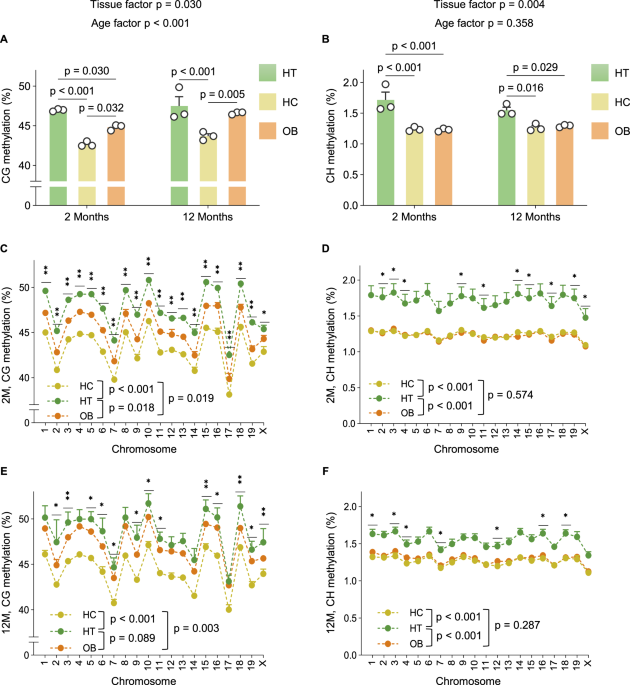
Targeting the hypothalamus for modeling age-related dna methylation and developing oxt-gnrh combinational therapy against alzheimer’s disease-like pathologies in male mouse model
- Select a language for the TTS:
- UK English Female
- UK English Male
- US English Female
- US English Male
- Australian Female
- Australian Male
- Language selected: (auto detect) - EN
Play all audios:

ABSTRACT The hypothalamus plays an important role in aging, but it remains unclear regarding the underlying epigenetics and whether this hypothalamic basis can help address aging-related
diseases. Here, by comparing mouse hypothalamus with two other limbic system components, we show that the hypothalamus is characterized by distinctively high-level DNA methylation during
young age and by the distinct dynamics of DNA methylation and demethylation when approaching middle age. On the other hand, age-related DNA methylation in these limbic system components
commonly and sensitively applies to genes in hypothalamic regulatory pathways, notably oxytocin (OXT) and gonadotropin-releasing hormone (GnRH) pathways. Middle age is associated with
transcriptional declines of genes which encode OXT, GnRH and signaling components, which similarly occur in an Alzheimer’s disease (AD)-like model. Therapeutically, OXT-GnRH combination is
substantially more effective than individual peptides in treating AD-like disorders in male 5×FAD model. In conclusion, the hypothalamus is important for modeling age-related DNA methylation
and developing hypothalamic strategies to combat AD. SIMILAR CONTENT BEING VIEWED BY OTHERS NONCANONICAL REGULATION OF IMPRINTED GENE _IGF2_ BY AMYLOID-BETA 1–42 IN ALZHEIMER’S DISEASE
Article Open access 04 February 2023 FTO-MEDIATED M6A DEMETHYLATION REGULATES GNRH EXPRESSION IN THE HYPOTHALAMUS VIA THE PLCΒ3/CA2+/CAMK SIGNALLING PATHWAY Article Open access 21 December
2023 CORTICOSTERONE INDUCES DISCRETE EPIGENETIC SIGNATURES IN THE DORSAL AND VENTRAL HIPPOCAMPUS THAT DEPEND UPON SEX AND GENOTYPE: FOCUS ON METHYLATED _NR3C1_ GENE Article Open access 16
March 2022 INTRODUCTION Aging-related neurodegenerative diseases such as AD have become major health problems among the elderly, but clinical solutions are still missing partly due to the
incomplete knowledge of this disease. Interestingly, recent research has suggested that the functioning of neurogenic brain regions in early life could have long-term effects on
aging-related neuronal degeneration and loss1,2,3,4. As is known, there are three major types of neurogenic brain regions, including the hippocampal dentate gyrus5,6, the subventricular
zone7,8,9,10, and the mediobasal hypothalamus11,12,13, which are responsible for adult neurogenesis related to the limbic system functions of the hippocampus, olfactory bulb (OB), and
hypothalamus, respectively. Recently, our research has led to an understanding that the hypothalamus plays a regulatory role in systemic aging and systemic physiology11,12,13,14,15, while
the underlying basis involves the actions from hypothalamic neuropeptides such as GnRH and OXT15,16,17,18. However, to date there is still a lack of research comparing the hypothalamus and
other limbic system components in the context of addressing aging or aging-related diseases. To fill this gap, we designed this research project to comparatively study the hypothalamus
versus the hippocampus and OB in the context of aging. For our approach, we resorted to DNA methylation epigenetic analysis, given the close relationships between aging and DNA methylation
established in research19,20,21,22,23,24,25,26,27,28,29,30,31,32,33. We were inspired to employ this approach, also because we aimed to explore if age-related DNA methylation in the
hypothalamus could be informative for developing hypothalamic peptide and/or endocrine approaches to address aging-related diseases such as AD. Hence, we carried out this project and
obtained multifaceted data supporting the hypothesis that the hypothalamus is important for modeling age-related DNA methylation epigenetics and developing a related hypothalamic strategy
based on the OXT-GnRH combinational therapy to combat AD. RESULTS AGE-RELATED DNA METHYLATION IN THE HYPOTHALAMUS VS. HIPPOCAMPUS AND OB To profile age-related hypothalamic DNA methylation
in standard C57BL/6 mice, we studied the hypothalamus which was compared to two other limbic system components: the hippocampus and OB. We employed bisulfite oligonucleotide-captured
sequencing (BOCS), a technique established for profiling CG-rich genomic regions. This approach covers all annotated promoters, CG islands, CG shore regions (±2 kb from each CG island), and
CG shelf regions (±2 kb from each shore and ±4 kb from each CG island)34,35. The hypothalamus, hippocampus, and OB were isolated from standard male C57BL/6 mice at the age of 2 months vs. 12
months, representing young and middle age, respectively. Studying the transition from young age to middle age can help identify early changes that could be more causally significant for
subsequent aging-related diseases. All data from this BOCS method were confirmed to meet the standard through quality check (Suppl. Figure 1 ̶ 3). Then, we computed the average methylation
levels of CG and CH sites (H stands for A, T, or C) for each tissue. As shown in Fig. 1A and B, it immediately gained our attention that compared to the hippocampus and OB, DNA methylation
levels were distinctly highest in the hypothalamus of young mice, but the difference tended to be less distinct in middle-aged mice. These overall relationships were also seen in many
individual chromosomes, as demonstrated in chromosome-wise analysis of these brain regions of young mice (Fig. 1C, D) and middle-aged mice (Fig. 1E, F). Taken together, apparently due to
different dynamics of CG methylation and CH demethylation, the pattern of DNA methylation in the hypothalamus distinctly differs from the patterns in the other two brain regions at young
age, but this difference declines when approaching middle age. REGION-RELATED DNA DIFFERENTIAL METHYLATION AT YOUNG AGE AND THE CHANGES BY MIDDLE AGE We then computed age-related
differentially methylated cytosines (DMCs) in each brain region between young and middle age but found only a small number of sites with statistical significance. We also examined how
differential methylation between brain regions could change during the transition to middle age. We found that DMCs between the hypothalamus and the other two brain regions were more heavily
attributed to hypermethylation than to hypomethylation; in contrast, DMCs between the hippocampus and OB were similarly attributed to hypermethylation and hypomethylation. These findings
are summarized in the Volcano plot (Fig. 2) and detailed with chromosome-wide analysis in the Manhattan plot (Suppl. Figure 4, Suppl. Dataset 1). By comparing the two age groups, we found
that DMCs between the hypothalamus and either of the other two brain regions were more than 60% lost by middle age; in contrast, DMCs between the hippocampus and OB in young mice were only
about 40% lost by middle age. Certainly, cellular heterogeneity among different brain regions can importantly account for DMCs between regions, but we expect that composition of major cell
types in a given tissue remains stable by middle age. Thus, we adopted a deconvolution method to test this point, as this approach has been established in the literature to computationally
analyze cell compositions in tissue samples36,37,38,39,40. Our deconvolution analysis led to the projection of 7 major cell types in each brain region (Suppl. Fig. 5); the potential
identities of these major cell types are only suggested, based on previous single-cell analysis of these brain regions in the literature41,42,43. Our analysis supports the point that middle
age does not significantly change the composition of these major cell types. Hence, while we do not exclude a contribution from smaller populations or subtypes, major cell types do not seem
to be a driving factor for changes in region-related differential methylation among these brain tissues as animals approach middle age. AGE-RELATED DIFFERENTIAL METHYLATED REGIONS IN THE
HYPOTHALAMUS, HIPPOCAMPUS AND OB Apart from DMC analysis, we also carried out differential methylation regions (DMRs) analysis, which span lengths of DNA sequences and thus can reveal DNA
methylation information more globally than locally. We analyzed age-dependent DMRs according to DNA length ranging from 300 to 1000 base pairs (bps). While the analysis yielded variable
numbers of DMRs due to changes in DNA lengths, three brain regions consistently exhibited similar patterns, as reflected by an analysis with DNA length of 500 bps (Fig. 3A, Suppl. Dataset
2). Remarkably, age-dependent DMRs in the hypothalamus exhibited a more balanced distribution between hypermethylation and hypomethylation, in sharp contrast to the unbalanced patterns in
the other two brain regions, where hypermethylation was stronger than hypomethylation (Fig. 3A). Also, as shown in Fig. 3B, chromosome-wide analysis further confirmed a balanced number of
hypothalamic DMRs between hypermethylation and hypomethylation; in contrast, DMRs in the hippocampus and OB were attributed to more hypermethylation than hypomethylation. Subsequently, we
explored the genes involved in age-related DMRs in these brain regions. We identified a pool of genes impacted across the hypothalamus, hippocampus, and OB, constituting approximately 12%,
9%, and 7% of the total affected genes in these brain regions, respectively. Overall, we identified 173 genes mapped onto DMRs due to aging across all three brain regions (Fig. 3C). Among
these 173 genes, 94, 60, and 34 genes showed more hypomethylation than hypermethylation in the hypothalamus, hippocampus, and OB, respectively, indicating a bias towards hypomethylation in
the hypothalamus compared to the other two brain regions. Subsequently, we conducted pathway analysis based on these 173 shared genes. As demonstrated in Fig. 3D, many of these genes were
associated with hypothalamic functions, such as circadian rhythm, reproductive function, endocrine gland hormones, feeding, metabolism, cardiovascular function, and immunity, suggesting that
DNA methylation changes related to hypothalamic function could be shared with other brain regions. PATHWAY ANALYSIS PER AGE-RELATED DMRS IN THE HYPOTHALAMUS, OB, AND HIPPOCAMPUS We
performed further analysis focusing on individual brain regions to explore the specific molecular pathways affected by age-related changes in DNA methylation. Our investigation involved
subsequent mapping of the genes associated with DMRs to a manually curated database of mouse cell signaling and metabolic pathways. Our findings, illustrated in Fig. 4A–C and Suppl. Dataset
3, highlighted the pathways influenced by age-related differential DNA methylation, characterized by the number of genes and their collective significance. Specifically, the pathways
identified for the hypothalamus included many components that regulate circadian rhythms and the hypothalamic reproductive peptides OXT and GnRH network (Fig. 4A). Of interest, the pathways
identified for the hippocampus and OB were also found to be significantly related to hypothalamic regulatory functions, for example, the circadian entrainment pathway, reproductive OXT
pathway, cortisol synthesis and secretion pathway, Cushing syndrome pathway, and aldosterone synthesis and secretion pathway for the hippocampus (Fig. 4B) and the circadian entrainment
pathway, OXT signaling pathway, and GnRH secretion pathway for OB (Fig. 4C). Most notably, based on two different computational approaches in Figs. 3 and 4, reproductive neuropeptide OXT and
GnRH pathways repeatedly emerged not only in the hypothalamus but also in the hippocampus and OB. In this context, we analyzed how OXT signaling could be related to GnRH signaling using a
protein-protein interaction program, showing a good number of co-components in OXT and GnRH signaling pathways (Suppl. Figure 6). Utilizing BOCS metadata, we investigated how aging affects
the methylation statuses of genes encoding OXT signaling and GnRH signaling components. As laid out in Fig. 4D, by middle age, many components in OXT signaling and GnRH signaling pathways
show age-dependent changes in DNA methylation status in the hypothalamus, hippocampus, and OB. Interestingly, in each brain region, a specific set of OXT and GnRH signaling components was
differentially methylated when comparing young and middle-age samples. Hence, aging is associated with diverse DNA methylation changes in the genes encoding molecular components of OXT and
GnRH signaling, with these variations being brain region-specific. DNA METHYLATION AND TRANSCRIPTION OF OXT AND GNRH GENES IN AGING OR AD-LIKE MODEL Our earlier work separately related GnRH
and OXT to systemic aging or physiology15,16,17,18, altogether suggesting that these peptides might have a close relationship in influencing brain health in conditions such as aging, an idea
which is validated through the DMR analysis presented above. Hence, we decided to directly examine the methylation statuses of _Oxt_ and _Gnrh1_ genes between young and middle-aged mice.
Since the hypothalamus exclusively produces both peptides during adulthood, we focused on analyzing DNA methylation data from the hypothalamic samples. We found that a list of cytosines in
gene body and promoter region of _Oxt_ became hypomethylated, while a smaller list of cytosines became hypermethylated in the hypothalamus by middle age (Fig. 5A, Suppl. Figure 7A). There
was also a list of cytosines in _Gnrh1_ gene body and promoter, which underwent hypomethylation or hypermethylation by middle age (Fig. 5B, Suppl. Figure 7B). Subsequently, we examined the
mRNA levels of _Oxt_ and _Gnrh1_ in the hypothalamus of mice at young age (2 months) compared to middle age (15 months). Through qRT-PCR method, we obtained data showing that mRNA levels of
_Oxt_ and _Gnrh1_ both dramatically dropped in the hypothalamus during the transition from young to middle age (Fig. 5C). Given our interest in aging-related disease, we employed 5xFAD mice,
an AD-like model associated with aging, to assess if similar changes might occur in the hypothalamus prior to the onset of AD-like phenotype. Indeed, the transcription levels of _Oxt_ and
_Gnrh1_ were also evidently downregulated in the hypothalamus of 5xFAD mice compared to wildtype controls (Fig. 5D). These results lend support to our prediction that declines in OXT and
GnRH pathways are co-involved in AD-like conditions which are associated with aging. DNA METHYLATION AND TRANSCRIPTION OF ADCY FAMILY MEMBERS IN AGING OR AD-LIKE MODEL In addition to _Oxt_
and _Gnrh1_, we noted that our age-related DMR analysis across the hypothalamus, hippocampus, and OB shown in Fig. 4 contained several adenylate cyclases (Adcy), a family of proteins that
co-mediate OXT and GnRH signaling (Suppl. Fig. 6). By modulating cAMP levels, Adcy members link OXT and GnRH stimulation to downstream protein kinases that regulate various events ranging
from gene expression and neurotransmitter release to synaptic plasticity. Abnormal _Adcy_ expression is associated with many neurological disorders, including AD and depressive
disorders44,45. For instance, loss of _Adcy5_ results in Parkinson’s disease-like disorders46, and _Adcy7_ is linked to familial major depression in both mice and humans47,48. Hence, our
methylation analysis further narrowed down to the promoter regions of _Adcy_ members, given the important role of promoters in gene expression, focusing on the hippocampus because of its
critical role in cognitive regulation. We found that total methylation levels in the promoter regions of several _Adcy_ genes were significantly lower in middle-aged mice compared to young
mice, while _Adcy_ genes often underwent hypomethylation in the proximal promoter regions by middle age (Fig. 6A). In this context, we examined hippocampal mRNA levels and found that middle
age was associated with significant downregulation across many _Adcy_ genes (Fig. 6B). Also, we examined the hippocampus of 5xFAD model and observed that hippocampal mRNA levels of several
_Adcy_ members were lower in this AD-like model compared to littermate controls (Fig. 6C). Taken together, transcription of _Oxt_ and _Gnrh1_ in the hypothalamus, as well as transcription of
_Adcy_ members in the hippocampus, are commonly defective in both the aging and the 5xFAD model. THERAPEUTIC SIGNIFICANCE OF OXT-GNRH COMBINATIONAL TREATMENT IN AD MOUSE MODEL While this
work began with DNA methylation analysis based on aging model, our analysis led to identification of OXT and GnRH pathways, which we confirmed at the gene transcriptional level to be
significantly altered in an AD-like model which is associated with aging. In the literature, a few studies recently suggested an effect of OXT in attenuating amyloid β (Aβ) deposition or
cognitive impairment in ddY or APP/PS1 mice or Sprague Dawley rats49,50,51,52,53,54. Since these studies were based on experimental conditions with relatively mild cognitive disorders, the
effects of OXT in treating AD still remain to be defined. Regarding GnRH, researchers have studied its potential in treating diseases such as cancers, and recently, it was shown to have an
effect in improving cognition in Down syndrome and dementia55,56,57, but the effect of GnRH on AD is unclear and it remains completely unknown if OXT and GnRH might be used in combination to
synergistically and effectively combat against AD. Hence, we studied a 9-month-old 5xFAD mouse model with strong manifestation of AD-like phenotypes, focusing to compare OXT-GnRH
combinational treatment vs. individual peptide treatment. We employed nasal administration, a method which has often been used in research to deliver peptides into the brain for treating
neurological diseases58,59,60,61,62,63,64. To help discern the effects of combinational vs. individual treatment, OXT and GnRH were administered nasally at relatively low doses (50 ng and 5
ng, respectively) for a relatively short treatment duration (2 months). After the completion of therapy, animals were assessed with a battery of non-invasive neurobehavioral assays,
including an open field, grip strength, y-maze, novel object recognition, social interaction, and Morris Water Maze for various aspects of physical and cognitive functions. As demonstrated
in Fig. 7, OXT-GnRH combinational treatment led to very robust and strong effects against various neurological disorders in this AD-like model, while the effects from single peptide
treatment were modest or non-significant. At the end, we assessed how OXT-GnRH therapy could affect Aβ levels in this model. Indeed, 5×FAD model had a very large amount of Aβ plaques
throughout various brain regions, represented by hippocampal CA1, CA3 and dentate gyrus (DG), entorhinal cortex, and mediobasal hypothalamus. In agreeing with the physiological effects,
OXT-GnRH combinational treatment led to a nearly complete reversal of Aβ deposition (Fig. 8). Compared to the combinational treatment, single peptide treatments were much less effective,
except that GnRH treatment was also sufficient to reduce Aβ plaques in the mediobasal hypothalamus, while Aβ plaques in fornix bundle in this region were not amended by any treatment. Taken
together, compared to single peptide treatment, OXT-GnRH combinational therapy increased the sensitivity and effectiveness in treating neurological disorders and reducing Aβ plaques in male
5×FAD model. Finally, given the observed transcriptional changes of _Adcy_ members in 5xFAD model (Fig. 6), we additionally assessed how OXT-GnRH treatment, compared to individual peptide
treatment, might affect promoter methylation of these _Adcy_ genes. Thus, we utilized the Next-Generation Sequencing-based Bisulfite Sequencing PCR (NGS-BSP) method to sequence the promoter
subregions of candidate _Adcy_ genes, focusing on subregions between CG island and encoding sequence. We performed target sequencing on hippocampal tissues obtained from the 5xFAD mice
following combinational versus individual treatment with OXT and GnRH, as described in Fig. 7. Although either treatment did not cause significant changes in average methylation levels of
the promoter subregions which we sequenced, our DMC analysis did lead to identification of several short sequences in which cytosine methylation levels were altered differently by OXT-GnRH
treatment compared to single peptide treatment (Suppl. Fig. 8). Admittedly, this research is still limited, but it might point to a future direction for studying loci effects of OXT-GnRH
treatment on gene promoter activity and if it could significantly affect the transcription of key genes in contributing to the combinational therapy against AD-like pathologies. DISCUSSION
In this project, we computationally analyzed age-related DNA methylation in the hypothalamus compared to two other limbic system components in a standard mouse model. This analysis led to
the discovery that the hypothalamus is characterized by distinctively high-level DNA methylation at young age and the distinct dynamics of DNA methylation and demethylation when approaching
middle age. Thus, compared to the other two limbic system regions, the hypothalamus contains more DNA methylation information during young age, contributing to its unique pattern of DNA
methylation prior to middle age. During the course of approaching middle age, this distinction of DNA methylation between the hypothalamus and the other limbic system regions becomes to be
declined. Thus, while aligning with the ‘hypothalamic control of aging’ paradigm which we previously proposed12,14,15, we further speculate that hypothalamic regulation of mammalian aging
could be causally related to loss of epigenetic information from DNA methylation in the genomes of the hypothalamus. In this context, more brain regions should be compared to the
hypothalamus in light of age-related DNA methylation. We also surprisingly found that not only the hypothalamus but also other limbic system regions commonly involve age-related DNA
methylation of genes related to various hypothalamic endocrine pathways and notably OXT and GnRH pathways. This finding suggests the importance of the hypothalamus in age-related brain
regional DNA methylation, warranting future research. Furthermore, the identification of OXT and GnRH pathways in this computational analysis lends support for investigating whether these
neuropeptides could be designed to target aging-related diseases. Hence, using an AD-like condition as an important example of aging-related diseases, we focused intensively on hypothalamic
neuropeptide OXT and GnRH pathways for the potential therapeutic development. Following the observation that multiple components in OXT and GnRH pathways become declined in relevant brain
regions at the gene transcriptional level in an AD-like mouse model, we were inspired to evaluate the effects of these two neuropeptides in combination versus individually for treating
AD-like pathologies in this mouse model. This effort led to a major finding, that is, OXT-GnRH combinational therapy had a robust effect against the severe symptoms in an aged 5×FAD mouse
model, showing significantly greater efficacy than individual peptide treatments. This finding can suggest a clinical strategy of using OXT-GnRH combinational therapy to target AD, which
would call for clinical research down the road. In the literature, OXT has recently gained some attention mostly for reducing Aβ deposition49,50,51,52,53,54, while it remains unknown if GnRH
could be used to treat AD. It is likely that individual peptides, especially when dose and treatment conditions are stronger, can each have a better effect on AD-like disorders, but
strategy of developing combinational treatments should have advantages, for instance, low dose of each peptide helps minimize off-target effects, and some therapeutic effects are expected to
require interactions and synergy of the two neuropeptide pathways which might not be achievable by individual peptides. Our study still has limitations, mostly due to practical constraints
during developing this project. For the computational study, we have not compared the hypothalamus with other limbic system components or other brain regions; to do so will be valuable to
solidify the model on hypothalamic DNA methylation modeling of aging. Technically, we recognize a limitation that we employed 3 replicates of animals per group in our computational assays
although it was often similarly used in the literature65,66,67, hence, to include more samples will likely lead to more information. Moreover, while our computational work was based on an
aging model, it would be more informative to include samples from aging-related diseases such as AD. For the therapeutic study, while our work was to initially establish the proof of
principle for using OXT-GnRH combination to target AD-like pathologies, future research is still needed to optimize dosage and duration, to assess additional ages and AD conditions, and to
profile any off-target effects. In particular, we acknowledge a limitation due to the absence of studies on female model, which needs future research to address. Last but not least, while
our therapeutics was based on an AD-like model as an example of aging-related disease, it will be very valuable to assess if this strategy could apply to other aging-related diseases, for
example, Parkinson’s disease. METHODS This study was conducted in compliance with all relevant ethical regulations. The animal research protocol was reviewed and approved by the
Institutional Animal Care and Use Committee of the Albert Einstein College of Medicine (Protocols #00001111, #00001385, #00001397, #00001398, #00001399 and their previously approved
versions). ANIMAL MODELS C57BL/6 mice and 5×FAD mice were obtained from Jackson Laboratory and housed under standard conditions in a 12-h light/12-h dark cycle with free access to food and
water. All mice in this study were male. All mice were kept under standard, infection-free housing with 3 to 5 mice per cage. Pathogen-free quality of mouse colonies was ensured through
quarterly serology, quarterly histopathologic exams and daily veterinarian monitoring of animal health and care. All mice were fed a standard chow from Lab Diet. Perfusion-induced euthanasia
was used for tissue sampling and carbon dioxide inhalation euthanasia was used for all other mice which were generated during the study according to the IACUC approved protocols. BISULFITE
OLIGONUCLEOTIDE-CAPTURED SEQUENCING (BOCS) The hypothalamus, hippocampus, and OB were obtained from standard, chow-fed male C57BL/6 mice at 2 vs. 12 months of age (_n_ = 3 mice per group).
DNA was extracted from these tissues using a Qiagen kit (Cat# 69506). The extracted DNA samples were then sheared into fragments of 150–200 base pairs using sonication, and the fragment
sizes were verified using the Agilent TapeStation with D1000 ScreenTape. The fragmented DNA underwent library preparation using the SureSelectXT Methyl-Seq Library Prep Kit, following the
manufacturer’s instructions. The generated libraries were subsequently hybridized with the SureSelectXT Mouse Methyl-Seq capture set for 16 h at 65 °C, specifically targeting all CG regions
including all annotated promoters, CG islands and shore regions (±2 kb from islands) as well as shelf regions (±2 kb from shores and ±4 kb from islands). The hybridized libraries were
captured using DynaBeads MyOne Streptavidin T1 magnetic beads. The captured libraries were eluted from magnetic beads using 0.1 M NaOH. Unmethylated cytosine residues in the captured DNA
libraries were then converted to uracil through bisulfite conversion using the EZ DNA Methylation-Gold Kit from Zymo Research. Following bisulfite conversion, DNA was desulfonated and
amplified via PCR. The amplified libraries were purified using AMPure XP beads. The purified libraries were indexed, allowing for the multiplexing of samples in a single sequencing run.
Quantification of DNA libraries was performed using the TapeStation with D1000 ScreenTape. The libraries were normalized to a concentration of 4 nM, pooled together, and further diluted to a
final concentration of 12 pM. The DNA libraries were sequenced on an Illumina MiSeq PE75 for quality control and sequenced on NextSeq500 High PE75 to obtain DNA methylation profiles. DNA
METHYLATION ANALYSIS FASTQ files were accessed from bisulfite sequencing for quality control with FastQC and paired-end reads were trimmed for quality with Illumina’s BaseSpace sequence hub
having MethylSeq v2.0.0. Trimming involved removing 3 nucleotides at the 5′ end and 4 nucleotides at the 3′ end, as well as adapter removal using FASTQ Toolkit v2.2. Only reads with a
Q-score >30 were used for mapping, reads which did not meet criteria after trimming were discarded. Alignment of trimmed bisulfite converted sequences was carried out using Bismark
Bisulfite Mapper v0.14.4/Bowtie 2 v2.2.2 against the mouse reference genome (GRCm38/mm10)68,69. Each sample yielded over 25 million aligned reads, resulting in an average target sequence
coverage ranging from 18 to 20×. The aligned BAM files were processed using SAMtools v1.270. After read mapping, methylation calling was carried out using the Bismark methylation extractor.
The percentage of methylation was determined by calculating the ratio of methylated cytosines to the total number of cytosines covered in the genome for each specific cytosine position.
Differential methylation analysis was primarily conducted in R v4.1.2, using MethylKit v1.20.071. Differentially methylated cytosines were determined from sites passing coverage criteria of
minimum 10. Logistic regression was employed to determine differentially methylated sites between tissues, and p-values were adjusted using a false-discovery rate (q-value). Sites with
q-value < 0.05 and methylation difference of ≥25% were considered statistically significant. For computing DMRs, criteria were set at a length of 500 bp showing >10% average
methylation difference. Gene annotation files for the mouse (GRCm38) were downloaded from Gencode and mapped with DMRs using BEDTools v2.30.071,72. Deconvolution analysis, a method for
analyzing cellular heterogeneity based on methylation sequencing data36,73,74,75, was used to computationally assess tissue heterogeneity of cell populations. The computation was based on
using DXM (Deconvolution of Subpopulations Existing in Methylation Data), a reference-free approach offering versatility across various Bisulfite Sequencing (BS) methodologies39 which was
established in the literature to accurately infer cell-type proportions40. DXM’s initial module “dxm_estimateFracs” was used within the Python environment. Enrichr was used to perform KEGG
pathway analysis, allowing the identification and enrichment analysis of genes within specific biological pathways76. Cytoscape, in conjunction with the String module, with confidence
>0.4 as the cut-off criteria, was employed to construct protein-protein interaction network, visualization and analysis of interconnections between pathway components77,78. Data were
processed and visualized in Python, and computational analyses were conducted using custom scripts or published tools in UNIX, R and Python environments. ANIMAL TREATMENT AND BEHAVIORAL
ASSAYS Male 5xFAD mice under standard housing and feeding were randomly assigned into various treatment groups to receive 50 ng OXT (Cat # 04375-1000IU, Sigma Aldrich) and 5 ng GnRH (Cat #
PEP-168, Invitrogen) treatment through daily nasal administration. Following 2-month treatment, animals were sequentially examined with a panel of non-invasive behavioral assays with
adequate recovery time between different assays. All behavioral tests were performed in an isolated and designated mouse behavioral room. An anymaze video-tracking system (version 4.99 m,
Stoelting) connected with a digital camera and computer was used to record the activities of mice during behavioral procedures. Grip test: Each mouse was positioned on a square grid with a
1-cm mesh, which was then inverted 30 cm above a protective pad. The mouse was then allowed to hang by its paws for a specified period, and the duration for which the mouse remained
suspended was recorded. Three repeats were performed for each animal with 30 min rest time between trials. Open field test: A mouse was placed in the lower right corner of a spacious, empty
white plastic chamber (40 cm length × 40 width and × 40 cm height) and was allowed to freely move in the chamber while movement and behavior were recorded by the camera for 10 min. Y-maze
test: A mouse was placed in the center of Y-maze apparatus and allowed to freely explore for 10 min. The time spent in each arm and the number of arm entries were recorded and analyzed. An
entry was considered a success when all four limbs entered an arm. Novel object recognition: A mouse was allowed to freely explore an open-field box (40 cm in length, 40 cm in width, and 40
cm in height) for 10 min prior to experimental sessions. During the familiarization session, the mouse was then allowed to freely explore two similar objects for 10 min. During the test
session, one of the two objects was replaced by a novel object for 10 min. The amount of time that the mouse spent exploring each object was recorded. A preference index was calculated using
the ratio of the amount of time exploring each object over the total time exploring both objects. Morris water maze (MWM): The test was performed using a round water tank (90 cm in
diameter) containing water at temperature of 22 to 23 °C and a non-toxic paint which was added to make opaque and white background. A circular, background color-matched platform with a
diameter of 10 cm was placed 25 cm from the wall of the tank and about 1 cm below the water surface which was thus invisible. Animals were trained for 5 days, 4 training sessions each day,
and the beginning positions for each training day were randomly chosen. At each training session, mice were placed in a beginning position in the maze and were trained to find the platform
within 60 s. Latency to reach the platform of each trial was recorded. On the next day following 5-day training sessions, probe trials were performed by removing the platform and the animal
was allowed to swim for 60 s and measured for swimming speed and the latency that the mouse swam to cross the location where the platform was previously located. Sociality: Social
interaction was tested in a gray 3-chamber neutral box cage (60 cm in length, 40 cm in width, and 22 cm in height). For adaptation phase, animals were allowed to explore freely for 10 min in
the neutral cage for habituating the testing conditions. For social affiliation phase, a new mouse (stranger) in a wire containment cup was placed in a side chamber. The subject mouse was
allowed free access to explore each of three chambers for 10 min. For preference testing phase, a second new mouse (new stranger) in a wire containment cup was placed in the opposite side
chamber. The subject mouse was allowed to freely explore each of three chambers for 10 min. The time spent in social interaction by sniffing was recorded. BRAIN SECTIONS AND IMMUNOSTAINING
The mice from the above therapeutic experiment were used for brain Aβ immunostaining (subgroup of brain samples from these mice used for DNA promoter target sequencing). To obtain brain
samples, mice were anesthetized with 3–5% isoflurane inhalation and were transcardially perfused with saline over 5 min before brains were collected. Brain hemispheres were post-fixed with
4% PFA and then infiltrated with 20–30% sucrose for immunostaining. Brain sections were generated with a thickness of 20 μm using a cryostat. To facilitate Aβ immunostaining, sections were
treated with 88% formic acid to expose the antigen, followed by blocking with a buffer solution containing 5% BSA, 5% goat serum, and 0.1% Triton X-100 in PBS. Subsequently, brain sections
were incubated overnight at 4 °C with mouse anti-Aβ primary antibody (anti-β-Αmyloid, BioLegend, #803003, 1:500) in a blocking buffer. Technical controls included appropriate species-matched
naive IgGs. Following three washes, the sections were incubated with secondary antibody (Goat anti-mouse IgG antibody Alexa Fluor 555, Thermo Fisher, #A21422, 1:500), and after three
washes, sections were covered with a mounting medium containing DAPI for visualizing the nuclei of cells in the sections. IMAGE CAPTURE AND QUANTIFICATION Brain sections were imaged for a
target region using the LAS X software-equipped Leica Stellaris 8 confocal microscope. The same confocal setting and parameters were used for all samples in this study. Confocal scanning was
set at 600 Hz scan speed, 4 lines average, and 2 frame average. 20×/0.75 dry objective was used for imaging hippocampal subregions, and 10×/0.40 dry objective used for imaging other brain
regions. Aβ plaque signals were imaged with an ALEXA channel at excitation wavelength of 553 nm and emission light collected with a wavelength of 558 to 730 nm. DAPI staining signal was
imaged at excitation wavelength of 405 nm and emission light collected with a wavelength of 430 to 558 nm. The pinhole size was set at 56.7 μm for both channels. Images were exported as
scaled viewer tiff files with lossless compression. ImageJ 1.54 f was used to quantitate image signal of interest. The same settings of ImageJ were used to process all images in this study.
RGB images were converted into 8-bit grayscale format for each channel by ImageJ, and the entire region of interest (ROI) was delineated according to the anatomic structure. Aβ plaques in an
ROI were subsequently identified using a threshold signal of 122 to 255 scale, and the plaque density of a target brain region was calculated as Aβ signal per area of ROI. QUANTITATIVE
RT-PCR The hypothalamus and hippocampus samples were obtained from standard male C57BL/6 mice at the age of 2 vs. 15 months and from male 5xFAD mice and littermate male WT controls at the
age of 8 months for extraction of total RNA using TRIzol reagent (Invitrogen), followed by reverse transcription using High-Capacity RNA to cDNA kit (Thermo Fisher) according to the
manufacturer’s instructions. Quantitative real-time PCR was conducted using Power SYBR Green PCR Master Mix (Thermo Fisher) with specific primer sets (see below). β-actin was used as a
reference and changes were calculated using 2-ΔΔCt method. _Oxt_: 5ʹ-GCTGCCAGGAGGAGAACTAC-3ʹ; 5ʹ-GGCAGCCATCTGCAAGAGAA-3ʹ _Gnrh1_: 5ʹ-GCATTCTACTGCTGACTGTGTGTT-3ʹ; 5ʹ-GTTCTGCCATTTGATCCACCT-3ʹ
_Adcy3_: 5ʹ-TCTTTGACTGCTACGTGGTAGT-3ʹ; 5ʹ-GGCCCGTGAAAAGTTCAGG-3ʹ _Adcy5_: 5ʹ-AAGATCCTCGGGGATTGTTACT-3ʹ; 5ʹ-CTCCCGGACCAACGAGATG-3ʹ _Adcy7_: 5ʹ-AAGGGGCGCTACTTCCTAAAT-3ʹ;
5ʹ-GTGTCTGCGGAGATCCTCA-3ʹ _Adcy9_: 5ʹ-CAACAGCGTGAGGGTCAAGAT-3ʹ; 5ʹ-CATGGAGTCGAATTTGGGGTC-3ʹ _β-actin_: 5ʹ-CCTCTATGCCAACACAGTGC-3ʹ; 5ʹ-GCTAGGAGCCAGAGCAGTAA-3ʹ STATISTICS AND REPRODUCIBILITY
Sample sizes were designed considering the relevant literature for physiological, biochemical and histological experiments12,14,15,79,80, relevant literature for DNA bisulfite
sequencing65,66,67, and relevant literature for target bisulfite sequencing67,81. Neurobehavioral tests were performed making group information blind to experimental performers when
collecting the data. All physiological and histological results represented repeated observations independently and through complimentary approaches. All data with biological replicates were
presented as mean ± S.E.M. The number of biological replicates and applied statistical methods are detailed in figure legends. Data were analyzed for parametric or non-parametric
distributions with statistical tools such as Shapiro-Wilk test, D’Agostino-Pearson test, Anderson-Darling test, and Kolmogorov-Smirnov test. Data that followed parametric distribution were
analyzed using ANOVA with Tukey’s multiple comparisons when involving more than two groups and two-tailed unpaired Student’s t-test when involving only two groups. Data that did not follow
parametric distribution were analyzed including Kruskal-Wallis test and Dunn’s multiple comparisons when having more than two groups and two-tailed Mann-Whitney test when having only two
groups. Additional statistics included logistic regression and Fisher’s Exact test contained in applied computational programs. Statistical significance of all data was determined at _p_
< 0.05. For computing differential methylation sites and regions, logistic regression-based modeling was applied in R-based MethylKit v1.20.0, with a false discovery rate set at _q_ <
0.05. REPORTING SUMMARY Further information on research design is available in the Nature Portfolio Reporting Summary linked to this article. DATA AVAILABILITY BOCS datasets for all
biological samples generated in this study are available in the NCBI Gene Expression Omnibus (GEO) repository with accession number GSE276875. Target promoter subregional data and raw
immunofluorescence images are deposited in publicly accessible platform Figshare (https://figshare.com/s/c2ed30d10acfa8a43f56). Source data are provided with this paper. There is no
restriction on data availability in this manuscript, and research materials developed from this study are freely available to research upon request. Source data are provided with this paper.
CODE AVAILABILITY Code/scripts for computational analysis are deposited in the publicly accessible GitHub repository
(https://github.com/DongshengCai/Brain-subregional-DNA-methylation-aging). REFERENCES * Shohayeb, B., Diab, M., Ahmed, M. & Ng, D. C. H. Factors that influence adult neurogenesis as
potential therapy. _Transl. Neurodegener._ 7, 4 (2018). Article PubMed PubMed Central Google Scholar * Culig, L., Chu, X. & Bohr, V. A. Neurogenesis in aging and age-related
neurodegenerative diseases. _Ageing Res. Rev._ 78, 101636 (2022). Article CAS PubMed PubMed Central Google Scholar * Dean, D. C. et al. Brain differences in infants at differential
genetic risk for late-onset Alzheimer disease: a cross-sectional imaging study. _Jama Neurol._ 71, 11–22 (2014). Article PubMed PubMed Central Google Scholar * Fu, H., Hardy, J. &
Duff, K. E. Selective vulnerability in neurodegenerative diseases. _Nat. Neurosci._ 21, 1350–1358 (2018). Article CAS PubMed PubMed Central Google Scholar * van Praag, H. et al.
Functional neurogenesis in the adult hippocampus. _Nature_ 415, 1030–1034 (2002). Article ADS PubMed PubMed Central Google Scholar * Kuhn, H. G., Dickinson-Anson, H. & Gage, F. H.
Neurogenesis in the dentate gyrus of the adult rat: age-related decrease of neuronal progenitor proliferation. _J. Neurosci._ 16, 2027–2033 (1996). Article CAS PubMed PubMed Central
Google Scholar * Lois, C. & Alvarez-Buylla, A. Proliferating subventricular zone cells in the adult mammalian forebrain can differentiate into neurons and glia. _Proc. Natl. Acad. Sci.
USA_ 90, 2074–2077 (1993). Article ADS CAS PubMed PubMed Central Google Scholar * Alvarez-Buylla, A. & Lois, C. Neuronal stem cells in the brain of adult vertebrates. _Stem Cells
(Dayt. Ohio)_ 13, 263–272 (1995). Article CAS Google Scholar * Doetsch, F., Caillé, I., Lim, D. A., García-Verdugo, J. M. & Alvarez-Buylla, A. Subventricular zone astrocytes are
neural stem cells in the adult mammalian brain. _Cell_ 97, 703–716 (1999). Article CAS PubMed Google Scholar * Alvarez-Buylla, A. & Garcia-Verdugo, J. M. Neurogenesis in adult
subventricular zone. _J. Neurosci._ 22, 629–634 (2002). Article CAS PubMed Google Scholar * Xiao, Y. Z. et al. Reducing hypothalamic stem cell senescence protects against
aging-associated physiological decline. _Cell Metab._ 31, 534–548.e535 (2020). Article CAS PubMed Google Scholar * Zhang, Y. L. et al. Hypothalamic stem cells control ageing speed partly
through exosomal miRNAs. _Nature_ 548, 52–57 (2017). Article ADS CAS PubMed Google Scholar * Li, J. X., Tang, Y. Z. & Cai, D. S. IKK beta/NF-kappa B disrupts adult hypothalamic
neural stem cells to mediate a neurodegenerative mechanism of dietary obesity and pre-diabetes. _Nat. Cell Biol._ 14, 999–1012 (2012). Article CAS PubMed Google Scholar * Wang, Z., Wu,
W., Kim, M. S. & Cai, D. GnRH pulse frequency and irregularity play a role in male aging. _Nat. Aging_ 1, 904–918 (2021). Article PubMed Google Scholar * Zhang, G. et al. Hypothalamic
programming of systemic ageing involving IKK-beta, NF-kappaB and GnRH. _Nature_ 497, 211–216 (2013). Article ADS CAS PubMed Google Scholar * Tang, Y. & Cai, D. Hypothalamic
inflammation and GnRH in aging development. _Cell Cycle_ 12, 2711–2712 (2013). Article CAS PubMed Google Scholar * Zhang, G. & Cai, D. Circadian intervention of obesity development
via resting-stage feeding manipulation or oxytocin treatment. _Am. J. Physiol. Endocrinol. Metab._ 301, E1004–E1012 (2011). Article CAS PubMed Google Scholar * Zhang, G. et al.
Neuropeptide exocytosis involving synaptotagmin-4 and oxytocin in hypothalamic programming of body weight and energy balance. _Neuron_ 69, 523–535 (2011). Article CAS PubMed PubMed
Central Google Scholar * Horvath, S. Erratum to: DNA methylation age of human tissues and cell types. _Genome Biol._ 16, 96 (2015). Article PubMed PubMed Central Google Scholar *
Hannum, G. et al. Genome-wide methylation profiles reveal quantitative views of human aging rates. _Mol. Cell_ 49, 359–367 (2013). Article CAS PubMed Google Scholar * Jones, M. J.,
Goodman, S. J. & Kobor, M. S. DNA methylation and healthy human aging. _Aging Cell_ 14, 924–932 (2015). Article CAS PubMed Google Scholar * Pal, S. & Tyler, J. K. Epigenetics and
aging. _Sci. Adv._ 2, e1600584 (2016). Article ADS PubMed Google Scholar * Dor, Y. & Cedar, H. Principles of DNA methylation and their implications for biology and medicine. _Lancet
(Lond., Engl.)_ 392, 777–786 (2018). Article CAS Google Scholar * Hou, Y. et al. Ageing as a risk factor for neurodegenerative disease. _Nat. Rev. Neurol._ 15, 565–581 (2019). Article
PubMed Google Scholar * Unnikrishnan, A. et al. The role of DNA methylation in epigenetics of aging. _Pharmacol. Therapeutics_ 195, 172–185 (2019). Article CAS Google Scholar * Zhang,
W., Qu, J., Liu, G. H. & Belmonte, J. C. I. The ageing epigenome and its rejuvenation. _Nat. Rev. Mol. Cell Biol._ 21, 137–150 (2020). Article CAS PubMed Google Scholar * Sikora, E.
et al. Cellular senescence in brain aging. _Front Aging Neurosci._ 13, 646924 (2021). Article CAS PubMed Google Scholar * Rutledge, J., Oh, H. & Wyss-Coray, T. Measuring biological
age using omics data. _Nat. Rev. Genet_ 23, 715–727 (2022). Article CAS PubMed Google Scholar * Seale, K., Horvath, S., Teschendorff, A., Eynon, N. & Voisin, S. Making sense of the
ageing methylome. _Nat. Rev. Genet_ 23, 585–605 (2022). Article CAS PubMed Google Scholar * Wang, K. et al. Epigenetic regulation of aging: implications for interventions of aging and
diseases. _Signal Transduct. Target Ther._ 7, 374 (2022). Article CAS PubMed PubMed Central Google Scholar * He, X., Memczak, S., Qu, J., Belmonte, J. C. I. & Liu, G. H. Single-cell
omics in ageing: a young and growing field. _Nat. Metab._ 2, 293–302 (2020). Article PubMed Google Scholar * Horvath, S. & Raj, K. DNA methylation-based biomarkers and the epigenetic
clock theory of ageing. _Nat. Rev. Genet._ 19, 371–384 (2018). Article CAS PubMed Google Scholar * Yang, J. H. et al. Loss of epigenetic information as a cause of mammalian aging.
_Cell_ https://doi.org/10.1016/j.cell.2022.12.027 (2023). Article PubMed PubMed Central Google Scholar * Masser, D. R. et al. Sexually divergent DNA methylation patterns with hippocampal
aging. _Aging Cell_ 16, 1342–1352 (2017). Article CAS PubMed PubMed Central Google Scholar * Masser, D. R. et al. Bisulfite oligonucleotide-capture sequencing for targeted base- and
strand-specific absolute 5-methylcytosine quantitation. _Age (Dordr.)_ 38, 49 (2016). Article PubMed Google Scholar * Song, J. & Kuan, P. F. A systematic assessment of cell type
deconvolution algorithms for DNA methylation data. _Brief Bioinform._ 23, https://doi.org/10.1093/bib/bbac449 (2022). * Rahmani, E. et al. Correcting for cell-type heterogeneity in DNA
methylation: a comprehensive evaluation. _Nat. Methods_ 14, 218–219 (2017). Article CAS PubMed Google Scholar * Rahmani, E. et al. Cell-type-specific resolution epigenetics without the
need for cell sorting or single-cell biology. _Nat. Commun._ 10, 3417 (2019). Article ADS PubMed PubMed Central Google Scholar * Fong, J. et al. Determining subpopulation methylation
profiles from bisulfite sequencing data of heterogeneous samples using DXM. _Nucleic Acids Res._ 49, e93 (2021). Article CAS PubMed PubMed Central Google Scholar * Jeong, Y. et al.
Systematic evaluation of cell-type deconvolution pipelines for sequencing-based bulk DNA methylomes. _Brief Bioinform._ 23, https://doi.org/10.1093/bib/bbac248 (2022). * Hajdarovic, K. H. et
al. Single-cell analysis of the aging female mouse hypothalamus. _Nat. Aging_ 2, 662–678 (2022). Article CAS PubMed PubMed Central Google Scholar * Yang, Y. T. et al. STAB2: an updated
spatio-temporal cell atlas of the human and mouse brain. _Nucleic Acids Res._ 52, D1033–D1041 (2024). Article PubMed Google Scholar * Tepe, B. et al. Single-cell RNA-Seq of mouse
olfactory bulb reveals cellular heterogeneity and activity-dependent molecular census of adult-born neurons. _Cell Rep._ 25, 2689–2703.e2683 (2018). Article CAS PubMed PubMed Central
Google Scholar * Devasani, K. & Yao, Y. Expression and functions of adenylyl cyclases in the CNS. _Fluids Barriers CNS_ 19, 23 (2022). Article CAS PubMed PubMed Central Google
Scholar * Mons, N., Segu, L., Nogues, X. & Buhot, M. C. Effects of age and spatial learning on adenylyl cyclase mRNA expression in the mouse hippocampus. _Neurobiol. Aging_ 25,
1095–1106 (2004). Article CAS PubMed Google Scholar * Iwamoto, T. et al. Motor dysfunction in type 5 adenylyl cyclase-null mice. _J. Biol. Chem._ 278, 16936–16940 (2003). Article CAS
PubMed Google Scholar * Joeyen-Waldorf, J. et al. Adenylate cyclase 7 is implicated in the biology of depression and modulation of affective neural circuitry. _Biol. Psychiatry_ 71,
627–632 (2012). Article CAS PubMed PubMed Central Google Scholar * Hines, L. M. et al. A sex-specific role of type VII adenylyl cyclase in depression. _J. Neurosci._ 26, 12609–12619
(2006). Article CAS PubMed PubMed Central Google Scholar * Takahashi, J. et al. Oxytocin reverses Aβ-induced impairment of hippocampal synaptic plasticity in mice. _Biochem Biophys. Res
Commun._ 528, 174–178 (2020). Article CAS PubMed Google Scholar * Takahashi, J. et al. Intracerebroventricular administration of oxytocin and intranasal administration of the oxytocin
derivative improve β-amyloid peptide (25-35)-induced memory impairment in mice. _Neuropsychopharmacol. Rep._ 42, 492–501 (2022). Article CAS PubMed Google Scholar * El-Ganainy, S. O. et
al. Intranasal oxytocin attenuates cognitive impairment, β-amyloid burden and tau deposition in female rats with Alzheimer’s disease: interplay of ERK1/2/GSK3β/caspase-3. _Neurochem. Res._
47, 2345–2356 (2022). Article CAS PubMed Google Scholar * Selles, M. C. et al. Oxytocin attenuates microglial activation and restores social and non-social memory in APP/PS1 Alzheimer
model mice. _iScience_ 26, 106545 (2023). Article ADS CAS PubMed Google Scholar * Koulousakis, P. et al. Exogenous oxytocin administration restores memory in female APP/PS1 Mice. _J.
Alzheimers Dis._ 96, 1207–1219 (2023). Article CAS PubMed Google Scholar * Ye, C. et al. Oxytocin nanogels inhibit innate inflammatory response for early intervention in Alzheimer’s
disease. _ACS Appl Mater. Interfaces_ 14, 21822–21835 (2022). Article CAS PubMed Google Scholar * Garrido, M. P., Hernandez, A., Vega, M., Araya, E. & Romero, C. Conventional and new
proposals of GnRH therapy for ovarian, breast, and prostatic cancers. _Front Endocrinol. (Lausanne)_ 14, 1143261 (2023). Article PubMed Google Scholar * Sterling, K., Cao, R. & Song,
W. Gonadotropin releasing hormone (GnRH): a hormone therapy boosts cognition in Down syndrome and dementia. _Signal Transduct. Target Ther._ 8, 49 (2023). Article CAS PubMed Google
Scholar * Manfredi-Lozano, M. et al. GnRH replacement rescues cognition in Down syndrome. _Science_ 377, eabq4515 (2022). Article CAS PubMed Google Scholar * Patel, D., Patel, B. &
Wairkar, S. Intranasal delivery of biotechnology-based therapeutics. _Drug Discov. Today_ 27, 103371 (2022). Article CAS PubMed Google Scholar * Akita, T. et al. Involvement of
trigeminal axons in nose-to-brain delivery of glucagon-like peptide-2 derivative. _J. Control Release_ 351, 573–580 (2022). Article CAS PubMed Google Scholar * Finger, E. et al. Adaptive
crossover designs for assessment of symptomatic treatments targeting behaviour in neurodegenerative disease: a phase 2 clinical trial of intranasal oxytocin for frontotemporal dementia
(FOXY). _Alzheimers Res Ther._ 10, 102 (2018). Article PubMed Google Scholar * Lindenmaier, Z. et al. Examining the effect of chronic intranasal oxytocin administration on the
neuroanatomy and behavior of three autism-related mouse models. _Neuroimage_ 257, 119243 (2022). Article CAS PubMed Google Scholar * Wang, Y. et al. Repeated oxytocin prevents central
sensitization by regulating synaptic plasticity via oxytocin receptor in a chronic migraine mouse model. _J. Headache Pain._ 22, 84 (2021). Article CAS PubMed Google Scholar * Bales, K.
L. et al. Long-term exposure to intranasal oxytocin in a mouse autism model. _Transl. Psychiatry_ 4, e480 (2014). Article CAS PubMed Google Scholar * Huang, H. et al. Chronic and acute
intranasal oxytocin produce divergent social effects in mice. _Neuropsychopharmacology_ 39, 1102–1114 (2014). Article CAS PubMed Google Scholar * Zocher, S., Overall, R. W., Lesche, M.,
Dahl, A. & Kempermann, G. Environmental enrichment preserves a young DNA methylation landscape in the aged mouse hippocampus. _Nat. Commun._ 12, 3892 (2021). Article ADS CAS PubMed
Google Scholar * Alberca, C. D., Papale, L. A., Madrid, A. & Alisch, R. S. Hippocampal and peripheral blood DNA methylation signatures correlate at the gene and pathway level in a mouse
model of autism. _Hum. Mol. Genet._ 32, 3312–3322 (2023). Article CAS PubMed Google Scholar * Hadad, N. et al. Absence of genomic hypomethylation or regulation of cytosine-modifying
enzymes with aging in male and female mice. _Epigenetics Chromatin_ 9, 30 (2016). Article PubMed Google Scholar * Langmead, B. & Salzberg, S. L. Fast gapped-read alignment with Bowtie
2. _Nat. Methods_ 9, 357–359 (2012). Article CAS PubMed Google Scholar * Krueger, F. & Andrews, S. R. Bismark: a flexible aligner and methylation caller for Bisulfite-Seq
applications. _Bioinformatics_ 27, 1571–1572 (2011). Article CAS PubMed Google Scholar * Danecek, P. et al. Twelve years of SAMtools and BCFtools. _Gigascience_ 10,
https://doi.org/10.1093/gigascience/giab008 (2021). * Akalin, A. et al. methylKit: a comprehensive R package for the analysis of genome-wide DNA methylation profiles. _Genome Biol._ 13, R87
(2012). Article PubMed PubMed Central Google Scholar * Quinlan, A. R. & Hall, I. M. BEDTools: a flexible suite of utilities for comparing genomic features. _Bioinformatics_ 26,
841–842 (2010). Article CAS PubMed Google Scholar * Titus, A. J., Gallimore, R. M., Salas, L. A. & Christensen, B. C. Cell-type deconvolution from DNA methylation: a review of recent
applications. _Hum. Mol. Genet._ 26, R216–R224 (2017). Article CAS PubMed PubMed Central Google Scholar * Lee, D., Lee, S. & Kim, S. PRISM: methylation pattern-based,
reference-free inference of subclonal makeup. _Bioinformatics_ 35, i520–i529 (2019). Article CAS PubMed PubMed Central Google Scholar * Barrett, J. E. et al. Quantification of tumour
evolution and heterogeneity via Bayesian epiallele detection. _BMC Bioinf._ 18, 354 (2017). Article Google Scholar * Kuleshov, M. V. et al. Enrichr: a comprehensive gene set enrichment
analysis web server 2016 update. _Nucleic Acids Res._ 44, W90–97, (2016). Article CAS PubMed PubMed Central Google Scholar * Szklarczyk, D. et al. The STRING database in 2021:
customizable protein-protein networks, and functional characterization of user-uploaded gene/measurement sets. _Nucleic Acids Res_ 49, D605–D612 (2021). Article CAS PubMed Google Scholar
* Shannon, P. et al. Cytoscape: a software environment for integrated models of biomolecular interaction networks. _Genome Res._ 13, 2498–2504 (2003). Article CAS PubMed PubMed Central
Google Scholar * Tang, Y., Zuniga-Hertz, J. P., Han, C., Yu, B. & Cai, D. Multifaceted secretion of htNSC-derived hypothalamic islets induces survival and antidiabetic effect via
peripheral implantation in mice. _Elife_ 9, https://doi.org/10.7554/eLife.52580 (2020). * Zhang, Y., Reichel, J. M., Han, C., Zuniga-Hertz, J. P. & Cai, D. Astrocytic process plasticity
and IKKbeta/NF-kappaB in central control of blood glucose, blood pressure, and body weight. _Cell Metab._ 25, 1091–1102.e1094 (2017). Article CAS PubMed PubMed Central Google Scholar *
Ren, J. et al. Intrauterine hyperglycemia exposure results in intergenerational inheritance via DNA methylation reprogramming on F1 PGCs. _Epigenetics Chromatin_ 11, 20 (2018). Article
PubMed PubMed Central Google Scholar Download references ACKNOWLEDGEMENTS We gratefully thank Cai lab members for technical support, thank W. Freeman and Oklahoma Nathan Shock Center on
Aging for providing kind assistance and Core support, and thank CD Genomics for target methylation sequencing. This research was supported through Einstein institutional resources and partly
through Milky Way Research Foundation award and National Institutes of Health R01AG031774 (all supports to D. Cai). AUTHOR INFORMATION Author notes * These authors contributed equally:
Salman Sadullah Usmani, Hyun-Gug Jung. AUTHORS AND AFFILIATIONS * Department of Molecular Pharmacology, Albert Einstein College of Medicine, Bronx, NY, USA Salman Sadullah Usmani, Hyun-Gug
Jung, Qichao Zhang, Min Woo Kim, Yuna Choi, Ahmet Burak Caglayan & Dongsheng Cai Authors * Salman Sadullah Usmani View author publications You can also search for this author inPubMed
Google Scholar * Hyun-Gug Jung View author publications You can also search for this author inPubMed Google Scholar * Qichao Zhang View author publications You can also search for this
author inPubMed Google Scholar * Min Woo Kim View author publications You can also search for this author inPubMed Google Scholar * Yuna Choi View author publications You can also search for
this author inPubMed Google Scholar * Ahmet Burak Caglayan View author publications You can also search for this author inPubMed Google Scholar * Dongsheng Cai View author publications You
can also search for this author inPubMed Google Scholar CONTRIBUTIONS S.S.U. performed all computational analysis on BOCS metadata and target promoter sequencing data, did statistics for all
sequencing data, contributed to data interpretation and provided writing assistance. H.J. performed animal treatment and behavioral study, performed qRT-PCR assays, and contributed to data
interpretation. Q.Z. performed histological and immunostaining experiment and contributed to data interpretation. M.W.K. co-performed animal treatment and behavioral assays. Y.C. contributed
to qRT-PCR primers and target promoter bisulfite sequencing. A.B.C. contributed to animal treatment study. D.C. conceived the hypothesis and ideas, conceptualized and constructed the
project, designed computational and experimental strategies, advised and supervised all experiments, instructed and concluded data analysis, led and concluded data interpretation, and wrote
the paper. All authors approved the paper. CORRESPONDING AUTHOR Correspondence to Dongsheng Cai. ETHICS DECLARATIONS COMPETING INTERESTS D.C. has an invention disclosure with Albert Einstein
College of Medicine (OXT-GnRH approaches for addressing AD or aging). Other authors declare no competing interests. PEER REVIEW PEER REVIEW INFORMATION _Nature Communications_ thanks Isabel
Castanho and the other anonymous reviewer(s) for their contribution to the peer review of this work. A peer review file is available. ADDITIONAL INFORMATION PUBLISHER’S NOTE Springer Nature
remains neutral with regard to jurisdictional claims in published maps and institutional affiliations. SUPPLEMENTARY INFORMATION SUPPLEMENTARY INFORMATION DESCRIPTION OF ADDITIONAL
SUPPLEMENTARY FILES SUPPLEMENTARY DATASET 1 SUPPLEMENTARY DATASET 2 SUPPLEMENTARY DATASET 3 REPORTING SUMMARY SOURCE DATA SOURCE DATA TRANSPARENT PEER REVIEW FILE RIGHTS AND PERMISSIONS OPEN
ACCESS This article is licensed under a Creative Commons Attribution-NonCommercial-NoDerivatives 4.0 International License, which permits any non-commercial use, sharing, distribution and
reproduction in any medium or format, as long as you give appropriate credit to the original author(s) and the source, provide a link to the Creative Commons licence, and indicate if you
modified the licensed material. You do not have permission under this licence to share adapted material derived from this article or parts of it. The images or other third party material in
this article are included in the article’s Creative Commons licence, unless indicated otherwise in a credit line to the material. If material is not included in the article’s Creative
Commons licence and your intended use is not permitted by statutory regulation or exceeds the permitted use, you will need to obtain permission directly from the copyright holder. To view a
copy of this licence, visit http://creativecommons.org/licenses/by-nc-nd/4.0/. Reprints and permissions ABOUT THIS ARTICLE CITE THIS ARTICLE Usmani, S.S., Jung, HG., Zhang, Q. _et al._
Targeting the hypothalamus for modeling age-related DNA methylation and developing OXT-GnRH combinational therapy against Alzheimer’s disease-like pathologies in male mouse model. _Nat
Commun_ 15, 9419 (2024). https://doi.org/10.1038/s41467-024-53507-8 Download citation * Received: 28 July 2023 * Accepted: 09 October 2024 * Published: 31 October 2024 * DOI:
https://doi.org/10.1038/s41467-024-53507-8 SHARE THIS ARTICLE Anyone you share the following link with will be able to read this content: Get shareable link Sorry, a shareable link is not
currently available for this article. Copy to clipboard Provided by the Springer Nature SharedIt content-sharing initiative