intriguing, but might potentially be
explained by other factors which cannot be sorted out using these data. Further work is needed not only to understand this heterogeneity but also to assess potential gain in predictive power
of polygenic risk score derived by exposure strata [38]. Importantly, a modest contribution of G×E to phenotypic variance does not rule out the potentially important role of G×E in the
etiology of these traits. And, for example, our stratified heritability analyses suggest a potential change in the genetic architecture of LDL conditional on smoking and BP traits
conditional on current drinking. The statistical power of the GWIS varied substantially across analyses. Taking the average sample size across all phenotype-exposure pairs analyzed per
ancestry, there was 80% power at an alpha threshold of 5 × 10−8 to detect interaction effect explaining 0.0096% (trans-ancestry, \(\bar N\) = 440 K), 0.016% (EA, \(\bar N\) = 271 K), 0.15%
(AA, \(\bar N\) = 27 K), 0.034% (ASA, \(\bar N\) = 123 K), and 0.22% (HISP, \(\bar N\) = 19 K) of the outcome variance. The observed enrichment for interaction effects in AA as compared to
other ancestries is therefore quite striking, and further investigation in larger data is required. It would also be of interest to explore whether interactions play a role in the well
documented differences in prevalence of both blood pressure outcomes (e.g. hypertension [39]) and lipids (e.g. low HDL [40]) in individuals from African-American ancestry. The sample size
was also critical when deriving heritability. Here, we only considered the European ancestry data as sample size for other cohorts was too small to derive meaningful estimates. Nevertheless,
statistical power remains limited in EA for _LDSC_ stratified analyses based on functional annotation, and future larger studies are also required to validate the observed enrichments. We
fully appreciate that the results from the several experiments we conducted are challenging to aggregate into a single uniform framework. Our analysis rather suggests first that even though
related traits share some features; they can also display substantial heterogeneity at other levels. For example, all lipids harbor more signals when accounting for drinking exposures, but
at the same time display very different patterns when investigating functional enrichment. It also suggests that the links between heritability, genetic mechanisms involved, and the
resulting distribution of G×E effect across SNPs are not straightforward. Finally, our careful assessment of each step of the analyses highlights that complexity also shows up at the
methodological level, with a potential for introducing bias at several stages, and so the extra care needed for interpretation. Despite those limitations, we argue that systematic and
careful evaluation of G×E across multiple phenotype-exposure-ancestry combinations, as done in this study, still provides critical insight of the interplay between genetic and environmental
factors, offering long-term opportunities for numerous additional follow-up analyses down to the biological mechanisms underlying the phenotypes and their interaction with the environment.
DATA AVAILABILITY All of the data used in this work are publicly available. Both the original GWAS summary results and the re-processed statistics generated as part of this study are
available via dbGaP (accession number phs000930). REFERENCES * McAllister K, Mechanic LE, Amos C, Aschard H, Blair IA, Chatterjee N, et al. Current challenges and new opportunities for
gene-environment interaction studies of complex diseases. Am J Epidemiol. 2017;186:753–61. Article Google Scholar * Gauderman WJ, Mukherjee B, Aschard H, Hsu L, Lewinger JP, Patel CJ, et
al. Update on the state of the science for analytical methods for gene-environment interactions. Am J Epidemiol. 2017;186:762–70. Article Google Scholar * Ritchie MD, Davis JR, Aschard H,
Battle A, Conti D, Du M, et al. Incorporation of biological knowledge into the study of gene-environment interactions. Am J Epidemiol. 2017;186:771–7. Article Google Scholar * Aschard H. A
perspective on interaction effects in genetic association studies. Genet Epidemiol. 2016;40:678–88. Article Google Scholar * Rao DC, Sung YJ, Winkler TW, Schwander K, Borecki I, Cupples
LA, et al. Multiancestry study of gene-lifestyle interactions for cardiovascular traits in 610 475 individuals from 124 cohorts: design and rationale. Circ Cardiovasc Genet. 2017;10:e001649.
Article Google Scholar * Kraft P, Yen YC, Stram DO, Morrison J, Gauderman WJ. Exploiting gene-environment interaction to detect genetic associations. Hum Hered. 2007;63:111–9. Article
CAS Google Scholar * Feitosa MF, Kraja AT, Chasman DI, Sung YJ, Winkler TW, Ntalla I, et al. Novel genetic associations for blood pressure identified via gene-alcohol interaction in up to
570K individuals across multiple ancestries. PLoS ONE. 2018;13:e0198166. Article Google Scholar * Sung YJ, Winkler TW, de Las Fuentes L, Bentley AR, Brown MR, Kraja AT, et al. A
large-scale multi-ancestry genome-wide study accounting for smoking behavior identifies multiple significant loci for blood pressure. Am J Hum Genet. 2018;102:375–400. Article CAS Google
Scholar * Sung YJ, de Las Fuentes L, Winkler TW, Chasman DI, Bentley AR, Kraja AT, et al. A multi-ancestry genome-wide study incorporating gene-smoking interactions identifies multiple new
loci for pulse pressure and mean arterial pressure. Hum Mol Genet. 2019;28:2615–33. Article CAS Google Scholar * de Vries PS, Brown MR, Bentley AR, Sung YJ, Winkler TW, Ntalla I, et al.
Multi-Ancestry Genome-Wide Association Study of Lipid Levels Incorporating Gene-Alcohol Interactions. Am J Epidemiol. 2019;188:1033–54. Article Google Scholar * Bentley AR, Sung YJ, Brown
MR, Winkler TW, Kraja AT, Ntalla I, et al. Multi-ancestry genome-wide gene-smoking interaction study of 387,272 individuals identifies new loci associated with serum lipids. Nat Genet.
2019;51:636–48. Article CAS Google Scholar * Kooperberg C, Leblanc M. Increasing the power of identifying gene x gene interactions in genome-wide association studies. Genet Epidemiol.
2008;32:255–63. Article Google Scholar * Chang CC, Chow CC, Tellier LC, Vattikuti S, Purcell SM, Lee JJ. Second-generation PLINK: rising to the challenge of larger and richer datasets.
Gigascience. 2015;4:7. Article Google Scholar * Genomes Project C, Auton A, Brooks LD, Durbin RM, Garrison EP, Kang HM, et al. A global reference for human genetic variation. Nature.
2015;526:68–74. Article Google Scholar * Kato N, Loh M, Takeuchi F, Verweij N, Wang X, Zhang W, et al. Trans-ancestry genome-wide association study identifies 12 genetic loci influencing
blood pressure and implicates a role for DNA methylation. Nat Genet. 2015;47:1282–93. Article CAS Google Scholar * Liang J, Le TH, Edwards DRV, Tayo BO, Gaulton KJ, Smith JA, et al.
Single-trait and multi-trait genome-wide association analyses identify novel loci for blood pressure in African-ancestry populations. PLoS Genet. 2017;13:e1006728. Article Google Scholar *
Warren HR, Evangelou E, Cabrera CP, Gao H, Ren M, Mifsud B, et al. Genome-wide association analysis identifies novel blood pressure loci and offers biological insights into cardiovascular
risk. Nat Genet. 2017;49:403–15. Article CAS Google Scholar * Below JE, Parra EJ, Gamazon ER, Torres J, Krithika S, Candille S, et al. Meta-analysis of lipid-traits in Hispanics
identifies novel loci, population-specific effects, and tissue-specific enrichment of eQTLs. Sci Rep. 2016;6:19429. Article CAS Google Scholar * Prins BP, Kuchenbaecker KB, Bao Y, Smart
M, Zabaneh D, Fatemifar G, et al. Genome-wide analysis of health-related biomarkers in the UK Household Longitudinal Study reveals novel associations. Sci Rep. 2017;7:11008. Article Google
Scholar * Surakka I, Horikoshi M, Magi R, Sarin AP, Mahajan A, Lagou V, et al. The impact of low-frequency and rare variants on lipid levels. Nat Genet. 2015;47:589–97. Article CAS Google
Scholar * Teslovich TM, Musunuru K, Smith AV, Edmondson AC, Stylianou IM, Koseki M, et al. Biological, clinical and population relevance of 95 loci for blood lipids. Nature.
2010;466:707–13. Article CAS Google Scholar * Laville V, Bentley AR, Prive F, Zhu X, Gauderman J, Winkler TW, et al. VarExp: estimating variance explained by genome-wide G×E summary
statistics. Bioinformatics. 2018;34:3412–4. Article CAS Google Scholar * Bulik-Sullivan BK, Loh PR, Finucane HK, Ripke S, Yang J. Schizophrenia Working Group of the Psychiatric Genomics
C, et al. LD Score regression distinguishes confounding from polygenicity in genome-wide association studies. Nat Genet. 2015;47:291–5. Article CAS Google Scholar * Laville V, Majarian T,
de Vries PS, Bentley AR, Feitosa MF, Sung YJ, et al. Deriving stratified effects from joint models investigating Gene-Environment Interactions. BMC Bioinformatics. 2020;21:251 Article
Google Scholar * Finucane HK, Bulik-Sullivan B, Gusev A, Trynka G, Reshef Y, Loh PR, et al. Partitioning heritability by functional annotation using genome-wide association summary
statistics. Nat Genet. 2015;47:1228–35. Article CAS Google Scholar * Finucane HK, Reshef YA, Anttila V, Slowikowski K, Gusev A, Byrnes A, et al. Heritability enrichment of specifically
expressed genes identifies disease-relevant tissues and cell types. Nat Genet. 2018;50:621–9. Article CAS Google Scholar * Klarin D, Damrauer SM, Cho K, Sun YV, Teslovich TM, Honerlaw J,
et al. Genetics of blood lipids among ~300,000 multi-ethnic participants of the Million Veteran Program. Nat Genet. 2018;50:1514–23. Article CAS Google Scholar * Clarke TK, Adams MJ,
Davies G, Howard DM, Hall LS, Padmanabhan S, et al. Genome-wide association study of alcohol consumption and genetic overlap with other health-related traits in UK Biobank (N = 112 117). Mol
Psychiatry. 2017;22:1376–84. Article CAS Google Scholar * Jorgenson E, Thai KK, Hoffmann TJ, Sakoda LC, Kvale MN, Banda Y, et al. Genetic contributors to variation in alcohol consumption
vary by race/ethnicity in a large multi-ethnic genome-wide association study. Mol Psychiatry. 2017;22:1359–67. Article CAS Google Scholar * Aschard H, Tobin MD, Hancock DB, Skurnik D,
Sood A, James A, et al. Evidence for large-scale gene-by-smoking interaction effects on pulmonary function. Int J Epidemiol. 2017;46:894–904. PubMed PubMed Central Google Scholar *
Gauderman WJ, Zhang P, Morrison JL, Lewinger JP. Finding novel genes by testing GxE interactions in a genome-wide association study. Genet Epidemiol. 2013;37:603–13. Article Google Scholar
* Zhang P, Lewinger JP, Conti D, Morrison JL, Gauderman WJ. Detecting gene-environment interactions for a quantitative trait in a genome-wide association study. Genet Epidemiol.
2016;40:394–403. Article Google Scholar * Lu Q, Powles RL, Wang Q, He BJ, Zhao H. Integrative tissue-specific functional annotations in the human genome provide novel insights on many
complex traits and improve signal prioritization in genome wide association studies. PLoS Genet. 2016;12:e1005947. Article Google Scholar * Lu Q, Powles RL, Abdallah S, Ou D, Wang Q, Hu Y,
et al. Systematic tissue-specific functional annotation of the human genome highlights immune-related DNA elements for late-onset Alzheimer’s disease. PLoS Genet. 2017;13:e1006933. Article
Google Scholar * Willer CJ, Li Y, Abecasis GR. METAL: fast and efficient meta-analysis of genomewide association scans. Bioinformatics 2010;26:2190–1. Article CAS Google Scholar *
Manning AK, LaValley M, Liu CT, Rice K, An P, Liu Y, et al. Meta-analysis of gene-environment interaction: joint estimation of SNP and SNP x environment regression coefficients. Genet
Epidemiol. 2011;35:11–8. Article Google Scholar * Aschard H, Chen J, Cornelis MC, Chibnik LB, Karlson EW, Kraft P. Inclusion of gene-gene and gene-environment interactions unlikely to
dramatically improve risk prediction for complex diseases. Am J Hum Genet. 2012;90:962–72. Article CAS Google Scholar * Aschard H, Zaitlen N, Lindstrom S, Kraft P. Variation in predictive
ability of common genetic variants by established strata: the example of breast cancer and age. Epidemiology. 2015;26:51–8. Article Google Scholar * Fryar CD, Ostchega Y, Hales CM, Zhang
G, Kruszon-Moran D. Hypertension prevalence and control among adults: United States, 2015–2016. NCHS Data Brief. 2017 p. 1–8. * Carroll MD, Fryar CD, Nguyen DT. Total and high-density
lipoprotein cholesterol in adults: United States, 2015–2018. NCHS Data Brief, no 363. Hyattsville, MD: National Center for Health Statistics. 2020. Download references ACKNOWLEDGEMENTS We
warmly thank all the past and present members of the Gene Lifestyle Interaction Working Group. The various Gene-Lifestyle Interaction projects, including this summary project, are largely
supported by a grant from the U.S. National Heart, Lung, and Blood Institute (NHLBI), the National Institutes of Health, R01HL118305. This work was also supported by the INCEPTION project
(PIA/ANR-16-CONV-0005). This research was supported in part by the Intramural Research Program of the National Human Genome Research Institute, National Institutes of Health. PSV was
supported by American Heart Association grant number 18CDA34110116. YJS was supported by the K25HL121091 award from NHLBI. JG was partly supported by the P01CA196569 grant from the National
Institutes of Health. Full set of study-specific funding sources and acknowledgments were included in the separate publications [7,8,9,10,11]. AUTHOR INFORMATION AUTHORS AND AFFILIATIONS *
Department of Computational Biology, Institut Pasteur, Université de Paris, F-75015, Paris, France Vincent Laville & Hugues Aschard * Metabolism Program, Broad Institute of MIT and
Harvard, Cambridge, MA, 02142, USA Timothy Majarian & Alisa K. Manning * Division of Biostatistics, Washington University, St. Louis, MO, 63110, USA Yun J. Sung, Karen Schwander, C.
Charles Gu & D. C. Rao * Division of Statistical Genomics, Department of Genetics, Washington University School of Medicine, St. Louis, MO, 63108-221, USA Mary F. Feitosa, Aldi T. Kraja
& Mike Province * Division of Preventive Medicine, Department of Medicine, Brigham and Women’s Hospital, Boston, MA, 02215, USA Daniel I. Chasman * Center for Research on Genomics and
Global Health, National Human Genome Research Institute, National Institutes of Health, Bethesda, MD, 20892, USA Amy R. Bentley & Charles N. Rotimi * Department of Biostatistics, Boston
University School of Public Health, Boston, MA, 2118, USA L. Adrienne Cupples * Framingham Heart Study, National Heart, Lung, and Blood Institute, National Institutes of Health, Bethesda,
MD, 20982, USA L. Adrienne Cupples * Human Genetics Center, Department of Epidemiology, Human Genetics, and Environmental Sciences, School of Public Health, The University of Texas Health
Science Center at Houston, Houston, TX, 77030, USA Paul S. de Vries, Michael R. Brown & Alanna C. Morrison * Division of Biostatistics, Department of Population and Public Health
Sciences, University of Southern California, Los Angeles, CA, 90032, USA W. James Gauderman * Clinical and Translational Epidemiology Unit, Massachusetts General Hospital, Boston, MA, 02114,
USA Alisa K. Manning * Department of Medicine, Harvard Medical School, Boston, MA, 02115, USA Alisa K. Manning * Program in Genetic Epidemiology and Statistical Genetics, Harvard T.H. Chan
School of Public Health, Boston, MA, 02115, USA Hugues Aschard * Department of Computational Biology, Institut Pasteur, Université de Paris, F-75015, Paris, France Vincent Laville &
Hugues Aschard * Metabolism Program, Broad Institute of MIT and Harvard, Cambridge, MA, 02142, USA Timothy Majarian & Alisa K. Manning * Division of Biostatistics, Washington University,
St. Louis, MO, 63110, USA Yun J. Sung, Karen Schwander, C. Charles Gu & D. C. Rao * Division of Statistical Genomics, Department of Genetics, Washington University School of Medicine,
St. Louis, MO, 63108-221, USA Mary F. Feitosa, Aldi T. Kraja & Mike Province * Division of Preventive Medicine, Department of Medicine, Brigham and Women’s Hospital, Boston, MA, 02215,
USA Daniel I. Chasman * Center for Research on Genomics and Global Health, National Human Genome Research Institute, National Institutes of Health, Bethesda, MD, 20892, USA Amy R. Bentley
& Charles N. Rotimi * Department of Biostatistics, Boston University School of Public Health, Boston, MA, 2118, USA L. Adrienne Cupples * Framingham Heart Study, National Heart, Lung,
and Blood Institute, National Institutes of Health, Bethesda, MD, 20982, USA L. Adrienne Cupples * Human Genetics Center, Department of Epidemiology, Human Genetics, and Environmental
Sciences, School of Public Health, The University of Texas Health Science Center at Houston, Houston, TX, 77030, USA Paul S. de Vries, Michael R. Brown & Alanna C. Morrison * Division of
Biostatistics, Department of Population and Public Health Sciences, University of Southern California, Los Angeles, CA, 90032, USA W. James Gauderman * Clinical and Translational
Epidemiology Unit, Massachusetts General Hospital, Boston, MA, 02114, USA Alisa K. Manning * Program in Genetic Epidemiology and Statistical Genetics, Harvard T.H. Chan School of Public
Health, Boston, MA, 02115, USA Hugues Aschard Authors * Vincent Laville View author publications You can also search for this author inPubMed Google Scholar * Timothy Majarian View author
publications You can also search for this author inPubMed Google Scholar * Yun J. Sung View author publications You can also search for this author inPubMed Google Scholar * Karen Schwander
View author publications You can also search for this author inPubMed Google Scholar * Mary F. Feitosa View author publications You can also search for this author inPubMed Google Scholar *
Daniel I. Chasman View author publications You can also search for this author inPubMed Google Scholar * Amy R. Bentley View author publications You can also search for this author inPubMed
Google Scholar * Charles N. Rotimi View author publications You can also search for this author inPubMed Google Scholar * L. Adrienne Cupples View author publications You can also search for
this author inPubMed Google Scholar * Paul S. de Vries View author publications You can also search for this author inPubMed Google Scholar * Michael R. Brown View author publications You
can also search for this author inPubMed Google Scholar * Alanna C. Morrison View author publications You can also search for this author inPubMed Google Scholar * Aldi T. Kraja View author
publications You can also search for this author inPubMed Google Scholar * Mike Province View author publications You can also search for this author inPubMed Google Scholar * C. Charles Gu
View author publications You can also search for this author inPubMed Google Scholar * W. James Gauderman View author publications You can also search for this author inPubMed Google Scholar
* D. C. Rao View author publications You can also search for this author inPubMed Google Scholar * Alisa K. Manning View author publications You can also search for this author inPubMed
Google Scholar * Hugues Aschard View author publications You can also search for this author inPubMed Google Scholar CONSORTIA THE CHARGE GENE-LIFESTYLE INTERACTIONS WORKING GROUP * Vincent
Laville * , Timothy Majarian * , Yun J. Sung * , Karen Schwander * , Mary F. Feitosa * , Daniel I. Chasman * , Amy R. Bentley * , Charles N. Rotimi * , L. Adrienne Cupples * , Paul S. de
Vries * , Michael R. Brown * , Alanna C. Morrison * , Aldi T. Kraja * , Mike Province * , C. Charles Gu * , W. James Gauderman * , D. C. Rao * , Alisa K. Manning * & Hugues Aschard
CORRESPONDING AUTHORS Correspondence to Vincent Laville or Hugues Aschard. ETHICS DECLARATIONS COMPETING INTERESTS The authors declare no competing interests. ETHICAL APPROVAL The study did
not require ethical approval as no human subject was involved. ADDITIONAL INFORMATION PUBLISHER’S NOTE Springer Nature remains neutral with regard to jurisdictional claims in published maps
and institutional affiliations. SUPPLEMENTARY INFORMATION SUPPLEMENTARY TABLES SUPPLEMENTARY NOTES AND FIGURES RIGHTS AND PERMISSIONS OPEN ACCESS This article is licensed under a Creative
Commons Attribution 4.0 International License, which permits use, sharing, adaptation, distribution and reproduction in any medium or format, as long as you give appropriate credit to the
original author(s) and the source, provide a link to the Creative Commons license, and indicate if changes were made. The images or other third party material in this article are included in
the article’s Creative Commons license, unless indicated otherwise in a credit line to the material. If material is not included in the article’s Creative Commons license and your intended
use is not permitted by statutory regulation or exceeds the permitted use, you will need to obtain permission directly from the copyright holder. To view a copy of this license, visit
http://creativecommons.org/licenses/by/4.0/. Reprints and permissions ABOUT THIS ARTICLE CITE THIS ARTICLE Laville, V., Majarian, T., Sung, Y.J. _et al._ Gene-lifestyle interactions in the
genomics of human complex traits. _Eur J Hum Genet_ 30, 730–739 (2022). https://doi.org/10.1038/s41431-022-01045-6 Download citation * Received: 23 June 2020 * Revised: 22 December 2021 *
Accepted: 10 January 2022 * Published: 22 March 2022 * Issue Date: June 2022 * DOI: https://doi.org/10.1038/s41431-022-01045-6 SHARE THIS ARTICLE Anyone you share the following link with
will be able to read this content: Get shareable link Sorry, a shareable link is not currently available for this article. Copy to clipboard Provided by the Springer Nature SharedIt
content-sharing initiative