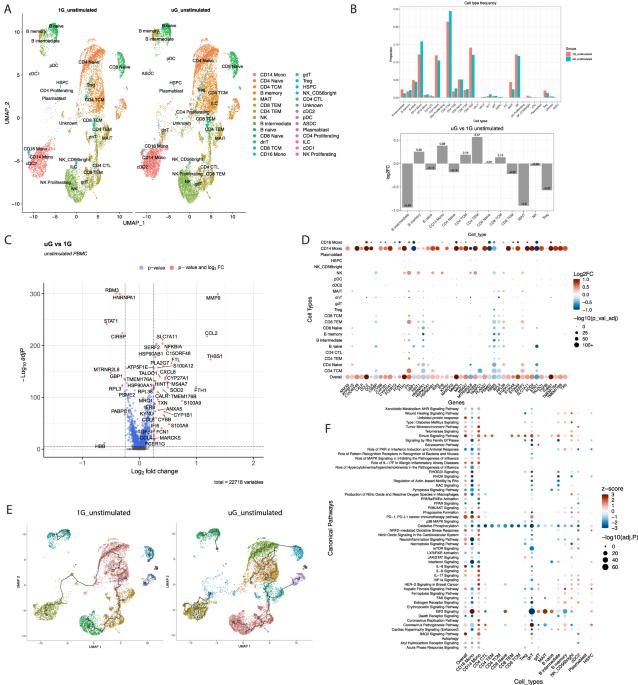
Single-cell analysis identifies conserved features of immune dysfunction in simulated microgravity and spaceflight
- Select a language for the TTS:
- UK English Female
- UK English Male
- US English Female
- US English Male
- Australian Female
- Australian Male
- Language selected: (auto detect) - EN
Play all audios:

ABSTRACT Microgravity is associated with immunological dysfunction, though the mechanisms are poorly understood. Here, using single-cell analysis of human peripheral blood mononuclear cells
(PBMCs) exposed to short term (25 hours) simulated microgravity, we characterize altered genes and pathways at basal and stimulated states with a Toll-like Receptor-7/8 agonist. We validate
single-cell analysis by RNA sequencing and super-resolution microscopy, and against data from the Inspiration-4 (I4) mission, JAXA (Cell-Free Epigenome) mission, Twins study, and spleens
from mice on the International Space Station. Overall, microgravity alters specific pathways for optimal immunity, including the cytoskeleton, interferon signaling, pyroptosis,
temperature-shock, innate inflammation (e.g., Coronavirus pathogenesis pathway and IL-6 signaling), nuclear receptors, and sirtuin signaling. Microgravity directs monocyte inflammatory
parameters, and impairs T cell and NK cell functionality. Using machine learning, we identify numerous compounds linking microgravity to immune cell transcription, and demonstrate that the
flavonol, quercetin, can reverse most abnormal pathways. These results define immune cell alterations in microgravity, and provide opportunities for countermeasures to maintain normal
immunity in space. SIMILAR CONTENT BEING VIEWED BY OTHERS SINGLE-CELL MULTI-OME AND IMMUNE PROFILES OF THE INSPIRATION4 CREW REVEAL CONSERVED, CELL-TYPE, AND SEX-SPECIFIC RESPONSES TO
SPACEFLIGHT Article Open access 11 June 2024 HUMAN IMMUNE SYSTEM ADAPTATIONS TO SIMULATED MICROGRAVITY REVEALED BY SINGLE-CELL MASS CYTOMETRY Article Open access 07 June 2021 IMMUNOLOGICAL
AND HEMATOLOGICAL OUTCOMES FOLLOWING PROTRACTED LOW DOSE/LOW DOSE RATE IONIZING RADIATION AND SIMULATED MICROGRAVITY Article Open access 01 June 2021 INTRODUCTION Astronauts in low earth
orbit (LEO), such as on the international space station (ISS), experience immune dysfunction associated with the microgravity environment. Multiple studies have described immune
dysregulation in short or long-term simulated1,2,3,4 or actual microgravity5,6,7,8,9. For the most part, such studies have described impaired T-cell responses, coupled with some form of
heightened innate immunity7,10, though some innate immune cells, like natural killer (NK) cells, also show impaired function11. Consistent with altered adaptive immunity, potentially due to
impaired cytotoxic and Th1 T cell function, and reduced NK cell function, astronauts develop increased reactivation of latent viruses, including herpes viruses (EBV, CMV,
VZV)3,7,12,13,14,15. In one study, viral shedding after 9–14 days of spaceflight was linked to changes in serum cytokines, including a preferential large increase in IL-4 compared to
interferon (IFN)γ, indicating a possible shift away from Th1 immunity towards Th2 immunity16. Consistently, some astronauts report heightened hypersensitivity reactions, such as increased
allergic and Th2-like responses in space7. Multiple studies using higher throughput approaches have started to add insight into pathways impacted by spaceflight. In the Twins study17, a
one-year ISS mission altered innate, adaptive, and NK cell-mediated immunity across bulk RNA sequencing analysis. In T cells, increases in DNA methylation were seen in the promoters of
_notch3_ for CD4+ T cells, linked to T cell differentiation, and in _scl1a5/asct2_, linked to activation, for CD8+ T cells. A total of 50 of 62 assayed cytokines were also altered by
spaceflight or landing17. During a recent multi-omic analysis, including bulk RNA and DNA methylation sequencing, of astronauts and mice in space, mouse organs such as the liver and kidney
demonstrated reduced IFN signatures, coupled to altered methylation patterns of these gene sets, while muscles had increased IFNγ, IL-1, and TNF10. Serum inflammatory markers from 59
astronauts in this study (and in a similar companion study) showed increased VEGF-1, IGF-1, and IL-1 during spaceflight, which resolved upon returning to Earth4,10. This same study also
identified mitochondrial dysfunction as a major response of different non-hematolymphoid tissues to spaceflight10. More recently, another study using a NASA-developed Rotating Wall Vessel,
which was employed in our current work, utilized a 41-parameter mass cytometry approach to show that short-term (18–22 hours) simulated microgravity can dampen NK cell, CD4+, and CD8+ T cell
responses to Concanavalin A/anti-CD28 stimulation, but potentiates STAT5 signaling to boost Tregs18. Despite these important advances, the core fundamental mechanisms, genes, and pathways
that are directly altered by microgravity to adversely impact immunity, including at single-cell resolution, are largely unknown. Interestingly, mechanical forces are emerging as critical
orchestrators of immune cell function, whereby mechanotransduction tunes immune cell responsiveness to danger signals19. Some of these effects occur through environmental modulation of
mechanosensing pathways that alter ion currents in cells, metabolism, or directly act on the cytoskeleton19. Thus, a spaceflight environment, which alters forces such as gravity, associated
hydrostatic pressure, and shear force20,21 onto immune cells likely directly contributes to immune system dysfunction. Here, using a common ground-based analog, the NASA developed low shear
modeled microgravity Rotating Wall Vessel (RWV)2,18,22, we examine in depth how short-term (25 hours) exposure to simulated microgravity impacts the human peripheral blood mononuclear immune
system in detail at single-cell resolution. Combining this data with validation experiments from mice and crewmembers in LEO, as well as machine learning algorithms, we identify numerous
core genes and pathways in immune cells that are altered by simulated microgravity or spaceflight, and identify numerous potential compounds that directly map onto immune cell
transcriptional signatures in simulated microgravity. RESULTS SIMULATED MICROGRAVITY ALTERS THE TRANSCRIPTIONAL LANDSCAPE OF INDIVIDUAL IMMUNE CELLS To begin understanding how simulated
microgravity impacts immune cell function, we loaded PBMC samples from two young healthy CMV+ donors, one male, and one female, into either RWV simulated microgravity (uG) or normal gravity
(1G) static controls for 16 hours of conditioning. The 16-hour-conditioning time point was chosen based on prior work that used approximately the same time and tracked transcriptional or
proteomic changes on immune cells to simulated microgravity1,18. PBMCs were either left unstimulated or stimulated for an additional 9 hours with R848, a standard TLR7/8 agonist. We chose
TLR7/8 as a putative target since it mimics viral infection, and is expressed on most human immune cells, including T cells23. Using this methodology, we next developed a single-cell atlas
of 55,648 human PBMCs exposed to these conditions. In the unstimulated state, after 25 hours of simulated microgravity, we identified 28 clusters of immune cells visualized by UMAP (Uniform
Manifold Approximation and Projection), including cell types such as mucosal associated invariant T cells (MAIT cells), double negative T cells, γδ T cells, innate lymphoid cells, and
plasmacytoid dendritic cells, which have rarely been studied in simulated microgravity (Fig. 1A). Simulated microgravity altered proportions of immune cell clusters to a mild extent, with B
intermediate cells, and MAIT cell proportions being most negatively impacted, and CD14+ monocytes, and CD4+ T effector memory (TEM) cells being most increased based on percent change (Fig.
1B). Across all immune populations, simulated microgravity altered expression of over 4500 genes with adj _P_ cutoff of <0.05 (Supplementary Data 1). This list was refined to a core list
of ~375 differentially expressed genes (DEGs) with an additional cutoff of |log2FC|> 0.1. This list was further condensed to visualize on a Volcano plot with |log2FC|> 0.25 (Fig. 1C),
showing only the very top positively and negatively altered genes. Volcano plots of DEGs for individual immune cell clusters are shown in Supplementary Fig. 1. Across all immune cells, some
of the most induced genes in simulated microgravity included acute response genes such as _s100a8_, _s100a9_, _s100a12_, _thbs1_, heat-shock genes such _hsp90ab1_, chemokines like _ccl2_,
_ccl4_, iron storage genes (_fth1_, _ftl_), and matrix metalloproteinases (_mmp9_). The most reduced genes in simulated microgravity included interferon response (_stat1_) and associated
guanylate binding proteins (_gbp1_), and cold shock genes (_rbm3_, _cirbp_). Expression of the top DEGs (with mitochondrial encoded genes excluded for visual simplicity) across 22
populations of immune cells are shown in Fig. 1D. CD14+ classical monocytes, CD16+ nonclassical monocytes, and natural killer (NK) cells exhibited the most pronounced changes across major
gene sets, consistent with short term simulated microgravity’s direct effect at reprogramming transcriptional changes most prominently in innate immunity. Consistently, using single-cell
trajectory analysis, we identify numerous trajectories mainly in the innate immune cell clusters, especially the monocyte cluster, in response to simulated microgravity. Trajectory analysis
is used to construct a path that describes how cells move through different states, and the numerous states seen in the monocyte cluster in simulated microgravity may reflect an increased
capacity to generate distinct transcriptional states to simulated microgravity (Fig. 1E). Ingenuity pathway analysis (IPA) (Fig. 1F, Supplementary Data 2) generated using our core list of
375 genes from the overall populations, as well as the DEGs in major immune cell types (Supplementary Data 3) revealed that monocytes, conventional dendritic cells type 2 (cDC2)s, double
negative (dn)T cells and NK cells show the most notable pathway alterations. Major pathways altered by simulated microgravity across immune cells included reductions in oxidative
phosphorylation, interferon signaling like protein kinase R (PKR) in interferon response, nuclear receptor signaling (LXR/RXR, PPAR, AHR), RHOA and pyroptosis signaling, as well as increases
in BAG2 (heat-shock protein 70 interactor) signaling, fibrosis signaling, actin-based motility, RAC, HIF1 signaling, acute phase response, oxidative stress and sirtuin signaling, amongst
others. Given that multiple pathways we detected were associated with inflammatory processes linked to aging (i.e., increased innate immunity coupled to reduced adaptive immunity), we next
determined whether acute exposure to simulated microgravity mimicked inflammatory aging processes in immune cells. We mapped the gene expression signatures of individual immune cells, and
overall immune signatures, against two recently developed inflammatory signatures of aging, the inflammatory age (iAge) clock24, and the SenMayo list of senescence associated secretory
inflammatory products25. Simulated microgravity induced a significant enrichment in inflammaging related genes, consistent with the notion that short term simulated microgravity can induce
aging-like inflammatory changes in unstimulated immune cells (Fig. 2A, B, Supplementary Fig. 2A). Next, because both spaceflight and aging are associated with reactivation of latent viruses,
we mined the meta-transcriptome of our single-cell analysis with meta-transcriptome detector (MTD) pipeline26. Surprisingly, we saw that as little as 25 hours of simulated microgravity
could induce the transcription of latent retroviruses and mycobacteria within human immune cells (Fig. 2C, Supplementary Figs. 2B, C and 3), directly implicating microgravity itself as a
contributing trigger for latent pathogen activation. We confirmed the meta-transcriptome results with a different alignment tool, and we could still detect increases in _Gammaretrovirus_ and
_Mycobacterium canettii_ transcripts seen with MTD pipeline (Supplementary Fig. 2B, C). Finally, as we identified strong changes in gene expression pathways linked to innate cells,
including those with the capacity to present antigen, we leveraged this knowledge to utilize NicheNet27 algorithms to generate a comprehensive predicted ligand:receptor interactome map of
human antigen-presenting cells (APCs, plus plasmacytoid dendritic cells) and T cells in simulated microgravity vs 1G (Fig. 2D–F). Across APC donors and recipient T cells, we identified
numerous significantly predicted ligand-receptor interactions to be elevated in simulated microgravity vs 1G. For instance, monocytes and dendritic cells induced IL-1 proteins while some B
cells provided IL-23A, and IL-7. All APCs provided unique chemokine signals to T cells. _Mmp9_, _ccl2,_ and _thbs1_ were amongst our most significantly induced genes in simulated
microgravity, and the products of these genes show differential predicted receptor expression (e.g., CD44, CD47, ITGB1, CCR4, CCR5) in T cells (Supplementary Figs. 4–6) but all show
predicted enhanced target gene expression in T cells. Thus, while simulated microgravity itself likely induces direct transcriptional changes in immune cells, we cannot exclude local
paracrine effects of secreted products from one immune cell to another also contributing to our overall gene expression and pathway changes. After stimulating PBMCs with a TLR7/8 agonist in
1G and simulated microgravity, we characterized 23 clusters of immune cells by UMAP (Fig. 3A). In contrast to the unstimulated conditions, stimulation with a TLR7/8 agonist induced a robust
preferential expansion of CD4+ central memory (TCM) cells (Fig. 3B). The microgravity itself impacted differential response to stimulation. Consistent with previous reports, simulated
microgravity dampened expansion/responses of NK cells, and CD8+ TEM cells to a lesser extent in the donors examined18, as well as MAIT cell numbers, a cell type with previously unknown
responses to microgravity. Simulated microgravity drove a preferential increase in CD14+ monocytes over 1G controls, indicating that this cell type is especially sensitive to the combination
of simulated microgravity and TLR7/8 activation. Across all cell types, the combination of simulated microgravity and TLR7/8 stimulation altered the expression of over 9000 differentially
expressed genes (DEGs) with adj _P_ cutoff of <0.05 (Supplementary Data 4). As with the unstimulated data, we refined this list to a core gene list of ~317 DEGs based on |log2FC| of
>0.2. This list was further reduced to visualize on a Volcano plot with |log2FC|>0.25 (Fig. 3C), showing only the most positively and negatively altered genes. Some of the most induced
genes by simulated microgravity over 1G in response to TLR7/8 agonist included cytokines and chemokines, such as _ccl8_, _ccl4_, _ccl7_, _cxcl8_, and _il1b_, and acute response proteins
like _s100a8_, _s100a9_, _s100a11_, and _thbs1_. Additional genes induced in simulated microgravity were linked to tryptophan breakdown (_ido1_), mitochondrial antioxidant defense (_sod2_),
the cytoskeleton (_rhoq_), and iron storage genes like _fth1_, _ftl_. The most downregulated genes when comparing simulated microgravity to 1G during TLR7/8 stimulation, included genes
belonging to guanylate binding proteins (_gbp1_, _gbp2_, _gbp4_, _gbp5_), which were the most reduced set of genes by fold change and adj P, as well as interferon pathway genes, like _irf1_,
_stat1_, _isg20_, _ifi16_, cold shock genes (_rbm3_, _cirbp_), cell killing genes (_prf1, gzmb_) and T/NK cell activation markers like _cd69_. Many of these genes were consistently altered
by simulated microgravity alone without stimulation, indicating a conserved response, even in the setting of additional exogenous stimulation with a TLR ligand. Expression of top DEGs across
19 populations of immune cells is shown in Fig. 3D. Volcano plots of DEGs for individual immune cell clusters are shown in Supplementary Fig. 7. CD14+ monocytes, NK cells, CD8+ TEM, and
CD4+ TCM cells showed the most significant changes in the top most altered genes induced by TLR7/8 agonist stimulation in simulated microgravity. Interestingly, using single-cell trajectory
analysis (Fig. 3E), we identified fewer trajectories in simulated microgravity stimulated with TLR7/8 compared to the 1G control. These findings suggest that under simulated microgravity,
cells display reduced differentiation states in response to stimulation. IPA results (Fig. 3F, Supplementary Data 5) generated using our core list of approximately 317 genes from the overall
populations, as well as the DEGs in major immune cell types (Supplementary Data 6) demonstrated that nearly all immune cells show changes across numerous pathways during microgravity and
TLR7/8 induction. Major pathways reduced across most immune cells in simulated microgravity included PKR in interferon response (and associated eif2 signaling), interferon signaling,
JAK/STAT signaling, pyroptosis signaling, cytotoxic T cell mediated killing of target cells and death receptor signaling. Major pathways induced by short term simulated microgravity included
sirtuin signaling, fibrosis signaling, signaling by Rho GTPases, BAG2 (heat-shock protein 70 interactor) signaling, HIF1α signaling, acute phase response and associated HMGB1 signaling,
amongst others. These pathways are consistent with microgravity facilitating innate like inflammation at the expense of interferon driven adaptive immunity and adaptive immune effector
function (e.g., CD8+ T cell killing). Despite some similarities in pathways altered to simulated microgravity alone (Fig. 1F), we actually detected a lower iAge score globally across all
immune populations in simulated microgravity plus TLR7/8 compared to 1G controls (Fig. 4A). While the reason for this finding is unclear, it appears to have been driven by a highly
significant reduction in score by naive B cells, naive CD4+ T cells, and reductions in lesser studied PBMC populations, hematopoietic stem and progenitor cells (HSPC)s and double negative T
cells. Lower iAge also could be reflective of altered immune activation in simulated microgravity, such as seen in CD16+ monocytes (Fig. 4A, right panel). Despite a reduction in iAge, we
still observed an increased SenMayo score in simulated PBMCs (Fig. 4B, Supplementary Fig. 8), illustrating different compositions of genes in these two gene sets. NicheNet analysis across
major APC types to T cells post TLR7/8 agonist in simulated microgravity vs 1G (Fig. 4C–E, and Supplementary Figs. 9–11) illustrated some of the significant cytokines, chemokines, surface
molecule ligands and receptors used in simulated microgravity upon TLR7/8 stimulation. Compared to the unstimulated interactome (Fig. 2D–F), we saw increased production and diversity of
inflammatory cytokines and chemokines used. We again noted IL-1 produced in APCs, but also noted increased TNF superfamily products like TNF, TNFSF12 (TNF-related weak inducer of apoptosis,
TWEAK) and TNFSF15 (vascular endothelial growth inhibitor, VEGI), and lymphotoxin (LTA) preferentially produced to modulate T cell function. Next, we assessed the differential responsiveness
of immune cells to TLR7/8 stimulation (Supplementary Fig. 12 and Supplementary Data 7). Under 1G conditions, stimulation led to a marked induction of CD4+ TCM, at the expense of CD14+
monocytes and CD4+ naive T cells, coupled to an expected pronounced inflammatory gene signature, including marked induction of interferon inducible genes, _gbp_ transcripts, and chemokines
across most immune cell populations (Supplementary Fig. 12A–E). Under simulated microgravity, TLR7/8 stimulation also induced CD4+ TCM, at the expense of naive CD4+ T cells, though
proportions of CD14+ monocyte populations did not reduce as seen in 1G (Supplementary Fig. 12F, G). In simulated microgravity, TLR7/8 stimulation also induced a robust expression of
inflammatory genes, including interferon inducing genes across most cell types (Supplementary Fig. 12H–J). Next, to determine the sensitivity of individual immune cell populations to TLR7/8
agonist in 1G vs simulated microgravity, we compared the differences in responsiveness to stimulation. We subtracted the fold change induction in 1G from induction in simulated microgravity
to determine sensitivity to stimulation. Remarkably, across overall immune cells, we see a pattern of reduced responsiveness to TLR7/8 simulation in simulated microgravity to most of the
highest genes induced at 1G (Supplementary Fig. 13A), with T cells and NK cells showing the most reduced inflammatory gene induction. T cells, NK cells, and overall across all cells
exhibited blunting of induction of numerous genes in interferon signaling, and _gbp_ genes in simulated microgravity in response to TLR7/8 agonist. Interestingly, monocytes tended to
maintain such responses better in simulated microgravity, consistent with their predisposition to some inflammatory pathways in simulated microgravity. Some chemokines, such as _ccl3_,
_ccl4_, _ccl8_, and _cxcl10_ appeared to be induced better in simulated uG across overall immune cell populations, though monocytes actually showed reduced induction of some of these
chemokines, likely due to their capacity to produce them in simulated microgravity without stimulation (Fig. 1D). Nonetheless, the overall effects in sensitivity to stimulation in the
“overall” category of immune cells largely followed the same pattern seen in the total magnitude of response of stimulated microgravity vs stimulated 1G (Fig. 3D). Next, we sought to
identify genes uniquely altered by simulated microgravity irrespective of stimulation, as well as genes unique to microgravity under stimulation. First, to identify unique genes, regardless
of stimulation, altered by simulated microgravity, we plotted out the most significant DEGs by the absolute sum of fold change under both stimulated and unstimulated conditions
(Supplementary Fig. 13B, Supplementary Data 8). Across overall immune cells, regardless of stimulation, we still identified conserved increases in chemokines and acute response factors,
coupled to reduced _gbp_ expression and other interferon genes (e.g., _irf1_, _stat1_) imparted by simulated microgravity. Next, we mapped out the overall overlap of gene signatures and
common genes between post-stimulation in 1G vs simulated microgravity (Supplementary Fig. 13C–E and Supplementary Data 9), and found that microgravity imparts a number of unique genes to TLR
ligation that are not seen in 1G. Overall, these findings identify core-conserved DEGs specifically sensitive to simulated microgravity as well as unique signatures to simulated
microgravity. Finally, we assessed if sex plays a role in the magnitude of response to simulated microgravity. In the unstimulated state, female cells showed only slightly more DEGs induced,
while the male cells had slightly more genes reduced (Supplementary Fig. 14A). Male NK cells and monocytes were more sensitive to microgravity while female B cells showed more sensitivity
than male B cells. Upon stimulation, male cells overall were more sensitive to simulated microgravity, especially in having more downregulated DEGs (Supplementary Fig. 14B). Volcano plots of
DEGs across all cell types between the female and male are shown in Supplementary Fig. 14C–F and Supplementary Data 10. In both male and female cells, acute phase response and inflammatory
genes like _mmp9_, _ccl2_, _s100a8_, and _thbs1_ were among the most induced genes, while reduced interferon regulators like _stat1_ and reduced cold shock genes like _rbm3_ and _cirbp_ were
consistently downregulated in both sexes in simulated microgravity. Upon stimulation, both sexes again show increases in the total magnitude of acute inflammatory, reactive oxygen species
(ROS)-related, and acute phase genes like chemokines, _thbs1_, _mmp9_, _ncf1,_ and _sod2_ in simulated microgravity coupled to reduced interferon, _gbp_s, cold shock, and some ribosomal
protein genes in simulated microgravity. Many of these changes are reflected in IPA pathway analysis by sex (Supplementary Fig. 14G) and many of these core features were also conserved when
data from sexes were pooled (Figs. 1F, 3F). SINGLE-CELL VALIDATION IDENTIFIES CORE FEATURES OF IMMUNE DYSFUNCTION IN MICROGRAVITY AND SPACEFLIGHT Next, to better validate conserved genes and
pathways from our single-cell signatures without TLR7/8 agonist in simulated microgravity, we compared our core signature of 375 DEGs against additional datasets. First, we repeated
experiments in a validation cohort of freshly isolated PBMCs from young donors (_n_ = 6, age range 20–46), and spun these PBMCs for 25 hours, prior to performing bulk RNA-seq analysis. Using
CIBERSORTx28, we first mapped predicted changes in population frequency and were able to confirm increased CD14+ monocyte frequencies in simulated microgravity, consistent with our
single-cell data (Supplementary Fig. 15, Supplementary Data 11). Between validation samples spun in simulated microgravity vs 1G controls, we identified 2149 genes differentially expressed
(Fig. 5A, Supplementary Data 12). Despite the variability of data inherent to bulk RNA-seq of different populations of cells between donors, we still saw a highly significant correlation in
normalized gene counts per specific gene between datasets both at 1G and in simulated microgravity (Fig. 5B). Moreover, we identified overlap in over 28% of our core signature genes (same
directionality) across all immune cells from our single-cell analysis (106/375 = 28.3%) (Fig. 5C). Many of the overlapping genes induced were consistent with our most robustly altered core
pathways from the single-cell data. For instance, we saw shared overlapping genes induced in acute immune responses (such as _s100a8_, _s100a12_, _thbs1, il1b_), chemokines (like _cxcl8_),
heat-shock proteins (_hsp90aa1_, _hspa1a_, _hspb1_), autophagy (_atg7_) and the actin cytoskeleton (_rhou_). Overlapping reduced genes in simulated microgravity, like in our single-cell
datasets, included interferon response (_stat1, irf1_) and associated guanylate binding proteins (_gbp1_, _gbp2_, _gbp4, gbp5_), and cold shock genes (_rbm3_), amongst others. Overall, there
was a highly significant enrichment and over representation of our core single-cell DEGs across our bulk validation cohort by Fisher’s Exact Test for gene overlap (Fig. 5C). We next sought
to validate overlapping genes against mice and people flown in LEO. While multiple stressors exist in LEO, the proximity to Earth and the presence of the Earth’s magnetic field negates some
effects of galactic cosmic rays, especially at the altitude of the ISS. Thus, microgravity plays an important role in driving phenotypic changes in LEO. To accomplish this goal, we first
mined data from NASA’s GeneLab database for its largest study looking at a major immune organ, the spleen, in mice flown on the ISS. The GLDS-420 study provides data from the spleens of ten
mice housed on the ISS for 33 days compared to ten ground controls. Though this cohort represents longer exposure to microgravity than our single-cell data’s more acute exposure, any
overlapping genes could represent persistent microgravity-sensitive immune cell genes across longer duration exposure. From the GLDS-420 dataset, we identified 1448 significant DEGs (Fig.
5D), of which 50/375 (13.3%) overlapped in the same direction as our single-cell core list (Fig. 5E). Interestingly, many of the overlapping genes were represented as part of altered core
pathways from the single-cell data. For instance, we saw shared induced overlapping genes in acute immune responses or complement (such as _c3_), autophagy (_atg7_), heat-shock responses
(_hsp90ab1_, _hsp90aa1_, _hspa1a_, _hspa1_), and the cytoskeleton (_dynll1_). Overlapping reduced genes included interferon response (_stat1_), and again, cold shock genes (_cirbp_, _rbm3_),
amongst others. Overall, we saw a significant enrichment in our core single-cell DEGs across mouse spleens flown in space by Fisher’s Exact Test for gene overlap (Fig. 5E). Pathway analysis
with IPA was next performed to identify major canonical pathways altered across all four complete datasets (single-cell unstimulated, single-cell stimulated with TLR7/8 agonist, Bulk
RNA-seq validation unstimulated, and GLDS-420), including overlapping pathways shared across all datasets. These pathways are displayed in a heat map for comparison (see below), and will be
described at that point. To better translate the usefulness of our single-cell atlas to human spaceflight, we compared our core list of 375 DEGs across single cells in simulated microgravity
to changes across all single cells from the Inspiration Four (I4) crew members. The I4 mission provides a compelling comparison since crew members spent up to three days in LEO, a timeline
not too different from our 25-hour time point. Moreover, I4 gene lists were also generated by single-cell sequencing, making it a comparable technology for our analysis. However, it is
important to note that the I4 datasets contain a few important caveats. First, the altitude flown by I4 crew (585 km/364miles) predispose the astronauts to higher radiation exposures than
what would typically be experienced on the ISS (408 km/254miles altitude). Additionally, the I4 datasets were derived from PBMC gene expression comparisons between post-flight (1 day after
Return/R + 1 in our case) vs preflight (44 days before launch/L-44 in our case). Since the changes from the I4 single-cell data represent changes encompassing effects of spaceflight, plus
return to the ground, including short-term exposures to hyper-gravity, and one day of return to 1 g gravity (all which manifest as increased gravity exposure to inflight conditions), we
considered overlapping immune cell genes in either direction on return to be gravity sensitive genes. Remarkably, despite these caveats, we found a very robust overlap of nearly 60% of DEGs
in PBMCs in simulated microgravity (210/375 = 56%) to be also significantly altered across all immune cells in the I4 mission (Fig. 5F, Supplementary Data 13). Of these significantly altered
genes across I4 data, 122 were altered in the same direction as I4 data, and 88 in the opposite direction. To gain a better understanding of the pathways and mechanisms impacted by gravity
and spaceflight in the immune system, we compared pathways between simulated microgravity and the entire I4 dataset (Fig. 5G, Supplementary Data 14, and Supplementary Fig. 16A). While we
consider all of these pathways to be potentially gravity sensitive, we considered pathways altered in the opposite directions to be potentially acutely sensitive to gravity, while those
pathways altered in the same direction likely take longer to normalize from a microgravity environment upon return. Both simulated microgravity and the I4 mission pathway results indicated
reduced T-cell effector subset development, reduced oxidative phosphorylation, and increased pathways associated with innate immunity (e.g., Coronavirus pathogenesis, FcR phagocytosis in
monocytes, cytokine storm, chemokine signaling, ROS production in macrophages), as well as hypoxia and glycolytic metabolism (HIF1α signaling) and cell stress (e.g., sirtuin signaling).
Interestingly, the return to gravity seen in the I4 mission reversed reductions in natural killer cell signaling and reversed pathways linked to poor adaptive immunity like IL-15 signaling,
suggesting these pathways may be sensitive to acute changes in gravity (Fig. 5G, Supplementary Data 14). From the I4 dataset, we also noticed a consistent reduction in ribosomal subunit
genes in the I4 data (Fig. 5F), which might be reflective of a stress response and reduced protein translation upon return to earth), and only some of these genes were reduced in simulated
microgravity. Consistently, there was a marked reduction in EIF2 signaling in the provided I4 DEGs. Across all pathways, regardless of direction, we noticed many pathways pertaining to the
cytoskeleton or to a mechanical extracellular environment (e.g., fibrosis, RAC, Rho family GTPases, RHOA, integrin signaling, leukocyte extravasation signaling, healing signaling etc.) to be
altered by simulated microgravity or by spaceflight on immune cells. Next, to look for overlapping genes relevant to longer exposures to human spaceflight, we compared our core simulated
microgravity signature of immune cells against available data from the JAXA Cell-Free Epigenome study in LEO (GLDS-530) and the Twins study17. For the JAXA mission, we had access to
cell-free RNA data, which can sometimes give insight into changes to PBMCs, amongst other cells29. During this mission, blood was sampled from 6 astronauts, with data pooled into a single
count, at days 5, 30, 60, 120 post launch. Given that cell-free RNA is not a fully ideal comparison to RNA-seq from isolated PBMCs, we focused only on the two early time points, 5 days and
30 days post launch, because we saw significant overlap between our single-cell data in both the three-day I4 mission and in the 33-day GLDS-420 dataset. Thus, we compared 5 days and 30 days
in-flight vs preflight differentially altered cell free RNA signatures for any possible overlap with our single-cell data in simulated microgravity. While we did not observe much overlap in
our core 375 immune gene signature at 5 days (less than 10 genes), we did see significant overlap by 30 days (42/375 = 11.2% overlap in the same direction) (Supplementary Fig. 16B).
Interestingly, it was observed that cell-free RNA levels generally decreased across most genes in flight. Consequently, we hypothesized that identifying genes exhibiting increased expression
could be particularly important for identifying over-represented processes. Remarkably, we did note that the most significantly elevated gene at 30 days in-flight vs preflight was _cdc42_,
a key modulator of the cytoskeleton, as well as _dynll1_ a dynein gene that was also upregulated in our single-cell analysis. We next reclustered our single-cell data (Supplementary Data 15)
to compare the DEGs in our equivalent single-cell populations with those obtained from sorted CD4+ T cells, CD8+ T cells, B cells, and lymphocyte-depleted immune cells from the NASA Twins
study, which compares in-flight vs ground twin control17. The Twins study provides intriguing data on the impact of LEO on the immune system, but has caveats in that exposure to LEO was
calculated in only a single individual through bulk RNA-seq, and at multiple time points across one full year in space, a different duration than our shorter gene sets. Nonetheless, compared
to our reclustered CD4+, CD8+, and CD19+ gene sets, we found significant overlap in some genes comparing the effects of simulated microgravity to spaceflight (Supplementary Fig. 17A–C).
Across multiple cell types, we saw changes in genes involved in redox regulation (e.g., reduced _txnip_), and in genes involved in interferon responses (e.g., reduced _stat1_ and _gbp5_).
Interestingly, we saw significantly reduced cold shock gene, _cirbp_, a similar functioning gene to _rbm3_, in B cells in space. In our lymphocyte depleted (i.e., myeloid) recluster, we saw
a large and highly significant overlap of about 163 genes with the Twins lymphocyte-depleted bulk RNA-seq data (Supplementary Fig. 17D). Many of the overlapping genes induced by simulated
microgravity or spaceflight included genes involved in innate immunity and inflammation (e.g., _il1b_, _s100a12_, _thbs1_ etc.), the cytoskeleton (_rhoq_, _rhou_), and hypoxia signaling
(e.g., _hif1α_). Some interesting downregulated genes in both datasets in myeloid cells included again _gbp5_, _cirbp_, _txnip_ like seen in T and B cells from the Twins study. We also noted
a number of overlapping downregulated genes in antigen presentation (e.g., _tap1_, _tap2_, _hla-e_, _hla-dp1a_, etc). IPA analysis on this data largely captured the increases in innate
immune inflammatory pathways, including increases in fibrosis signaling, IL-6 signaling, acute phase response, cytokine storm, and HIF1α signaling seen across some of our previous datasets
(Supplementary Fig. 17E, Supplementary Data 16). Overall, these data enforce the idea of classically activated basal myeloid inflammatory changes in microgravity and spaceflight. Given that
many of our altered pathways in simulated microgravity involved predicted mitochondrial dysfunction and/or the cytoskeleton, we used Airyscan super-resolution confocal microscopy to
characterize immune cell mitochondrial and actin morphological networks to look for alterations in simulated microgravity compared to 1G controls. Interestingly, while 25 hours of simulated
microgravity did not alter mean cell area across PBMCs, it did alter actin granularity parameters, as well as intensity and variance, consistent with cytoskeletal changes in acute simulated
microgravity (Fig. 6A–C), though these differences are mostly subtle to the naked eye. Using three-dimensional (3D) super-resolution imaging, 25 hours of simulated microgravity did not alter
cell or nucleus volume, or nucleus shape, but it increased mean cell surface area and actin spike length, and decreased sphericity of the cells across PBMCs (Fig. 6A, B, D, Supplementary
Fig. 18A, B). Remarkably, 1G immune cells and simulated microgravity immune cells demonstrate unique spectral changes to actin rearrangement post TLR stimulation, such that TLR stimulation
resulted in a different pattern of actin granularity spectral change in 1G compared to stimulation in simulated microgravity (Fig. 6C). The effect of microgravity on the cytoskeleton in
unstimulated immune cells was similar to the effect of TLR activation in 1G. During TLR stimulation in simulated microgravity, immune cells followed a unique dynamic actin rearrangement
pattern, potentially even reversing the pattern observed in 1G with TLR stimulation. These results suggest that simulated microgravity itself may induce immune cytoskeleton alterations,
which may mimic aspects of TLR ligation on the cytoskeleton. Short-term exposure to simulated microgravity showed some increases in the mitochondrial MitoTracker Red staining intensity and
variance in the unstimulated conditions, without changes to fiber length, size, or volume (Supplementary Fig. 18C). Since we detected morphological changes to the actin network, as well as
noting changes in multiple altered cytoskeleton-related pathways across multiple datasets, including in the pathways “Signaling by Rho Family GTPases” or “regulation of actin-based motility
by Rho”, we next sought to screen for active GTP-bound Rho GTPases, Rac1, RhoA, and Cdc42, using G-LISA technology30 across further batches of isolated paired PBMCs. After 25 hours of
simulated microgravity, regardless of stimulation conditions, we saw elevated levels of active GTP-bound Cdc42, consistent with cytoskeleton mobilization and the increase in actin spikes
(indicative of filopodia)31 observed due to simulated microgravity (Fig. 6E). Active GTP-Rac1 was not altered at baseline in simulated microgravity, though showed a trend to induction with
TLR7/8 stimulation (Supplementary Fig. 18D, E). Levels of active GTP-RhoA were low in our samples, but trended lower in simulated microgravity without stimulation, and higher under
stimulation, analogous to our single-cell data predictions (Supplementary Fig. 18D, E). Overall, these data suggest that simulated microgravity changes some Rho GTPase activity consistent
with our transcriptional data, though ultimate impacts on cytoskeleton shape, variance, and dynamics likely involve additional contributing factors, including possibly other Rho GTPase
family members not assessed. We next sought to investigate our core signature of reduced IFN signaling elicited in microgravity across immune cells (Fig. 6F, Supplemental Fig. 19A, B).
Specifically, we assessed whether reduced interferon signaling was due to reduced local production of interferons. Supernatants from 25 hours unstimulated or 9 hours R848 simulated (25 hours
total culture) PBMCs were assessed by ELISA for total IFNα (detecting 12 IFNα subtypes), and IFNγ. Simulated microgravity significantly reduced both IFNα and IFNγ secretion with TLR7/8
stimulation. At baseline, the levels of these cytokines were low, and variable, and thus not significantly different between 1G and simulated microgravity. These findings point to reduced
production of IFNs in simulated microgravity, at least under TLR stimulation, as measured by ELISA, potentially as one contributing mechanism to reduced interferon signaling observed at the
transcriptional level. Finally, to functionally validate how simulated microgravity impacts overall immune cell cytokine production, with and without TLR7/8 stimulation, across many
cytokines simultaneously, we performed a 48-plex Luminex assay on cytokines secreted by PBMCs from 12 donors (Supplementary Fig. 19C, D). Consistent with our single-cell and bulk RNA
sequencing data, simulated microgravity was associated with increased or trending increases in mainly innate/monocyte immune cell-derived inflammatory cytokines and chemokines (e.g., IL-6,
IL-8, IL-12p40, CCL4), coupled to a reduction in cytokines that associate with T cell activation or proliferation (e.g., IL-2, IL-7, IL-15). Concurrently, the Luminex results showed a
significant IFNγ and a trending IFNα2 reduction upon TLR7/8 agonist stimulation in simulated microgravity, consistent with our above ELISA data (Fig. 6F). IL-1, commonly induced in our
sequencing data, also appeared elevated in simulated microgravity, though it exhibited high variability, precluding significance in the unstimulated state. In the stimulated state, IL-1β was
significantly increased in simulated microgravity by Luminex analysis. Given the overlapping similarities between cytokines in the Luminex data and sequencing data for IL-1β, IL-6, and
IL-8, we further assessed these cytokines by ELISA validation. Both IL-6 and IL-8 showed significant or near-significant increases by ELISA in simulated microgravity, while IL-1β
demonstrated a trending increase (Fig. 6G, Supplementary Fig. 19E). Upon stimulation, simulated microgravity further facilitated near-significant increases in IL-1β and IL-8 as validated by
ELISA (Supplementary Fig. 19F). To better understand how certain cell populations respond to TLR7/8 stimulation in simulated microgravity, we further validated key cytokines, IL-1β, IL-6,
and IFNγ, by intracellular flow cytometry in monocyte, NK, and T cell subsets exposed to simulated microgravity compared to 1G conditions (Supplementary Figs. 20, 21). Consistent with
Luminex and ELISA data, we saw increased IL-1β production across all characterized monocyte populations (Supplementary Fig. 21A, B). Interestingly, despite no overall differences in IL-6 by
Luminex or ELISA in simulated microgravity to TLR7/8 stimulation, we still detected significant increases in a subset of monocytes only, as well as in NK cells (Supplementary Fig. 21C, E).
Despite increased cytokine production, we did not detect increases in the activation marker, HLA-DR, in monocyte populations (Supplementary Fig. 21D). NK cells also showed a reduction in the
proportion producing IFNγ, as well as reduced proportions of expression in the activation marker, CD69, and degranulation marker, LAMP-1, consistent with reduced functionality and response
to stimulation in simulated microgravity (Supplementary Fig. 21F, G). T cell subsets were less altered, though we still detected near significant or significant reductions in the proportions
of CD4+ and CD8+ central memory T cells expressing activation marker, CD69, and in effector memory CD4+ T cells expressing proliferation marker, Ki67 (Supplementary Fig. 21H). Taken
together with our ELISA and Luminex data, these findings demonstrate that simulated microgravity, alone or in the presence of TLR7/8 agonist, can functionally alter cytokine production
across immune cells. In general, consistent with sequencing data, the features demonstrate monocyte inflammatory function coupled to impaired T cell and NK cell functionality in simulated
microgravity. Thus, changes in cytokine signaling observed in simulated microgravity may occur at least in part to changes in upstream cytokine production. REVERSING SIMULATED MICROGRAVITY
EFFECTS ON THE IMMUNE SYSTEM We have characterized multiple genes and pathways altered by simulated microgravity in the immune system; however, whether there are specific drugs or
supplements that can directly target microgravity effects on immune cells is poorly characterized. Thus, we have utilized an in-house compound-gene interactome machine learning technology
(Gene Compound Enrichment Analysis, GCEA), building on the HyperFoods model32, for the identification of drugs and food supplements that significantly map to altered genes in a dataset.
Overall, our pipeline assesses >2 million interactions between genes, drugs, and foods, across DrugBank, LINCS, and FoodDB32 (Fig. 7A). Using these algorithms across our core signature of
375 DEGs altered by simulated microgravity across the immune system, we identified 115 compounds with adj _p_ < 0.05, and 474 compounds with _p_ < 0.05 that significantly map to our
signature (Fig. 7B and Supplementary Data 17). Figure 7B shows the top 50 most significantly overlapping compounds to enriched DEGs. We next chose one compound, quercetin, based on its
widespread availability for future travelers to space, and for its prominence as an anti-aging supplement, to validate whether it can reverse transcriptional insults of microgravity on the
immune system. PBMCs (donors from the Fig. 5A cohort) were subjected to 25 hours in simulated microgravity, with or without quercetin (50 μM), for bulk RNA-seq analysis. Remarkably, at the
gene level, quercetin reversed the direction of expression of 70% (74/106) of the 106 genes (Fig. 5C) core signature generated as significantly overlapping genes between single-cell and bulk
RNA-seq validation (Fig. 7C). Reversal of gene expression was significant by correlation analysis (Fig. 7C) and demonstrated by GSEA enrichment plot (Fig. 7D). IPA pathway analysis was then
performed to characterize pathways altered by quercetin, and compared against non-treated bulk RNA-seq controls, as well as pathways altered across our other 3 major datasets, including
single-cell sequencing and GLDS-420 spleens in space. Overall, pathway analysis across all datasets showed consistent impacts of simulated and actual microgravity on pathways essential for
optimal immunity (Fig. 7E, Supplementary Data 18). Some of the most consistently induced pathways in simulated microgravity and/or space included “coronavirus pathogenesis pathway” (linked
to innate immune activation), acute phase responses, leukocyte extravasation signaling, IL-6 signaling, BAG2 signaling (linked to heat-shock proteins and proteostasis), sirtuin signaling,
and to a lesser extent “regulation of actin-based motility by Rho”, RAC signaling, PKA signaling, and oxidative stress response. Major pathways attenuated by microgravity were linked to
immunity, including antimicrobial immunity, pyroptosis signaling, as well as “interferon signaling” (including PKR in IFN induction). Other reduced pathways across most datasets included
reduced nuclear receptor activation (including LXR/RXR, PPAR, AHR) and reduced T cell NUR77 (activation) signaling. Interestingly, we noted some genes were consistently reduced across all
datasets, though were not properly represented in pathway analysis. The most striking of these genes is _rbm3_, a cold-shock protein, which was significantly reduced in all four of the
microgravity datasets, as well as in the I4 and JAXA mission (30-day timepoint). _Rbm3_ was also reduced in the Twins study inflight data across all sorted immune cells, though not reaching
significance. Administration of quercetin in simulated microgravity could reverse many of the altered transcriptional signatures elicited by simulated microgravity on the immune system (Fig.
7E). Some of the major pathways it could reverse include “regulation of actin-based motility by Rho”, leukocyte extravasation signaling, RAC signaling, LXR/RXR, PPAR signaling, NUR77
signaling in T cells, “coronavirus pathogenesis” (innate immunity), acute phase response, fibrosis, IL-6 signaling, amongst others. Though quercetin has gained prominence for its senolytic
properties33, our results show that reducing senescence pathways was only one of many (approximately 174) pathway effects this compound has on immune cells in simulated microgravity
(Supplementary Data 18). Nonetheless, in simulated microgravity, quercetin could reduce senescence and age-associated inflammatory gene outputs, as demonstrated by reductions in both the
SenMayo and iAge index scores (Fig. 7F). These changes occurred for the most part by downregulating inflammatory genes. Despite the marked transcriptional reversal in simulated microgravity
observed with one compound, quercetin failed to reverse reductions in interferon signaling, a major hallmark of microgravity on immune system dysfunction from our data. Other studies have
also linked microgravity and spaceflight to mitochondrial dysfunction and ROS production10,34,35. In this regard, quercetin also showed a robust capacity to reduce ROS levels after 25 hours
of simulated microgravity (Fig. 7G), though ROS was only marginally increased as a trend by simulated microgravity itself after 25 hours, likely due to the expression of endogenous
antioxidant systems at this timepoint34. Consistently, we also observed increased oxidative stress responses, such as NRF2-mediated or sirtuin signaling in many of our transcriptomic
datasets by IPA analysis (Fig. 7E). DISCUSSION Immune dysfunction during spaceflight is an important health risk, and manifests primarily as increased vulnerability to opportunistic
infections, including latent viral reactivation3. Latent viruses can reactivate on both short- and long-term spaceflights, and commonly involve herpes viruses (HSV1, EBV, CMV,
VZV)12,13,14,15. Astronauts also experience heightened skin sensitivity reactions3,13,36, and this mechanism was thought to be related to a possible Type 2 immune bias in space7,16,36.
Recent work in simulated microgravity has also shown reduced JAK/STAT signaling in CD8+ T cells, coupled to increased pSTAT5 signaling in Tregs. Despite these important advances, major
mechanisms explaining these phenotypes of immune dysfunction, in simulated microgravity have remained unclear. We have identified numerous core pathways and genes altered across human immune
cells in simulated microgravity, with validation against datasets of humans in LEO, as well as spleens from mice flow on the ISS. Overall, we noted changes consistent with basal innate
immune cell inflammatory changes in simulated microgravity, coupled with distinct pathways of dysfunction in multiple immune cells. Specifically, the most consistently reproduced pathways
impacted by simulated microgravity across immune cells in both single-cell and validation cohorts included changes to pathways and signaling linked to acute phase response signaling,
Coronavirus pathogenesis, IL-6 signaling, the cytoskeleton, interferon response, pyroptosis, heat-shock, nuclear receptors, and sirtuin biology. The link between the cytoskeleton and other
pathways here may be especially relevant in immune dysfunction. Cytoskeleton dynamics are controlled by a number of factors, but small GTPases, including Ras homology (Rho) GTPases, are
major orchestrators with critical impact on immune cell function, migration, gene expression, trafficking, phagocytosis, proliferation, and antigen recognition31. Of note, Rho GTPases have
been implicated in response to simulated microgravity in other cell types, but this connection is understudied in immune cells37. Across most datasets, we saw changes to Rho GTPase
signaling, including individually in RAC, RHOA, or CDC42 signaling, or combined in a global “regulation of actin-based motility by Rho” pathway in IPA. While we did notice some variability
between our initial unstimulated vs stimulated single-cell data in these pathways, these pathways tended to show reduced RHOA signaling without stimulation, coupled with increased RAC
signaling, analogous to what we observed from the I4 crew members upon landing. The JAXA6 dataset also demonstrated _cdc42_ to be the most significantly induced cell-free transcript in
astronauts after 30 days in space. Pathways strongly linked to cytoskeletal remodeling, such as leukocyte extravasation, were also typically induced in most of our datasets. We also observed
changes in some active Rho GTPases by G-LISA, as well as in F-actin granularity, variance, 3D surface area, sphericity, actin protrusion length, and dynamic change to TLR stimulation by
super-resolution microscopy, providing further evidence for changes in actin, including possibly immune cytoskeleton alteration or dysfunction, in simulated microgravity. Importantly,
changes to the actin cytoskeleton are now being linked to the ability of an immune cell to mount an interferon response. Indeed, danger-sensing molecules like TLRs utilize Rho GTPases to
facilitate IFN responses38,39, or antiviral sensors can directly modulate actin rearrangement40. One example is the PKR antiviral response, which was consistently downregulated by simulated
microgravity in our datasets. In this system, PKR binds gelsolin to enforce basal innate immune defense, though upon viral sensing, PKR dissociates from gelsolin, leading to the severing of
actin, and activation of RIG-I-like receptor (RLR)s signaling and interferon response41. Other antiviral sensors like RIG-I directly bind F-actin in resting cells, and then relocalize to the
mitochondria via actin rearrangements on viral infection, to induce type 1 IFN42,43. In single-cell data, reduced interferon signaling without stimulation was seen mainly in monocytes,
linking it to innate immunity, though with TLR7/8 stimulation, reduced interferon signaling was seen across many cells, including most T cell subsets, and NK cells, displaying the broad
importance of this pathway across most immune cells to microgravity. In simulated microgravity, we saw reduced IFNα production by ELISA with stimulation, so we cannot rule out the
possibility that the reduced IFN signaling seen in simulated microgravity starts with reduced capacity for IFN production in some conditions, in addition to potential defects in downstream
signaling itself. While we have focused on type 1 IFN signaling, some reduced interferon responses are also linked to reduced signaling from the IFN gamma receptor (IFNGR). Consistently, we
also noted reduced IFNγ production in simulated microgravity upon TLR7/8 stimulation. Whether the cytoskeleton is needed for IFNGR clustering and signaling remains to be seen44. Consistent
with reduced interferon signaling in simulated microgravity, we noticed a reduction in some IFN-inducible GTPase superfamily genes, namely guanylate binding proteins (GBPs) across our
datasets. Various GBPs (e.g., _gbp5_) were reduced as well in the Twins study. Interestingly, GBPs, which are heavily induced by IFNγ signaling, have been shown to be critical in maintaining
responses to _mycobacterium tuberculosis_45,46, and we see reactivation of similar bacteria (in addition to some retroviruses) in simulated microgravity after as little as 25 hours of
exposure. GBPs and associated IFN responses also help direct inflammasome activation and pyroptosis (an inflammatory form of cell death) linked to antimicrobial defense that was consistently
down in monocytes and B cells in simulated microgravity, and in nearly all immune cells in response to TLR7/8 stimulation in simulated microgravity46,47. Interestingly, pyroptosis and
inflammasome activation can also be directly controlled by Rho GTPases and the cytoskeleton48,49. Another pathway found consistently down across datasets included LXR signaling.
Interestingly, LXR signaling also can promote antimicrobial defense mechanisms. Macrophage LXR has been shown to reduce bacterial infection by reducing intracellular NAD+ in a CD38 manner,
with mechanistic impacts on the cytoskeleton50. Whether NAD+ levels fall in microgravity remains to be seen, though we did see an interesting increase in sirtuin signaling across datasets,
including in the I4 mission. Sirtuins may be functioning to counter acute oxidative stress in microgravity34,51. We also saw reduced oxidative phosphorylation transcriptional signatures
across all unstimulated immune cells in simulated microgravity. Altered metabolite levels (and possibly ROS) from impaired oxidative phosphorylation might also contribute to HIF1α
stabilization52 as observed in some of our simulated microgravity and spaceflight datasets. Reduced oxidative phosphorylation can be associated with increased glycolysis in immune cells53,
fueling “M1-like” pro-inflammatory changes in macrophages, potentiating NF-κb signaling, acute responses and IL-6 or IL-1 release, cytokines frequently induced in microgravity. Consistently,
we did notice a preferential enrichment of predicted “macrophage classical activation” signatures across our gene sets in the Twins study. Interestingly, we noted frequent increases in
heat-shock genes, coupled to increased associated BAG signaling pathways across antigen-presenting cells (monocytes, B cells, and DCs), as well as in double negative T cells. Heat-shock
expression may be reflective of altered proteostasis in simulated microgravity10, and may be required for adaptation to mechanical unloading in some cells34, though this may also be linked
to higher temperatures. Across all gene sets, we noticed a reduction in the cold shock gene, _rbm3_, which was reduced in nearly all immune cells in our single-cell data. Increased
heat-shock coupled with reduced cold shock genes raises the possibility of higher intracellular temperatures directly induced by microgravity, but whether microgravity, or associated
increase in cytokines or binding partners such as IL-1ra, directly induce the observed “space fever” in astronauts requires further insight54. Interestingly, we did notice a number of
significant IL-1 ligands in innate cell to T cell interactions in our microgravity Interactome, highlighting the possible importance of this cytokine family and downstream interacting
molecules. Pertaining the aforementioned skin lesions in astronauts, it has been postulated that persistent skin hypersensitivity reactions in some crew members may be linked to allergic
responses7,16. While analysis of our datasets cannot rule out this possibility, we did not observe increased Th2 signatures across our simulated microgravity systems, or with our specific
gene sets validated across the I4 mission. We also saw inconsistent changes to IL-23 and IL-17 signaling across our datasets, though these cytokines are known contributors to skin disease55.
While the root causes of such lesions cannot be inferred from our data analysis, it likely involves changes in the crosstalk with the skin microbiome, in addition to intrinsic immune cell
abnormalities. Interestingly, we did see reduced aryl hydrocarbon receptor (AHR) signaling in most of our datasets, especially in CD14 monocytes and conventional type 2 dendritic cells,
raising the idea of reduced AHR signaling in space to contribute to skin lesions56,57. However, we saw AHR signaling was enriched in the Twins study gene set result and so more
experimentation is needed to tease out a possible role for the AHR in astronaut skin lesions. Using in house machine learning algorithms, we identified numerous compounds mapping to
microgravity’s transcriptional response to the immune system. This algorithm focuses on the strength of interaction and does not specify direction. However, we tested one of the most
significantly interacting compounds, the flavonol, quercetin, for its ability to reverse transcriptional changes to simulated microgravity on the immune system, and found that it could
reverse approximately 70% of altered core genes. Of note, quercetin reversed numerous pathways, including core pathways such as reduced nuclear receptor activation, sirtuin signaling,
Coronavirus pathogenesis pathway, associated acute phase responses and IL-6 signaling. Quercetin also showed impact on the cytoskeleton, favoring a freezing of pathways linked to its
mobility in microgravity, by reducing genes associated with Rho GTPase signaling (e.g., reducing RAC, RHOA and CDC42 signaling), and boosting RHOGDI signaling. Despite these changes,
quercetin was unable to revert the core immunosuppression pathway of reduced interferon responses. However, since actin skeleton mobility is needed to induce an IFN response in many
instances40, too much interference could contribute to a persistent lack of IFN signaling here, and might represent a further mechanism of immune suppression mediated by quercetin that
requires more study. Interestingly, after 25 hours in simulated microgravity, we saw variable results on the induction of senescence pathways, though quercetin markedly reduced senescence
associated transcripts in our data. Thus, while quercetin acts in part through its senotherapeutic mechanisms32, the large breadth of additional other pathways suggests multiple beneficial
modes of activity for immune modulation in microgravity. Our data support a hypothetical model where microgravity alters forces sensed by immune cells, leading to changes in the actin
cytoskeleton, and nuclear receptor signaling, coupled to changes in core pathways in space such as mitochondrial dysfunction and oxidative stress. Recent work in other cells, such as
endothelial cells, has identified cytoskeletal abnormalities as a key feature of simulated microgravity that drives autophagy and a reduction in mitochondrial mass after 72 hours of
exposure35. Our datasets would support some of these findings. Combined, these pathways would contribute to reduced oxidative phosphorylation and associated basal inflammatory processes, as
well as reduced viral sensing pathways, associated reduced interferon responses and altered pyroptosis capability. Reduced interferon responses and signaling, impact both innate cells like
monocytes and NK cells, as well as adaptive cells like T cells. Such changes could cumulate in viral or mycobacterial reactivation in microgravity. These processes would also be complemented
by the psychological and physiological stresses of spaceflight, which also may independently associate with viral reactivation12,14,58. Thus, an important future direction of research is to
address whether altered cytoskeleton, or associated reductions in interferon related gene products, including GBPs, are actively driving the reactivation of mycobacteria and latent viruses
that we see in simulated microgravity. Another related important avenue of future research is to better understand how changes to force action on immune cells link to the mitochondria
dysfunction hallmark of spaceflight and metabolic alterations, and to map such changes to immune cell metabolism as has been done to other mechanical forces19,59. Moreover, the immune system
in spaceflight is also under the influence of changing pressure gradients, such as increased pressures in parts of the upper body60, coupled to lower central venous pressure and potentially
altered shear force61,62,63. These changes may also contribute to altered immune function, including the increased IL-1 and IL-6, two cytokines sensitive to external force cues, seen in
spaceflight, with potential for intersecting signaling nodes across mechanotransduction and other pathways such as mitochondrial dysfunction within immune cells19. More work is needed to
understand how these additional changes in force impact innate and adaptive immunity during spaceflight and how they interact with the effects of microgravity. Finally, despite the multiple
proposed mechanisms resulting from this study, much of it was produced using simulated microgravity as a model system, which has its own caveats18, though we did identify core reproduced
pathways from spleens in mice on the ISS and some overlap with the I4 mission and Twins study. Further studies applying single cell-omic technologies to immune cells during in-flight
missions will no doubt provide answers to refine the proposed mechanisms of immune dysfunction in space. As well, integrating more in-depth chromatin and analytical approaches, such as
ATAC-seq, can help detail the regulatory changes that might be underlying these immune phenotypes64. Overall, our current work provides a resource to better understand “astroimmunology”, in
particular how and why the immune system changes in simulated microgravity and spaceflight. These results also provide opportunities to develop countermeasures that will help normalize
immune cell function in microgravity and spaceflight. METHODS Studies were conducted under the supervision and in accordance with ethical guidelines of the Buck Institute for Research on
Aging. In particular, protocols for the purchasing of human blood products from Stanford University are described in the next section below. HUMAN BLOOD SAMPLE AND CELL CULTURE De-identified
peripheral blood buffy coat samples were obtained from the Stanford University Blood Center under official signed contract agreements with the Buck Institute for Research on Aging,
following the Stanford Blood Center’s Certification of Human Subjects Approval for minimal risk research-related activities (IRB eProtocol# 13942). A total of 27 healthy human buffy coats
between the ages of 20 and 46 were purchased from the Stanford University Blood Center. PBMCs were isolated using a Ficoll gradient method. PBMCs were counted and resuspended in complete
media at 1 × 106 cells/ml (RPMI 1640, 10% Fetal Bovine Serum, 2 mM L-glutamine, 1% penicillin/streptomycin, 0.1 mM non-essential Amino acid,1 mM sodium pyruvate, 50 μM 2-mercaptoethanol,10
mM HEPES). To generate simulated microgravity, the cell suspension was loaded into 10 ml disposable high aspect ratio vessels (Synthecon, Houston, TX) and rotated at 15 rpm for 25 hours. For
the 1G control, the cell suspension was plated in standard 6-well culture plates, as standard static culture plates or culture flasks have been shown to be comparable to static high aspect
ratio vessels by others in major immunological assays2. 1G and simulated microgravity cultures were simultaneously placed in the same 37 C, 5% CO2 incubator. To stimulate PBMCs, samples were
mixed with 1 μM R848 (TLR7/8 agonist, Invivogen, San Diego, CA) after 16 hours, for 9 hours of stimulation. At the end of each experiment, the cell suspension was quickly collected, spun
down at 500 × _g_, washed with phosphate-buffered saline, and used for downstream analysis. For super-resolution imaging cells were fixed before centrifuging. SINGLE-CELL RNA SEQUENCING 1 ×
104 PBMCs from each condition were counted and loaded on the 10X Genomics Chromium Controller and the libraries were prepared using Chromium Next GEM Single-Cell 5’ Reagent Kit v2 according
to the manufacturer’s protocol (10X Genomics, Pleasanton, CA). The quality of libraries was assessed using Agilent TapeStation 4200 (Santa Clara, CA), and test-sequenced on Illumina NextSeq
550 (San Diego, CA). The full sequencing was performed on an Illumina NovaSeq 6000 by SeqMatic (Fremont, CA). PROCESSING OF SINGLE-CELL RNA-SEQ DATA Data processing was performed using 10x
Genomics Cell Ranger v6.1.2 and MTD26 pipelines. The “cellranger count” was used to perform transcriptome alignment, filtering, and UMI counting from the FASTQ (raw data) files. Alignment
was done against the human genome GRCh38-2020-A. Cell numbers after processing were: 1G unstimulated 13,304 cells, uG unstimulated 21,709 cells, 1G stimulated 16,397 cells, and uG stimulated
14,913 cells. The MTD pipeline was used to generate the single-cell microbiome count matrix from the FASTQ files. Downstream analyses were performed in R (version 4.2.0), primarily using
the Seurat R package (version 4.1.1)65,66 and custom analysis scripts. First, we executed a quality control step that removed the cells containing >10% mitochondrial RNA and ≤250
genes/features. The doublet cells were identified and removed from the downstream analysis by using the DoubletFinder R package (version 2.0.3)67 with parameters PCs = 1:30, pN = 0.25, and
nExp = 7.5%. To avoid the influence of hemoglobin transcripts on the analysis, we filtered out the putative red blood cells (defined by the method below) before the following process. A
total of 55,648 cells remained for subsequent analysis. Raw RNA counts were first normalized and stabilized with the SCTransform v2 function (SCT), then followed by the CCA integration
workflow for joint analysis of single-cell datasets. In doing so, the top 3000 highly variable genes/features among the datasets were used to run SCT; and then 3000 highly variable
genes/features and the 30 top principal components (PCs) with k.anchor = 5 were used to find “anchors” for integration. The clustering step was executed by using the 30 top PCs summarizing
the RNA expression of each cell with a resolution parameter of 0.8. To identify putative cell types, Azimuth (version 0.3.2)65 pipeline was used with the reference dataset of Human–PBMC
celltype.l2. Cell type annotation results from Azimuth were validated by checking the markers of each cell type (Supplementary Data 3). Gene differential expression analyses were done by
Seurat PrepSCTFindMarkers then FindAllMarkers/FindMarkers functions with MAST68 algorithm. The pseudo-bulk analysis was conducted to find overall DEGs of uG against 1G in either unstimulated
or stimulated PBMCs, using the FindMarker function with parameter min.pct = 0.005 and logFC = 0.1. To compare the stimulated and unstimulated PBMCs under uG and 1G conditions, we subtracted
log2FC values of their DEGs (uG−1G). The top 50 most upregulated DEGs between stimulated and unstimulated PBMCs under 1G were used for comparison. FindConservedMarkers function was used to
find DEGs that are conserved between the groups with the same parameter settings as FinderMarkers. The top 50 conserved DEGs specifically sensitive to uG were selected based on the rank of
the absolute sum of log2FC values, derived separately from the sum of positive log2FC values and the sum of negative log2FC values. Rank-Rank Hypergeometric Overlap (RRHO) analysis69,70 was
performed by using RRHO2 R package (version 1.0) to compare the differential expression patterns between 1G and uG of stimulated vs unstimulated PBMCs. The ranks of the genes in the two gene
lists were determined by calculating −log10(adj._p_value)*log2FC. PATHWAY ANALYSIS Following differential expression, Ingenuity Pathway Analysis (IPA, Qiagen) was used to discover changes
in enriched pathways in each comparison. DEGs with _p_ values < 0.05 and |Log2FC|> 0.1 were incorporated into the IPA canonical pathway analysis. TRAJECTORY ANALYSIS To study the
inferred trajectory of PBMC differentiation, cell trajectory analysis was performed by using the Monocle 3 R package (version 1.2.9)71,72. We first subsetted Seurat data to uG and 1G groups
then run the functions as.cell_data_set(), cluster_cells(), and learn_graph(). Then, we ran order_cells() with the selection of cell types representing early development stages (CD4 naive, B
naive, plasmablast, and HSPC) as the roots of the trajectory. CALCULATING CELL SCORES OF INFLAMMATORY AGING AND CELLULAR SENESCENCE The inflammatory aging (iAge)24 index was calculated by
the sum of the cell scores that count by multiplying normalized and transformed gene expression with the corresponding coefficient of the gene in the iAge gene set. Cellular senescence was
scored using Seurat AddModuleScore function65,73 on the SenMayo gene set25. VIRAL AND MICROBIAL ABUNDANCE ANALYSIS The output reads counts from MTD pipeline were then combined with the host
reads and analyzed in R with Seurat package and other customized scripts. The relative abundance (frequency) of a virus or microbe was determined by dividing its reads count by the total
reads count (host and non-host) in that sample. The classification results were further validated using a different method Magic-BLAST74. APCS TO T CELL INTERCELLULAR COMMUNICATION To study
the difference in intercellular communication from APCs to T cells between uG and 1G, we used nichenetr R packages (version 1.1.0)27 to analyze cells in the dataset belonging to APCs (B
cells, DCs, or monocytes) and T cell types. The “Differential NicheNet” workflow was implemented. The expressed genes in sender cells—APCs were selected if they were expressed in at least
10% of that APC cell population. The gene set of interest in receiver cells—T cells was defined by adjusted _p_ value ≤ 0.05 and Log2FC ≥ 0.25 in the DEGs. Top 30 ligands that were further
used to predict activated target genes and construct an activated ligand-receptor network. Default settings were used for all other parameters. BULK RNA SEQUENCING Total RNA was extracted
using RNeasy Plus Mini Kit (Cat# 74134, Qiagen) as per the manufacturer’s instructions. RNA quantity check, preparation of RNA library, and mRNA sequencing were conducted by Novogene Co.,
LTD (CA, US). About 20 million paired-end 150 bp reads per sample were generated from Illumina NovaSeq 6000 Sequencing System. FASTQ raw reads were analyzed using the MTD pipeline26.
Differential gene expression analysis between groups was done by DESeq2 R package (version 1.36.0)75 with control for the subject effect. Genes with adjusted _p_ value < 0.05 were
considered as differentially expressed. DEGs with _p_ values < 0.05 and |Log2FC|>0.5 were used for the IPA canonical pathway analysis. Different from single-cell (SC), to calculate the
iAge index for bulk RNA-seq, normalized and transformed gene expression was multiplied with the gene’s coefficient in the iAge gene set, then summed for each sample. Cellular senescence was
scored using the ssGSEA76 method on the SenMayo gene set. Cell Type Frequency Changes within PBMCs were predicted by CIBERSORTx Docker image–Fractions Mode version 1.0. Our single-cell
RNA-seq data from PBMCs was used to build the Signature Matrix File as the reference to predict the cell proportion in the bulk RNA-seq data. MOUSE SPLEEN BULK RNA-SEQ ANALYSIS Mouse spleen
Bulk RNA-seq raw data was acquired from NASA GeneLab Data Repository with the accession ID: GLDS-420. Ten mice in space flight and ten mice in ground control (GC) were used in the
experiment. The detailed study description and experiment protocols are on the data repository https://genelab-data.ndc.nasa.gov/genelab/accession/GLDS-420. MTD pipeline was used to process
the FASTQ raw data, generate the count matrix, and then analyze differentially expressed genes between Flight and Ground groups. GENE SET OVERLAPPING ANALYSIS The _p_ value of gene
overlapping between two datasets was calculated by Fisher’s Exact Test in GeneOverlap R package [Shen L, Sinai ISoMaM (2022)]. _GeneOverlap: Test and visualize gene overlaps_. R package
version 1.32.0 [http://shenlab-sinai.github.io/shenlab-sinai/]. The 375 DEGs in uG vs. 1G from unstimulated PBMCs single-cell RNA-seq results were used to match with the genes from PBMC bulk
RNA-seq, I4, or JAXA studies. For the mouse genes in GLDS-420, we first convert them to the human orthologous before the analysis. In the matched genes, those expressions that were in the
same log2FC direction as 375 DEGs as well as with _p_ value < 0.05, were considered overlapping (except for I4, where either direction was considered overlapping). Complete linkage
hierarchical clustering was used to analyze dissimilarities in genes or pathways between datasets, and the results were visualized by the ComplexHeatmap R package (version 2.12.0)77.
Moreover, the IPA canonical pathway analysis was performed on the matched genes of I4 and Twins studies. The 106 core gene set was constituted by DEGs that consistently change their log2FC
directions in both SC and bulk data of PBMCs. The alteration of the core gene set by the compound was measured by Gene Set Enrichment Analysis (GSEA)76,78 and Pearson correlation test.
COMPOUND ANALYSIS FDA-approved drugs (_n_ = 1692) are selected from the DrugBank database and food compounds (_n_ = 7962) are selected from the FoodDB database as previously described32.
LINCS compounds (_n_ = 5414) are obtained from the LINCS L1000 project. ‘Compound’ is used as a general term for ‘drug’, ‘food compound’ and ‘LINCS compound’ throughout the document.
Compound-protein interactions are extracted from the STITCH database v5.079 by matching the InChI keys of drugs/food/LINCS compounds. STITCH collects information from multiple sources and
individual scores from each source are combined into an overall confidence score. After processing, three datasets are obtained: (i) drug-gene interaction dataset containing 1890 drugs and
16,654 genes with 542,577 interactions (ii) food compound-gene interaction dataset containing 7654 compounds and 116,375 genes and 818,737 interactions (iii) LINCS compound-gene interaction
dataset containing 5414 compounds and 16,794 genes and 692,152 interactions. Statistical significance for the overlap between compound genes and the DEGs from the uG vs 1G of the
unstimulated PBMCs single-cell RNA-seq is calculated using Fisher’s Exact Test. The universal gene set contains all genes that interact with at least one compound. The compound with a low
_p_ value interacts with a higher proportion of the DEGs than that expected by chance. Statistically significant compounds were then obtained after the Bonferroni adjustment of _p_ values.
The pipeline for this compound analysis is implemented in the R script GCEA. CELL STAINING AND IMAGING (SUPER-RESOLUTION MICROSCOPY) Live PBMCs were stained with 60 nM MitoTracker
Red-CMX-Ros (ThermoFisher, Waltham, MA) either in 6-well plates or in the microgravity chambers for the last 2 hr of the microgravity simulation. At the end of the microgravity simulation
cells were immediately fixed by 1:1 mixing the cell suspensions with 2× concentrated fixative (10% Sucrose (w/v) 120 mM KCl, 1% (w/v) glutaraldehyde, 8% (w/v) PFA pH 7.4) and incubated for
15 minutes at room temperature followed by 15 minutes on ice. Fixed cells were washed and stored in PBS until further staining for up to a week at 4 °C. 1 million fixed cells were
resuspended in 1 mL of permeabilization solution (0.1% TritonX-100 in PBS) for 5 minutes. After twice washing in PBS, pellets were resuspended in 0.5 mL 1% BSA PBS containing
Phalloidin-iFluor-488 (cat# ab176753, Abcam plc., Cambridge, UK) at the manufacturer’s recommended dilution, and were incubated for 90 minutes with gentle agitation. After washing in PBS,
cells were stained with Hoechst 33342 (1 µg/mL in PBS) for 10 minutes. The fixed-stained cells were immobilized at 3 × 105 cells per well density in glass-bottom 96-well microplates (Greiner
Bio-One, Monroe, NC), which were pre-coated with polyethyleneimine (1:15,000 (w/v)) for 16 hours in a 37 °C incubator, and washed twice with PBS. Microplates with the cell suspensions were
centrifuged in a swing plate rotor centrifuge (Eppendorf 5810 R) at 400 × _g_ and for 10 min and then fixed on the surface by adding an equal volume of 8% (w/v) PFA for 5 min. Finally, the
fixative was replaced with 100 µL of antifade reagent (Vector Prolong Gold (ThermoFisher)). Samples were imaged immediately after this procedure. IMAGE ACQUISITION Immobilized fixed-stained
PBMCs were imaged on a Zeiss LSM980 Airyscan2 laser scanning confocal microscope (Carl Zeiss Microscopy, White Plains, NY). Single PBMCs were manually selected for recording based on
low-resolution preview scans showing only nuclei. All singlet cells were selected in a small neighborhood to avoid biases. In each microscopy session, 24-40 cells were selected for recording
in one well for each condition. This was performed in an interleaved manner, capturing 6-8 cells at a time, and then moving to the next well and then repeating this multiple times using the
Experiment Designer module for automation. Super-resolution volumes of (358 × 358 × 70 pixels, 0.035 × 0.035 × 0.13 µm/voxel resolution) were recorded in the above-determined positions
using Definite Focus autofocusing. A Plan-Apochromat 63 × 1.40 Oil lens, Airyscan2 SR (super-resolution) mode with optimal sampling and frame switching between 3 fluorescence channels to
minimize spectral cross-bleed were used. MitoTracker Red, iFluor488, and Hoechs33342 were excited with 561, 488, and 405 nm solid-state lasers, respectively, using the optimal emission
filter for each channel. 3D Airyscan2 processing was performed with standard filtering settings. With PBMCs from four donors, in six staining and microscopy sessions total of 930 valid
volumes have been recorded, and are available at https://doi.org/10.5281/zenodo.8415196. 2-DIMENSIONAL IMAGE ANALYSES Staining intensities, mitochondrial size, and punctate over diffuse
index (defined as variance over mean) were determined in Image Analyst MKII 4.1.14 (Image Analyst Software, Novato, CA) in maximum intensity projection images using a custom pipeline
available at https://github.com/gerencserlab/Superresolution-actin-and-mitochondria-analysis. Cellpose 2.0 with the “cyto2” neural network was used for finding cells in the images based on
nuclear and actin staining80. Protruding actin bundles were analyzed by first binarizing projection images of actin using the trainable LABKIT segmentation81, and this was followed by
separation of protrusions and measurement of their maximal distance from the bulk of the cell using morphological erosion and distance image functions in Image Analyst MKII. Rescaled
projection images were saved and further analyzed in CellProfiler 4.2.482, where images were segmented for nuclei and these segments were extended to the cell boundaries based on the
phalloidin staining. These profiles were used for measuring shape, granularity spectrum, and texture in actin, mitochondria, and nuclei. For actin granularity spectrum measurement the
following parameters were used in CellProfiler MeasureGranularity function: “Subsampling factor for granularity measurements” = 1, “Subsampling factor for background reduction = 0.125”,
“Radius of structuring element” = 12, “Range of granular spectrum” = 16. Similar results were obtained using a set of discrete Fourier transformation-based Butterworth bandpass filters in
Image Analyst MKII for analysis of actin granularity spectrum changes in simulated microgravity and TLR stimulation. Here a series of 16 adjacent 4-pixels wide (in Fourier space of a 512 ×
512 pixels image), 300-order bandpass filters with “Corrected Integral” normalization and absolute value calculation83 were used starting at 1 pixel, and mean pixel intensities over whole
cells in the filtered images were normalized to the unfiltered image. We have previously shown that this technique is primarily sensitive to sub-resolution changes in thickness of underlying
filamentous structures84, such as actin bundles in this case. We found no changes in granularity spectra measured by CellProfiler or Image Analyst MKII when analyzing mitochondria or nuclei
of the same cells, excluding optical biases. 3-DIMENSIONAL IMAGE ANALYSES Mitochondria:cell volume fraction was measured using a modification of the “Mitochondria:cell volume fractionator
(basic)” pipeline in Image Analyst MKII85, using the hole-filled actin image as cell marker and MitoTracker Red as mitochondrial marker, and all image planes to measure areas of
mitochondrial and cell profiles. Cell and nucleus volumes and surface areas were measured using Imaris 9.9 (Oxford Instruments, Concord, MA) using the Cell and Batch modules. For 2D and 3D
image analyses, tabular data generated by Image Analyst MKII, CellProfiler, and Imaris were matched to conditions in Microsoft Excel and in Mathematica 13 (Wolfram Research, Champaign, IL)
and visualized in Prism 9 (GraphPad, La Jolla, CA) for statistical analysis. Two-tailed Welch’s _t_ test is used for all comparisons. CDC42, RAC1, AND RHOA G-LISA ACTIVATION ASSAY PBMCs from
different conditions were collected and 7 × 106 cells were lysed and snap-frozen immediately in liquid nitrogen. Cell lysate protein concentrations were measured using Precision Red
Advanced Protein Assay Reagent (cat# ADV02, Cytoskeleton Inc., Denver, CO) and equalized. The GTP-bound Cdc42, Rac1, and RhoA levels were performed according to the manufacturer’s protocol
(cat# BK127-S, BK128-S, and BK124-S respectively, Cytoskeleton Inc.) and measured with a spectrophotometer at 490 nm. ROS DETECTION The abundance of ROS was measured via
2’,7’-dichlorodihydrofluorescein diacetate (DCFDA). Collected cells (100,000 cells per well) from each condition were incubated with 10 μM DCFDA Staining Buffer in dark at 37 °C for 30
minutes as per the manufacturer’s suggestions (cat# 601520; Cayman Chemical, Ann Arbor, MI). The fluorescence was measured with a Pherastar FSx (BMG Labtech Inc., Cary, NC) microplate reader
with the excitation wavelength at 495 nm, and emission at 530 nm. LUMINEX BEAD ARRAY Cell culture media (supernatant) from all experimental conditions were separately collected and
snap-frozen. Samples were sent to the Stanford Human Immune Monitoring Center and MILLIPLEX 48 Plex Premixed Magnetic Bead Panel (MilliporeSigma, Burlington, MA) was performed per the
manufacturers’ instructions. IFN ELISA LEVEL MEASUREMENT Cell culture media (supernatant) from microgravity and 1G ± R848 were separately collected at each experiment and snap-frozen. The
samples were then thawed and used to detect the levels of IFNγ (cat# 430104; Biolegend Inc., San Diego, CA), IFNα all subtypes (cat# 41135; Pestka Biomedical Laboratories, Inc., Piscataway,
NJ) per the manufacturers’ instructions. IL ELISA LEVEL MEASUREMENT Cell culture media (supernatant) from microgravity and 1G were separately collected at each experiment and snap-frozen.
The samples were then thawed and used to detect the levels of IL-8 (cat# 431504; Biolegend Inc., San Diego, CA), IL-6 (cat# 430504; Biolegend Inc., San Diego, CA), IL-1β (cat# 437004;
Biolegend Inc., San Diego, CA) per the manufacturers’ instructions. FLOW CYTOMETRY Single-cell suspensions from different donors and conditions were stained with LIVE/DEAD Fixable Blue Dead
Cell Stain kit (cat# L34962; Invitrogen) for viability followed by Fc-blocking with human IgG (cat# AG714, Sigma-Aldrich) at room temperature for 10 mins. For staining of intracellular
cytokines, single-cell suspension was stimulated with 1 μM R848 in the presence of 2.5ug/ml Brefeldin A (cat# 420601; Biolegend) for 9 hours prior to surface staining. The cells were further
stained with fluorophore-conjugated surface antibodies for 20 min at 4 °C and intracellular antibodies for 30 min at room temperature following fixation and permeabilization using Foxp3
staining buffer set (cat# 00-5523-00; eBioscience). The surface and intracellular antibody panel are listed in Supplementary Data 19. Cell phenotyping was analyzed on a Cytek Aurora
instrument and analyzed using FlowJo. JAXA TRANSCRIPTOMIC DATA JAXA cell-free RNA differential expression data was shared by Dr. Masafumi Muratani at the University of Tsukuba. Briefly,
blood samples were collected from six astronauts before, during, and after the spaceflight on the ISS. For this mission, we specifically made use of data from the samples of six astronauts,
pooled into a single count, at day 5 and also at day 30, post-launch (i.e., in-flight), compared to pre-launch. In this study, human blood from astronauts was collected using Vacutainer
EDTA-plasma separate gel collection tubes and centrifuged for 30 min at 3800 rpm (1239 × _g_, ISS) or 1600 × _g_ (ground) before freezing at −95 °C (ISS) or −80 °C (ground). Cell-free RNA
was purified from plasma samples through a TRIzol/chloroform method, sequenced (SMART-seq Stranded Kit, Takara Bio), and analyzed by the team leading the JAXA collaboration. Data was
provided in csv format as normalized mean counts and normalized SEM of each gene at preflight and inflight time points. DEGs between 30 days in-flight and preflight time points were
calculated by log2FC with _p_ value < 0.05. The overlapping DEGs that are consistent in their log2FC directions with SC 375 gene signature are shown in Supplementary Fig. 16B.
INSPIRATION4 MISSION DATA Four astronauts’ transcriptomic data from the Inspiration4 (I4) mission was collected by Dr. Christopher E. Mason and his team at Cornell University. Blood samples
were collected before (preflight L-92, L-44, and L-3), during, and after (R + 1) the 3-day spaceflight in the SpaceX Dragon capsule. For this mission, we made use of data provided to us from
the samples of the four astronauts comparing post-flight (R + 1) vs preflight (L-44) DEGs. Referring to the analysis workflow used by the I4 Cornell team, a list of fold change and _p_
values based on post flight vs preflight findings was generated. The Seurat FindMarker parameters used to calculate I4 DEGs were the same as those used for our 375 DEGs. Next, the DEGs and
pathway overlap from I4 single-cell analysis were calculated by using the methods described above in the section on Gene set overlapping analysis. NASA TWINS STUDY DATA Gene expression data
from the NASA Twins study17 was provided by Cem Meydan in the Mason Lab in csv format and organized by Dr. Afshin Beheshti as normalized mean counts for four immune cell types. In brief, one
astronaut was monitored before, during, and after a 1-year mission onboard the ISS, and his identical twin sibling was also monitored at the same time serving as a genetically matched
ground control for this study. The NASA Twins study team provided DEGs in their four immune cell types with a list of fold change and _p_ values based on inflight vs preflight findings. We
reclustered our identified cell populations and computed DEGs accordingly. Next, the DEGs and pathway overlap from the Twins analysis were calculated by using the methods described above in
the section on Gene set overlapping analysis. ROS REDUCTION COMPOUND Quercetin (Sigma-Aldrich, St Louis, MO) stock solution was prepared with DMSO at 1000×. In the cell culture experiments
utilizing quercetin, the concentration of quercetin was decided based on existing literature86,87,88,89. After incubation with quercetin, cells were counted with a Cellometer Auto 2000 Cell
Viability Counter (Nexcelom, San Diego, CA), which utilizes Acridine Orange and Propidium iodide dual-staining systems to accurately distinguish live vs dead cells. After 25 hours of 50 μM
quercetin treatment, the cell viability across PBMCs in both 1G and simulated microgravity conditions were at least 93%. There were no statistical differences in viability observed between
the groups with and without quercetin treatment. STATISTICAL ANALYSES In addition to the methods described above, the Wilcoxon Rank Sum Test was used to assess whether the distributions of
data from cell score or microbial abundance were significantly different between the 1G and uG cell populations from single-cell data. The association between single-cell and bulk RNA-seq in
gene expressions was tested by Spearman’s correlation. Mann–Whitney test was performed on ROS reduction by quercetin. Unpaired parametric two-tailed _t_ tests were performed on single-cell
iAge, SenMayo, and imaging analyses for statistics. G-LISA, ELISA, Luminex, and DCFDA results were assessed by paired parametric two-tailed _t_ test. However, given our existing
transcriptomic and cytokine data showed decreased interferon coupled to increased IL-1β, IL-6, and IL-8 production in microgravity, for validation the flow cytometry results were assessed by
one-tail paired parametric _t_ test. In PBMCs bulk RNA-seq results, the difference in iAge and SenMayo scores of samples with or without compound treatment was evaluated by two-tailed
paired parametric _t_ test. R (version 4.2.0) and GraphPad Prism 9 were used to conduct the statistical analyses. Significance was set at 0.05. Outliers in datasets were assessed using
Grubbs’ test (alpha = 0.01) and specified in figure legends if any were removed. REPORTING SUMMARY Further information on research design is available in the Nature Portfolio Reporting
Summary linked to this article. DATA AVAILABILITY The raw single-cell and bulk RNA-seq data generated in this study are deposited in the Gene Expression Omnibus (GEO) database under
accession code GSE218937. Mouse spleen Bulk RNA-seq raw data was acquired from NASA GeneLab Data Repository with the accession ID: GLDS-420. All other data are available in the article and
its Supplementary files or from the corresponding author upon request. Source data including cell proportion, gene set score, GLISA, ELISA, Luminex, and flow results are provided as a Source
Data file. Source data are provided with this paper. CODE AVAILABILITY The code used for the analysis of sequencing data is available at GitHub and Zenodo repository
[https://github.com/FEI38750/Immune_Dysfunction_in_Microgravity; https://zenodo.org/record/8247816]90. Code for GCEA is available upon request from the corresponding author. REFERENCES *
Martinez, E. M., Yoshida, M. C., Candelario, T. L. T. & Hughes-Fulford, M. Spaceflight and simulated microgravity cause a significant reduction of key gene expression in early T-cell
activation. _Am. J. Physiol. Regul. Integr. Comp. Physiol._ 308, R480–R488 (2015). Article CAS PubMed PubMed Central Google Scholar * Tackett, N. et al. Prolonged exposure to simulated
microgravity diminishes dendritic cell immunogenicity. _Sci. Rep._ 9, 13825 (2019). Article ADS PubMed PubMed Central Google Scholar * Crucian, B. et al. Immune system dysregulation
occurs during short duration spaceflight on board the space shuttle. _J. Clin. Immunol._ 33, 456–465 (2013). Article CAS PubMed Google Scholar * Paul, A. M. et al. Beyond low-earth
orbit: characterizing immune and microrna differentials following simulated deep spaceflight conditions in mice. _iScience_ 23, 101747 (2020). Article ADS CAS PubMed PubMed Central
Google Scholar * Guéguinou, N. et al. Could spaceflight-associated immune system weakening preclude the expansion of human presence beyond Earth’s orbit? _J. Leukoc. Biol._ 86, 1027–1038
(2009). Article PubMed Google Scholar * Makedonas, G. et al. Specific immunologic countermeasure protocol for deep-space exploration missions. _Front. Immunol._ 10, 2407 (2019). Article
PubMed PubMed Central Google Scholar * Crucian, B. E. et al. Immune system dysregulation during spaceflight: potential countermeasures for deep space exploration missions. _Front.
Immunol._ 9, 1437 (2018). Article PubMed PubMed Central Google Scholar * Chang, T. T. et al. The Rel/NF-κB pathway and transcription of immediate early genes in T cell activation are
inhibited by microgravity. _J. Leukoc. Biol._ 92, 1133–1145 (2012). Article CAS PubMed PubMed Central Google Scholar * Hughes-Fulford, M., Chang, T. T., Martinez, E. M. & Li, C.-F.
Spaceflight alters expression of microRNA during T-cell activation. _FASEB J._ 29, 4893–4900 (2015). Article CAS PubMed PubMed Central Google Scholar * da Silveira, W. A. et al.
Comprehensive multi-omics analysis reveals mitochondrial stress as a central biological hub for spaceflight impact. _Cell_ 183, 1185–1201.e20 (2020). Article PubMed PubMed Central Google
Scholar * Bigley, A. B. et al. NK cell function is impaired during long-duration spaceflight. _J. Appl. Physiol._ 126, 842–853 (2019). Article CAS PubMed Google Scholar * Rooney, B. V.,
Crucian, B. E., Pierson, D. L., Laudenslager, M. L. & Mehta, S. K. Herpes virus reactivation in astronauts during spaceflight and its application on earth. _Front. Microbiol._ 10, 16
(2019). Article PubMed PubMed Central Google Scholar * Crucian, B. et al. Alterations in adaptive immunity persist during long-duration spaceflight. _NPJ Microgravity_ 1, 15013 (2015).
Article PubMed PubMed Central Google Scholar * Mehta, S. K. et al. Latent virus reactivation in astronauts on the international space station. _NPJ Microgravity_ 3, 11 (2017). Article
PubMed PubMed Central Google Scholar * Mehta, S. K. et al. Localization of VZV in saliva of zoster patients. _J. Med. Virol._ 89, 1686–1689 (2017). Article CAS PubMed Google Scholar *
Mehta, S. K. et al. Reactivation of latent viruses is associated with increased plasma cytokines in astronauts. _Cytokine_ 61, 205–209 (2013). Article CAS PubMed Google Scholar *
Garrett-Bakelman, F. E. et al. The NASA twins study: a multidimensional analysis of a year-long human spaceflight. _Science_ 364, eaau8650 (2019). Article ADS CAS PubMed PubMed Central
Google Scholar * Spatz, J. M. et al. Human immune system adaptations to simulated microgravity revealed by single-cell mass cytometry. _Sci. Rep._ 11, 11872 (2021). Article ADS CAS
PubMed PubMed Central Google Scholar * Du, H. et al. Tuning immunity through tissue mechanotransduction. _Nat. Rev. Immunol._ 23, 174–188 (2023). Article CAS PubMed Google Scholar *
Freed, L. E. & Vunjak-Novakovic, G. Spaceflight bioreactor studies of cells and tissues. _Adv. Space Biol. Med._ 8, 177–195 (2002). Article CAS PubMed Google Scholar * Nelson, E. S.,
Mulugeta, L. & Myers, J. G. Microgravity-induced fluid shift and ophthalmic changes. _Life (Basel)_ 4, 621–665 (2014). ADS PubMed Google Scholar * Barrila, J. et al. Spaceflight
analogue culture enhances the host-pathogen interaction between salmonella and a 3-D biomimetic intestinal co-culture model. _Front. Cell. Infect. Microbiol._ 12, 705647 (2022). Article CAS
PubMed PubMed Central Google Scholar * Caron, G. et al. Direct stimulation of human T cells via TLR5 and TLR7/8: flagellin and R-848 up-regulate proliferation and IFN-gamma production
by memory CD4+ T cells. _J. Immunol._ 175, 1551–1557 (2005). Article CAS PubMed Google Scholar * Sayed, N. et al. An inflammatory aging clock (iAge) based on deep learning tracks
multimorbidity, immunosenescence, frailty and cardiovascular aging. _Nat. Aging_ 1, 598–615 (2021). Article PubMed PubMed Central Google Scholar * Saul, D. et al. A new gene set
identifies senescent cells and predicts senescence-associated pathways across tissues. _Nat. Commun._ 13, 4827 (2022). Article ADS CAS PubMed PubMed Central Google Scholar * Wu, F.,
Liu, Y.-Z. & Ling, B. MTD: a unique pipeline for host and meta-transcriptome joint and integrative analyses of RNA-seq data. _Brief. Bioinformatics_ 23, bbac111 (2022). Article PubMed
Google Scholar * Browaeys, R., Saelens, W. & Saeys, Y. NicheNet: modeling intercellular communication by linking ligands to target genes. _Nat. Methods_ 17, 159–162 (2020). Article CAS
PubMed Google Scholar * Newman, A. M. et al. Determining cell type abundance and expression from bulk tissues with digital cytometry. _Nat. Biotechnol._ 37, 773–782 (2019). Article CAS
PubMed PubMed Central Google Scholar * Vorperian, S. K. & Moufarrej, M. N. Tabula Sapiens Consortium & Quake, S. R. Cell types of origin of the cell-free transcriptome. _Nat.
Biotechnol._ 40, 855–861 (2022). Article CAS PubMed PubMed Central Google Scholar * Nataraj, N. B. et al. Nucleoporin-93 reveals a common feature of aggressive breast cancers: robust
nucleocytoplasmic transport of transcription factors. _Cell Rep._ 38, 110418 (2022). Article CAS PubMed PubMed Central Google Scholar * El Masri, R. & Delon, J. RHO GTPases: from
new partners to complex immune syndromes. _Nat. Rev. Immunol._ 21, 499–513 (2021). Article CAS PubMed Google Scholar * Veselkov, K. et al. HyperFoods: Machine intelligent mapping of
cancer-beating molecules in foods. _Sci. Rep._ 9, 9237 (2019). Article ADS PubMed PubMed Central Google Scholar * Zhu, Y. et al. The Achilles’ heel of senescent cells: from
transcriptome to senolytic drugs. _Aging Cell_ 14, 644–658 (2015). Article CAS PubMed PubMed Central Google Scholar * Cazzaniga, A., Locatelli, L., Castiglioni, S. & Maier, J. A. M.
The dynamic adaptation of primary human endothelial cells to simulated microgravity. _FASEB J._ 33, 5957–5966 (2019). Article CAS PubMed Google Scholar * Locatelli, L., Cazzaniga, A.,
De Palma, C., Castiglioni, S. & Maier, J. A. M. Mitophagy contributes to endothelial adaptation to simulated microgravity. _FASEB J._ 34, 1833–1845 (2020). Article CAS PubMed Google
Scholar * Crucian, B. et al. Incidence of clinical symptoms during long-duration orbital spaceflight. _Int. J. Gen. Med._ 9, 383–391 (2016). Article PubMed PubMed Central Google Scholar
* Guignandon, A. et al. Rac1 GTPase silencing counteracts microgravity-induced effects on osteoblastic cells. _FASEB J._ 28, 4077–4087 (2014). Article CAS PubMed PubMed Central Google
Scholar * Liu, S. et al. RhoB induces the production of proinflammatory cytokines in TLR-triggered macrophages. _Mol. Immunol._ 87, 200–206 (2017). Article CAS PubMed Google Scholar *
Barrio, L., Saez de Guinoa, J. & Carrasco, Y. R. TLR4 signaling shapes B cell dynamics via MyD88-dependent pathways and Rac GTPases. _J. Immunol._ 191, 3867–3875 (2013). Article CAS
PubMed Google Scholar * Trono, P., Tocci, A., Musella, M., Sistigu, A. & Nisticò, P. Actin cytoskeleton dynamics and type I IFN-mediated immune response: a dangerous liaison in cancer?
_Biology (Basel)_ 10, 913 (2021). CAS PubMed Google Scholar * Irving, A. T. et al. Regulation of actin dynamics by protein kinase R control of gelsolin enforces basal innate immune
defense. _Immunity_ 36, 795–806 (2012). Article CAS PubMed Google Scholar * Ohman, T., Rintahaka, J., Kalkkinen, N., Matikainen, S. & Nyman, T. A. Actin and RIG-I/MAVS signaling
components translocate to mitochondria upon influenza A virus infection of human primary macrophages. _J. Immunol._ 182, 5682–5692 (2009). Article PubMed Google Scholar * Mukherjee, A. et
al. Retinoic acid-induced gene-1 (RIG-I) associates with the actin cytoskeleton via caspase activation and recruitment domain-dependent interactions. _J. Biol. Chem._ 284, 6486–6494 (2009).
Article CAS PubMed PubMed Central Google Scholar * Blouin, C. M. & Lamaze, C. Interferon gamma receptor: the beginning of the journey. _Front. Immunol._ 4, 267 (2013). Article
PubMed PubMed Central Google Scholar * Kim, B.-H., Shenoy, A. R., Kumar, P., Bradfield, C. J. & MacMicking, J. D. IFN-inducible GTPases in host cell defense. _Cell Host Microbe_ 12,
432–444 (2012). Article CAS PubMed PubMed Central Google Scholar * Tretina, K., Park, E.-S., Maminska, A. & MacMicking, J. D. Interferon-induced guanylate-binding proteins:
guardians of host defense in health and disease. _J. Exp. Med._ 216, 482–500 (2019). Article CAS PubMed PubMed Central Google Scholar * Fisch, D. et al. Human GBP1 is a microbe-specific
gatekeeper of macrophage apoptosis and pyroptosis. _EMBO J._ 38, e100926 (2019). Article PubMed PubMed Central Google Scholar * Xu, H. et al. Innate immune sensing of bacterial
modifications of Rho GTPases by the Pyrin inflammasome. _Nature_ 513, 237–241 (2014). Article ADS CAS PubMed Google Scholar * Dufies, O. et al. Escherichia coli Rho GTPase-activating
toxin CNF1 mediates NLRP3 inflammasome activation via p21-activated kinases-1/2 during bacteraemia in mice. _Nat. Microbiol._ 6, 401–412 (2021). Article CAS PubMed PubMed Central Google
Scholar * Matalonga, J. et al. The nuclear receptor LXR limits bacterial infection of host macrophages through a mechanism that impacts cellular NAD metabolism. _Cell Rep._ 18, 1241–1255
(2017). Article CAS PubMed Google Scholar * Singh, C. K. et al. The role of sirtuins in antioxidant and redox signaling. _Antioxid. Redox Signal._ 28, 643–661 (2018). Article CAS
PubMed PubMed Central Google Scholar * Hayashi, Y., Yokota, A., Harada, H. & Huang, G. Hypoxia/pseudohypoxia-mediated activation of hypoxia-inducible factor-1α in cancer. _Cancer
Sci._ 110, 1510–1517 (2019). Article CAS PubMed PubMed Central Google Scholar * Van den Bossche, J., O’Neill, L. A. & Menon, D. Macrophage immunometabolism: where are we (going)?
_Trends Immunol._ 38, 395–406 (2017). Article PubMed Google Scholar * Stahn, A. C. et al. Increased core body temperature in astronauts during long-duration space missions. _Sci. Rep._ 7,
16180 (2017). Article ADS PubMed PubMed Central Google Scholar * Liu, T. et al. The IL-23/IL-17 pathway in inflammatory skin diseases: from bench to bedside. _Front. Immunol._ 11,
594735 (2020). Article CAS PubMed PubMed Central Google Scholar * Peng, G. et al. Human β-defensin-3 attenuates atopic dermatitis-like inflammation through autophagy activation and the
aryl hydrocarbon receptor signaling pathway. _J. Clin. Invest._ 132, e156501 (2022). Article CAS PubMed PubMed Central Google Scholar * Kim, H.-B. et al. Aryl hydrocarbon receptors:
evidence of therapeutic targets in chronic inflammatory skin diseases. _Biomedicines_ 10, 1087 (2022). Article CAS PubMed PubMed Central Google Scholar * Crucian, B. E. et al.
Countermeasures-based improvements in stress, immune system dysregulation and latent herpesvirus reactivation onboard the international space station - relevance for deep space missions and
terrestrial medicine. _Neurosci. Biobehav. Rev._ 115, 68–76 (2020). Article CAS PubMed Google Scholar * Chakraborty, M. et al. Mechanical stiffness controls dendritic cell metabolism and
function. _Cell Rep._ 34, 108609 (2021). Article CAS PubMed Google Scholar * Tanaka, K., Nishimura, N. & Kawai, Y. Adaptation to microgravity, deconditioning, and countermeasures.
_J. Physiol. Sci._ 67, 271–281 (2017). Article PubMed Google Scholar * White, R. J. & Blomqvist, C. G. Central venous pressure and cardiac function during spaceflight. _J. Appl.
Physiol._ 85, 738–746 (1998). Article CAS PubMed Google Scholar * Buckey, J. C. et al. Central venous pressure in space. _J. Appl. Physiol._ 81, 19–25 (1996). Article PubMed Google
Scholar * Dixon, J. B. & Clark, T. K. Sensorimotor impairment from a new analog of spaceflight-altered neurovestibular cues. _J. Neurophysiol._ 123, 209–223 (2020). Article PubMed
Google Scholar * Gertz, M. L. et al. Multi-omic, single-cell, and biochemical profiles of astronauts guide pharmacological strategies for returning to gravity. _Cell Rep._ 33, 108429
(2020). Article CAS PubMed PubMed Central Google Scholar * Hao, Y. et al. Integrated analysis of multimodal single-cell data. _Cell_ 184, 3573–3587.e29 (2021). Article CAS PubMed
PubMed Central Google Scholar * Satija, R., Farrell, J. A., Gennert, D., Schier, A. F. & Regev, A. Spatial reconstruction of single-cell gene expression data. _Nat. Biotechnol._ 33,
495–502 (2015). Article CAS PubMed PubMed Central Google Scholar * McGinnis, C. S., Murrow, L. M. & Gartner, Z. J. DoubletFinder: doublet detection in single-cell RNA sequencing
data using artificial nearest neighbors. _Cell Syst._ 8, 329–337.e4 (2019). Article CAS PubMed PubMed Central Google Scholar * Finak, G. et al. MAST: a flexible statistical framework
for assessing transcriptional changes and characterizing heterogeneity in single-cell RNA sequencing data. _Genome Biol._ 16, 278 (2015). Article PubMed PubMed Central Google Scholar *
Cahill, K. M., Huo, Z., Tseng, G. C., Logan, R. W. & Seney, M. L. Improved identification of concordant and discordant gene expression signatures using an updated rank-rank
hypergeometric overlap approach. _Sci. Rep._ 8, 9588 (2018). Article ADS PubMed PubMed Central Google Scholar * Plaisier, S. B., Taschereau, R., Wong, J. A. & Graeber, T. G.
Rank-rank hypergeometric overlap: identification of statistically significant overlap between gene-expression signatures. _Nucleic Acids Res._ 38, e169 (2010). Article PubMed PubMed
Central Google Scholar * Qiu, X. et al. Single-cell mRNA quantification and differential analysis with Census. _Nat. Methods_ 14, 309–315 (2017). Article CAS PubMed PubMed Central
Google Scholar * Trapnell, C. et al. The dynamics and regulators of cell fate decisions are revealed by pseudotemporal ordering of single cells. _Nat. Biotechnol._ 32, 381–386 (2014).
Article CAS PubMed PubMed Central Google Scholar * Tirosh, I. et al. Dissecting the multicellular ecosystem of metastatic melanoma by single-cell RNA-seq. _Science_ 352, 189–196 (2016).
Article ADS CAS PubMed PubMed Central Google Scholar * Boratyn, G. M., Thierry-Mieg, J., Thierry-Mieg, D., Busby, B. & Madden, T. L. Magic-BLAST, an accurate RNA-seq aligner for
long and short reads. _BMC Bioinformatics_ 20, 405 (2019). Article PubMed PubMed Central Google Scholar * Love, M. I., Huber, W. & Anders, S. Moderated estimation of fold change and
dispersion for RNA-seq data with DESeq2. _Genome Biol._ 15, 550 (2014). Article PubMed PubMed Central Google Scholar * Barbie, D. A. et al. Systematic RNA interference reveals that
oncogenic KRAS-driven cancers require TBK1. _Nature_ 462, 108–112 (2009). Article ADS CAS PubMed PubMed Central Google Scholar * Gu, Z., Eils, R. & Schlesner, M. Complex heatmaps
reveal patterns and correlations in multidimensional genomic data. _Bioinformatics_ 32, 2847–2849 (2016). Article CAS PubMed Google Scholar * Subramanian, A. et al. Gene set enrichment
analysis: a knowledge-based approach for interpreting genome-wide expression profiles. _Proc. Natl Acad. Sci. USA_ 102, 15545–15550 (2005). Article ADS CAS PubMed PubMed Central Google
Scholar * Szklarczyk, D. et al. STITCH 5: augmenting protein-chemical interaction networks with tissue and affinity data. _Nucleic Acids Res._ 44, D380–D384 (2016). Article CAS PubMed
Google Scholar * Stringer, C., Wang, T., Michaelos, M. & Pachitariu, M. Cellpose: a generalist algorithm for cellular segmentation. _Nat. Methods_ 18, 100–106 (2021). Article CAS
PubMed Google Scholar * Arzt, M. et al. LABKIT: labeling and segmentation toolkit for big image data. _Front. Comput. Sci_. 4, https://www.biorxiv.org/content/10.1101/2021.10.14.464362v1
(2022). * Stirling, D. R. et al. CellProfiler 4: improvements in speed, utility and usability. _BMC Bioinformatics_ 22, 433 (2021). Article PubMed PubMed Central Google Scholar *
Gerencsér AA, A. A. & Adam-Vizi, V. Selective, high-resolution fluorescence imaging of mitochondrial Ca2+ concentration. _Cell Calcium_ 30, 311–321 (2001). Article PubMed Google
Scholar * Gerencser, A. A., Doczi, J., Töröcsik, B., Bossy-Wetzel, E. & Adam-Vizi, V. Mitochondrial swelling measurement in situ by optimized spatial filtering: astrocyte-neuron
differences. _Biophys. J._ 95, 2583–2598 (2008). Article ADS CAS PubMed PubMed Central Google Scholar * Gerencser, A. A. et al. Quantitative measurement of mitochondrial membrane
potential in cultured cells: calcium-induced de- and hyperpolarization of neuronal mitochondria. _J. Physiol. (Lond.)_ 590, 2845–2871 (2012). Article CAS PubMed Google Scholar * Li, D.
et al. Quercetin alleviates ferroptosis of pancreatic β cells in type 2 diabetes. _Nutrients_ 12, 2954 (2020). Article CAS PubMed PubMed Central Google Scholar * Ortega, R. &
García, N. The flavonoid quercetin induces changes in mitochondrial permeability by inhibiting adenine nucleotide translocase. _J. Bioenerg. Biomembr._ 41, 41–47 (2009). Article CAS PubMed
Google Scholar * Jiang, J.-J., Zhang, G.-F., Zheng, J.-Y., Sun, J.-H. & Ding, S.-B. Targeting mitochondrial ROS-mediated ferroptosis by quercetin alleviates high-fat diet-induced
hepatic lipotoxicity. _Front. Pharmacol._ 13, 876550 (2022). Article CAS PubMed PubMed Central Google Scholar * Bouamama, S. & Bouamama, A. Quercetin handles cellular
oxidant/antioxidant systems and mitigates immunosenescence hallmarks in human PBMCs: an in vitro study. _J. Biochem. Mol. Toxicol_. 37, e23354 (2023). * Wu, F.
FEI38750/Immune_Dysfunction_in_Microgravity: Immune_Dysfunction_in_Microgravity. _Zenodo_ https://doi.org/10.5281/zenodo.8247816 (2023). Download references ACKNOWLEDGEMENTS We would like to
thank the crew members of I4, JAXA, and Twins study for contributing valuable samples to improve our understanding of the immune system in space. We would like to thank Dr. Cheryl Nickerson
(Arizona State University) for technical discussions on simulated microgravity ground analogs, and Dr. Brian Crucian (NASA) for discussions on translational aspects of the immune system in
space. We would like to thank Dr. Christopher Chin (Cornell University), Dr. Remi V. Klotz (University of Maryland) and Dr. Min Yu (University of Maryland) for assistance with I4 single cell
preparation and analysis. We would like to thank Ryan Kwok and Ritesh Tiwari for technical assistance with flow cytometry. This work was supported in part through funds derived from the
Buck Institute for Research on Aging (D.A.W., D.F.), and the Huiying Memorial Foundation (D.A.W.). T.R.V. and J.B. are funded by a T32 NIH fellowship grant (NIA T32 AG000266). C.E.M. thanks
the Scientific Computing Unit (SCU) at WCM, the WorldQuant Foundation, NASA (NNX14AH50G, NNX17AB26G, 80NSSC22K0254, NNH18ZTT001N-FG2, 80NSSC22K0254, NNX16AO69A), the National Institutes of
Health (R01MH117406), and LLS (MCL7001-18, LLS 9238-16). Figure 7A was created with BioRender.com. AUTHOR INFORMATION Author notes * These authors contributed equally: Fei Wu, Huixun Du. *
These authors jointly supervised this work: Christopher E. Mason, David Furman, Daniel A. Winer. AUTHORS AND AFFILIATIONS * Buck Institute for Research on Aging, Novato, CA, 94945, USA Fei
Wu, Huixun Du, Priya Makhijani, Nicolas Martin, Chad A. Lerner, Khiem Nguyen, Jordan Baechle, Taylor R. Valentino, Matias Fuentealba, Juliet M. Bartleson, Heather Halaweh, Simon Melov, Akos
A. Gerencser, Herbert G. Kasler, David Furman & Daniel A. Winer * Leonard Davis School of Gerontology, University of Southern California, Los Angeles, CA, 90089, USA Huixun Du &
Daniel A. Winer * Department of Physiology and Biophysics, Weill Cornell Medicine, New York, NY, 10021, USA Eliah Overbey, JangKeun Kim, Cem Meydan & Christopher E. Mason * Department of
Immunology, University of Toronto, Toronto, ON, M5S 1A8, Canada Priya Makhijani & Daniel A. Winer * Department of Laboratory Medicine and Pathobiology, University of Toronto, Toronto,
ON, M5S 1A8, Canada Shawn Winer & Daniel A. Winer * Pathology and Laboratory Medicine, Mount Sinai Hospital, Toronto, ON, Canada Shawn Winer * Department of Medicine, University of
Virginia, Charlottesville, VA, USA Francine Garrett-Bakelman * Department of Biochemistry and Molecular Genetics, University of Virginia, Charlottesville, VA, USA Francine Garrett-Bakelman *
Stanford Cardiovascular Institute, Stanford University School of Medicine, Stanford, CA, 94305, USA Nazish Sayed * Transborder Medical Research Center, University of Tsukuba, Ibaraki,
305-8575, Japan Masafumi Muratani * Department of Genome Biology, Faculty of Medicine, University of Tsukuba, Ibaraki, 305-8575, Japan Masafumi Muratani * Blue Marble Space Institute of
Science, Space Biosciences Division, NASA Ames Research Center, Moffett Field, CA, 94043, USA Afshin Beheshti * Stanley Center for Psychiatric Research, Broad Institute of MIT and Harvard,
Cambridge, MA, 02142, USA Afshin Beheshti * The HRH Prince Alwaleed Bin Talal Bin Abdulaziz Alsaud Institute for Computational Biomedicine, Weill Cornell Medicine, New York, NY, 10021, USA
Christopher E. Mason * WorldQuant Initiative for Quantitative Prediction, Weill Cornell Medicine, New York, NY, 10021, USA Christopher E. Mason * The Feil Family Brain and Mind Research
Institute, Weill Cornell Medicine, New York, NY, 10021, USA Christopher E. Mason * Stanford 1000 Immunomes Project, Stanford University School of Medicine, Stanford, CA, USA David Furman *
Institute for Research in Translational Medicine, Universidad Austral, CONICET, Pilar, Buenos Aires, Argentina David Furman * Division of Cellular & Molecular Biology, Toronto General
Hospital Research Institute (TGHRI), University Health Network, Toronto, ON, M5G 1L7, Canada Daniel A. Winer Authors * Fei Wu View author publications You can also search for this author
inPubMed Google Scholar * Huixun Du View author publications You can also search for this author inPubMed Google Scholar * Eliah Overbey View author publications You can also search for this
author inPubMed Google Scholar * JangKeun Kim View author publications You can also search for this author inPubMed Google Scholar * Priya Makhijani View author publications You can also
search for this author inPubMed Google Scholar * Nicolas Martin View author publications You can also search for this author inPubMed Google Scholar * Chad A. Lerner View author publications
You can also search for this author inPubMed Google Scholar * Khiem Nguyen View author publications You can also search for this author inPubMed Google Scholar * Jordan Baechle View author
publications You can also search for this author inPubMed Google Scholar * Taylor R. Valentino View author publications You can also search for this author inPubMed Google Scholar * Matias
Fuentealba View author publications You can also search for this author inPubMed Google Scholar * Juliet M. Bartleson View author publications You can also search for this author inPubMed
Google Scholar * Heather Halaweh View author publications You can also search for this author inPubMed Google Scholar * Shawn Winer View author publications You can also search for this
author inPubMed Google Scholar * Cem Meydan View author publications You can also search for this author inPubMed Google Scholar * Francine Garrett-Bakelman View author publications You can
also search for this author inPubMed Google Scholar * Nazish Sayed View author publications You can also search for this author inPubMed Google Scholar * Simon Melov View author publications
You can also search for this author inPubMed Google Scholar * Masafumi Muratani View author publications You can also search for this author inPubMed Google Scholar * Akos A. Gerencser View
author publications You can also search for this author inPubMed Google Scholar * Herbert G. Kasler View author publications You can also search for this author inPubMed Google Scholar *
Afshin Beheshti View author publications You can also search for this author inPubMed Google Scholar * Christopher E. Mason View author publications You can also search for this author
inPubMed Google Scholar * David Furman View author publications You can also search for this author inPubMed Google Scholar * Daniel A. Winer View author publications You can also search for
this author inPubMed Google Scholar CONTRIBUTIONS F.W., H.D., D.F., and D.A.W. contributed to the experimental design, execution, and analysis of the study. F.W., H.D., and D.A.W. drafted
the manuscript, and D.F. and S.W. edited the manuscript. H.D., F.W., C.A.L., N.M., J.M.B., H.H. P.M., T.R.V., H.G.K., N.S., and A.A.G. assisted with in vitro and experiment execution and
analysis of data. F.W., H.D., M.F., J.B., and K.N. performed computational analyses of single-cell datasets or validation data. E.O., C.M., J.K., and C.E.M. led I4 data acquisition and
sharing. M.M. led JAXA study data acquisition and sharing. A.A.G. performed microscopy image analysis. C.M., C.E.M., F.G-B., and A.B. led Twins study data acquisition and sharing. S.M.,
M.M., A.A.G., A.B., and C.E.M. supervised aspects of the study. D.F. and D.A.W. supervised the overall implementation of the study. All authors had an opportunity to view and edit the
manuscript. CORRESPONDING AUTHORS Correspondence to Christopher E. Mason, David Furman or Daniel A. Winer. ETHICS DECLARATIONS COMPETING INTERESTS D.F. is a founder of Edifice Health, a
company that utilizes inflammatory biomarkers (e.g., iAge) to predict age-related diseases. D.F., C.E.M., and D.A.W. are co-founders of Cosmica Biosciences, a company that studies altered
biological aging in spaceflight exposures. J.B. and F.W. are stakeholders in Cosmica Biosciences. A.A.G. has a financial interest in Image Analyst Software, which makes the software Image
Analyst MKII used here for image analysis. The Buck Institute (F.W., H.D., J.B., D.F., D.A.W.) has filed a patent application (application number: 63/520,327) on using simulated microgravity
to mimic and counter aging and spaceflight effects in cells based on data from this manuscript. The remaining authors declare no competing interests. PEER REVIEW PEER REVIEW INFORMATION
_Nature Communications_ thanks Xin Zhou, Junwei Shi, and the other, anonymous, reviewer(s) for their contribution to the peer review of this work. A peer review file is available. ADDITIONAL
INFORMATION PUBLISHER’S NOTE Springer Nature remains neutral with regard to jurisdictional claims in published maps and institutional affiliations. SUPPLEMENTARY INFORMATION SUPPLEMENTARY
INFORMATION PEER REVIEW FILE DESCRIPTION OF ADDITIONAL SUPPLEMENTARY FILES SUPPLEMENTARY DATA 1 SUPPLEMENTARY DATA 2 SUPPLEMENTARY DATA 3 SUPPLEMENTARY DATA 4 SUPPLEMENTARY DATA 5
SUPPLEMENTARY DATA 6 SUPPLEMENTARY DATA 7 SUPPLEMENTARY DATA 8 SUPPLEMENTARY DATA 9 SUPPLEMENTARY DATA 10 SUPPLEMENTARY DATA 11 SUPPLEMENTARY DATA 12 SUPPLEMENTARY DATA 13 SUPPLEMENTARY DATA
14 SUPPLEMENTARY DATA 15 SUPPLEMENTARY DATA 16 SUPPLEMENTARY DATA 17 SUPPLEMENTARY DATA 18 SUPPLEMENTARY DATA 19 REPORTING SUMMARY SOURCE DATA SOURCE DATA RIGHTS AND PERMISSIONS OPEN ACCESS
This article is licensed under a Creative Commons Attribution 4.0 International License, which permits use, sharing, adaptation, distribution and reproduction in any medium or format, as
long as you give appropriate credit to the original author(s) and the source, provide a link to the Creative Commons licence, and indicate if changes were made. The images or other third
party material in this article are included in the article’s Creative Commons licence, unless indicated otherwise in a credit line to the material. If material is not included in the
article’s Creative Commons licence and your intended use is not permitted by statutory regulation or exceeds the permitted use, you will need to obtain permission directly from the copyright
holder. To view a copy of this licence, visit http://creativecommons.org/licenses/by/4.0/. Reprints and permissions ABOUT THIS ARTICLE CITE THIS ARTICLE Wu, F., Du, H., Overbey, E. _et al._
Single-cell analysis identifies conserved features of immune dysfunction in simulated microgravity and spaceflight. _Nat Commun_ 15, 4795 (2024). https://doi.org/10.1038/s41467-023-42013-y
Download citation * Received: 06 December 2022 * Accepted: 27 September 2023 * Published: 11 June 2024 * DOI: https://doi.org/10.1038/s41467-023-42013-y SHARE THIS ARTICLE Anyone you share
the following link with will be able to read this content: Get shareable link Sorry, a shareable link is not currently available for this article. Copy to clipboard Provided by the Springer
Nature SharedIt content-sharing initiative