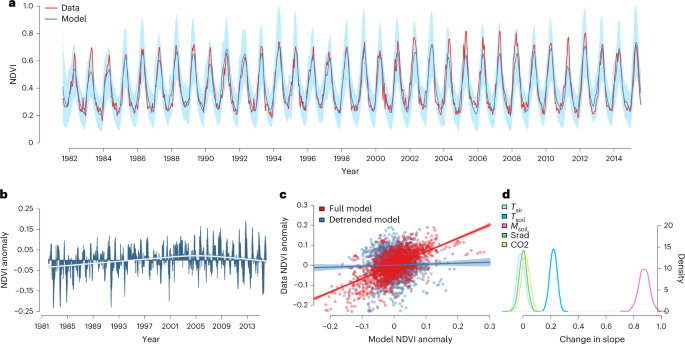
Shifts in vegetation activity of terrestrial ecosystems attributable to climate trends
- Select a language for the TTS:
- UK English Female
- UK English Male
- US English Female
- US English Male
- Australian Female
- Australian Male
- Language selected: (auto detect) - EN
Play all audios:

ABSTRACT Climate change is expected to impact the functioning of the entire Earth system. However, detecting changes in ecosystem dynamics and attributing such change to anthropogenic
climate change has proved difficult. Here we analyse the vegetation dynamics of 100 sites representative of the diversity of terrestrial ecosystem types using remote-sensing data spanning
the past 40 years and a dynamic model of plant growth, forced by climate reanalysis data. We detect a change in vegetation activity for all ecosystem types and find these changes can be
attributed to trends in climate-system parameters. Ecosystems in dry and warm locations responded primarily to changes in soil moisture, whereas ecosystems in cooler locations responded
primarily to changes in temperature. We find that the effects of CO2 fertilization on vegetation are limited, potentially due to masking by other environmental drivers. Observed trend
switching is widespread and dominated by shifts from greening to browning, suggesting many of the ecosystems studied are accumulating less carbon. Our study reveals a clear fingerprint of
climate change in the change exhibited by terrestrial ecosystems over recent decades. SIMILAR CONTENT BEING VIEWED BY OTHERS DISTINCT RESPONSE OF GROSS PRIMARY PRODUCTIVITY IN FIVE
TERRESTRIAL BIOMES TO PRECIPITATION VARIABILITY Article Open access 16 October 2020 THE GLOBAL CARBON SINK POTENTIAL OF TERRESTRIAL VEGETATION CAN BE INCREASED SUBSTANTIALLY BY OPTIMAL LAND
MANAGEMENT Article Open access 18 January 2022 SEASONAL BIOLOGICAL CARRYOVER DOMINATES NORTHERN VEGETATION GROWTH Article Open access 12 February 2021 MAIN Climate change is impacting the
structure and functioning of terrestrial ecosystems1,2,3,4,5,6. Detecting this change and attributing it to climate change is a major research challenge in Earth systems science7. Detection
is difficult because signals in the data can be diluted by the stochasticity inherent in the system, the short length of available time series, errors in the observation process and the
often overriding effect of land-use change. Attribution is difficult because ecosystem dynamics can be nonlinear and asynchronous and the dynamics are co-limited by environmental drivers
that are often highly correlated with one another. For example, biomass increases that are expected as a consequence of enhanced carbon assimilation by elevated atmospheric CO2
concentrations may be constrained by water and nutrient availability8,9,10,11. These difficulties mean that convincing detection and attribution of climate change impacts on ecosystems is
limited to a small number of well-studied systems7. The most convincing evidence to date for attributing changes in ecosystems to climate change comes from the high latitudes where
temperatures are increasing from the lower thermal limits of multiple ecological processes7,12. In such examples, the case for attribution has been constructed from experimental, modelling
and observational lines of evidence derived from multiple studies. Yet it has emerged that even in these high-latitude ecosystems, where there is convincing evidence for change, not all
instances of these ecosystems have changed in the same way13,14,15. Some cases exhibited greening (an increase in vegetation activity), others browning (a decrease in vegetation activity),
and for yet others, the initially reported trends had to be revised as the observation window has expanded in time and space10,14,15,16,17. This suggests that robust detection and
attribution require longer time series and assessments at multiple instances of each of the world’s major ecosystem types. Earth observation satellite programmes provide multi-decade time
series of vegetation activity and allow the monitoring of many sites. Previous studies have used Earth observation data to detect change in vegetation activity across the entire land surface
and identified that vegetation change is to some extent sensitive to climate-system parameters18,19,20,21,22,23. However, such complete analyses of the land surface include pervasive
land-use impacts in the data24, which may confound climate change and land use as drivers of vegetation change. Interpretation is further complicated by the fact that previous studies used
model-based interpretations of the radiometric data collected by satellites such as net primary productivity (NPP18,21,25), gross primary productivity (GPP23,25) and leaf area index (LAI20).
A STATE-SPACE METHOD FOR DETECTION AND ATTRIBUTION Deepening our understanding of how ecosystems respond to climate change requires a robust detection and attribution methodology that
addresses the problems highlighted in the previous paragraphs. In this study, we develop and apply a new method for detecting and attributing climate change impacts on terrestrial ecosystems
that addresses confounding effects of land-use change, biases associated with short time series, limitations of correlative methods and ambiguities with interpreting NPP, GPP and LAI
modelled from satellite-derived data. We detect change using high-quality, long-term remotely sensed normalized difference vegetation index (NDVI) and enhanced vegetation index (EVI) time
series. NDVI and EVI data products are sensitive indicators of leaf area and chlorophyll content, and unlike NPP, GPP and LAI products, they are based purely on the radiometrically and
geometrically corrected measurements made by satellites. The NDVI time series we use spans 1981–2015 and was derived from the Advanced Very High Resolution Radiometer (AVHRR) satellite
programme as part of the Global Inventory Modeling and Mapping Studies (GIMMS) project26,27. The EVI dataset we use spans 2000–2019 and is a product of the Moderate Resolution Imaging
Spectroradiometer (MODIS) programme28. GIMMS NDVI provides a longer record, while MODIS EVI offers the opportunity to use a vegetation index that does not saturate at high chlorophyll
situations and is less sensitive to atmospheric and soil contamination28. To avoid the confounding effects of direct human impacts on vegetation, we carefully selected 100 sites, distributed
across the major ecosystems of the world, where direct land-use impacts are absent (Methods). We considered only grid cells (the GIMMS NDVI grid cells are 1/12° in size, ~9 km) that show no
signs of anthropogenic land transformation in the observation period. We further included only sites where the vegetation is relatively homogeneous within the grid cell. The MODIS EVI data
were resampled to the GIMMS NDVI grid (Methods). Our final sample is stratified to include at least five examples of each of the major ecosystems of the world. These criteria yielded a total
of 100 sites (Extended Data Figs. 2 and 3) covering tropical evergreen forest (RF, _n_ = 16), boreal forest (BF, _n_ = 10), temperate forest (TF, _n_ = 12), savannah (SA, _n_ = 18),
shrubland (SH, _n_ = 16), grassland (GR, _n_ = 14), tundra (TU, _n_ = 9) and Mediterranean-type ecosystems (MT, _n_ = 5). At each site, we attributed the detected vegetation index change to
climate-system parameters (soil temperature, air temperature, soil moisture, solar radiation, atmospheric CO2 concentration) by using a process-based model of plant growth29,30 forced by
weekly climate-system data. The plant growth model allows us to interpret how temporal variation in satellite observations of vegetation indices relate to variation in climate-system
parameters31,32. A state-space modelling framework33 is used to estimate the parameters of the growth model from the vegetation index time series. The state-space analysis assumes that the
remotely sensed NDVI or EVI observations arise from an unobserved underlying dynamic process that is represented by the plant growth model (Methods). The analysis furthermore treats process
and observation uncertainty separately, allowing us to account for how observation uncertainty related to cloud and snow cover15,18,19 structures uncertainty in the analysis. The process
model we use29 simulates a single plant that represents a virtual phytometer exposed to different environmental conditions; it therefore differs from the Dynamic Global Vegetation Models34
used in Earth system model attribution studies20,21,23. The simulations focus on modelling the plant’s carbon and nitrogen assimilation and, in a separate process, how these assimilates
influence growth29. Assimilation and growth processes in the model are co-limited by combinations of air and soil temperature, soil moisture, solar radiation and atmospheric CO230,35
(Methods). The model’s structure thereby represents a hypothesis of how environmental factors co-limit the growth of a phytometer. The first step in our analysis is to use the state-space
approach to estimate the model parameters for each vegetation index time series (Fig. 1a). We then use time-series decomposition methods to identify anomalies in the vegetation indices (Fig.
1b) and to generate detrended time series of the climate-system forcing data (air and soil temperature, soil moisture, solar radiation, atmospheric CO2; Methods). The fitted model is then
used with the full and detrended climate data to predict the observed anomalies in the vegetation indices. To attribute vegetation trends to trends in the climate-system data, we use the
slope of the regression (with intercept 0) between observed and modelled anomalies (Fig. 1c). Attribution is diagnosed if the slope of this regression from model runs forced by the full
climate data (red line in Fig. 1c) is positive and clearly higher (no overlap in the credible intervals of the slopes; Extended Data Figs. 9a and 10a) than the regression slope from model
runs forced by climate data with trends removed (blue line in Fig. 1c). That is, attribution is diagnosed if trends in climate-system variables statistically enhance the ability to predict
the observed anomalies in the vegetation indices. For cases where attribution is supported, we estimate the relative importance of trends in each climate-system variable in explaining the
anomalies. This was achieved by assessing the change in regression slope induced by separately removing trends in each climate-system variable (Fig. 1d). The same analyses were repeated
using the EVI time series (Extended Data Figs. 4, 9b and 10b). WIDESPREAD SHIFTS FROM GREENING TO BROWNING The anomaly trends observed in the satellite vegetation indices revealed
qualitatively different patterns when interpreted using trend analyses. To quantify these different patterns, we used both quadratic polynomials and a bent-cable piecewise linear regression.
Both approaches allow one to test whether there is a long-term trend and whether there has been a shift in this long-term trend. We classified the response trends as being (1) hat shaped
where trends in the vegetation indices increased at first (‘greening’) but later declined (‘browning’), (2) cup shaped where trends decreased initially and later increased or (3) linear
where no change in the sign of the trend was detected. The majority of sites illustrated hat-shaped trends, fewer sites illustrated cup-shaped trends and only a minority of sites illustrated
linear trends (Fig. 2 and Extended Data Fig. 5). In the analyses that used the shorter EVI time series, the cup-shaped trends were more common than in the analyses that used NDVI data
(Extended Data Fig. 5). In the NDVI data, overall increasing and decreasing vegetation greenness trends were almost equally distributed, while in the EVI data, more sites revealed increasing
trends. Analysing these trends for the common (15 yr) time window for which both data products are available revealed similar distributions although the EVI data revealed more increasing
trends than the NDVI data (Extended Data Fig. 6). Despite uncertainty associated with vegetation index datasets and time-series window (1982–2015 or 2000–2019), both analyses agreed that
nonlinearity dominated and that greening trends switching to browning trends was the most common pattern in the data. The dominance of shifts from greening to browning trends in vegetation
activity detected here contradicts the predominance of positive trends previously reported20 but is supported by studies that have shown negative NDVI and EVI trends36. Hat-shaped trend
switching has been reported in high-latitude ecosystems where initial greening trends attributed to warming have now been replaced by browning trends attributed to seasonal water
deficits10,37. Positive to negative trend switching has also been reported in Amazonian forests, and predictions are that African tropical forests will follow suit38. Switching of the kind
observed here is in all likelihood driven by interactions between climate-system drivers and internal ecosystem dynamics39, which makes the prediction of future trends highly context
dependent. ATTRIBUTION OF VEGETATION ACTIVITY CHANGE In 80 (NDVI data) or 75 (EVI data) of the 100 study sites, the model was able to explain variation in the observed trends in the
vegetation indices and attribute this to trends in the climate-forcing data (Extended Data Fig. 9). This demonstrates a clear fingerprint of climate change on the activity of the world’s
ecosystems. A classification of sites according to the relative importance of climate-system variables driving vegetation anomalies yielded two groups that were qualitatively consistent
between the NDVI and EVI analyses (Fig. 3 and Extended Data Fig. 7). In the first group, moisture was the dominant factor explaining anomalies in the vegetation data; this group consisted
primarily of grassland, savannah and shrubland sites. In the second group, vegetation index anomalies were explained by a combination of factors, with temperature often playing a central
role; this group consisted primarily of boreal forest, temperate forest and tundra sites. It is notable that trends in CO2 and solar radiation seldom had a dominant influence on the model’s
ability to predict the observed anomalies in the vegetation indices (Fig. 3 and Extended Data Fig. 7). The lack of evidence for dominating CO2 effects contradicts a previous study of LAI20
but is consistent with analyses that conclude that CO2 enrichment effects on vegetation biomass are conditional on nutrient and water supply8,11, mycorrhiza9 and successional stage25 and
with studies that suggest that CO2 enrichment effects on GPP and biomass accumulation may be weakening as global warming progresses10,18,23,38. Which environmental factors dominated the
anomaly response was clearly structured in climate space. A discriminant analysis revealed that the major groups in Fig. 3 and Extended Data Fig. 7 can be separated using mean annual
temperature and mean annual soil moisture (Fig. 4a and Extended Data Fig. 8a). Sites where anomalies in vegetation indices were attributed to anomalies in soil moisture were located in warm
and dry locations. Sites where a mixture of factors, often dominated by temperature, explained anomalies in vegetation indices were centred in cooler and moister locations. The 20 (NDVI
data) and 25 (EVI data) sites where anomalies in the forcing data could not explain vegetation index anomalies were centred in warmer and moister locations (sites labelled 0 in Fig. 4 and
Extended Data Fig. 8); indeed, the majority of tropical rainforest sites were in this group. In general, these findings are consistent with fundamental ecological premises that warm and dry
ecosystems are water limited and that cooler ecosystems are more likely to be temperature limited. What is perhaps unexpected was that changes in vegetation indices in ecosystems in warm and
moist locations could not be attributed to changes in a third factor, such as atmospheric CO2. However, we would also expect our method to have lower statistical power in environments with
low seasonal and interannual variation in vegetation activity simply because these situations yield vegetation index time series with lower information content. This is particularly true for
the NDVI analysis since NDVI is known to saturate at the LAIs typical of tropical forests. Furthermore, moist tropical regions are often phosphorus limited40, which may constrain the
ability of such ecosystems to respond to elevated CO2 (ref. 9). The shape of the vegetation response and whether the response is increasing or decreasing over time (as summarized in Fig. 2)
were not clearly structured in environmental space (Fig. 4 and Extended Data Fig. 8). The complexity in these patterns is consistent with modelling studies that predicted that transitions in
ecosystem behaviour will occur at different points in time for different geographic locations41 and with remote-sensing and field studies that have reported both increasing and decreasing
vegetation responses within regions and observation time windows14,16,17,38. CO-LIMITING DRIVERS OF CHANGE Overall, we have detected change in indices of vegetation activity in all
terrestrial ecosystem types, and in the majority of cases studied (80% for the NDVI analysis and 75% for the EVI analysis) we were able to attribute observed changes in vegetation indices to
trends in climate-system variables. Temperature and moisture trends explained most of the variation, whereas increasing CO2 and changes in surface solar radiation were seldom important. The
apparent insensitivity of vegetation to CO2 is due to co-limitation processes in the growth model that allow the model to accommodate no increases in plant biomass despite increasing
potential carbon assimilation caused by increasing atmospheric CO2. For example, in the model, elevated CO2 allows for higher potential rates of carbon assimilation, but decreases in soil
moisture can prevent this potential carbon assimilation from being realized. In addition, the model can represent that higher carbon assimilation will not lead to enhanced growth as long as
one of nitrogen, temperature and soil moisture is limiting. Analogous co-limitation pathways allow the model to simulate situations in high latitudes where increasing temperatures promote
growth, yet increasing water deficits retard growth12,42. The dominance of hat-shaped responses in the vegetation indices analysed here suggests that terrestrial ecosystems, which have been
a valuable carbon sink in recent decades6, may sequester less carbon in coming decades10,18,23,38. However, NDVI and EVI provide an incomplete picture of ecosystem carbon sequestration43.
This is because NDVI and EVI, although sensitive to changes in the fraction of photosynthetically active radiation that is absorbed by leaves, are less sensitive to how efficiently this
fraction is translated into assimilated carbon (light use efficiency (LUE))43. It follows that both the NDVI and EVI data may poorly represent changes in LUE, which is known to increase with
elevated CO244. Plants that invest the spoils of increased LUE in root growth, defence, mutualisms or reproduction may therefore go undetected in our analysis. This incomplete picture of
vegetation carbon dynamics could be improved by considering additional data sources. For example, satellite-based observations of chlorophyll fluorescence provide new opportunities for
estimating LUE43,45, allowing the separation of carbon assimilation from leaf area dynamics46. The state-space model used here could be expanded to simultaneously model a fraction of
absorbed photosynthetically active radiation index (EVI or NDVI) from the process model’s biomass and a LUE index (for example, solar-induced fluorescence) from the model’s carbon
assimilation. This would further constrain the model’s parameter estimates and allow attribution of both biomass and GPP trends to climate-system variables. Dealing with climate change is at
minimum a three-step process: detection, attribution and adaptation/mitigation. We have illustrated a generally applicable method for detection and attribution of climate change impacts on
the world’s major terrestrial ecosystems. In this study, we detected that many ecosystems are on hat-shaped trajectories, where initial greening trends have switched to browning trends,
suggesting that the majority of the ecosystems studied here may be accumulating less leaf biomass and potentially less carbon than they did previously. The detection and attribution
methodology may help adaptation and mitigation strategists better understand the trajectories that ecosystems are on. In particular, our analyses revealed geographic coherence as to which
environmental drivers are driving change, which may help the design of ecosystem restoration programmes. METHODS PLANT GROWTH MODEL WITHOUT ENVIRONMENTAL FORCING The model without
environmental forcing closely follows the original description of the Thornley transport resistance (TTR) model29. A summary of the model parameters is provided in Supplementary Table 2. The
shoot and root mass pools (MS and MR, in kg structural dry matter) change as a function of growth and loss (equations (1) and (2)). The litter (_k_L) and maintenance respiration (_r_) loss
rates (in kg kg−1 d−1) are treated as constants. In the original model description29 _r_ = 0. The parameter _K__M_ (units kg) describes how loss varies with mass (MS or MR). Growth (_G_s and
_G_r, in kg d−1) varies as a function of the carbon and nitrogen concentrations (equations (3) and (4)). CS, CR, NS and NR are the amounts (kg) of carbon and nitrogen in the roots and
shoots. These assumptions yield the following equations for shoot and root dry matter,
$${\mathrm{MS}}[t+1]={\mathrm{MS}}[t]+{G}_{{\mathrm{S}}}[t]-\frac{({k}_{{\mathrm{L}}}+r){\mathrm{MS}}[t]}{1+\frac{{K}_{{{M}}}}{{\mathrm{MS}}[t]}},$$ (1)
$${\mathrm{MR}}[t+1]={\mathrm{MR}}[t]+{G}_{{\mathrm{R}}}[t]-\frac{({k}_{{\mathrm{L}}}+r){\mathrm{MR}}[t]}{1+\frac{{K}_{{{M}}}}{{\mathrm{MR}}[t]}},$$ (2) where _G_S and _G_R are
$${G}_{{\mathrm{S}}}=g\frac{{\mathrm{CS}}\times {\mathrm{NS}}}{{\mathrm{MS}}},$$ (3) $${G}_{{\mathrm{R}}}=g\frac{{\mathrm{CR}}\times {\mathrm{NR}}}{{\mathrm{MR}}},$$ (4) and _g_ is the
growth coefficient (in kg kg−1 d−1). Carbon uptake _U_C is determined by the net photosynthetic rate (_a_, in kg kg−1 d−1) and the shoot mass (equation (5)). Similarly, nitrogen uptake
(_U_N) is determined by the nitrogen uptake rate (_b_, in kg kg−1 d−1) and the root mass. The parameter _K_A (units kg) forces both photosynthesis and nitrogen uptake to be asymptotic with
mass. The second terms in the denominators of equations (5) and (6) model product inhibitions of carbon and nitrogen uptake, respectively; that is, the parameters _J_C and _J_N (in kg kg−1)
mimic the inhibition of source activity when substrate concentrations are high,
$${U}_{{\mathrm{C}}}=\frac{a{\mathrm{MS}}}{\left(1+\frac{{\mathrm{MS}}}{{K}_{{\mathrm{A}}}}\right)\left(1+\frac{{\mathrm{CS}}}{{\mathrm{MS}}\times {J}_{{\mathrm{C}}}}\right)},$$ (5)
$${U}_{{\mathrm{N}}}=\frac{b{\mathrm{MR}}}{\left(1+\frac{{\mathrm{MR}}}{{K}_{{\mathrm{A}}}}\right)\left(1+\frac{{\mathrm{NR}}}{{\mathrm{MR}}\times {J}_{{\mathrm{N}}}}\right)}.$$ (6) The
substrate transport fluxes of C and N (_τ_C and _τ_N, in kg d−1) between roots and shoots are determined by the concentration gradients between root and shoot and by the resistances. In the
original model description29, these resistances are defined flexibly, but we simplify and assume that they scale linearly with plant mass, $${\tau }_{{\mathrm{C}}}=\frac{{\mathrm{MS}}\times
{\mathrm{MR}}}{{\mathrm{MS}}+{\mathrm{MR}}}\left(\frac{{\mathrm{CS}}}{{\mathrm{MS}}}-\frac{{\mathrm{CR}}}{{\mathrm{MR}}}\right)$$ (7) $${\tau }_{{\mathrm{N}}}=\frac{{\mathrm{MS}}\times
{\mathrm{MR}}}{{\mathrm{MS}}+{\mathrm{MR}}}\left(\frac{{\mathrm{NR}}}{{\mathrm{MR}}}-\frac{{\mathrm{NS}}}{{\mathrm{MS}}}\right)$$ (8) The changes in mass of carbon and nitrogen in the roots
and shoots are then $${\mathrm{CS}}[t+1]={\mathrm{CS}}[t]+{U}_{{\mathrm{C}}}[t]-{f}_{{\mathrm{C}}}{G}_{{\mathrm{s}}}[t]-{\tau }_{{\mathrm{C}}}[t]$$ (9)
$${\mathrm{CR}}[t+1]={\mathrm{CR}}[t]+{\tau }_{{\mathrm{C}}}[t]-{f}_{{\mathrm{C}}}{G}_{{\mathrm{r}}}[t]$$ (10) $${\mathrm{NS}}[t+1]={\mathrm{NS}}[t]+{\tau
}_{{\mathrm{N}}}[t]-{f}_{{\mathrm{N}}}{G}_{{\mathrm{s}}}[t]$$ (11) $${\mathrm{NR}}[t+1]={\mathrm{NR}}[t]+{U}_{{\mathrm{N}}}[t]-{f}_{{\mathrm{N}}}{G}_{{\mathrm{r}}}[t]-{\tau
}_{{\mathrm{N}}}[t]$$ (12) where _f_C and _f_N (in kg kg−1) are the fractions of structural carbon and nitrogen in dry matter. ADDING ENVIRONMENTAL FORCING TO THE PLANT GROWTH MODEL In this
section, we describe how the net photosynthetic rate (_a_), the nitrogen uptake rate (_b_), the growth rate (_g_) and the respiration rate (_r_) are influenced by environmental-forcing
factors. These environmental-forcing effects are described in equations (13)–(17) and summarized graphically in Extended Data Fig. 1. All other model parameters are treated as constants.
Previous work that implemented the TTR model as a species distribution model30 is used as a starting point for adding environmental forcing. As in this previous work30, we assume that
parameters _a_, _b_ and _g_ are co-limited by environmental factors in a manner analogous to Liebig’s law of the minimum, which is a crude but pragmatic abstraction. The implementation here
differs in some details. Unlike previous work30, we use the Farquhar model of photosynthesis47,48 to represent how solar radiation, atmospheric CO2 concentration and air temperature co-limit
photosynthesis35. We assume that the Farquhar model parameters are universal and that all vegetation in our study uses the C3 photosynthetic pathway. The Farquhar model photosynthetic rates
are rescaled to [0,_a_max] to yield _a_fqr. The effects of soil moisture (_M_soil) on photosynthesis are represented as an increasing step function \({{{{S}}}}(M_{\mathrm{soil}},{\beta
}_{1},{\beta }_{2})=\max \left\{\min \left(\frac{M_{\mathrm{soil}}-{\beta }_{1}}{{\beta }_{2}-{\beta }_{1}},1\right),0\right\}\). This allows us to redefine _a_ as, $$a={a}_{{\mathrm{fqr}}}\
{{{{S}}}}(M_{\mathrm{soil}},{\beta }_{1},{\beta }_{2})$$ (13) The processes influencing nitrogen availability are complex, and global data products on plant available nitrogen are
uncertain. We therefore assume that nitrogen uptake will vary with soil temperature and soil moisture. That is, the nitrogen uptake rate _b_ is assumed to have a maximum rate (_b_max) that
is co-limited by soil temperature _T_soil and soil moisture _M_soil, $$b={b}_{{\mathrm{max}}}\ {{{{S}}}}({T}_{soil},{\beta }_{3},{\beta }_{4})\ {{{{Z}}}}(M_{\mathrm{soil}},{\beta
}_{5},{\beta }_{6},{\beta }_{7},{\beta }_{8}).$$ (14) In equation (14), we have assumed that the nitrogen uptake rate is a simple increasing and saturating function of temperature. We have
also assumed that the nitrogen uptake rate is a trapezoidal function of soil moisture with low uptake rates in dry soils, higher uptake rates at intermediate moisture levels and lower rates
once soils are so moist as to be waterlogged. The trapezoidal function is \({{{{Z}}}}(M_{\mathrm{soil}},{\beta }_{5},{\beta }_{6},{\beta }_{7},{\beta }_{8})=\max \left\{\min
\left(\frac{M_{\mathrm{soil}}-{{{{{\beta }}}}}_{5}}{{{{{{\beta }}}}}_{6}-{{{{{\beta }}}}}_{5}},1,\frac{{{{{{\beta }}}}}_{8}-M_{\mathrm{soil}}}{{\beta }_{8}-{\beta }_{7}}\right),0\right\}\).
The previous sections describe how the assimilation of carbon and nitrogen by a plant are influenced by environmental factors. The TTR model describes how these assimilate concentrations
influence growth (equations (3) and (4)). In our implementation, we additionally allow the growth rate to be co-limited by temperature (soil temperature, _T_soil) and soil moisture
(_M_soil), $$g={g}_{{\mathrm{max}}}\ {{{{Z}}}}({T}_{{\mathrm{soil}}},{\beta }_{9},{\beta }_{10},{\beta }_{11},{\beta }_{12})\ {{{{S}}}}(M_{\mathrm{soil}},{\beta }_{13},{\beta }_{14}).$$ (15)
We use _T_soil since we assume that growth is more closely linked to soil temperature, which varies slower than air temperature. The respiration rate (_r_, equations (1) and (2)) increases
as a function of air temperature (_T_air) to a maximum _r_max, $$r={r}_{{\mathrm{max}}}{{{{S}}}}({T}_{{\mathrm{air}}},{\beta }_{15},{\beta }_{16}).$$ (16) The parameter _r_ is best
interpreted as a maintenance respiration. Growth respiration is not explicitly considered; it is implicitly incorporated in the growth rate parameter (_g_, equation (15)), and any
temperature dependence in growth respiration is therefore assumed to be accommodated by equation (15). Fire can reduce the structural shoot mass MS as follows,
$${\mathrm{MS}}[t+1]={\mathrm{MS}}[t](1-{{{{S}}}}(F,{\beta }_{17},{\beta }_{18})).$$ (17) where _F_ is an indicator of fire severity at a point in time (for example, burnt area) and the
function _S_(_F_, _β_17, _β_18) allows MS to decrease when the fire severity indicator _F_ is high. If _F_ = 0, this process plays no role. This fire impact equation was used in preliminary
analyses, but the data on fire activity did not provide sufficient information to estimate _β_17 and _β_18; we therefore excluded this process from the final analyses. We further estimate
two additional _β_ parameters (_β__a_ and _β__b_) so that each site can have unique maximum carbon and nitrogen uptake rates. Specifically, we redefine _a_ as \({a}^{{\prime} }={\beta }_{a}\
a\) and _b_ as \({b}^{{\prime} }={\beta }_{b}\ b\). DATA SOURCES AND PREPARATION To describe vegetation activity, we use the GIMMS 3g v.1 NDVI data26,27 and the MODIS EVI28 data. The GIMMS
data product has been derived from the AVHRR satellite programme and controls for atmospheric contamination, calibration loss, orbital drift and volcanic eruptions26,27. The data provide 24
NDVI raster grids for each year, starting in July 1981 and ending in December 2015. The spatial resolution is 1/12° (~9 × 9 km). The EVI data used are from the MODIS programme’s Terra
satellite; it is a 1 km data product provided at a 16-day interval. We use data from the start of the record (February 2000) to December 2019. The MODIS data product (MOD13A2) uses a
temporal compositing algorithm to produce an estimate every 16 days that filters out atmospheric contamination. The EVI is designed to reduce the effects of atmospheric, bare-ground and
surface water on the vegetation index28. For environmental forcing, we use the ERA5-Land data31,32 (European Centre for Medium-Range Weather Forecasts Reanalysis v. 5; hereafter, ERA5). The
ERA5 products are global reanalysis products based on hourly estimates of atmospheric variables and extend from present back to 1979. The data products are supplied at a variety of spatial
and temporal resolutions. We used the monthly averages from 1981 to 2019 at a 0.1° spatial resolution (~11 km). The ERA5 data provide air temperature (2 m surface air temperature), soil
temperature (0–7 cm soil depth), surface solar radiation and volumetric soil water (0–7 cm soil depth). Fire data were taken from the European Space Agency Fire Disturbance Climate Change
Initiative’s AVHRR Long-Term Data Record Grid v.1.0 product49. This product provides gridded (0.25° resolution) data of monthly global (from 1982 to 2017) burned area derived from the AVHRR
satellite programme. As mentioned, the fire data did not enrich our analysis, and the analyses we present here therefore exclude further consideration of the fire data. All data were
resampled to the GIMMS grid. The mean pixel EVI was then calculated for each GIMMS cell for each time point in the MODIS EVI data. We used linear interpolation on the NDVI, EVI and ERA5
environmental-forcing data to estimate each variable on a weekly time step. This served to set the time step of the TTR difference equations to one week and to synchronize the different time
series. SITE SELECTION The GIMMS grid cells define the spatial resolution of our sample points. GIMMS grid cells are large (1/12°, ~9 km), meaning that most grid cells contain multiple
land-cover types. We focused on wilderness landscapes, and our aim was to find multiple grid cells for the major ecosystems of the world. We used the following classification of ecosystem
types to guide the stratification of our grid-cell selection: tropical evergreen forest (RF), boreal forest (BF), temperate evergreen and temperate deciduous forest (TF), savannah (SA),
shrubland (SH), grassland (GR), tundra (TU) and Mediterranean-type ecosystems (MT). We used the following criteria to select grid cells. (1) Selected grid cells should contain relatively
homogeneous vegetation. Small-scale heterogeneity was allowed (for example, catenas, drainage lines, peatlands) as long as many of these elements are repeated in the scene (for example,
rolling hills were accepted, but elevation gradients were rejected). (2) Sites should have no signs of transformative human activity (for example, tree harvesting, crop cultivation, paved
surfaces). We used the Time Tool in Google Earth Pro, which provides annual satellite imagery of the Earth from 1984 onwards, to ensure that no such activity occurred during the observation
period (note that the GIMMS record starts in July 1981; however, it is likely that evidence of transformative activity between July 1981 and 1984 would be visible in 1984). Grid cells with
extensive livestock holding on non-improved pasture were included. In some cases, a small agricultural field or pasture was present, and such grid cells were used as long as the field or
pasture was small and remained constant in size. (3) Grid cells should not include large water bodies, but small drainage lines or ponds were accepted as long as they did not violate the
first criterion. (4) Grid cells should be independent (neighbouring grid cells were not selected) and cover the major ecosystems of the world. Using these criteria, we were able to include
100 sites in the study (Extended Data Figs. 2 and 3 and Supplementary Table 4). STATE-SPACE MODEL We used a Bayesian state-space approach. Conceptually, the analysis takes the form,
$$M[t]=f(M[t-1],{\boldsymbol{\beta}},{\boldsymbol{\theta}}_{t-1},{\epsilon }_{t-1})$$ (18) $${\mathrm{VI}}[t]=m\ M[t]+\eta .$$ (19) Here _M_[_t_] is the plant growth model’s prediction of
biomass (_M_ = MS + MR) at time _t_, and _ϵ__t_−1 is the process error associated with the state variables. In the model, each underlying state variable (MS, MR, CS, CR, NS and NR) has an
associated process error term. The function _f_(_M_[_t_ − 1], _Β_, _Θ__t_−1, _ϵ__t_−1) summarizes that the development of _M_ is influenced by the state variables MS, MR, CS, CR, NS and NR,
the environmental-forcing data _Θ__t_−1 and the _Β_ parameters. The observation equation (equation (19)) uses the parameter _m_ to link the VI (vegetation index, either NDVI or EVI)
observations to the modelled state _M_. The parameter _η_ is the observation error. Equation (19) assumes that there is a linear relationship between modelled biomass (_M_) and VI, which is
a simplification of reality50,51,52. The observation error _η_ is structured by our simplification of the data products quality scores (coded _Q_ = 0, 1, 2, with 0 being good and 2 being
poor; Supplementary Table 3) to allow the error to increase with each level of the quality score. Specifically, we define _η_ = _e_0 + _e_1 × _Q_. The model was formulated using the R
package LaplacesDemon53. All _β_ parameters are given vague uniform priors. The parameter _m_ is given a vague normal prior (truncated to be >0). The process error terms are modelled
using normal distributions, and the variances of the error terms are given vague half-Cauchy priors. The _e__x_ parameters are given vague normal priors. The model also requires the
parameterization of _M_[0], the initial vegetation biomass; _M_[0] is given a vague uniform prior. We used the twalk Markov chain Monte Carlo (MCMC) algorithm as implemented in
LaplacesDemon53 and its default control parameters to estimate the posterior distributions of the model parameters. We further fitted the model using DEoptim54,55, which is a robust genetic
algorithm that is known to perform stably on high-dimensional and multi-modal problems56, to verify that the MCMC algorithm had not missed important regions of the parameter space. The
models estimated with MCMC had significantly lower log root-mean-square error than models estimated with DEoptim (paired _t_-test NDVI analysis: _t_ = –2.9806, degrees of freedom (d.f.) =
99, _P_ = 0.00362; EVI analysis: _t_ = –4.6229, d.f. = 99, _P_ = 1.144 × 10–5), suggesting that the MCMC algorithm performed well compared with the genetic algorithm. ANOMALY EXTRACTION AND
TREND ESTIMATION We use the ‘seasonal and trend decomposition using Loess’ (STL57) as implemented in the R58 base function stl. STL extracts the seasonal component _s_ of a time series using
Loess smoothing. What remains after seasonal extraction (the anomaly or remainder, _r_) is the sum of any long-term trend and stochastic variation. We estimate the trend in two ways. First,
we estimate the trend by fitting a quadratic polynomial (_r_ = _a_ + _b__x_ + _c__x_2) to the remainder (_r_ is the remainder, _x_ is time and _a_, _b_ and _c_ are regression coefficients).
The use of polynomials allows the data to specify whether a trend exists, whether the trend is linear, cup or hat shaped and whether the overall trend is increasing or decreasing. As a
second method, we estimate the trend by fitting a bent-cable regression to the remainder. Bent-cable regression is a type of piecewise linear regression for estimating the point of
transition between two linear phases in a time series59,60. The model takes the form _r_ = _b_0 + _b_1_x_ + _b_2 _q_(_x_, _τ_, _γ_)60. Here _r_ is the remainder, _x_ is time, _b_0 is the
initial intercept, _b_1 is the slope in phase 1, the slope in phase 2 is _b_2 − _b_1 and _q_ is a function that defines the change point: \(q(x,\tau ,\gamma )=\frac{{(x-\tau +\gamma
)}^{2}}{4\gamma }I(\tau -\gamma < \tau +\gamma )+(x-\tau )I(x > \tau +\gamma )\); _τ_ represents the location of the change point and _γ_ the span of the bent cable that joins the two
linear phases; _I_(_A_) is an indicator function that returns 1 if _A_ is true and 0 if _A_ is false. The bent-cable model allows the data to specify whether a trend exists and whether there
has been a switch in the trend, thereby allowing the identification of whether the trend is linear, cup or hat shaped and whether the overall trend is increasing or decreasing. Both the
polynomial and bent-cable models were estimated using LaplacesDemon’s53 Adaptive Metropolis MCMC algorithm and vague priors, although for the bent-cable model we constrained _τ_ to be in the
middle 70% of the time series and _γ_ to be at most two years. The STL extraction of the seasonal components in the air temperature, soil temperature, soil moisture and solar radiation data
(there is no stochasticity or seasonal trend in the CO2 data we used) allows us to simulate detrended time series _d_ of these forcing variables as \(d=\bar{y}+s+{{{{N}}}}(\mu ,\sigma )\)
where _N_(_μ_, _σ_) is a normally distributed random variable with mean and standard deviation estimated from the remainder _r_ (we verified that _r_ was well described by the normal
distribution), \(\bar{y}\) is the mean of the data over the time series and _s_ is the seasonal component extracted by STL. For CO2, the detrended time series is simply the average CO2 over
the time series. DATA AVAILABILITY The NDVI data used in this study are from the GIMMS 3g v.1 NDVI data product26,27, downloaded from https://ecocast.arc.nasa.gov/data/pub/gimms/3g.v1/ on 8
January 2019. The EVI data are from the MODIS Vegetation Indices data product28, downloaded from https://lpdaac.usgs.gov/products/mod13a2v006/ on 1 April 2020. The climate-system data were
from the ERA5-Land monthly averaged data from 1950 to present32 downloaded from the Climate Data Store (https://doi.org/10.24381/cds.68d2bb3) on 3 July 2020. Annual historical atmospheric
CO2 concentrations were taken from ISIMIP, downloaded 3 June 2020. In preliminary analyses we used fire data from the ESA Fire Disturbance Climate Change Initiative’s AVHRR LTDR Grid v.1.0
product49 from https://doi.org/10.5285/4f377defc2454db9b2a6d032abfd0cbd downloaded on 2 July 2020. CODE AVAILABILITY A C version of the TTR-SDM growth model described in Methods that can be
called from R is available at https://github.com/pfloek-bt/TTRcodeAttribution. CHANGE HISTORY * _ 09 MAY 2023 A Correction to this paper has been published:
https://doi.org/10.1038/s41561-023-01196-1 _ REFERENCES * Cramer, W. et al. Global response of terrestrial ecosystem structure and function to CO2 and climate change: results from six
dynamic global vegetation models. _Glob. Change Biol._ 7, 357–373 (2001). Article Google Scholar * Parmesan, C. & Yohe, G. A globally coherent fingerprint of climate change impacts
across natural systems. _Nature_ 421, 37–42 (2003). Article Google Scholar * Menzel, A. et al. European phenological response to climate change matches the warming pattern. _Glob. Change
Biol._ 12, 1969–1976 (2006). Article Google Scholar * Nolan, C. et al. Past and future global transformation of terrestrial ecosystems under climate change. _Science_ 361, 920–923 (2018).
Article Google Scholar * Zhang, T., Niinemets, U., Sheffield, J. & Lichstein, J. W. Shifts in tree functional composition amplify the response of forest biomass to climate. _Nature_
556, 99–102 (2018). Article Google Scholar * Bonan, G. B. & Doney, S. C. Climate, ecosystems, and planetary futures: the challenge to predict life in Earth system models. _Science_
359, eaam8328 (2018). Article Google Scholar * Settele, J. et al. in _Climate Change 2014: Impacts, Adaptation, and Vulnerability_ (eds Fields, C. B. et al.) Ch. 4 (Cambridge Univ. Press,
2014). * Reich, P. B., Hobbie, S. E. & Lee, T. D. Plant growth enhancement by elevated CO2 eliminated by joint water and nitrogen limitation. _Nat. Geosci._ 7, 920–924 (2014). Article
Google Scholar * Terrer, C., Vicca, S., Hungate, B. A., Phillips, R. P. & Prentice, I. C. Mycorrhizal association as a primary control of the CO2 fertilization effect. _Science_ 353,
72–74 (2016). Article Google Scholar * Peñuelas, J. et al. Shifting from a fertilization-dominated to a warming-dominated period. _Nat. Ecol. Evol._ 1, 1438–1445 (2017). Article Google
Scholar * Hovenden, M. J. et al. Globally consistent influences of seasonal precipitation limit grassland biomass response to elevated CO2. _Nat. Plants_ 5, 167–173 (2019). Article Google
Scholar * Bjorkman, A. D. et al. Plant functional trait change across a warming tundra biome. _Nature_ 562, 57–62 (2018). Article Google Scholar * McManus, K. M. et al. Satellite-based
evidence for shrub and graminoid tundra expansion in northern Quebec from 1986 to 2010. _Glob. Change Biol._ 18, 2313–2323 (2012). Article Google Scholar * Pan, N. et al. Increasing global
vegetation browning hidden in overall vegetation greening: insights from time-varying trends. _Remote Sens. Environ._ 214, 59–72 (2018). Article Google Scholar * Myers-Smith, I. H. et al.
Complexity revealed in the greening of the Arctic. _Nat. Clim. Change_ 10, 106–117 (2020). Article Google Scholar * de Jong, R., Verbesselt, J., Schaepman, M. E. & de Bruin, S. Trend
changes in global greening and browning: contribution of short-term trends to longer-term change. _Glob. Change Biol._ 18, 642–655 (2012). Article Google Scholar * Sulla-Menashe, D.,
Woodcock, C. E. & Friedl, M. A. Canadian boreal forest greening and browning trends: an analysis of biogeographic patterns and the relative roles of disturbance versus climate drivers.
_Environ. Res. Lett._ 13, 014007 (2018). Article Google Scholar * Zhao, M. & Running, S. W. Drought-induced reduction in global terrestrial net primary production from 2000 through
2009. _Science_ 329, 940–943 (2010). Article Google Scholar * Seddon, A. W. R., Macias-Fauria, M., Long, P. R., Benz, D. & Willis, K. J. Sensitivity of global terrestrial ecosystems to
climate variability. _Nature_ 531, 229–232 (2016). Article Google Scholar * Zhu, Z. C. et al. Greening of the Earth and its drivers. _Nat. Clim. Change_ 6, 791–795 (2016). Article Google
Scholar * Smith, W. K. et al. Large divergence of satellite and Earth system model estimates of global terrestrial CO2 fertilization. _Nat. Clim. Change_ 6, 306–310 (2016). Article Google
Scholar * Buitenwerf, R., Rose, L. & Higgins, S. I. Three decades of multi-dimensional change in global leaf phenology. _Nat. Clim. Change_ 5, 364–368 (2015). Article Google Scholar
* Wang, S. et al. Recent global decline of CO2 fertilization effects on vegetation photosynthesis. _Science_ 370, 1295–1300 (2020). Article Google Scholar * Song, X.-P. et al. Global land
change from 1982 to 2016. _Nature_ 560, 639–643 (2018). Article Google Scholar * Jiang, M. et al. The fate of carbon in a mature forest under carbon dioxide enrichment. _Nature_ 580,
227–231 (2020). Article Google Scholar * Tucker, C. J. et al. An extended AVHRR 8 km NDVI dataset compatible with MODIS and SPOT vegetation NDVI data. _Int. J. Remote Sens._ 26, 4485–4498
(2005). Article Google Scholar * Pinzon, J. E. & Tucker, C. J. A non-stationary 1981–2012 AVHRR NDVI3g time series. _Remote Sens._ 6, 6929–6960 (2014). Article Google Scholar *
Didan, K. _Mod13q1 V006 MODIS/Terra Vegetation Indices 16-Day l3 Global 1km SIN Grid V006._ (NASA EOSDIS Land Processes DAAC, 2015). https://doi.org/10.5067/MODIS/MOD13A2.006 * Thornley, J.
H. Modelling shoot:root relations: the only way forward? _Ann. Bot._ 81, 165–171 (1998). Article Google Scholar * Higgins, S. I. et al. A physiological analogy of the niche for projecting
the potential distribution of plants. _J. Biogeogr._ 39, 2132–2145 (2012). Article Google Scholar * Hersbach, H. et al. The ERA5 global reanalysis. _Q. J. R. Meteorol. Soc._ 146, 1999–2049
(2020). Article Google Scholar * Muñoz Sabater, J. et al. Era5-Land: a state-of-the-art global reanalysis dataset for land applications. _Earth Syst. Sci. Data_ 13, 4349–4383 (2021).
Article Google Scholar * Cressie, N. & Wikle, C. K. _Statistics for Spatio-Temporal Data_ (Wiley & Sons, 2011). * Prentice, I. C. et al. in _Terrestrial Ecosystems in a Changing
World_ (eds Canadell, J. G. et al.) 175–192 (Springer, 2007). * Conradi, T. et al. An operational definition of the biome for global change research. _N. Phytol._ 227, 1294–1306. (2020).
Article Google Scholar * Zhao, M. & Running, S. W. Response to comments on “Drought-induced reduction in global terrestrial net primary production from 2000 through 2009".
_Science_ 333, 1093–1093 (2011). Article Google Scholar * Reich, P. B. et al. Effects of climate warming on photosynthesis in boreal tree species depend on soil moisture. _Nature_ 562,
263–267 (2018). Article Google Scholar * Hubau, W. et al. Asynchronous carbon sink saturation in African and Amazonian tropical forests. _Nature_ 579, 80–87 (2020). Article Google Scholar
* Odum, E. P. The strategy of ecosystem development. _Science_ 164, 262–270 (1969). Article Google Scholar * McGroddy, M. E., Daufresne, T. & Hedin, L. O. Scaling of C:N:P
stoichiometry in forests worldwide: implications of terrestrial Redfield-type ratios. _Ecology_ 85, 2390–2401 (2004). Article Google Scholar * Higgins, S. I. & Scheiter, S. Atmospheric
CO2 forces abrupt vegetation shifts locally, but not globally. _Nature_ 488, 209–212 (2012). Article Google Scholar * Buermann, W. et al. Widespread seasonal compensation effects of
spring warming on northern plant productivity. _Nature_ 562, 110–114 (2018). Article Google Scholar * Smith, W. K., Fox, A. M., MacBean, N., Moore, D. J. P. & Parazoo, N. C.
Constraining estimates of terrestrial carbon uptake: new opportunities using long-term satellite observations and data assimilation. _N. Phytol._ 225, 105–112 (2020). Article Google Scholar
* Ainsworth, E. A. & Long, S. P. What have we learned from 15 years of free-air CO2 enrichment (FACE)? A meta-analytic review of the responses of photosynthesis, canopy properties and
plant production to rising CO2. _N. Phytol._ 165, 351–372 (2005). Article Google Scholar * Porcar-Castell, A. et al. Linking chlorophyll _a_ fluorescence to photosynthesis for remote
sensing applications: mechanisms and challenges. _J. Exp. Bot._ 65, 4065–4095 (2014). Article Google Scholar * Magney, T. S. et al. Mechanistic evidence for tracking the seasonality of
photosynthesis with solar-induced fluorescence. _Proc. Natl Acad. Sci. USA_ 116, 11640–11645 (2019). Article Google Scholar * Farquhar, G. D., von Caemmerer, S. & Berry, J. A. A
biochemical model of photosynthetic CO2 assimilation in leaves of C3 species. _Planta_ 149, 78–90 (1980). Article Google Scholar * von Caemmerer, S. _Biochemical Models of Leaf
Photosynthesis_ (CSIRO, 2000). * Chuvieco, E., Pettinari, M., Otón, G., Storm, T. & Padilla Parellada, M. _ESA Fire Climate Change Initiative (Fire-cci): AVHRR-LTDR Burned Area Grid
Product_ Version 1.0. (Centre for Environmental Data Analysis, 2019); https://doi.org/10.5285/4f377defc2454db9b2a6d032abfd0cbd * Box, E. O., Holben, B. N. & Kalb, V. Accuracy of the
AVHRR vegetation index as a predictor of biomass, primary productivity and net CO2 flux. _Vegetatio_ 80, 71–89 (1989). Article Google Scholar * Wessels, K. J. et al. Relationship between
herbaceous biomass and 1 km2 advanced very high resolution radiometer (AVHRR) NDVI in Kruger National Park, South Africa. _Int. J. Remote Sens._ 27, 951–973 (2006). Article Google Scholar
* Zhu, X. & Liu, D. Improving forest aboveground biomass estimation using seasonal Landsat NDVI time-series. _ISPRS J. Photogramm. Remote Sens._ 102, 222–231 (2015). Article Google
Scholar * _LaplacesDemon: Complete Environment for Bayesian Inference_ R package v.16.1.4 (Statisticat-LLC, 2020). * Storn, R. & Price, K. Differential evolution–a simple and efficient
heuristic for global optimization over continuous spaces. _J. Glob. Optim._ 11, 341–359 (1997). Article Google Scholar * Mullen, K., Ardia, D., Gil, D., Windover, D. & Cline, J.
DEoptim: an R package for global optimization by differential evolution. _J. Stat. Softw_. https://doi.org/10.18637/jss.v040.i06 (2011). * Ardia, D., Boudt, K., Carl, P., Mullen, K. M. &
Peterson, B. G. Differential evolution with DEoptim: an application to non-convex portfolio optimization. _R J._ 3, 27–34 (2011). Article Google Scholar * Cleveland, R. B., Cleveland, W.
S., McRae, J. E. & Terpenning, I. STL: a seasonal-trend decomposition procedure based on Loess (with discussion). _J. Off. Stat._ 6, 3–73 (1990). Google Scholar * R Core Team _R: A
Language and Environment for Statistical Computing_ (R Foundation for Statistical Computing, 2020). * Chiu, G., Lockhart, R. & Routledge, R. Bent-cable regression theory and
applications. _J. Am. Stat. Assoc._ 101, 542–553 (2006). Article Google Scholar * Khan, S. A. & Kar, S. C. Generalized bent-cable methodology for changepoint data: a Bayesian approach.
_J. Appl. Stat._ 45, 1799–1812 (2018). Article Google Scholar * Whittaker, R. H. _Communities and Ecosystems_ (Macmillan, 1975). Download references ACKNOWLEDGEMENTS S.I.H. acknowledges
funding support from the BMBF SPACES project EMSAfrica grant number 01LL1801A and from the DFG project HI 1106/11-1. E.M. acknowledges a DAAD doctoral scholarship. FUNDING Open Access
funding provided by Universität Bayreuth. AUTHOR INFORMATION AUTHORS AND AFFILIATIONS * Plant Ecology, University of Bayreuth, Bayreuth, Germany Steven I. Higgins, Timo Conradi & Edward
Muhoko Authors * Steven I. Higgins View author publications You can also search for this author inPubMed Google Scholar * Timo Conradi View author publications You can also search for this
author inPubMed Google Scholar * Edward Muhoko View author publications You can also search for this author inPubMed Google Scholar CONTRIBUTIONS S.I.H. designed the study, performed the
analyses and led the writing. T.C. and E.M. performed the site selection and contributed to study design, interpretation of the analyses and writing. CORRESPONDING AUTHOR Correspondence to
Steven I. Higgins. ETHICS DECLARATIONS COMPETING INTERESTS The authors no conflict of interest. PEER REVIEW PEER REVIEW INFORMATION _Nature Geoscience_ thanks Sara Vicca, Steven Running and
the other, anonymous, reviewer(s) for their contribution to the peer review of this work. Primary Handling Editors: Tamara Goldin and Simon Harold, in collaboration with the _Nature
Geoscience_ team. ADDITIONAL INFORMATION PUBLISHER’S NOTE Springer Nature remains neutral with regard to jurisdictional claims in published maps and institutional affiliations. EXTENDED DATA
EXTENDED DATA FIG. 1 GRAPHICAL DESCRIPTION OF _Β_ PARAMETERS. Graphical representation of how the _β_ parameters define the influence of environmental forcing variables on rates in the
plant growth model as described by equations 13 to 17. All units are rescaled to the range 0-1 and the _β_ parameters represent positions on the x-axes. The effect of photosynthetically
active radiation, air temperature and atmospheric CO2 on carbon assimilation is described by the Farquhar photosynthesis model. Two additional _β_ parameters (_β__a_ and _β__b_) which
describe the the influence of site fertility on carbon and nitrogen assimilation are not represented in this diagram. In addition, for all analyses we set fire severity to zero, thereby
ignoring the effects of fire. EXTENDED DATA FIG. 2 DISTRIBUTION OF THE 100 STUDY SITES. The different colours indicate the biome assignments made using the authors assessment of the Google
Earth imagery. BF=boreal forest, GR=grassland, MT=Mediterranean type ecosystems, RF=tropical evergreen forest, SA=savanna, SH=shrubland, TF=temperate forest, TU=tundra. EXTENDED DATA FIG. 3
DISTRIBUTION OF STUDY SITES IN CLIMATE SPACE. The distribution of the 100 study sites in the temperature and mean annual precipitation climate space used by Whittaker61. The data points are
superimposed on Whittaker’s61 biome scheme. BF=boreal forest, GR=grassland, MT=Mediterranean type ecosystems, RF=tropical evergreen forest, SA=savanna, SH=shrubland, TF=temperate forest,
TU=tundra. EXTENDED DATA FIG. 4 EVI TIME SERIES ANALYSIS OF VEGETATION ACTIVITY FOR A SAVANNA SITE IN THE BURKINA FASO NATIONAL PARK. (A) MODIS EVI time series of vegetation activity (data)
and the state space model’s fit to these data (model). The blue polygon shows the 95% credible intervals around the mean model predictions which includes parameter, process and observation
uncertainty. (B) Anomalies in the NDVI data (blue bars) and the fit of a bent-cable regression to these anomalies. The polygons show the 95 % credible intervals of the bent-cable regression
predictions. (C) Zero intercept regression showing the model’s ability to predict observed anomalies, with full and detrended climate forcing data. Polygons show the 95% credible intervals
of the mean regression line. (D) Posterior density of the change in the full model’s regression slope (as shown in panel C) caused by removing trends in a climate forcing variable from the
full model; in this example the ability to predict anomalies was sensitive to soil moisture and soil temperature anomalies. Figure 1 shows an analogous plot using GIMMS NDVI data. EXTENDED
DATA FIG. 5 DISTRIBUTION OF NDVI AND EVI ANOMALY RESPONSE TYPES. The frequency of cup (initial decrease, subsequent increase), hat (initial increase, subsequent decrease), and linear time
trends in the anomalies in NDVI and EVI. The number of anomaly trends that showed an overall increase or decrease over the time series are indicated by different colours. Overall increases
or decreases less than 2 % in EVI were classified as small. The response types were classified from bent-cable and polynomial regression models fitted to the anomaly data from each site.
Panels show results using polynomical regression on the NDVI data (a), bent-cable regression on the EVI data (b) and polynomial regression on the EVI data. Figure 2 shows results for the
bent-cable regression on the NDVI data. EXTENDED DATA FIG. 6 COMPARISON OF ANOMALY RESPONSE TYPES FOR 2000-2015 FOR NDVI AND EVI DATA. Repeat of the analyses shown in Fig. 2 and Extended
Data Fig. 5 using the common time window (years 2000-2015) for which both EVI and NDVI data are available. Shown are the frequencies of cup (initial decrease, subsequent increase), hat
(initial increase, subsequent decrease), and linear time trends in the anomalies in vegetation indices. The number of anomaly trends that showed an overall increase or decrease over the time
series are indicated by different colours (the category “small" as defined in Fig. 2 and Extended Data Fig. 5 was not detected in these analyses). These plots reveal that although the
analysis time window influences the trends detected, that the EVI and NDVI data reveal similar trends, albeit with more decreasing trends in the NDVI data and more cup shaped trends when
using bent-cable regression. The EVI and NDVI response shapes of individual sites agreed for 52% and 45% of cases (respectively for polynomial and bent-cable analyses). The EVI and NDVI
response trends of individual sites agreed for 67% and 59% of cases (respectively for polynomial and bent-cable analyses). EXTENDED DATA FIG. 7 SENSITIVITY OF EVI VEGETATION ANOMALIES TO
CLIMATE ANOMALIES. The sensitivity is quantified as the effect of each of five forcing factors (T air = air temperature, T soil = soil temperature, M soil = soil moisture, S rad = solar
radiation and CO2 = atmospheric CO2 concentration) on the slope describing the ability of the model to predict anomalies in the vegetation activity time series (Extended Data Fig. 4). The
slope with the full model is represented by the red colour ramp. Shown in the matrix are the 75 of 100 sites where anomalies in vegetation activity could be attributed to the environmental
forcing factors. The coloured circles indicate the response groups the sites are assigned to by an unsupervised classification. The site names are codes indicating ecosystem type (BF=boreal
forest, GR=grassland, MT=Mediterranean type ecosystems, RF=tropical evergreen forest, SA=savanna, SH=shrubland, TF=temperate forest, TU=tundra), country and site name. Red colours indicate
high sensitivity, blue colours indicate low sensitivity. See Fig. 3 for the same analysis using NDVI data. EXTENDED DATA FIG. 8 ECOSYSTEM SENSITIVITY CLASSES IN RELATION TO CLIMATE (USING
EVI DATA). (A) The distribution of attribution classes (Extended Data Fig. 7) in bivariate temperature and moisture climate space. Points represent the locations of the 100 study sites in
climate space. Attribution classes are the two major groups classified in Extended Data Fig. 7. Sites labelled 0 are sites excluded from Extended Data Fig. 7 because the model could not
explain observed anomalies. The colours of the sites labelled 1 and 2 correspond to the groups classified in Extended Data Fig. 7. The ellipses represent the fitted covariance estimates of
the classes as estimated by discriminant analysis based on Gaussian finite mixture modelling. (B, C) The shape and direction of the anomaly response (as defined in Extended Data Fig. 5)
plotted in bivariate climate space. The mean annual temperature and mean annual soil moisture were calculated over the study period using the ERA5 reanalysis data. Panels D, E and F plot the
points in panels A, B and C in geographic space. See Fig. 4 for the same analysis using NDVI data. EXTENDED DATA FIG. 9 ANOMALY PREDICTION WITH FULL AND DETRENDED CLIMATE DATA. The slopes
of the zero intercept regression of the model’s ability to predict anomalies in vegetation activity when using the full and detrended climate data (for example Fig. 1C). The points represent
the mean of the posterior estimates of the slope, the tick marks span the 95 % credible intervals of the estimates. Attribution is diagnosed if the slopes are positive and clearly higher
(no overlap in the credible intervals) for model runs forced by the full climate data than for model runs forced by climate data with trends removed. Panel (a) shows the results when using
the NDVI data and panel (b) when using EVI data. EXTENDED DATA FIG. 10 SUMMARY OF NDVI AND EVI ANOMALY PREDICTION WITH FULL AND DETRENDED CLIMATE DATA. Probability density functions of the
slopes of the zero intercept regression of the model’s ability to predict anomalies in vegetation activity when using the full and detrended climate data (shown in Extended Data Fig. 9).
Solid lines are for all sites, broken lines are for the cases where attribution was diagnosed.Panel (a) shows the results when using the NDVI data and panel (b) when using EVI data.
SUPPLEMENTARY INFORMATION SUPPLEMENTARY INFORMATION Supplementary Information, Tables 1–4 and the Farquhar photosynthesis model. RIGHTS AND PERMISSIONS OPEN ACCESS This article is licensed
under a Creative Commons Attribution 4.0 International License, which permits use, sharing, adaptation, distribution and reproduction in any medium or format, as long as you give appropriate
credit to the original author(s) and the source, provide a link to the Creative Commons license, and indicate if changes were made. The images or other third party material in this article
are included in the article’s Creative Commons license, unless indicated otherwise in a credit line to the material. If material is not included in the article’s Creative Commons license and
your intended use is not permitted by statutory regulation or exceeds the permitted use, you will need to obtain permission directly from the copyright holder. To view a copy of this
license, visit http://creativecommons.org/licenses/by/4.0/. Reprints and permissions ABOUT THIS ARTICLE CITE THIS ARTICLE Higgins, S.I., Conradi, T. & Muhoko, E. Shifts in vegetation
activity of terrestrial ecosystems attributable to climate trends. _Nat. Geosci._ 16, 147–153 (2023). https://doi.org/10.1038/s41561-022-01114-x Download citation * Received: 15 January 2021
* Accepted: 06 December 2022 * Published: 06 February 2023 * Issue Date: February 2023 * DOI: https://doi.org/10.1038/s41561-022-01114-x SHARE THIS ARTICLE Anyone you share the following
link with will be able to read this content: Get shareable link Sorry, a shareable link is not currently available for this article. Copy to clipboard Provided by the Springer Nature
SharedIt content-sharing initiative