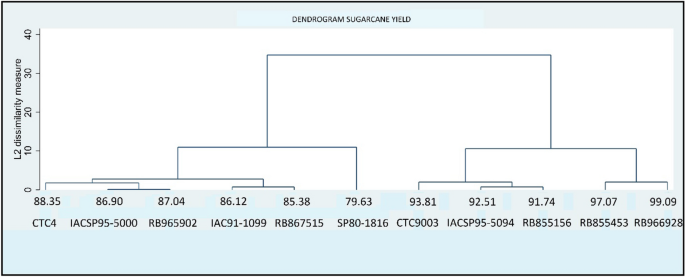
Measuring climate change’s impact on different sugarcane varieties production in the south of goiás
- Select a language for the TTS:
- UK English Female
- UK English Male
- US English Female
- US English Male
- Australian Female
- Australian Male
- Language selected: (auto detect) - EN
Play all audios:

ABSTRACT A crucial aspect analysed during the last years, aiming to improve sugarcane production, is the impact of climate change on sugarcane productivity. One of the strategies to mitigate
climate change's impact on sugarcane yield is the development of new varieties known to positively affect crop production. This paper analysed how climate change impacts sugarcane
production regarding the different planted varieties. Data regarding sugarcane harvest were collected from a cooperative in the south of Goiás state—Brazil, the second biggest national
sugarcane producer. Results indicate that climate impact on sugarcane yield is irrelevant when controlling for different varieties. Considering the results presented in this work, the
Brazilian government should keep the incentives for the development of new sugarcane varieties and, at the same time, spur sugarcane producers to use the new sugarcane varieties. The results
imply that if the variety is correctly chosen, sugarcane can be produced without harming the environment, contributing to reaching SDG 15. Moreover, it is less probable that an adverse
climatic event will destroy the planted area, preventing sugarcane producers from severe loss and contributing to achieving SDGs number 1 and 2. SIMILAR CONTENT BEING VIEWED BY OTHERS IMPACT
OF RECENT CLIMATE CHANGE ON CORN, RICE, AND WHEAT IN SOUTHEASTERN USA Article Open access 08 October 2022 ANTICIPATED CHANGES IN SOYBEAN DROUGHT STRESS AND YIELD STABILITY UNDER FUTURE
CLIMATES IN NORTHEAST CHINA Article Open access 18 March 2025 IMPACTS OF RECENT CLIMATE CHANGE ON CROP YIELD CAN DEPEND ON LOCAL CONDITIONS IN CLIMATICALLY DIVERSE REGIONS OF NORWAY Article
Open access 03 March 2023 INTRODUCTION Brazil is the largest sugarcane producer in the world, having produced more than 654 million tons in the 2020/2021 harvest. In the same year, Goiás
produced 74.04 million tons of sugarcane1. These data place the State of Goiás as the second major Brazilian sugarcane producer, behind the State of São Paulo. Brazil has a long history of
developing new sugarcane varieties, having invested in this research for over 30 years2. Oliveira, Barbosa, and Daros2 state that in 2020, genetically modified sugarcane varieties provided
solely by RIDESA (the oldest Brazilian sugarcane genetic research company) represented more than 60% of Brazilian sugarcane fields, having reached 68% in 20152. The Brazilian Agricultural
Research Company – EMBRAPA’s Technological Information Agency (EMBRAPA-AGEITEC)3 argues that a significant part of the rise in sugarcane productivity is related to using new sugarcane
varieties. According to some authors, the sugarcane production expansion results from occupying the natural area through deforestation, causing environmental and social issues4,5,6.
Antonelli7, for example, argues that food production contributes heavily to climate change and pollution. The former discussed issues denote the importance of research for increasing
sustainable sugarcane production and reducing social and environmental impacts. An important step is to understand each variable's behaviour in sugarcane production. In this sense, some
studies have tried to estimate the impact of specific variables on sugarcane production8,9,10,11,12. One aspect that has been recently analysed is the impact of climate change on sugarcane
productivity. Gbetibouo and Hassan13, Adhikari, Nejadhashemi and Woznicki14, Ali et al.15, and Knox et al.16, for example, agree that climate change has exerted and will employ a significant
impact on agriculture, including sugarcane production. Climate change has sometimes led to severe economic and non-economic loss17. Wheeler and Von Braun18 state that climate change can
endanger food production, slowing the progress toward the end of hunger and worsening food insecurity. One approach to mitigate climate change's impact on sugarcane production and
productivity is the development of new varieties19,20,21,22,23,24. Despite its possible positive effects, developing new sugarcane varieties demand further crop-variety-specific research25.
Nevertheless, few researchers, such as Linnenluecke et al.26 and Verma et al.27, researched this subject. Others, such as Dias and Sentelhas23, performed their analysis considering specific
sugarcane varieties. This lack of information regarding sugarcane crops with different varieties indicates a considerable research gap once the varieties’ specificities may impact the final
production. The main objective of this paper is to analyse how climate change impacts sugarcane yield, considering the climatic variables and the different planted varieties. The hypothesis
guiding this research argues that the climatic impacts on sugarcane productivity lose strength by controlling for sugarcane varieties. The main research objective is aligned with United
Nations Sustainable Development Goals (SDG) 2 (zero hunger) and 15 (life on land). Nevertheless, Aguilar-Rivera28 states that improving sugarcane production and productivity also
collaborates to reach SDG number 1 (ending poverty in all its forms everywhere), 3 (ensuring healthy lives and promoting well-being for all at all ages), 7 (ensuring access to affordable,
reliable, sustainable, and modern energy for all), 9 (building resilient infrastructure, promoting inclusive and sustainable industrialization and foster innovation). CLIMATIC CHANGE AND
GOIÁS’ SUGARCANE PRODUCTION SUGARCANE AND CLIMATE VARIATION A critical approach for increasing sugarcane productivity is to understand each variable’s behaviour, enabling the structure of
more reliable production models. Ahmad et al.29 and Abdoulaye et al.30 highlight the importance of analysing the impact of climate change on sugarcane production. Singh et al.31 and Swami,
Dave, and Parthasarathy32 argue that climate change has become a significant threat to sustainable agriculture. Santos et al.19 argue that climate change promoted greater crop demand,
including higher water scarcity resistance and soil conditions difficulties. Khan, Shah, and Iftikhar-Ul-Husnain33 assert that climate change has led to global warming, unpredicted storms,
drought, flood, and crop losses, affecting agricultural productivity and causing food insecurity. Akbar and Gheewala34 and Mulinde et al.35 discuss the possible threats climate change poses
to agriculture and food production. Khan, Shah, and Iftikhar-Ul-Husnain33, Silva et al.36, McGree et al.37, Kelkar, Kulkarni, and Rao38 and Chandio et al.39 analysed the impact of climate
change on sugarcane production in different places and came to a similar conclusion. The authors claim that the amount of rain is positively related to the increase in productivity, while
the increase in temperature has an inverse relationship. Singh et al.31 and Swami, Dave, and Parthasarathy32 agree that rising temperatures harm sugarcane yield. In its turn, Jyoti and
Singh40 came to a different conclusion on the rainfall´s impact on sugarcane production. They argued that climate change and rainfall negatively affect Indian sugarcane yield. Analysing the
impact of climate change on the southeast region of Punjab, Pakistan, Akbar and Gheewala34 concluded that the evapotranspiration rate is expected to increase in the future, leading to an
expansion in sugarcane and cotton crop water requirement. Linnenluecke et al.26 investigated how climate change impacted sugarcane output in different regions of Australia. The authors state
that increased atmospheric carbon concentration and maximum temperatures have harmed sugarcane production after 1995. Linnenluecke et al.26 considered the impact of the different sugarcane
varieties on sugarcane production. As far as we know, this is the only research considering this specificity in the econometric model. Ahmad et al.29 concluded that irrigation, rainfall, and
soil condition positively and significantly impact sugarcane production decrease in Pakistan. Heureux et al.41 studied how climate trends will impact Pakistan’s agricultural production in
Indus River Basin. The authors concluded that temperatures rising caused by climate change would negatively affect the primary crop production in the region, including sugarcane production.
Rehman et al.42 analysed how Pakistan’s agricultural crop is related to CO2 emission and discovered that sugarcane is positively associated with this gas, which has responsibility for global
warming. Ncoyini, Savage, and Strydom43 state that climate change and the lack of access to climate change information by small-scale farmers have decreased sugarcane production in South
Africa. Some researchers however found no impact of extreme climate change on sugarcane production. Kumar et al.44 for example argues that neither Indian summer monsoon rainfall, nor the El
Niño influenced in Indian sugarcane production during the analysed years. The authors claim that strategies adopted by sugarcane producers, such as irrigation were able to diminish climate
impact influence. Others such as Ray et al.45 argue that climate change impact on crop production (including sugarcane) varies according to the region studied. Therefore, meanwhile climate
change has reduced sugarcane production, in places such as Northern Africa, Sub Saharan Africa and Western, Southern and South-eastern Asia) in other regions climate change has increased
sugarcane production (the Americas, Central and Eastern Asia and Western and Southern Europe). Some authors tried to predict how climate change will impact sugarcane production in the
future. Santos et al.19 simulated several scenarios through DSSAT/CANEGRO model for the production based at Alagoas, Brazil. The authors concluded that temperature rise will cause energy
sugarcane yield to increase until 2060. Da Silva et al.46 warn that due to the lack of rainfall caused by climate change, in the future, a significant part of the sugarcane crop in the state
of São Paulo will require irrigation. Jaiswal et al.47 used the CANEGRO-Sugarcane crop model to predict the influence of climate change on sugarcane production in the mid and long future.
The authors conclude that a rise in the minimum and maximum temperature in the future will lead to an accelerated plant growth, but a shorter amount of sucrose. Verma et al.27 used
DSSAT-CSM-CANEGRO for simulating climate change impact on different Indian sugarcane varieties the authors demonstrate how climate change impact the different sugarcane varieties
distinctively. Despite important, crop simulation must be analysed carefully. Yin et al.48 demonstrate that Chinese sugarcane crop simulation made from 1980 to 2009 presents wrong results,
due to problems with the main models used. SUGARCANE VARIETIES Sugarcane’s characteristics related to resisting adverse climatic situation, including high or low temperatures and drought
issues, has already been observed by several authors19,20,21,22,23,24,49. Different crop varieties require different crop-variety-specific research25,50. According to EMBRAPA-AGEITEC3, the
sugarcane genetic improvement promoted by different laboratories aiming to better respond to soil and climate adversities and diseases has been paramount for increased sugarcane productivity
in the past years. Antonelli7 states that genomic sequencing and gene-editing techniques are paramount to increasing crop production without harming the environment, resisting climate
change, and resistance to pests and diseases. Ali et al.15 state that in order to mitigate the effects of climate change, it is necessary to develop different crop varieties. Oliveira,
Barbosa, and Daros2 state that the main target of sugarcane genetic improvement programs is productivity upgrading. IAC51 states that new varieties are being developed to guarantee better
productivity, focusing on regional specificities. EMBRAPA-AGEITEC3 and CTC52 assert that several varieties must be used to ensure better results. Oliveira, Barbosa, and Daros2 present the
main varieties used in Brazil in the 2019/2020 harvest. This information is replicated in Appendix A. According to EMBRAPA-AGEITEC3, the main sugarcane varieties used in Brazil come from the
following laboratories: Interuniversity Network for the Sugar Energy Sector Development (RIDESA), COPERSUCAR, Sugarcane Technology Center (CTC), and Campinas Agronomic Institute (IAC). Each
sugarcane variety possesses different characteristics, making it adequate for different regions. EMBRAPA-AGEITEC3 displays the differences between the main sugarcane varieties used in
Brazil. Table 1 summarizes the critical information. The information displayed in Table 1 indicate that it is essential to choose the suitable variety according to the region and the
different characteristics of each farm. Considering the significant disparity between different varieties, it becomes evident that sugarcane research must consider which variety is being
studied to avoid spurious results. SUGARCANE GENETIC RESEARCH IN BRAZIL Brazil has a long history of genetic research related to sugarcane. As stated by Oliveira, Barbosa, and Daros2, in
1971, the Sugar and Alcohol Institute (IAA), which belonged to the Industry and Trade Ministry, created the Sugarcane Improvement National Program (Planalsucar), which main target was to
increase the national sugarcane yield. According to Oliveira, Barbosa, and Daros2, the Planalsucar developed and launched the RB sugarcane variety, which would become one of the main
sugarcane varieties used in Brazil. Today, the RB variety is developed by RIDESA, once the Planalsucar was closed in 1990. Gazaffi et al.53 state that in the 2015/2016 season, 68% of the
sugarcane-grown area was composed of RB varieties. Machado Jr and Braga Jr54 argue that the RB varieties are among those with more longevity, and that even though some of the varieties were
developed at the beginning of the 1990s, they are still among the best available options. Various sugarcane genetic research has been developed in Brazil. As stated in the previous section,
Brazil has four prominent sugarcane genetic research institutes: RIDESA, COPERSUCAR, CTC, and IAC. Rossetto et al.55 state that eight new sugarcane varieties are launched each year in
Brazil, and that to increase sugarcane yield, producers must correctly choose the sugarcane variety that best suits their location. Boschiero et al.56 agree that choosing the correct
sugarcane variety is crucial for achieving better results. Aiming to improve Brazilian sugarcane productivity, Dias et al.57 investigated the impact of silicon treatment in four different
sugarcane varieties used in São Paulo: RB867515, RB72454, SP81-3250, and SP83-2847. The authors claim that the RB72454 and SP83-2847 varieties were more responsive (presented better
productivity) than the others. Chapola et al.58 show that some sugarcane varieties responded better to the Orange Rust disease in Brazil. The authors conclude that over the years, sugarcane
producers migrated to sugarcane varieties more resistant to the disease. After many years of genetic research, in 2017, the first genetically modified sugarcane cultivar (CTC20BT) was
commercialized. Cheavegatti-Gianotto et al.59 present this variety´s development, deregulation, and commercialization. RESEARCH METHODOLOGY DATABASE Data regarding sugarcane harvest were
collected in a sugarcane cooperative in the south of Goiás. The data sent by the cooperative refers to the total area production, production separated by sugarcane variety, cuts separated by
crop, the yield of each cultivar, and the city where the crop was harvested. All data refer to 2021 and are farm-level detailed. According to the sugarcane cooperative, 27 sugarcane
varieties are used by farmers in that region. Table 2 presents the used sugarcane varieties, the frequency in the dataset, their percentual share of the production, and the cumulative
percentage. Despite the vast number of varieties, the first eleven represent over 90% of the total production. Thus, aiming to avoid collinearity problems, only those eleven first varieties
were considered in the analysis. All climate data were collected according to the city where the farm is located. Data concerning climate, such as precipitation and average temperature, were
collected at INMET60. CO2 emission data was collected at SEEG61 and concerned the year 2019, the last year with available data. According to Silva et al.36 results, the mean temperature is
expected to present a negative relationship, while precipitation presents a positive relationship to sugarcane yield. Marengo et al.62 confirm that temperature variation impacts sugarcane
yield. Concerning CO2 emissions, according to Linnenluecke et al.26, it is expected that this variable presents a positive impact on sugarcane yield. Crop cost data and fixed costs were
collected at CONAB63. The detailed production cost data are presented in Table 3. It is essential to notice that the Brazilian cost structure differs from other countries, like Pakistan,
where, according to Muzammil, Zahid, and Breuer64, a considerable share of sugarcane crop cost constitutes the irrigation structure. Moreover, besides adding cost to the production, the
irrigation process may also increase pollution once it is based on diesel power. Following Ali, Zubair, and Hussain65, Crop Cost is expected to present a positive relationship with Sugarcane
Yield. In order to analyse the statistical characteristics (mean, standard deviation, maximum and minimum values) of the considered data, the descriptive statistics of the analysed
variables was performed. Results are presented in Appendix B. The analysis indicates that although Precipitation and Mean Temperature present slight variations across the region, the same
cannot be said regarding CO2 emissions. Crop Cost also presents huge variation; however, it must be considered that crop area varies across the farmers, impacting crop cost. METHODOLOGY The
research methodology adopted in this work was based on the investigation performed by Silva et al.36. The authors gathered municipality-level data and performed a panel data analysis,
controlling for the region in which data had been collected in the Paraíba State, Brazil. Despite the importance of the research made by the authors, in trying to perform a similar analysis
in the state of Goiás, we considered it necessary to add some information to the model. This research performed a cross-section analysis due to the availability of data. Also, this work
focused on a specific region in the south of the state of Goiás. Therefore, the soil interference in sugarcane production was neutralized. Moreover, unlike Silva et al.36, this work used the
sugarcane yield as a dependent variable. Ahmad et al.29 explain that climate change can severely impact sugarcane yield. Therefore, using the sugarcane yield of each sugarcane variable will
provide a better understanding of the relationship between climate change and sugarcane production. Following Ali, Zubair, and Hussain65, the total cost of fertilizer and machinery was used
as the control variable. However, different from Ali, Zubair, and Hussain65, this work used each farm´s total crop expenditure and total fixed cost. As exposed before, those numbers are
provided by CONAB and represent an average estimation of the farm’s expenditure, separated by the size of the farm. CONAB classifies the farms into Familiar Agriculture or Company, based on
Brazilian law 11.326/2006 66, which provides unique benefits to small farmers. Therefore, before collecting data from CONAB, all the farmers listed by the sugarcane cooperative were
separated according to their size. Linnenluecke et al.26, Chandio et al.39, and Rehman et al.42 argue that CO2 emissions can also impact sugarcane production. This work followed these
authors and included the CO2 variable in the model. After collecting the data, a Cluster Analysis was performed. The dendrogram was performed after Ward’s Linkage clustering, with Euclidean
Dissimilarity measure, based on the average sugarcane yield of each variety of the 2021 harvest. Afterward performing the Cluster analysis, data were prepared for the regression examination.
For the regression analysis, the Stata 17 software was used. First, heteroskedasticity and multicollinearity tests were performed. Heteroskedasticity problems were solved using cluster
standard errors. The variance inflation factor (VIF) test was used for the multicollinearity. Multicollinearity problems appeared after inserting Radiation and Humidity variables. In order
to prevent the study from spurious results, following Gujarati and Porter67, those variables were retrieved from the study. After the control tests, the regression analysis was performed
using the model presented in the next section. MODEL The econometric model used in the regression analysis is presented in Eq. (1): $$\begin{aligned} \Upsilon i = & \beta 0 + \beta
1\;Precipitation + \beta 2\;Mean\; Temperature + \beta 3CO_{2} \\ & + \beta 4CUTi + \beta 15\;Crop \;cost + \beta 16 \;Fixed\; cost + u \\ \end{aligned}$$ (1) Υi is the sugarcane yield
of the analysed variety in the period. \(\beta 0\) is the coefficient of the constant of the equation. Precipitation, Mean Temperature, and CO2 refer to the climatic information of each city
where the farm is located. β4 is the number of times the crop has been cut (harvested). Crop cost and Fixed cost refers to the production cost. Finally, µ is the error term of the equation.
After the establishment of the econometric model, the regression analysis was performed after the Ordinary Least Squares method. RESULTS Figure 1 exhibits the dendrogram. The Fig. 1
displays the average sugarcane yield of each variety and the name of each variety right below. As can be seen, there are four major groups. The first one comprises the CTC4, IACSP95-5000,
RB965902, IAC91-1099, and RB867515 varieties. The second group is constituted solely by the SP80-1816 variety. The CTC9003, IACSP95-5094, and RB855156 varieties form the third one. The
fourth group contains the RB855453 and the RB966928 varieties. Figure 1 shows that the SP80-1816 variety is less productive among the group. Regarding production, the two most productive
varieties (RB855453 and RB966928) represent only 1.10% of the total production (considering only the eleven most essential varieties production). The second most productive group – group
number 3 – is responsible for 0.7% of the production, while group 2 (the less productive) answers for 0.06% of the total production. Most cooperative sugarcane production comprises group 1,
with more than 98% of the total production. Regarding the regression analysis, Table 4 presents the results: Table 4 results indicate that neither rainfall, mean temperature, nor even CO2
emissions impact sugarcane yield for most of the varieties. Table 4 rainfall’s result is in line with26,50. Table 4 results show that climatic variables lose their importance in sugarcane
yield when using the appropriate sugarcane variety. Regarding the variable Precipitation, RB855453 (column 9) was the only sugarcane variety with significant result. Finally, although small,
sugarcane variety RB965902 (column 10) presented significant results for Crop Cost and Fixed Cost. ROBUSTNESS CHECK The regression analysis was performed again to analyse how sugarcane
production answered extreme climate conditions, replacing the mean temperature variable for maximum and minimum temperature. Due to collinearity problems, the regression was run separately.
Figure 2 presents how Maximum and Minimum temperature impacts on the sugarcane yield of each variety. The tables containing the regression results can be found at Appendix C. None of the
presented results were significant. Nevertheless, it is possible to observe that Minimum temperature possess a larger impact on sugarcane yield that Maximum temperature. ROBUSTNESS CHECK
2–BOOTSTRAP ANALYSIS Because of the small number of observations, a Bootstrap analysis was performed. The Bootstrap method was first presented by Efron and Tibshirani68 and, according to
Joshi, Seidel-Morgenstern and Kremling69, it uses stochastic elements and repeated simulations to analyse the properties under consideration and overcome the theoretical limitations of a
finite sample. The Bootstrap regression was performed considering the three temperature variables. The regressions are presented in Appendix D. DISCUSSION Precipitation results presented few
significant results, indicating that precipitation does not affect sugarcane yield. In fact, in some cases, precipitation presented negative results (RB855156, column 5 Table C1 and Table
D2, RB867515, column 2 Table D1 and Table D3). Those results confirm Ali et al.15, Knox et al.16, and Kumar et al.44. Regarding the Brazilian studies, we believe that the contradictory
results presented by this research can be explained by two main reasons: 1° Silva et al. 36 conducted their research at Paraíba state, which is much drier than the South of Goiás. 2° Santos
et al.19 and Da Silva et al.46 performed simulations for future crops, which provided forecasts, and not real interaction among the variables. The lack of significant results in all
temperature (mean, minimum and maximum) regressions confirms Knox et al.16 and Kumar et al.44 papers. However, even though it was insignificant, Fig. 2 and Tables C1, C2, D2 and D3
demonstrate that minimum temperature has a more decisive influence on sugarcane yield. This result aligns with Swami, Dave, and Parthasarathy32, who observed the same pattern in their study.
Moreover, Fig. 2 and Tables C2 and D3 results do not confirm Marengo et al.62. The authors demonstrate that several crop productions, including sugarcane, were impacted by the unusual cold
conditions reported for the 2021 winter period (June–August). One possible explanation for the different results presented in this research is that most of the sugarcane production of Goiás
state comes from the “Year Sugar” method, in which the sugarcane crop is planted between October and December and harvested after one year. Therefore, the cold event registered in 2021 did
not affect the sugarcane crop of the South Region of Goiás because when the cold happened, the sugarcane crop was being harvested or on the verge of it. Concerning the CO2 variable, the
RB867515 variety (column 2) presented significant positive results for the CO2 Emissions in Table D1, and it was confirmed in Tables D2 and D3. This result means that, although small, the
increase in temperature resulting from the increase in CO2 emissions may benefit this variety yield. Relating to the CO2 emissions in Table 4, the lack of significance, contrasting with
Linnenluecke et al.26 results, may be explained by the difference in the location of the sugarcane crop. As the state of Goiás is significantly closer to the equatorial line than Australia,
the CO2 emissions in that region may provide a warmer climate, which benefits the sugarcane crop. It must be stressed that apart from significant results presented by the RB867515 variety in
the Bootstrap regression (Tables D1, D2 and D3), all the other sugarcane varieties in all the other analyses (Tables 4, C1, C2, D1, D2 and D3) presented non-significant results, which
confirms the hypothesis above. Regarding the fact that our research confirmed so few results, we believe there are four possible explanations: * 1. More control variables were inserted,
guaranteeing that climatic variables presented their real impact without “aggregating” missing variables’ results. The Cut variable, for example, that represents how many times the crop has
been harvested, which is critical information when analysing the sugarcane crop and presented significant results in almost all cases, is hardly ever used as a control variable. Silva et
al.36, for example, only presented control variables for the different state regions. McGree et al.37 only used climatic variables in their model. The same problem can be observed in the
research of Kelkar Kulkarni and Rao38. Ahmad et al.29 investigated separate climatic events, such as rainfall, without inserting temperature variables in their study. * 2. As we controlled
for the different sugarcane varieties, the climate variables’ impact lost strength; therefore, their results were not significant. Despite many authors’ awareness of the importance of
accounting for different sugarcane varieties, only few authors such as Dias & Sentelhas23, Linnenluecke et al.26 and Verma et al.27 took this information under consideration. * 3. The
lack of information may have impacted the results presented (from this and other works). Knox et al.16 argue that the lack of data has led to contradictory and non-significant results in
their work. The difficulty in having access to real data is an obstacle when researching the impacts of climate change on sugarcane yield. This situation may also explain the amount of work
focusing on future forecasts, which may or may not provide correct answers once they cannot access all the future outcomes that may influence sugarcane yield. Indeed, it is possible to
notice that many simulation research presents contradictory results when compared to those which had access to real data – Santos et al.19 and Silva et al.36 for Brazil, Singh et al.31 and
Swami, Dave, and Parthasarathy32 for Maharashtra – India, for example. According to Yin et al.48, many crop simulations provided for China present wrong results due to problems with the
models used, which underestimate multi-year sugarcane yield and fall short of simulating the pattern of interannual variability. * 4. Results may change according to the region studied. Knox
et al.16, Swami, Dave, and Parthasarathy32, Jyoti and Singh40, and Ray et al.45 proved it in their work. Moreover, more subtle facts can influence the climate change impact on sugarcane
yield. Ncoyini, Savage, and Strydom43, for example, demonstrate that the deprivation of access to climate change information and the absence of know-how to deal with it increases the
potential damage caused by climate change in South Africa. CONCLUSIONS Since the beginning of the 1970s, Brazil has invested in the sugarcane genetic research. However, despite all the
efforts made in the genetic field, only a small number of research have been made aiming to analyse how those new varieties interact with climate change conditions. This paper intended to
answer this question. Results indicate that climate conditions do not affect the sugarcane yield when controlling for the sugarcane variety. This conclusion does not align with most research
regarding the relationship between sugarcane-climate change. However, as exposed in the previous chapters, most of the research does not consider the different sugarcane varieties.
Moreover, most of them lack consistent control variables in their econometric model. Results are essential to increase sugarcane productivity and its final production. It becomes clear that
when controlling for the correct sugarcane varieties, the adverse climate conditions are minimized, making it possible to produce sugarcane without harming the environment, contributing to
reaching SDG 15. Moreover, it is less probable that an adverse climatic event will destroy the planted area, preventing sugarcane producers from losing their investment. This result is
essential if we consider the small producer, who has no condition to afford significant losses. This outcome is vital to achieving SDGs numbers 1 and 2. Moreover, following Pienkowski et
al.70 results, by helping to increase sugarcane production and diminish food insecurity, this work also contributes to SDG 3. According to the above-quoted authors, food insecurity may
develop depression and mental health problems among the population. Despite all the benefits provided by different sugarcane varieties regarding the climatic change, it is essential to
notice that most of the varieties used by sugarcane producers of the studied region were developed in the 1990s. Machado Jr and Braga Jr54 claim that most Brazilian sugarcane production
relies on “older” varieties. Therefore, considering the results presented in this work, in order to increase sustainable sugarcane production, preventing from endanger natural resources,
restrain hunger, and without being threatened by climatic change, the Brazilian government should keep the incentives and sustain the development of new sugarcane varieties, and, at the same
time, spur sugarcane producers to use the new sugarcane varieties. Sugarcane genetic research is well-developed in Brazil, and several institutions promote different sugarcane varieties to
surmount different production difficulties. However, this only happens for some sugarcane country producers. Hence, other countries' policymakers should elaborate long-term policies
supporting the research and adoption of different sugarcane varieties. With the appropriate sugarcane variety, climate change impacts on sugarcane production will be mitigated, assuring
there will not be a shortfall of production in the future. Despite the originality of this work, it contains some limitations. First, it considered one-year data observation only. It is
suggested that other studies with larger datasets are performed. Secondly, it observed only one production region. Different regions should be analyzed in future research to enhance the
understanding of climate change impacts. Finally, the utilization of annual data does not provide a good overview of the relationship between climate change-sugarcane yield, once more
critical than the amount of precipitation in the determined region when such rain falls. The same can be said regarding hot weather. When it comes to precipitation, for example, it must be
analysed if it is raining when sugarcane is planted or not. Therefore, future research should use a monthly database indicating when sugarcane is planted and harvested. DATA AVAILABILITY The
data that support the findings of this study are available from the studied sugarcane cooperative but restrictions apply to the availability of these data, which were used under license for
the current study, and so are not publicly available. Data are however available from the corresponding author upon reasonable request and with permission of the sugarcane cooperative.
REFERENCES * CONAB—(National Supply Company). Historical Series of Crops. brasilia, Sugarcane – Industry and Agriculture (in portuguese). Available online
https://www.conab.gov.br/info-agro/safras/serie-historica-das-safras. Access on May, 10 (2022). * Oliveira, R. A., Barbosa, G. V. S., Daros, E. 50 Anos de Variedades RB de Cana de Açúcar: 30
Anos de RIDESA. (2022) Preprint at: https://www.ridesa.com.br/_files/ugd/242756_f3e42975bd9a42589327663cde52e255.pdf. * EMBRAPA – AGEITEC – (Brazilian Agricultural Research Company –
EMBRAPA’s Technological Information Agency). Knowledge tree – sugarcane. (2022) Preprint at:
https://www.agencia.cnptia.embrapa.br/gestor/cana-de-acucar/arvore/CONTAG01_42_1110200717570.html. * Moroni, M. S. C., Da Silva, O. G. M., Zambon, V., Nocelli, R. C. F. Cultura de cana de
açúcar no Brasil: manejo, impactos econômicos, sociais e ambientais In: Fontanetti CS, Bueno OC (eds) _Cana de Açúcar e Seus Impactos: Uma Visão Acadêmica_. (Canal Editora, 2017). *
Rodrigues, L. D. _A cana de açúcar como matéria-prima para a produção de biocombustíveis: Impactos ambientais e o zoneamento agroecológico como ferramenta para mitigação_ (Federal University
of Juiz de Fora, 2010). Google Scholar * Ferreira, B. P., Maida, M. Monitoramento dos recifes de coral no Brasil—Situação atual e perspectivas. _Environmental ministry of Brazil
biodiversity and forest secretary_, p. 116 (2006). * Antonelli, A. Indigenous knowledge is key to sustainable food systems. _Nature_ 613, 239–242. https://doi.org/10.1038/d41586-023-00021-4
(2023). Article ADS CAS PubMed Google Scholar * Andrade, A. S., Resende, R. S., Moura, M. S. B., Bufon, V. B. Irrigação em cana de açúcar In: Silvaf C, Alves BJR, Freitas PL (eds)
S_istema de Produção Mecanizada em Canadeaçúcar Integrada à Produção de Energia e Alimentos_ (Embrapa, 2017). * Rossetto, R., Spironello, A., Cantarella, H. & Quaggio, J. A. Calagem para
a cana-de-açúcar e sua interação com a adubação potássica. _Bragantia_ 63, 105–119 (2004). Article CAS Google Scholar * Oliveira, R. A. & Bueno, L. R. O impacto do financiamento do
Pronaf sobre indicadores agrícolas nas lavouras do estado do Paraná: Uma análise de dados em painel. _Redes_ 24, 292–309. https://doi.org/10.17058/redes.v24i1.7599 (2019). Article Google
Scholar * Amorin, M. T. A. _et al._ Impact of soil types on sugarcane development monitored over time by remote sensing. _Precis. Agric_ 23, 1532–1552.
https://doi.org/10.1007/s11119-022-09896-1 (2022). Article Google Scholar * Tayyab, M. _et al._ Sugarcane cultivar-dependent changes in assemblage of soil rhizosphere fungal communities in
subtropical ecosystem. _Environ. Sci. Pollut. Res._ 29, 20795–20807. https://doi.org/10.1007/s11356-021-17229-4 (2022). Article Google Scholar * Gbetibouo, G. A. & Hassan, R. M.
Measuring the economic impact of climate change on major South African fields crops: A Ricardian approach. _Global Planet. Change_ 47, 143–152.
https://doi.org/10.1016/j.gloplacha.2004.10.009 (2005). Article ADS Google Scholar * Adhikari, U., Nejadhashemi, A. P. & Woznicki, S. A. Climate change and Eastern Africa: A review of
impact on major crops. _Food Energy Secur._ 4, 110–132. https://doi.org/10.1002/fes3.61 (2015). Article Google Scholar * Ali, S. _et al._ Climate change and its impact on the yield of
major food crops: Evidence from Pakistan. _Foods_ 6, 39–57. https://doi.org/10.3390/foods6060039 (2017). Article PubMed PubMed Central Google Scholar * Knox, J., Hess, T., Daccache, A.
& Wheeler, T. Climate change impacts on crop productivity in Africa and South Asia. _Environ. Res. Lett._ 7, 034032. https://doi.org/10.1088/1748-9326/7/3/034032 (2012). Article ADS
Google Scholar * Nand, M. M., Barsdsley, D. K. & Suh, J. Addressing unavoidable climate change loss and damage: A case study from Fiji’s sugar industry. _Clim. Change_ 176, 21.
https://doi.org/10.1007/s10584-023-03482-8 (2023). Article ADS Google Scholar * Wheeler, T. & Von Braun, J. Climate change impacts on global food security. _Science_ 341, 508–513.
https://doi.org/10.1126/science.1239402 (2013). Article ADS CAS PubMed Google Scholar * Santos, L. R. _et al._ Energy cane yield simulated by the Dssat/Canegro model using climate
scenarios in Teotônio Vilela, AL, Brazil. _Rev. Bras. Ciênc. Agrár._ 16, 92. https://doi.org/10.5039/agraria.v16i4a92 (2021). Article Google Scholar * Mourice, S. K. Climate change will
intensify drought risk at the newly established Mkulazi II sugar estate, Mvomero district, Tanzania. _Sugar Tech._ 22, 157–170. https://doi.org/10.1007/s12355-019-00748-3 (2020). Article
CAS Google Scholar * Silva, W. K. M., Medeiros, S. E. L., Da Silva, L. P., Coelho Junior, L. M. & Abrahão, R. Sugarcane production and climate trends in Paraíba State (Brazil).
_Environ. Monit. Assess._ 192, 392. https://doi.org/10.1007/s10661-020-08358-3 (2020). Article CAS PubMed Google Scholar * Sonkar, G., Singh, N., Mall, R. K., Singh, K. K. & Gupta,
A. Simulating the impacts of climate change on sugarcane in diverse agro-climatic zones of Northern India using Canegro-sugarcane model. _Sugar Tech._ 22, 460–472.
https://doi.org/10.1007/s12355-019-00787-w (2020). Article CAS Google Scholar * Dias, H. B. & Sentelhas, P. C. Assigning the performance of two gridded weather data for sugarcane crop
simulations with a process-based model in center-South Brazil. _Int. J. Biometereol._ 65, 1881–1893. https://doi.org/10.1007/s00484-021-02145-6 (2021). Article ADS Google Scholar * Guga,
S. _et al._ Combining Maxent model and landscape pattern theory for analyzing interdecadal variation of sugarcane climate suitability in Guangxi, China. _Ecol. Indic._ 131, 108152.
https://doi.org/10.1016/j.ecolind.2021.108152 (2021). Article Google Scholar * Dias, H. B. _et al._ Traits for canopy development and light interception by twenty-seven Brazilian sugarcane
varieties. _Field Crops Res._ 249, 107716. https://doi.org/10.1016/j.fcr.2020.107716 (2020). Article Google Scholar * Linnenluecke, M. K., Zhou, C., Smith, T., Thompson, N. &
Nucifora, N. The impact of climate change on the Australian sugarcane industry. _J. Clean. Prod._ 246, 118974. https://doi.org/10.1016/j.jclepro.2019.118974 (2020). Article Google Scholar
* Verma, A. K., Garg, P. K., Prasad, K. S. H. & Dadhwal, V. K. Variety-specific sugarcane yield simulations and climate change impacts on sugarcane yield using DSSAT-CSM-CANEGRO model.
_Agric. Water Manag._ 275, 108034 (2023). Article Google Scholar * Aguilar-Rivera, N. Bioindicators for the sustainability of sugar agro-industry. _Sugar Tech._ 24, 651–661.
https://doi.org/10.1007/s12355-021-01105-z (2022). Article CAS PubMed PubMed Central Google Scholar * Ahmad, B. _et al._ Enhancement of sugarcane production by counteracting the adverse
effects of climate change in Sindh province. _Pakistan Growth Change_ 53, 76–90. https://doi.org/10.1111/grow.12561 (2022). Article Google Scholar * Abdoulaye, A. O., Lu, H., Zhu, Y.
& Hamoud, Y. A. Future water requirements of the main crops cultivated in the Niger river basin. _Atmosphere_ 12, 439–462. https://doi.org/10.3390/atmos12040439 (2021). Article ADS
Google Scholar * Singh, R. N. _et al._ Long-term spatiotemporal trends of temperature associated with sugarcane in west India. _Arab. J. Geosci._ 14, 1955.
https://doi.org/10.1007/s12517-021-08315-5 (2021). Article Google Scholar * Swami, D., Dave, P. & Parthasarathy, D. Analysis of temperature variability and extremes with respect to
crop threshold temperature for Maharashtra. _India. Theor. Appl. Climatol._ 144, 861–872. https://doi.org/10.1007/s00704-021-03558-4 (2021). Article ADS Google Scholar * Khan, A. U.,
Shah, A. H. & Iftikhar-Ul-Husnain, M. Impact of climate change on the net revenue of major crop growing farmers in Pakistan: A Ricardian approach. _Clim. Change Econ._ 12, 2150006.
https://doi.org/10.1142/S2010007821500068 (2021). Article Google Scholar * Akbar, H. & Gheewala, S. H. Effect of climate change on cash crops yield in Pakistan. _Arab. J. Geosci._ 13,
533. https://doi.org/10.1007/s12517-020-05333-7 (2020). Article Google Scholar * Mulinde, C. _et al._ Projected climate in coffee-based farming systems: Implications for crop suitability
in Uganda. _Reg. Environ. Sci._ 22, 1930. https://doi.org/10.1007/s10113-022-01930-2 (2022). Article Google Scholar * Silva, W. K. M., Freitas, G. P., Junior, L. M. C., Pinto, P. A. L. A.
& Abrahão, R. Effects of climate change on sugarcane production in the state of Paraíba (Brazil): A panel data approach (1990–2015). _Clim. Change_ 154, 195–209.
https://doi.org/10.1007/s10584-019-02424-7 (2019). Article ADS Google Scholar * Mcgree, S., Schreider, S., Kuleshov, Y. & Prakash, B. On the use of mean and extreme climate indices to
predict sugar yield in Western Fiji. _Weather Clim. Extrem._ 29, 100271. https://doi.org/10.1016/j.wace.2020.100271 (2020). Article Google Scholar * Kelkar, S. M., Kulkarni, A. & Rao,
K. K. Impact of climate change variability and change on crop production in Maharashtra. _India. Curr. Sci._ 118, 1235–1245. https://doi.org/10.18520/cs/v118/i8/1235-1245 (2020). Article
Google Scholar * Chandio, A. A. _et al._ Assessing the impacts of climate change on cereal production in Bangladesh: Evidence from ARDL modeling approach. _Int. J. Clim. Change Strat.
Manag._ 14, 125–147. https://doi.org/10.1108/IJCCSM-10-2020-0111 (2021). Article Google Scholar * Jyoti, B. & Singh, A. K. Projected sugarcane yield in different climate changes
scenarios in Indian states: A state-wise panel data exploration. _Int. J. Food Agric. Econ._ 8, 343–365. https://doi.org/10.22004/ag.econ.307654 (2020). Article Google Scholar * Heureux,
A. M. C. _et al._ Climate trends and extremes in the Indus river basin, Pakistan: Implications for agricultural production. _Atmosphere_ 13, 378–393. https://doi.org/10.3390/atmos13030378
(2022). Article ADS Google Scholar * Rehman, A., Ma, H., Ozturk, I. & Ahmad, M. I. Examining the carbon emissions and climate impacts on main agricultural crops production and land
use: Updated evidence from Pakistan. _Environ. Sci. Pollut. Res._ 29, 868–882. https://doi.org/10.1007/s11356-021-15481-2 (2022). Article CAS Google Scholar * Ncoyini, Z., Savage, M. J.
& Strydom, S. Limited access and use of climate information by small-scale sugarcane farmers in South Africa: A case study. _Clim. Serv._ 26, 100285.
https://doi.org/10.1016/j.cliser.2022.100285 (2022). Article Google Scholar * Kumar, K. K., Kumar, K. R., Ashrit, R. G., Desphande, N. R. & Hansen, J. W. Climate impacts on Indian
agriculture. _Int. J. Climatol._ 24, 1375–1393. https://doi.org/10.1002/joc.1081 (2004). Article Google Scholar * Ray, D. K. _et al._ Climate change has likely already affected global food
production. _Plos One_ 14, e0217148. https://doi.org/10.1371/journal.pone.0217148 (2019). Article CAS PubMed PubMed Central Google Scholar * Da Silva, G. J. _et al._ Aptitude of areas
planned for sugarcane cultivation expansions in the state of São Paulo, Brazil: A study based on climate change effects. _Agric. Ecosyst. Environ._ 305, 107164.
https://doi.org/10.1016/j.agee.2020.107164 (2021). Article CAS Google Scholar * Jaiswal, R. _et al._ Indian sugarcane under warming climate: A simulation study. _Eur. J. Agron._ 144,
126760. https://doi.org/10.1016/j.eja.2023.126760 (2023). Article Google Scholar * Yin, D., Yan, J., Li, F. & Song, T. Evaluation of global gridded crop models in simulation sugarcane
yield in China. _Atmos. Ocean. Sci. Lett._ 16, 100329. https://doi.org/10.1016/j.aosl.2023.100329 (2023). Article Google Scholar * Verma, R. R., Srivastava, T. K. & Singh, P. Climate
change impacts on rainfall and temperature in sugarcane growing upper Gangetic plains of India. _Theor. Appl. Climatol._ 135, 279–292. https://doi.org/10.1007/s00704-018-2378-8 (2019).
Article ADS Google Scholar * Sarker, A. R., Alam, K. & Gow, J. Performance of rain-fed Aman rice yield in Bangladesh in the presence of climate change. _Renew. Agric. Food Syst._ 34,
304–312. https://doi.org/10.1017/S1742170517000473 (2019). Article Google Scholar * IAC—(Agricultural Institute). _Novas Variedades de Cana Iac São Liberadas ao Setor Nesta Terça_, 23.
Available online: http://www.iac.sp.gov.br/noticiasdetalhes.php?pag=1&ano=2021&id=1436 Access on May 10 (2022). * CTC—(Sugarcane Tree Technological Center). _Plantio Com Variedades
Modernas Minimiza Impacto da Seca_. Available online https://cienciadacana.com.br/plantio-com-variedades-modernas-minimiza-impacto-da-seca/ Access on May 10 (2022). * Gazaffi, R. _et al._ RB
varieties: A major contribution to the sugarcane industry in Brazil. _Proc. Int. Soc. Sugarcane Technol._ 29, 1677–1682 (2016). Google Scholar * Machado, G. R. R. Jr. & Braga, R. L. C.
Jr. Sugarcane cultivars grown from 1991 to 2017 in the Brazilian industry. _Proc. Int. Soc. Sugarcane Technol._ 30, 118–120 (2019). Google Scholar * Rossetto, R. _et al._ Sustainability in
sugarcane supply chain in Brazil: Issues and way forward. _Sugar Tech._ 24, 941–966. https://doi.org/10.1007/s12355-022-01170-y (2022). Article CAS Google Scholar * Boschiero, B. N. _et
al._ Biomass yield, nutrient removal, and chemical composition of energy cane genotypes in southeast Brazil. _Indust. Crops Prod._ 191, 115993. https://doi.org/10.1016/j.indcrop.2022.115993
(2023). Article CAS Google Scholar * Dias, F. L. F., Rossetto, R., Landell, M. G. A. & Mendes, A. Silicon amendment in the productivity of four sugarcane varieties in Brazil. _Proc.
Int. Soc. Sugarcane Technol._ 27, 1–7 (2010). Google Scholar * Chapola, R. G. _et al._ Diversity of sugarcane cultivars under cultivation in São Paulo and Mato Grosso do Sul states 10 years
after the detection of orange rust in Brazil. _Proc. Int. Soc. Sugarcane Technol._ 30, 1743–1747 (2019). Google Scholar * Cheavegatti-Gianotto, A. _et al._ Development of CTC20BT, the
first genetically modified sugarcane commercially available in the world. _Proc. Int. Soc. Sugarcane Technol._ 30, 1272–1279 (2019). Google Scholar * INMET—(National Meteorological
Institute). _Annual Historical Data_. Available online: https://portal.inmet.gov.br/dadoshistoricos Access on March 02 (2023). * SEEG—(Greenhouse Gas Emissions and Removal Estimating
System). _Municipality Emission_. Available online https://plataforma.seeg.eco.br/cities/statistics Access on March 02 (2023). * Marengo, J. _et al._ A cold wave of winter 2021 in Central
South America: Characteristics and impacts. _Clim. Dyn._ https://doi.org/10.1007/s00382-023-06701-1 (2023). Article PubMed PubMed Central Google Scholar * CONAB—(National Supply
Company). _Sugarcane cost timeline 2008–2021_. Available online https://www.conab.gov.br/info-agro/custos-de-producao/planilhas-de-custo-de-producao/itemlist/category/800-cana-de-acucar.
Access April 24 (2022). * Muzammil, M., Zahid, A. & Breuer, L. Economic and environmental impact assessment of sustainable future irrigation practices in the Indus Basin of Pakistan.
_Sci. Rep._ 11, 23466. https://doi.org/10.1038/s41598-021-02913-9 (2021). Article ADS CAS PubMed PubMed Central Google Scholar * Ali, S., Zubair, M. & Hussain, S. The combined
effect of climatic factors and technical advancement on yield of sugarcane by using ARDL approach: evidence from Pakistan. _Environ. Sci. Pollut. Res._ 28, 39787–398.
https://doi.org/10.1007/s11356-021-13313-x (2021). Article Google Scholar * BRAZIL. Law n° 11,326, July 24, 2006. Determines the rules for the familiar agriculture and rural families
enterprise national plan. Official National Diary: Brasilia, p. 1. Available online http://www.planalto.gov.br/ccivil_03/_ato2004-2006/2006/lei/l11326.htm Accessed on May 12 (2022). *
Gujarati, D. N. & Porter, D. C. _Econometria Básica_ 5th edn. (AMGH, 2011). Google Scholar * Efron, B. & Tibshirani, R. J. _An Introduction to the Bootstrap_ (Chapman & Hall,
1993). Book MATH Google Scholar * Joshi, M., Seidel-Morgenstern, A. & Kremling, A. Exploiting the Bootstrap method for quantifying parameter confidence intervals in dynamical systems.
_Metab. Eng._ 8, 447–455. https://doi.org/10.1016/j.ymben.2006.04.003 (2006). Article CAS PubMed Google Scholar * Pienkowski, T., Keane, A., Kinyanda, E., Asiimwe, C. &
Milner-Gulland, E. J. Predicting the impacts of land management for sustainable development on depression risk in a Ugandan case study. _Sci. Rep._ 12, 11607.
https://doi.org/10.1038/s41598-022-14976-3 (2022). Article ADS CAS PubMed PubMed Central Google Scholar Download references FUNDING This work was supported by FAPEG (Research Support
Foundation in Goiás) and CAPES (Coordination for the Improvement of Higher Education Personnel). AUTHOR INFORMATION AUTHORS AND AFFILIATIONS * Pontifical Catholic University of Goiás,
Pontifical Catholic University of Goiás. Avenida Universitária, nº 1440, Área 3, Bloco D, 3ºandar, Setor Universitário, 74065-010, Goiânia, Goiás, Brasil Thiago Vizine Da Cruz & Ricardo
Luiz Machado Authors * Thiago Vizine Da Cruz View author publications You can also search for this author inPubMed Google Scholar * Ricardo Luiz Machado View author publications You can also
search for this author inPubMed Google Scholar CONTRIBUTIONS T.V.C.: Conceptualization, methodology, software, validation, formal analysis, investigation, data curation, writing—original
draft, writing—review & editing. R.L.M.: Conceptualization, methodology, validation, formal analysis, investigation, resources, writing-—review & editing, visualization, supervision,
project administration, fund acquisition. Neither the article nor portions of it have been previously published elsewhere. The manuscript is not under consideration for publication in
another journal, and will not be submitted elsewhere before the Scientific Reposts Journal editorial process is completed. All authors consent to the publication of the manuscript in the
Scientific Reports Journal, should the article be accepted by the Editor-in-chief upon competition of the refereeing process. CORRESPONDING AUTHOR Correspondence to Thiago Vizine Da Cruz.
ETHICS DECLARATIONS COMPETING INTERESTS The authors declare no competing interests. ADDITIONAL INFORMATION PUBLISHER'S NOTE Springer Nature remains neutral with regard to jurisdictional
claims in published maps and institutional affiliations. SUPPLEMENTARY INFORMATION SUPPLEMENTARY INFORMATION. RIGHTS AND PERMISSIONS OPEN ACCESS This article is licensed under a Creative
Commons Attribution 4.0 International License, which permits use, sharing, adaptation, distribution and reproduction in any medium or format, as long as you give appropriate credit to the
original author(s) and the source, provide a link to the Creative Commons licence, and indicate if changes were made. The images or other third party material in this article are included in
the article's Creative Commons licence, unless indicated otherwise in a credit line to the material. If material is not included in the article's Creative Commons licence and your
intended use is not permitted by statutory regulation or exceeds the permitted use, you will need to obtain permission directly from the copyright holder. To view a copy of this licence,
visit http://creativecommons.org/licenses/by/4.0/. Reprints and permissions ABOUT THIS ARTICLE CITE THIS ARTICLE Da Cruz, T.V., Machado, R.L. Measuring climate change’s impact on different
sugarcane varieties production in the South of Goiás. _Sci Rep_ 13, 11637 (2023). https://doi.org/10.1038/s41598-023-36582-7 Download citation * Received: 28 October 2022 * Accepted: 06 June
2023 * Published: 19 July 2023 * DOI: https://doi.org/10.1038/s41598-023-36582-7 SHARE THIS ARTICLE Anyone you share the following link with will be able to read this content: Get shareable
link Sorry, a shareable link is not currently available for this article. Copy to clipboard Provided by the Springer Nature SharedIt content-sharing initiative