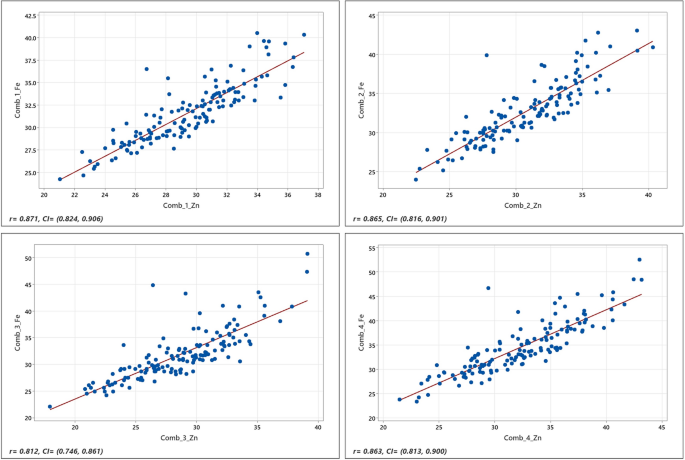
Genome-wide association study and expression of candidate genes for fe and zn concentration in sorghum grains
- Select a language for the TTS:
- UK English Female
- UK English Male
- US English Female
- US English Male
- Australian Female
- Australian Male
- Language selected: (auto detect) - EN
Play all audios:

ABSTRACT Sorghum germplasm showed grain Fe and Zn genetic variability, but a few varieties were biofortified with these minerals. This work contributes to narrowing this gap. Fe and Zn
concentrations along with 55,068 high-quality GBS SNP data from 140 sorghum accessions were used in this study. Both micronutrients exhibited good variability with respective ranges of
22.09–52.55 ppm and 17.92–43.16 ppm. Significant marker-trait associations were identified on chromosomes 1, 3, and 5. Two major effect SNPs (S01_72265728 and S05_58213541) explained 35% and
32% of Fe and Zn phenotypic variance, respectively. The SNP S01_72265728 was identified in the cytochrome P450 gene and showed a positive effect on Fe accumulation in the kernel, while
S05_58213541 was intergenic near Sobic.005G134800 (zinc-binding ribosomal protein) and showed negative effect on Zn. Tissue-specific in silico expression analysis resulted in higher levels
of Sobic.003G350800 gene product in several tissues such as leaf, root, flower, panicle, and stem. Sobic.005G188300 and Sobic.001G463800 were expressed moderately at grain maturity and
anthesis in leaf, root, panicle, and seed tissues. The candidate genes expressed in leaves, stems, and grains will be targeted to improve grain and stover quality. The haplotypes identified
will be useful in forward genetics breeding. SIMILAR CONTENT BEING VIEWED BY OTHERS GENOME-WIDE ASSOCIATION STUDY UNCOVERS GENOMIC REGIONS ASSOCIATED WITH GRAIN IRON, ZINC AND PROTEIN
CONTENT IN PEARL MILLET Article Open access 10 November 2020 GENETIC DISSECTION OF GRAIN IRON AND ZINC, AND THOUSAND KERNEL WEIGHT IN WHEAT (_TRITICUM AESTIVUM_ L.) USING GENOME-WIDE
ASSOCIATION STUDY Article Open access 20 July 2022 DISCOVERY AND VALIDATION OF CANDIDATE GENES FOR GRAIN IRON AND ZINC METABOLISM IN PEARL MILLET [_PENNISETUM GLAUCUM_ (L.) R. BR.] Article
Open access 06 October 2020 INTRODUCTION After a decades-long fall and five years of stability since 2014, the global Prevalence of Undernourishment (PoU) spiked during 2019 and 2020 due to
the COVID-19 pandemic1. In 2020, 21% of the population in Africa was hungry1. Asia's enormous population houses 54% of the world's hungry1. According to the World Health
Organization2,3,4, 149.2 million children under five were stunted, with diet-related deaths amounting to 45%, whereas 462 million adults were underweighting in 2014. African region tops this
critical situation with 56.2 million children, followed by 49.8 million in South-East Asia, and 22.9 million children in the Eastern Mediterranean region5. Malnutrition is the inadequate
consumption of micronutrients like iodine, vitamin A, iron, and zinc which affects worldwide public health2,6. Therefore, inadequate nutrition threatens the health and development of
populations worldwide, especially children and pregnant women in countries with lower income. The importance of iron (Fe) in human food cannot be overemphasized. Iron deficiency anemia is a
common type of anemia described as blood lacking adequate healthy red blood cells carrying oxygen to the body's tissues7,8,9. Anemia decreases physical and mental capabilities but is
rarely discovered, and when worsens it results in death. Anemia during pregnancy can impede growth and development due to preterm delivery, low-birth-weight infants, maternal death, and
reduced iron storage. In 2019, 29.9% of reproductive-age women (nearly half a billion women aged 15–49), 36.5% of pregnant women, and 39.8% of 6–59-month-olds (269 million children) had
anemia10. On the other hand, zinc, one of the most abundant trace elements, is the most widely distributed trace element in the body after iron. Zinc has a structural role in over 2500
transcription factors, is necessary for the activity of over 300 proteins structure and enzymes activity, and controls thousands of genes and gene expressions11,12. Zinc is important in DNA
synthesis, cell proliferation, protein synthesis, wound healing, and immune system support. It considerably impacts macrophages, neutrophils, and other complementary activity at the
microcellular level13,14,15. Two billion people are still at risk of zinc deficiency, with infants, toddlers, and pregnant and lactating women being the most vulnerable due to their higher
zinc requirements16,17. Zinc must be consumed or supplemented because the body cannot store it; its insufficiency is a global health issue, especially in developing nations like India,
Pakistan, Ethiopia, Indonesia, and Vietnam18. External nutrient supplementation and fortification are the most widely used methods to counter micronutrient malnutrition. Biofortified foods
can also be produced through plant breeding and/or agronomic practices designed to increase the density of vitamins and minerals in the crop of interest. It is now acknowledged that
developing high-nutrient cultivars is cheap, and making it affordable for low-income populations is a promising strategy to reduce micronutrient malnutrition. Sorghum is an ideal crop under
current and future climate change scenarios because it generates a good net return with low input and can feed a larger population, especially in semi-arid ecologies where wheat (_Triticum
aestivum_ L.), maize (_Zea mays_ L.), and rice (_Oryza sativa_) cannot be produced sustainably due to climate adversities, particularly high temperatures and increased drought stress19,20.
Sorghum is a staple food for more than 500 million people in Africa and Asia21. The estimated global sorghum consumption is expected to reach 26.5 million metric tons by the year 2026.
Nigeria emerged as the leading consumer of sorghum in 2021, consuming a staggering 5.7 million metric tons. India, China, and Ethiopia were next, with 3.9, 3.7, and 3.5 million metric tons,
respectively22. Due to its low glycemic index and high antioxidant content, sorghum grain is a popular gluten-free and celiac-safe diet23, and its consumption is expected to increase in
developed countries driven by not only its life-promoting properties but also by its cultivation as a substitute of major cereals in those parts of the world. Biofortifying sorghum using
conventional plant breeding and molecular technologies approaches can improve sorghum as a nutrient-dense and climate-resilient crop. Previous studies on grain Fe and Zn concentrations in
sorghum found significant genetic variability and strong heritability24,25. Madhusudhana et al.26 recently observed a highly significant G × E for Fe, but not for Zn. A significant G × E
indicates that a genotype can perform well in particular environments but not others. Data from several trials showed high broad sense heritability for sorghum grain Fe (> 85%) and Zn
concentrations (> 82%)27. Kumar et al.23 concluded that additive gene action controls Zn, and therefore high Zn lines can be developed by increasing the frequency of favorable alleles
through intercrosses and other population improvement strategies. Additive and non-additive gene actions were found to regulate Fe concentration23, implying that this trait can be improved
through heterosis breeding on the one hand, and crossing and selecting superior progeny, on the other. The highly significant and positive correlation between general combining ability (GCA)
and line performance per se suggested that parental genotype performance per se can predict the grain Fe and Zn concentrations in hybrids and breeding lines23. Although sorghum germplasm
has shown large variability and genetic heritability for iron and zinc content25, only a few Fe and Zn biofortified varieties have been released thus far28. Indeed, the first India’s
biofortified sorghum was released only two years ago, in 202229. This work is, therefore, a contribution to the identification of major quantitative traits loci (QTLs) and genes governing Fe
and Zn biofortification for use in breeding e.g., through introgressions in a sustained marker-assisted selection to create new biofortified varieties and improve the nutritional quality of
the existing cultivars. Recently, biparental mapping populations were used to identify QTLs for Fe and Zn in sorghum24. However, the QTLs found using this method were of low resolution. In
contrast, linkage disequilibrium (LD)-based association mapping (Genome-Wide Association Study) can boost mapping resolution by representing a more diverse gene pool and accounting for past
meiotic events. This technique was used in various previous research works to find markers non-randomly associated with the phenotype of interest in a broader process of forward genetics and
breeding. Girma et al.30, Enyew et al.31, Wondimu et al.32 carried out GWAS on sorghum agro-morphological traits, while other GWAS were successfully conducted on nutritional traits such as
polyphenol33,34, protein, fat, and starch35, mineral traits36, and biomass related traits37. Several genomic regions governing grain Fe and Zn have already been identified in other cereals
such as Pearl Millet38,39, Wheat40,41, Maize42, Barley43, and Rice44. However, no reports on identifying SNPs and genes controlling grain Fe and Zn in sorghum are available. This work was
therefore undertaken to close this gap; it is the first to report the marker traits associations (MTAs) for grain Fe and Zn in sorghum using SNPs and minicore lines. We aimed at identifying
SNPs associated with grain Fe and Zn concentration in the sorghum kernel, mining underlying candidate genes using a part of the ICRISAT’s minicore set of sorghum collection, and implementing
several GWAS models. ICRISAT’s genebank is the repository of the largest sorghum germplasm collection i.e., over 42,880 accessions from over 94 countries worldwide
(http://genebank.icrisat.org/). The mini core, a gateway to the germplasm, is highly genetically diverse and is an efficient option for carrying out association mapping and allele mining for
traits of interest45. The outcome of this work is, therefore, expected to benefit the scientific community across the globe. MATERIALS AND METHODS MULTI-ENVIRONMENT FIELD TRIALS AND PLANT
MATERIALS The experimental materials for the current study consisted of 140 diverse germplasm accessions representing a part of a sorghum minicore maintained at the International Crops
Research for the Semi-Arid Tropics (ICRISAT, Patancheru), from 58 different countries (Supplementary Table S1). These germplasm accessions were evaluated for grain Fe and Zn content (ppm) in
post-rainy seasons of two consecutive years across two locations. The seasons included post rainy 2020 (PR 2020) and post rainy 2021 (PR 2021), while the locations were the International
Crops Research Institute for the Semi-Arid Tropics (ICRISAT, Patancheru) (17.53°N, 78.27°E) located at an altitude of 545 m above mean sea level and Vasantrao Naik Marathwada Krishi
Vidyapeeth (VNMKV, Parbhani) (18.45°N, 76.13°E) located at an altitude of 357 m above mean sea level. Soil testing analysis using the DTPA method for the experiment fields at Parbhani was
done at the Department of Soil Science, COA, Parbhani, while, for ICRISAT fields it was done at Charles Renard Analytical Laboratory, ICRISAT, Patancheru. The available Fe and Zn content in
our research field was beyond the minimum required levels (4.50 to 6.50 mg kg−1 Fe and 0.6 to 0.9 mg kg−1 Zn)46 and ample for normal growth and development of plants. Soil type at Parbhani
was deep and black whereas, at ICRISAT it was shallow and light black. Irrigation was provided at each important crop stages to grow healthy plants including the grains development stage.
The experiments were conducted in the post-rainy season to ensure the good quality of the seed. The experimental design was alpha lattice with two replications. Each germplasm was grown in a
single row of 4 m long at both locations with inter and intra-row spacing of 60 × 15 cm. Standard agronomic practices were followed for successful crop development in each season.
PHENOTYPING AND ESTIMATION OF FE AND ZN CONCENTRATION IN THE GRAINS The panicles were harvested to measure the Fe and Zn content from the grains as they reached the physiological maturity
stage. The panicles from five random plants from each plot were selfed before the flowering stage to obtain pure seed. Upon maturity, these panicles were harvested and stored separately in a
dry cloth bag to produce clean grain samples for micronutrient analysis. The harvested panicles underwent a 7-day sun drying to achieve a post-harvest grain moisture level of 10–12%,
essential for mitigating fungal infections. Threshing was meticulously conducted in cloth bags to prevent sample contamination by extraneous particles. Subsequently, the seeds underwent
cleaning to eliminate glumes, anthers, dust, and other contaminants. A composite seed sample weighing approximately 30 g was then collected for each plot. Analysis of grain Fe and Zn content
was performed using X-ray fluorescence spectrometry (XRF) (Make: Hitachi High-Tech, Japan; model: X-Supreme8000), a calibrated, efficient, non-destructive, and cost-effective analytical
method. STATISTICAL ANALYSES A combined analysis of variance (ANOVA) was performed to assess the main and interaction effects of Environment (E) and Genotype (G), considering E, G, and
replications as random effects. The individual variance of environments was modelled using the Residual Maximum Likelihood (REML) procedure using SAS Mixed procedure using SAS v9.447. Best
Linear Unbiased Predictors (BLUPs) were estimated using below equation: $${Y}_{ijkl}=M+{E}_{i}+{R(E)}_{j(i)}+{G}_{k}+{GE}_{jk}+{e}_{ijkl}$$ where \({Y}_{ijkl}, M, {E}_{i}, {R(E)}_{j(i)},
{G}_{k}, {GE}_{jk},\text{ and }{e}_{ijkl}\), respectively, stand for the measurement on plot \(l\) in environment \(i\), block \(j\), containing genotype \(k\), the overall mean of all plots
in all environments, the effect of environment (trial) \(i\), the effect of replicate \(j\) within environment \(i\), the effect of genotype \(k\), the interaction of genotype \(k\) with
environment \(i\), the plot residual. The heritability was estimated as repeatability48 using the formula: $$H^{2} = \frac{{\sigma_{g}^{2} }}{{\sigma_{g}^{2} + \left(
{{\raise0.7ex\hbox{${\sigma_{gxe}^{2} }$} \!\mathord{\left/ {\vphantom {{\sigma_{gxe}^{2} } E}}\right.\kern-0pt} \!\lower0.7ex\hbox{$E$}}} \right) + \left(
{{\raise0.7ex\hbox{${\sigma_{e}^{2} }$} \!\mathord{\left/ {\vphantom {{\sigma_{e}^{2} } {r*E}}}\right.\kern-0pt} \!\lower0.7ex\hbox{${r*E}$}}} \right)}}$$ where \({\sigma }_{g}^{2}, {\sigma
}_{gxe}^{2}, and {\sigma }_{e}^{2}\) are genetic variance component, genotype × environment variance component and residual variance respectively; \(E\) and \(r\) are the number of
environments and replications, respectively. The four best combinations of environments were selected for phenotypic data processing in this study, based upon the relatively high
repeatability (Table 1) from the combined analysis of variance. All combinations of the environments are presented in Supplementary Table S2. ISOLATION OF DNA AND GENOTYPING Genomic DNA from
the single plant of sorghum minicore samples was isolated from 30 days old seedlings using the QIAGEN DNAeasy 96 plant kit. Purity and quantity of the extracted DNA was determined using gel
electrophoresis and a Qubit 2.0 Fluorometer (Life Technologies, Carlsbad, CA) respectively and finally diluted to 30 ng/µl. Genotype-by-sequencing (GBS) libraries were prepared using the
restriction enzyme ApeK1 according to Elshire et al.49. SNPs were called using the TASSEL v5.2 GBS pipeline50 against the _S. bicolor_ BTx623 reference genome v3.1.1 (www.phytozome.net)51
using Bowtie v2.5.152 with default parameters. Raw SNPs were called from the 140 genotypes and subsequently filtered to retain bi-allelic markers with < 40% missing data as suggested in
Ali et al.53, maximum of 20% of heterozygosity and a minimum of 5% minor allele frequency (MAF). The retained high-quality SNPs were used in this work for genetic diversity, population
structure and marker-traits association analyses (MTAs). The MTAs were performed using R GAPIT 3 pipeline54. POPULATION STRUCTURE The high-quality SNPs were used to determine the genetic
distance between the sorghum accessions using R “amap” and “labdsv” packages. The phylogeny analysis was performed with the Euclidean method with 1000 bootstrap replications with R
"ape" package55 and the neighbor-joining tree was visualized in iTOL tree viewer56. The Principal Coordinates Analysis (PCo) between the accessions was measured with R
"labdsv" package (https://CRAN.R-project.org/package=labdsv). The admixture analysis was performed with ADMIXTURE 1.3.057 with expected K in the range of 2 to 15 and the K with the
lowest cross-validation error considered as optimal sub-populations. The genome-wide Linkage Disequilibrium (LD) was generated using the r2 values calculated with TASSEL v5.250. ASSOCIATION
MAPPING We performed GWAS with multiple models viz., MLMM, SUPER, BLINK and FarmCPU. Manhattan and Quantile–Quantile (Q–Q) plots were visualized in RStudio58 using GAPIT 3 package54. The
spurious associations in GWAS were corrected using Bonferroni Correction (5% level of significance)59 and significant MTAs, corresponding to putative QTLs for the studied traits, were
determined by the _P-value_ less than 0.05/m, with m being the number of markers60. In the present investigation, Bonferroni correction value is calculated at 9.07 × 10–5. Further, the
percentage of phenotypic variance explained (PVE) by all significant SNPs was generated either by GAPIT 3 built-in algorithms or calculated in RStudio as the squared correlation between the
phenotype (BLUPs) and genotype of the SNP accounting for the above-described populations of environments. The Linux bash and _in-house_ developed script was used to find the haplotype blocks
and to identify the promising germplasms exhibiting high Fe and Zn content from the sorghum population. CANDIDATE GENE IDENTIFICATION AND IN SILICO GENE EXPRESSION ANALYSIS Single
nucleotide polymorphisms (SNPs) explaining more than 7.5% of the phenotypic variance of their associated traits were identified and their genomic regions were further analyzed in the process
of functional GWAS and candidate gene identification. To perform functional GWAS, an interval of 51.77 Kb upstream and downstream the SNP position was considered, based on a genome-wide
linkage disequilibrium (LD) decay cut-off at r2 = 0.1. Annotation details for genes and respective Gene Ontologies (GO) within each region were retrieved using the Phytomine interface
implemented in Phytozome61. JGI Plant Gene Atlas (https://plantgeneatlas.jgi.doe.gov/)62 was used to check the tissue-specific expression of candidate genes and to access the expression data
for 36 tissues at juvenile, vegetative, and reproductive stages. Gene expression was in the unit of Fragments per kilobase of transcripts per million mapped reads (FPKM). The expression
heatmap was generated using TBtools II63. ETHICS STATEMENT This research does not involve the ethics of human and animal experiments. INSTITUTIONAL, NATIONAL, AND INTERNATIONAL GUIDELINES
AND LEGISLATION STATEMENT The seeds of sorghum used in this work were collected from ICRISAT’s Genebank (http://genebank.icrisat.org/). The authors confirm that all methods applied in this
work were performed in accordance with the relevant guidelines/regulations/legislation. RESULTS STATISTICAL ANALYSES The repeatability i.e., test–retest reliability, resulted relatively
highest (56%) for Fe in combination 4, followed by combinations 1, 3 and 2 with 46, 45 and 41%, respectively. Similarly for Zn the highest repeatability values (74%) were observed in
combination 4 followed by combinations 1, 3 and 2 with 66, 64 and 57%, respectively, as shown in Table 1. The combined ANOVA showed significant differences among the genotypes and a
significant genotype × environment for both Fe and Zn traits (Table 2). As depicted in Table 2 and in Fig. 1, the concentration of Fe and Zn varied over a wide range, and the correlation
between the two micronutrients was high (r > 0.80). The frequency distributions of the phenotypic data followed a normal distribution, as suggested by Shapiro—Wilks normality test64; and
Ryan-Joiner statistics65 (Fig. 2). GENETIC DIVERSITY, POPULATION STRUCTURE, AND LINKAGE DISEQUILIBRIUM (LD) We retained 55,068 high quality SNPs that were well distributed across the genome
(Fig. 3) with a density of ~ 5505 markers per chromosome (Chr) and around 80 markers per Mb region. Genomic regions with high marker density were observed on Chr 01 and Chr 02 with an
average magnitude of 91 markers per Mb on both chromosomes. Chr 06 has averaged 86 markers per 1 Mb region with high-density regions between 47 and 52 Mb. Chr 08 and 09 also showed
high-density regions between 57–63 Mb and 55–74 Mb, respectively. Linkage-disequilibrium decay (LD decay) is determined using the entire set of markers. The LD decay plot was plotted as LD
(r2) against the distance in base pairs (bp). The overall LD decay across the genome estimated at 51.77 kb (Fig. 4a). The unweighted unrooted neighbor-joining tree (Fig. 4b) used to depict
the phylogenetic diversity showed that the genotypes clustered based on the races, but also revealed a significant level of admixtures (Fig. 4b). A similar clustering was confirmed using the
PCA with the first 3 PCs explaining 39.13%, 19.27% and 11.20% genetic variation (GVE), respectively (Fig. 4c), which gives a total of 70% GVE. The optimum population size estimation using
the ADMIXTURE model also gave K = 4 as the point with the lowest cross-validation error (CV error) (Fig. 4d). MARKER-TRAIT ASSOCIATIONS A genome-wide association analysis was undertaken to
use the whole-genome high-quality 55,068 marker information, high-repeatability phenotypic data, and four single-locus (SUPER) and multi-locus (BLINK, MLMM, FarmCPU) algorithms, and evaluate
the linkage disequilibrium (MTAs) that existed between genetic variations and nutritional traits viz., grain Fe and Zn concentration in the panel of 140 sorghum genotypes. For both Fe and
Zn traits, five MTAs were detected, each, by BLINK and MLMM GWAS models, whereas FarmCPU and SUPER, each, detected two. A threshold of MAF (≥ 0.05) was set to account for the limitations of
GWAS66 and to correct for spurious MTAs. Table 3 displays a comprehensive compilation of significant markers that showed significant effects. The Manhattan plots (Fig. 5) showcased the GWAS
output, portraying where these highly promising markers assert their statistical significance amidst the vast complexity of the genome. Additionally, these markers were carefully selected
based on their elevated values in the Quantile-Quantile (QQ) plots (Fig. 5), indicating their non-random association with the traits of interest. We identified 5 consistent MTAs for Zn and 4
for Fe that were revealed by at least one model in at least one trial combination (Table 3; Fig. 5). Three of the 5 MTAs for Zn were detected using the statistically stringent MLMM
model54,67 while the remaining 2 were detected using the SUPER model. All Zn MTAs were detected for trial combination 2, except one (S05_58213541), that was detected for trial combinations 1
and 2. The highest PVE reported for Zn was 31.8% (Table 3). The strongest MTA (S01_72265728) reported for Fe was detected by 3 models including the most stringent, MLMM (Table 3). The same
MTA was also detected across trial combinations 2 and 4, and was just 1.5 Mb downstream to a Zn MTA locus detected by marker S01_73777110 (Table 3). The highest PVE for this Fe MTA was
34.7%. The other MTAs for Fe were detected by BLINK (S03_73164578, S04_43148417, S05_67287071) and FarmCPU (S05_67287071), all of which had the highest PVE at 14.7%. Supplementary Table S3
depicts further information about significant MTAs along with MAF, effects, genes, and the functional annotation. CANDIDATE GENES ASSOCIATED WITH GRAIN FE AND ZN Candidate genes for each of
the MTAs were identified within the window of LD block (51.77 kb upstream–downstream) as defined by the LD decay estimation (Supplementary Table S4; Fig. 4a). Moreover, Table 4 provides a
summary of all significant markers that were identified to be localized within genes, and the corresponding annotations. A total of 5 genes were identified for both traits studied (Table 4).
Two significant and genic SNPs associated with Fe were linked with Sobic.001G445900 and Sobic.005G188300 genes which play a significant role in Cytochrome P450, heme binding, iron ion
binding, oxidoreductase activity, and monooxygenase activity. The genes are putatively involved in other processes including acting on paired donors with incorporation or reduction of
molecular oxygen in dhurrin biosynthetic process, jasmonic acid mediated signaling pathway, leaf shaping response to brassinosteroid sterol, and metabolic process unidimensional cell growth.
Similarly, three significant SNPs associated with Zn were located in Sobic.001G017500, Sobic.001G463800 and Sobic.003G350800 genes which are putatively associated several properties
including NAD(P)-binding domain, Glucose/ribitol dehydrogenase, NADPH-cytochrome P450 reductase, peptidase S8/S53 domain-containing protein and malate dehydrogenase acting in
deoxyribonucleoside triphosphate catabolic process, nucleoside triphosphate catabolic process, nucleotide metabolic process, NADH metabolic process, carbon fixation, photosynthesis,
magnesium ion binding and metal ion binding. HAPLOTYPE BLOCK ANALYSIS In the analysis of trait-associated markers, an adjacent set of five markers was utilized to assess the haplotype block
for each marker. This approach resulted in an average block size of 109 kb for iron (Fe) trait markers and 66 kb for zinc (Zn) trait markers. The analysis identified a total of eleven
accessions exhibiting promising characteristics for Fe traits, and fifteen accessions demonstrating favorable attributes for Zn traits. Notably, five accessions viz., IS22294, IS29239,
IS22239, IS29565, and IS23514 were found to possess haplotypes blocks of markers associated with both Fe and Zn traits (Fig. 6). Additionally, based on the Zn haploblock analysis, three
accessions (IS22239, IS21512, and IS19676) demonstrated unique pattern of haplotypes indicating that these are related and have the strong variation in Block 1. EXPRESSION ANALYSIS The
tissue-specific expression of the identified genes was analyzed in 36 sorghum tissues at three different growth stages of the plant (Supplementary Table S5, Fig. 7). Based on the FPKM
values, an expression heatmap was generated, plotting the expression data of five candidate genes across 36 sorghum tissues (Supplementary Table S5, Fig. 7). Out of five genes,
Sobic.003G350800 which is a peptidase S8/S53 domain-containing protein, showed higher level of expression in several tissues such as leaf, root, flower, panicle, and stem. Conversely,
Sobic.001G017500 is not expressed in any of the tissues. Sobic.005G188300 and Sobic.001G463800 genes are expressed moderately at grain maturity and anthesis stages in leaf, root, panicle,
and seed tissues. Sobic.001G445900 gene showed high expression at juvenile and grain maturity stage. DISCUSSION Iron (Fe) and zinc (Zn) are essential micronutrients for both human and animal
nutrition, and their importance in sorghum lies in their role in promoting overall health and preventing nutrient deficiencies, also known as hidden hunger. In addition to preventing
micronutrient malnutrition, these micronutrients are essential for cognitive development, immune system function, agricultural productivity, and socio-economic development. Hence,
biofortified sorghum provides a readily available and affordable source of essential micronutrients, preventing and alleviating micronutrient deficiencies and preserving traditional food
culture. It preserves traditional food preferences, promotes economic development, and contributes to climate resilience as sorghum is a C4 crop with high level of resource use efficiency.
Few biofortified sorghum varieties were released under the world’s drylands and this gap motivated our research work; we aimed to identify the genomic regions associated with grain Fe and Zn
for downstream use in sorghum biofortification breeding. Several GWAS studies has identified the genes governing complex traits, revolutionizing agricultural improvement. Research on pulses
and grains revealed genes related to the accumulation of micronutrients, including the pathway for the carotenoid production in maize68. Additionally, wheat displayed 92 SNP trait
correlations linked to 10-grain mineral areas. Twenty genes were functionally annotated to demonstrate their significance in grain mineral accumulation; most of these genes were found in the
D-genome, indicating control over wheat grain mineral diversity69. The genetic architecture of seed molybdenum and selenium in wild and cultivated chickpea was investigated using GWAS70.
After 180 entries were surveyed, 16 SNPs were linked to these characteristics. Similarly, 22 quantitative trait nucleotides (QTNs) for grain nitrogen were found by phenotyping 174 accessions
of Croatian common bean land races for the seed concentrations of eight micronutrients71. In our study, using sorghum entries from the ICRISAT’s minicore provided a guarantee that the
uncovered major QTLs and proxy SNPs would benefit wider research communities. To the best of our knowledge, this work is the first to report on the marker traits associations for grain Fe
and Zn in sorghum using SNPs and minicore lines. In this study, the analysis of variance (ANOVA) was carried out for both the characters viz. grain Fe and grain Zn over four combinations of
environments. The result indicates highly significant differences among the genotypes for both traits, which revealed the existence of sufficient variation for effective GWAS, statistical
inferences, and selection of superior plant ideotypes. Despite the study's small population size, the identified MTAs were significant and agreed with previous results, indicating that
the population size did not pose severe data quality challenges33,72,73. This study demonstrated that GWAS is a powerful tool for identifying potential genetic factors that contribute to
important traits in sorghum genotypes, even with a small sample size. The repeatability for different combinations was varied, the highest was measured for Fe and Zn (56% and 74%,
respectively) in combination 4, whereas low values were registered in combination 2 i.e., 41% and 57% for Fe and Zn, respectively. The genetic basis of traits like grain Fe and Zn content
can be complex, involving multiple genes with small effects24, and this can explain the observed moderate repeatability. The observed medium to high broad-sense heritability/repeatability
and wide variation within population for the evaluated micronutrients are the precondition for a successful GWAS74,75,76. The association between grain Fe and Zn showed significant and high
positive values and the trend was similar across environments, implying that selection for either mineral can be used as proxy for the other. Such a favorable relationship between these
micronutrients were reported earlier in sorghum77,78,79,80 and other cereals, such as pearl millet81,82,83,84,85,86, maize87,88, rice89,90, and wheat91,92,93,94. Research on sorghum grain Fe
and Zn so far could only identify a few small effect QTLs and some putative candidate genes24, without meaningful use in sorghum genetic biofortification. In this study we used multiple
popular GWAS models i.e., MLMM, BLINK, FarmCPU and SUPER33,37; the latter is a single locus model, while the remaining three are multi-locus. The use of multiple models allowed to detect
more reliable QTLs i.e., those that were co-detected by more than one model95. On the other hand, although multi-locus GWAS models showed advantage over single-locus GWAS methods96, a
combination of single-locus methods and multi-locus methods was used in this work as recommended by97,98,99,100 to improve the detection power and robustness of GWAS. The FarmCPU method
offers superior statistical power by dividing Multiple Loci Linear Mixed Models into fixed effects model (FEM) and random effects model (REM), removing confounding, and controlling false
positives; the SUPER model addresses computing issues with MLM, while BLINK improves statistical power with using LD information33,54. Structure analyses showed that the minicore population
was genetically structured with an estimated four subpopulations, and corrective measures were implemented in the GWAS to account for population structure and cryptic relationships to avoid
false positive associations33,101,102. With the use of GBS SNP data, we identified nine highly significant MTAs for grain Fe and Zn with P values ranging from 3.88 × 10–12 to 5.19 × 10–7,
explaining 7.5 to 34.7% of phenotypic variation (PVE). Four MTAs for Fe were identified on chromosomes 01, 03, 04 and 05 while five MTAs for Zn were identified on chromosomes 01, 02, 03 and
05. Two major SNPs were identified for Fe and Zn: for iron, the SNP S01_72265728 was identified in the cytochrome P450 gene, while the SNP S05_58213541 associated with Zn is intergenic and
near Sobic.005G134800 (2.8 kb) which codes for a zinc binding ribosomal protein. S01_72265728 was associated with positive effect on Fe accumulation in the kernel, while S05_58213541 was
associated with negative effect on Zn accumulation. Many other differentially expressed genes are involved in the uptake of minerals. In the case of the gene for Fe, the Cytochrome P450 gene
is involved in mineral uptake for both Fe and Zn. Cytochrome P450 is a pigment with heme-protein properties and participates in several catalytic processes that involve specific heme
group103. Cytochrome P450s catalyze a wide range of chemical reactions and have different enzymatic mechanisms and complex substrate specificities. The enzyme structures of different P450
possess a heme-binding mode with an unusually long heme-binding loop and a unique I-helix which may involved in Fe uptake from the soil104. A study on barley collection identified
single-nucleotide polymorphisms for Fe and Zn biofortification in which cytochrome P450 superfamily protein was found to be involved in element transport, iron, and zinc binding43. Plant
cytochrome P450 (P450) participates in a wide range of biosynthetic reactions and targets a variety of biological molecules105. However, there is no specific information on how cytochrome
P450 gene is involved in Fe and Zn uptake. Satyavathi et al.106 identified a cytochrome P450 superfamily protein involved in element transport, iron, and zinc binding, and found out that it
was present in the genotypes with high Fe and Zn contents. A study on rice biofortification with zinc and selenium found that the expression pattern of a Cytochrome P450 (CYP) gene followed
the mineral accumulation in flag leaves107. Such mixed reports suggests that more research is needed to fully understand the function of cytochrome P450 gene in mineral uptake. In pearl
millet, it was reported that the cytochrome P450 proteins are up-regulated during panicle initiation105. The process of iron uptake by plants is an extremely energy-intensive mechanism108. A
plant's ability to extract iron from the complex or chelating molecule by reducing Fe+++ to Fe + + is essential for iron absorption108. Uptake of Fe from the soil is dependent on
other cations in the soil solution such as manganese (Mn) and calcium (Ca). Accumulation of Fe in grain is a very complex process and many factors such as polyphenol content and other
stresses play a significant role in Fe concentration in the grain. In another study found that drought stress alters iron accumulation in sorghum seeds, and photosynthesis impaired by
drought stress might trigger a disturbance in iron homeostasis109. The same authors hypothesized the that increased vacuolar transporters and ferritin might be involved in the regulation of
iron accumulation in sorghum seeds under drought stress. Zinc-binding proteins are involved in abiotic stress tolerance and play a significant role in root hair growth under stress
conditions. It was reported that the metal binding proteins facilitates the absorption of Zn and metal ions by procuring the binding sites110 but in our study, the identified SNP
(S05_58213541) showed a negative effect which could mean that there is an association between that SNP and a particular trait, but the presence of a specific allele at that SNP locus is
associated with a decrease or reduction in the trait of interest, Zn concentration in this case. This can indicate that either the plant absorbed the Zn ions from the soil and sequester it
but unable to translocate it to the grains, or the plant with that particular SNP was unable to uptake enough Zn form the soil. Whether this is a direct effect (modified gene product) or a
non-allelic interaction, it is to be investigated. This gene can also be a candidate for genome editing in order to improve Zn concentration in the kernel. If the associated gene is knocked
out, there may be chances of significantly increasing the concentration of Zn in grains. Most putative genes identified in this study are zinc-binding or zinc ion-binding proteins. The gene
ontology CCHH term indicates the presence of transcripts involved in metal ion binding activity, indicating their role in uptake and transport of Fe and Zn. A zinc finger is a small protein
structural motif characterized by the coordination of one or more zinc ions (Zn2 +) to stabilize the fold. Zinc finger proteins are transcription factors with the finger domain, which plays
a significant role in gene regulation and are required for transcriptional activation. Based on the results, among the four combinations of locations, combination 2 consisting of three
locations identified the most MTAs. The identified candidate genomic regions/candidate genes are likely to have an important role in achieving high Fe and Zn content in sorghum grains. The
identified SNPs can therefore be validated and used in developing nutritionally improved, Fe and Zn rich sorghum cultivars, which would help address micronutrient deficiencies and increase
food security. The correlation between Fe and Zn concentration in the sorghum grain was high (r > 0.80) but we could not come across pleiotropic QTLs (SNPs) in this study. The lack of
common SNPs in this study may indicates that the effects of the genetic factors that influence Fe and Zn concentrations may vary depending on the environmental conditions. Indeed, a
correlation of 0.80 implies that 36% of the variance in sorghum grain Fe or Zn concentration cannot be explained by neither of the two metals. Haplotype-based breeding has recently come to
prominence as an effective approach to developing crop varieties that meet particular requirements111. To be used in breeding programs, this breeding strategy needs to first determine
superior haplotypes. Haplotypes are distinct sets of alleles found on a single chromosome that are inherited together with a limited probability of contemporary recombination112. Breeders
can increase the accuracy of genomic predictions and breeding strategies by more effectively defining haplotypes using linkage disequilibrium-based techniques and haplotype diversity113,114.
Recently, researchers explained the genetic diversity and evolutionary background of sorghum accessions by identifying several haplotypes of genes such as _Dry_115 and _Sh1_116 in
domesticated and wild sorghum lines. Furthermore, Wu et al.117 conducted a population genetic study that demonstrated the _Sh1_ and _SbTB1_ regions were subject to strong selection during
the domestication of sorghum. Moreover, it has resulted in the potential role of _SbTB1_ haplotype in controlling the number of lateral branches in sorghum in domesticated and wild
accessions. To the best of our knowledge, there is no specific study that directly addresses haplotype analysis in grain Fe and Zn in sorghum, and this is the first study to report
haplotypes for grain Fe and Zn. In the present investigation, the haplotype analysis used sets of five markers to evaluate haplotype blocks, resulting in average block sizes of 109 kb for
iron (Fe) trait markers and 66 kb for zinc (Zn) trait markers. It identified eleven accessions with promising Fe traits and fifteen with favorable Zn traits. The identified haploblocks were
further used to identify sorghum accessions that inherited iron and/or zinc QTLs stably and possibly with rare crossing-over events. Notably, five accessions possessed haplotype blocks
associated with both Fe and Zn traits. Furthermore, three accessions showed unique haplotype patterns related to strong variation in Block 1 based on Zn haploblock analysis. Thus, it will be
beneficial to: (1) target the identified haploblock-containing plants as potential QTL donors in sorghum crossing blocks, and (2) tailor plant architecture and enhance grain nutrient
content by incorporating these haplotypes through haplotype-based breeding in sorghum breeding programs. In this work, the baseline expression profiles of the identified genes were shown
using heat map. The gene Sobic.003G350800 encoding for a protein which contains the peptidase S8/S53 domain, was found to be highly expressed in a variety of tissues, including the leaf,
root, flower, panicle, and stem. Nearly all plant species include peptidase S8/S53 domain subtilases, which are subtilisin-like proteases that regulate a variety of biotic and abiotic
stressors, and are expressed in a variety of tissues. _Sorghum bicolor_ contains 57 genes belonging to the S8 family118. Subtilase family members exhibit remarkable functional versatility,
from protein expression to stress responses, and modulating plant growth and development from seed development to senescence119. Genes, Sobic.005G188300 and Sobic.001G463800 are expressed
moderately at grain maturity and anthesis stages in leaf, root, panicle, and seed tissues. These genes mainly encode for malate dehydrogenase (MDH: EC 1.1.1.82) in C4 plants. The functional
annotation of Sobic.001G463800 showed its role in iron ion binding activity, which is probably one of the factors determining the success of biofortification, nutrient bioavailability at
different plant growth stages, from soil to plant tissues. In nature, the synthesis of malate is catalyzed by the MDH enzyme through the reversible reduction of oxaloacetate to malate. As
such, MDH is therefore indirectly but strongly associated with iron and other nutrients uptake in plants. Indeed, Malate is a key product of plant metabolism with diverse functional roles in
plants120,121, including, but not limited to: respiration and energy generation, photosynthesis (both C3 and C4), fatty acid oxidation, lignin biosynthesis, pulvinal and stomatal function,
nitrogen (N2) fixation and amino acid biosynthesis, ion balance, uptake of phosphorus (P) and iron (Fe), and aluminum (Al) tolerance121,122,123. Cultivar differences, such as low nutrient
mobility and remobilization efficiency, also affect the effectiveness of biofortification, particularly in relation to leaves and edible parts124. The identified candidate genes showing high
micronutrients expression in leaves, stem, and grains at different growth stages (juvenile, vegetative, and reproductive stages) can be targeted to increase the nutritional value of both
grain and stover, providing health-promoting food, feed, and forage. Overall, this study provides invaluable information on the genetic basis of grain Fe and Zn contents in sorghum and
identifies candidate genes and genomic regions that can be used in breeding programs to improve these micronutrients. However, more research works are needed to further characterize these
genomic regions and candidate genes and to validate their role in grain Fe and Zn concentration in sorghum grain before they are implemented in sorghum breeding programs. DATA AVAILABILITY
The datasets created during and/or analyzed during the current investigation are not publicly available, although the corresponding author can provide them upon justifiable request.
REFERENCES * FAO. World Food and Agriculture—Statistical Yearbook 2021. Rome (2021). https://doi.org/10.4060/cb4477en. * WHO. Malnutrition.
https://www.who.int/news-room/fact-sheets/detail/malnutrition (2021a). Accessed 4 Sept 2023. * WHO. Children: improving survival and well-being.
https://www.who.int/news-room/fact-sheets/detail/children-reducing-mortality#:~:text=Malnourished%20children%2C%20particularly%20those%20with,under%2D5%20years%20of%20age (2020). Accessed 21
Aug 2023. * WHO. _World Health Statistics 2022: Monitoring Health for the SDGs, Sustainable Development Goals_. Geneva: World Health Organization; 2022. Licence: CC BY-NC-SA 3.0 IGO. ISBN
978-92-4-005114-0 (2022). * WHO. _World Health Statistics 2023: Monitoring Health for the SDGs, Sustainable Development Goals_. Geneva: World Health Organization; 2023. Licence: CC BY-NC-SA
3.0 IGO (2023a). * Ingle, K. P. _et al._ Biofortified foxtail millet: towards a more nourishing future. _Plant Growth Regul._ 99, 25–34. https://doi.org/10.1007/s10725-022-00900-2 (2023).
Article CAS Google Scholar * Abbaspour, N., Hurrell, R. & Kelishadi, R. Review on iron and its importance for human health. _J. Res. Med. Sci._ 19(2), 164–174 (2014). PubMed PubMed
Central Google Scholar * Piskin, E., Cianciosi, D., Gulec, S., Tomas, M. & Capanoglu, E. Iron absorption: factors, limitations, and improvement methods. _ACS Omega_ 7(24), 20441–20456.
https://doi.org/10.1021/acsomega.2c01833 (2022). Article CAS PubMed PubMed Central Google Scholar * WHO. Anaemia. Available from:
https://www.who.int/news-room/fact-sheets/detail/anaemia. Accessed 25th Sept 2023 (2023b). * WHO. Anaemia in women and children.
https://www.who.int/data/gho/data/themes/topics/anaemia_in_women_and_children#:~:text=In%202019%2C%20global%20anaemia%20prevalence,39.1%25)%20in%20pregnant%20women (2021b). Accessed 04th
Sept. 2023. * Saper, R. B. & Rash, R. Zinc: an essential micronutrient. _Am. Fam. Physician_ 79(9), 768–772 (2009). PubMed PubMed Central Google Scholar * McClung, J. P. Iron, zinc,
and physical performance. _Biol. Trace Elem. Res._ 188, 135–139. https://doi.org/10.1007/s12011-018-1479-7 (2019). Article CAS PubMed Google Scholar * Fallah, A., Mohammad-Hasani, A.
& Colagar, A. H. Zinc is an essential element for male fertility: A review of Zn roles in men’s health germination, sperm quality, and fertilization. _J. Reprod. Infertil._ 19(2), 69–81
(2018). PubMed PubMed Central Google Scholar * Sanna, A., Firinu, D., Zavattari, P. & Valera, P. Zinc status and autoimmunity: A systematic review and meta-analysis. _Nutrients_
10(1), 68. https://doi.org/10.3390/nu10010068 (2018). Article CAS PubMed PubMed Central Google Scholar * Skalny, A. V., Aschner, M. & Tinkov, A. A. Zinc. _Adv. Food Nutr. Res._ 96,
251–310. https://doi.org/10.1016/bs.afnr.2021.01.003 (2021). Article CAS PubMed PubMed Central Google Scholar * Hambidge, K. M. & Krebs, N. F. Zinc deficiency: A special challenge.
_J. Nutr._ 137(4), 1101–1105. https://doi.org/10.1093/jn/137.4.1101 (2007). Article CAS PubMed Google Scholar * Hussain, A. _et al._ Mechanistic impact of zinc deficiency in human
development. _Front. Nutr._ 9, 717064. https://doi.org/10.3389/fnut.2022.717064 (2022). Article CAS PubMed PubMed Central Google Scholar * Narváez-Caicedo, C., Moreano, G., Sandoval, B.
A. & Jara-Palacios, M. Á. Zinc deficiency among lactating mothers from a peri-urban community of the Ecuadorian andean region: An initial approach to the need of zinc supplementation.
_Nutrients_ 10(7), 869. https://doi.org/10.3390/nu10070869 (2018). Article CAS PubMed PubMed Central Google Scholar * DeFries, R. _et al._ Climate resilience of dry season cereals in
India. _Sci. Rep._ 13, 9960. https://doi.org/10.1038/s41598-023-37109-w (2023). Article ADS CAS PubMed PubMed Central Google Scholar * Aruna, C. & Madhusudhana, R. Breeding for
yield and adaptation. In _Sorghum in the 21st Century: Food—Fodder—Feed—Fuel for a Rapidly Changing World_ (eds Tonapi, V. A. _et al._) (Springer, Singapore, 2020).
https://doi.org/10.1007/978-981-15-8249-3_15. Chapter Google Scholar * Khoddami, A. _et al._ Sorghum in foods: Functionality and potential in innovative products. _Crit. Rev. Food Sci.
Nutr._ 63(9), 1170–1186. https://doi.org/10.1080/10408398.2021.1960793 (2023). Article CAS PubMed Google Scholar * Sorghum Market Outlook 2022—2026. (2022. Global Sorghum Trends in 2022.
https://www.reportlinker.com/clp/global/482866#:~:text=Global%20Sorghum%20Trends%20in%202022&text=Global%20consumption%20of%20sorghum%20is,with%205.7%20million%20metric%20tons * Kumar,
A. A., _et al_. Recent advances in sorghum biofortification research. In _Plant Breeding Reviews_ (ed. by Jules, J.), Vol. 39. https://doi.org/10.1002/9781119107743.ch3 (2015). * Kotla, A.
_et al._ Identification of QTLs and candidate genes for high grain Fe and Zn concentration in sorghum [_Sorghum bicolor_ (L.) Moench]. _J. Cereal Sci._
https://doi.org/10.1016/j.jcs.2019.102850 (2019). Article Google Scholar * Ashok Kumar, A., Reddy, B. V. S., Ramaiah, B., Sahrawat, K. L. & Pfeiffer, W. H. Genetic variability and
character association for grain iron and zinc contents in sorghum germplasm accessions and commercial cultivars. _Eur. J. Plant Sci. Biotech._ 6(Special Issue 1), 66–70 (2012). Google
Scholar * Madhusudhana, R. _et al._ Genetic variability, G × E interaction and stability for iron and zinc content in sorghum grains in advanced breeding lines. _J. Cereal Sci._
https://doi.org/10.1016/j.jcs.2023.103653 (2023). Article Google Scholar * Hariprasanna, K., Agte, V. & Patil, J. V. Genotype × environment interactions for grain micronutrient
contents in sorghum [_Sorghum bicolor_ (L.) Moench]. _Indian J. Genet. Plant Breed._ 72(4), 429–434. https://doi.org/10.5958/j.0975-6906.74.1.006 (2012). Article CAS Google Scholar *
Garg, M. _et al._ Biofortified crops generated by breeding, agronomy, and transgenic approaches are improving lives of millions of people around the world. _Front. Nutr._ 5, 12.
https://doi.org/10.3389/fnut.2018.00012 (2018). Article CAS PubMed PubMed Central Google Scholar * Gaddameedi, A. _et al._ The location of iron and zinc in grain of conventional and
biofortified lines of sorghum. _J. Cereal Sci._ https://doi.org/10.1016/j.jcs.2022.103531 (2022). Article Google Scholar * Girma, G. _et al._ A large-scale genome-wide association analyses
of Ethiopian sorghum landrace collection reveal loci associated with important traits. _Front. Plant Sci._ https://doi.org/10.3389/fpls.2019.00691 (2019). Article PubMed PubMed Central
Google Scholar * Enyew, M. _et al._ Genome-wide analyses using multi-locus models revealed marker-trait associations for major agronomic traits in _Sorghum bicolor_. _Front. Plant Sci._
https://doi.org/10.3389/fpls.2022.999692 (2022). Article PubMed PubMed Central Google Scholar * Wondimu, Z., Dong, H., Paterson, A. H., Worku, W. & Bantte, K. Genome-wide association
study reveals genomic loci influencing agronomic traits in Ethiopian sorghum (_Sorghum bicolor_ (L.) Moench) landraces. _Mol. Breed._ https://doi.org/10.1007/s11032-023-01381-5 (2023).
Article PubMed PubMed Central Google Scholar * Habyarimana, E., Dall’Agata, M., De Franceschi, P. & Baloch, F. S. Genome-wide association mapping of total antioxidant capacity,
phenols, tannins, and flavonoids in a panel of _Sorghum bicolor_ and _S. bicolor_ × _S. halepense_ populations using multi-locus models. _PLoS ONE_ 14(12), e0225979.
https://doi.org/10.1371/journal.pone.0225979 (2019). Article CAS PubMed PubMed Central Google Scholar * Rhodes, D. H. _et al._ Genome-wide association study of grain polyphenol
concentrations in global sorghum [_Sorghum bicolor_ (L.) Moench] germplasm. _J. Agric. Food Chem._ 62(45), 10916–10927. https://doi.org/10.1021/jf503651t (2014). Article CAS PubMed Google
Scholar * Rhodes, D. H. _et al._ Genetic architecture of kernel composition in global sorghum germplasm. _BMC Genom._ https://doi.org/10.1186/s12864-016-3403-x (2017). Article Google
Scholar * Shakoor, N. _et al._ Integration of experiments across diverse environments identifies the genetic determinants of variation in sorghum bicolor seed element composition. _Plant
Phys._ 170(4), 1989–1998. https://doi.org/10.1104/pp.15.01971 (2016). Article CAS Google Scholar * Habyarimana, E., De Franceschi, P., Ercisli, S., Baloch, F. S. & Dall’Agata, M.
Genome-wide association study for biomass related traits in a panel of _Sorghum bicolor_ and _S. bicolor_ × _S. halepense_ populations. _Front. Plant Sci._ 11, 551305.
https://doi.org/10.3389/fpls.2020.551305 (2020). Article PubMed PubMed Central Google Scholar * Pujar, M. _et al._ Genome-wide association study uncovers genomic regions associated with
grain iron, zinc and protein content in pearl millet. _Sci. Rep._ https://doi.org/10.1038/s41598-020-76230-y (2020). Article PubMed PubMed Central Google Scholar * Anuradha, N. _et al._
Deciphering genomic regions for high grain iron and zinc content using association mapping in pearl millet. _Front. Plant Sci._ 8, 412. https://doi.org/10.3389/fpls.2017.00412 (2017).
Article CAS PubMed PubMed Central Google Scholar * Zhou, Z. _et al._ Identification of novel genomic regions and superior alleles associated with Zn accumulation in wheat using a
genome-wide association analysis method. _Int. J. Mol. Sci._ https://doi.org/10.3390/ijms21061928 (2020). Article PubMed PubMed Central Google Scholar * Krishnappa, G. _et al._
Identification of novel genomic regions for biofortification traits using an SNP marker-enriched linkage map in wheat (_Triticum aestivum_ L.). _Front. Nutr._
https://doi.org/10.3389/fnut.2021.669444 (2021). Article PubMed PubMed Central Google Scholar * Hindu, V. _et al._ Identification and validation of genomic regions influencing kernel
zinc and iron in maize. _Theo. Appl. Genet._ 131, 1443–1457. https://doi.org/10.1007/s00122-018-3089-3 (2018). Article CAS Google Scholar * Nyiraguhirwa, S. _et al._ A genome-wide
association study identifying single-nucleotide polymorphisms for iron and zinc biofortification in a worldwide barley collection. _Plants_ 11(10), 1349.
https://doi.org/10.3390/plants11101349 (2022). Article CAS PubMed PubMed Central Google Scholar * Talukdar, P. _et al._ Identification of genomic loci regulating grain iron content in
aus rice under two irrigation management systems. _Food Energy Secur._ https://doi.org/10.1002/fes3.329 (2022). Article PubMed Google Scholar * Upadhyaya, H. D., Vetriventhan, M. &
Deshpande, S. Sorghum germplasm resources characterization and trait mapping. In _The Sorghum Genome. Compendium of Plant Genomes_ (eds Rakshit, S. & Wang, Y. H.) (Springer, Cham, 2016).
Google Scholar * Shukla, A. K. _et al._ Deficiency of phyto-available sulphur, zinc, boron, iron, copper and manganese in soils of India. _Sci. Rep._ 11, 19760.
https://doi.org/10.1038/s41598-021-99040-2 (2021). Article ADS CAS PubMed PubMed Central Google Scholar * SAS Institute Inc. _SAS/STAT® 15.1 User’s Guide_ (SAS Institute Inc, Cary,
2018). Google Scholar * Falconer, D. S. _Introduction to Quantitative Genetics_ 3rd edn. (Longman, New York, 1989). Google Scholar * Elshire, R. J. _et al._ A robust, simple
Genotyping-by-Sequencing (GBS) approach for high diversity species. _PLoS ONE_ 6(5), e19379. https://doi.org/10.1371/journal.pone.0019379 (2011). Article ADS CAS PubMed PubMed Central
Google Scholar * Bradbury, P. J. _et al._ TASSEL: Software for association mapping of complex traits in diverse samples. _Bioinformatics_ https://doi.org/10.1093/bioinformatics/btm308
(2007). Article PubMed Google Scholar * McCormick, R. F. _et al._ The Sorghum bicolor reference genome: Improved assembly and annotations, a transcriptome atlas, and signatures of genome
organization. _Plant J._ 93(2), 338–354. https://doi.org/10.1111/tpj.13781 (2017). Article CAS PubMed Google Scholar * Langmead, B. & Salzberg, S. L. Fast gapped-read alignment with
Bowtie 2. _Nat. Methods_ 9, 357–359. https://doi.org/10.1038/nmeth.1923 (2012). Article CAS PubMed PubMed Central Google Scholar * Ali, M., Zhang, Y. Rasheed, A. Wang, J. & Zhang,
L. Genomic prediction for grain yield and yield-related traits in Chinese winter wheat. _Int. J. Mol. Sci._ 21(4) 1342. https://doi.org/10.3390/ijms21041342 (2020). * Wang, J. & Zhang,
Z. GAPIT version 3: Boosting power and accuracy for genomic association and prediction. _Geno. Proteom. Bioinform._ 19(4), 629–640. https://doi.org/10.1016/j.gpb.2021.08.005 (2021). Article
Google Scholar * Paradis, E., Claude, J. & Strimmer, K. APE: Analyses of phylogenetics and evolution in R language. _Bioinformatics_ 20, 289–290.
https://doi.org/10.1093/bioinformatics/btg412 (2004). Article CAS PubMed Google Scholar * Letunic, I. & Bork, P. Interactive Tree of Life (iTOL) v4: Recent updates and new
developments. _Nucl. Acids Res._ https://doi.org/10.1093/nar/gkz239 (2019). Article PubMed PubMed Central Google Scholar * Alexander, D. H., Novembre, J. & Lange, K. Fast model-based
estimation of ancestry in unrelated individuals. _Genome Res._ https://doi.org/10.1101/gr.094052.109 (2009). Article PubMed PubMed Central Google Scholar * RStudio Team. _RStudio:
Integrated Development for R. RStudio_ (PBC, Boston, MA, 2020). http://www.rstudio.com/ * Kuo, K. H. M. Multiple testing in the context of gene discovery in sickle cell disease using
genome-wide association studies. _Genom. Insights_ https://doi.org/10.1177/1178631017721178 (2017). Article Google Scholar * Xu, Y. _et al._ Genome-wide association mapping of starch
pasting properties in maize using single-locus and multi-locus models. _Front. Plant Sci._ 9, 1311. https://doi.org/10.3389/fpls.2018.01311 (2018). Article PubMed PubMed Central Google
Scholar * Goodstein, D. M. _et al._ Phytozome: a comparative platform for green plant genomics. _Nucleic Acids Res._ 40, D1178–D1186. https://doi.org/10.1093/nar/gkr944 (2012). Article CAS
PubMed Google Scholar * Sreedasyam, A. _et al._ JGI Plant Gene Atlas: An updateable transcriptome resource to improve functional gene descriptions across the plant kingdom. _Nucleic
Acids Res._ https://doi.org/10.1093/nar/gkad616 (2023). Article PubMed PubMed Central Google Scholar * Chen, C. _et al._ TBtools-II: A “One for All, All for One” bioinformatics platform
for biological big-data mining. _Molec. Plant._ https://doi.org/10.1016/j.molp.2023.09.010 (2023). Article Google Scholar * Shapiro, S. S. & Wilk, M. B. An analysis of variance test
for normality (complete samples). _Biometrika_ 52(3–4), 591–611. https://doi.org/10.1093/biomet/52.3-4.591 (1965). Article MathSciNet Google Scholar * Ryan, T. A. & Joiner, B. L.
_Normal Probability Plots and Tests for Normality_ (Technical Report, Statistics Department, The Pennsylvania State University, 1976). * Chouraki, V. & Seshadri, S. Genetics of
Alzheimer’s Disease. In _Advances in Genetics_ (eds by Friedmann, T., Dunlap, J. C., & Goodwin, S. F.) 245–294. https://doi.org/10.1016/b978-0-12-800149-3.00005-6 (2014). * Alemu, A. _et
al._ Multi-model genome-wide association and genomic prediction analysis of 16 agronomic, physiological and quality related traits in ICARDA spring wheat. _Euphytica_ 217, 205.
https://doi.org/10.1007/s10681-021-02933-6 (2021). Article CAS Google Scholar * Suwarno, W. B., Pixley, K. V., Palacios-Rojas, N., Kaeppler, S. M. & Babu, R. Genome-wide association
analysis reveals new targets for carotenoid biofortification in maize. _Theor. Appl. Genet._ 128, 851–864. https://doi.org/10.1007/s00122-015-2475-3 (2015). Article CAS PubMed PubMed
Central Google Scholar * Bhatta, M. _et al._ Genome-wide association study reveals novel genomic regions associated with 10 grain minerals in synthetic hexaploid wheat. _Int. J. Mol. Sci._
19(10), 3237. https://doi.org/10.3390/ijms19103237 (2018). Article CAS PubMed PubMed Central Google Scholar * Özkuru, E. et al. Genome-wide association studies of molybdenum and
selenium concentrations in _C. arietinum_ and _C. reticulatum_ seeds. _Mol. Breed._, 39(3). https://doi.org/10.1007/s11032-019-0947-x (2019). * Gunjača, J. _et al._ Genome-wide association
studies of mineral content in common bean. _Front. Plant Sci._ https://doi.org/10.3389/fpls.2021.636484 (2021). Article PubMed PubMed Central Google Scholar * Lee, Y. _et al._
Genome-wide association study (GWAS) of the agronomic traits and phenolic content in sorghum (_Sorghum bicolor_ L.) genotypes. _Agronomy_ 13(6), 1449.
https://doi.org/10.3390/agronomy13061449 (2023). Article CAS Google Scholar * Kawahigashi, H., Kasuga, S., Okuizumi, H., Hiradate, S. & Yonemaru, J. Evaluation of Brix and sugar
content in stem juice from sorghum varieties. _Grassl. Sci._ 59(1), 11–19. https://doi.org/10.1111/grs.12006 (2013). Article CAS Google Scholar * Wang, J., Yu, J., Lipka, A. E. &
Zhang, Z. Interpretation of Manhattan plots and other outputs of genome-wide association studies. In _Genome-Wide Association Studies. Methods in Molecular Biology_ Vol. 2481 (eds
Torkamaneh, D. & Belzile, F.) (Humana, New York, 2022). https://doi.org/10.1007/978-1-0716-2237-7_5. Chapter Google Scholar * Yoosefzadeh-Najafabadi, M., Eskandari, M., Belzile, F.
& Torkamaneh, D. Genome-wide association study statistical models: A review. In _Genome-Wide Association Studies Methods in Molecular Biology_ Vol. 2481 (eds Torkamaneh, D. &
Belzile, F.) (Humana, New York, 2022). https://doi.org/10.1007/978-1-0716-2237-7_4. Chapter Google Scholar * Abed, A. & Kehel, Z. Preparation and curation of multiyear, multilocation,
multitrait datasets. In _Genome-Wide Association Studies. Methods in Molecular Biology_ Vol. 2481 (eds Torkamaneh, D. & Belzile, F.) (Humana, New York, 2022).
https://doi.org/10.1007/978-1-0716-2237-7_6. Chapter Google Scholar * Ashok Kumar, A., Anuradha, K. & Ramaiah, B. Increasing grain Fe and Zn concentration in sorghum: progress and way
forward. _J. SAT Agric. Res._ 11, 1–5 (2013). Google Scholar * Reddy, B. V. S., Ramesh, S. & Longvah, T. Prospects of breeding for micronutrients and β-carotene-dense sorghums. _Intern.
Sorghum Millets Newsl._ 46, 10–14 (2005). Google Scholar * Reddy, P. S. _et al._ Association of grain Fe and Zn contents with agronomic traits in sorghum. _Indian J. Plant Genet. Res._ 23,
280–284 (2010). Google Scholar * Nguni, D., Geleta, M., Hofvander, P., Fatih, M. & Bryngelsson, T. Comparative genetic diversity and nutritional quality variation among some important
Southern African sorghum accessions [_Sorghum bicolor_ (L.) Moench]. _Aust. J. Crop Sci._ 6, 56–64 (2012). CAS Google Scholar * Velu, G. _et al._ Prospects of breeding biofortified pearl
millet with high grain iron and zinc content. _Plant Breed._ 126, 182–185. https://doi.org/10.1111/j.1439-0523.2007.01322.x (2007). Article CAS Google Scholar * Velu, G., Rai, K. N.,
Sahrawat, K. L. & Sumalini, K. Variability for grain iron and zinc contents in pearl millet hybrids. _J. SAT Agric. Res._ 6, 1–4 (2008). Google Scholar * Gupta, S. K., Velu, G., Rai, K.
N. & Sumalini, K. Association of grain iron and zinc content with grain yield and other traits in pearl millet (_Pennisetum glaucum_ (L.) R. Br.). _Crop Improv._ 36, 4–7 (2009). Google
Scholar * Rai, K. N., Govindaraj, M. & Rao, A. S. Genetic enhancement of grain iron and zinc content in pearl millet. _Qual. Assur. Saf. Crops Foods._ 4, 119–125.
https://doi.org/10.1111/j.1757-837X.2012.00135.x (2012). Article CAS Google Scholar * Govindaraj, M. _et al._ Combining ability and heterosis for grain iron and zinc densities in pearl
millet. _Crop Sci._ 53, 507–517. https://doi.org/10.2135/cropsci2012.08.0477 (2013). Article CAS Google Scholar * Kanatti, A. _et al._ Grain iron and zinc density in pearl millet:
combining ability, heterosis and association with grain yield and grain size. _SpringerPlus_ 3, 763. https://doi.org/10.1186/2193-1801-3-763 (2014). Article CAS PubMed PubMed Central
Google Scholar * Oikeh, S. O., Menkir, A., Maziya-Dixon, B., Welch, R. & Glahn, R. P. Assessment of concentrations of iron and zinc and bioavailable iron in grains of early maturing
tropical maize varieties. _J. Agric. Food Chem._ 51, 3688–3694. https://doi.org/10.1021/jf0261708 (2003). Article CAS PubMed Google Scholar * Oikeh, S. O. _et al._ Environmental
stability of iron and zinc concentrations in grain of elite early-maturing tropical maize genotypes grown under field conditions. _J. Agric. Sci._ 142, 543–551.
https://doi.org/10.1017/S0021859604004733 (2004). Article CAS Google Scholar * Stangoulis, J. C. R., Huynh, B. L., Welch, R. M., Choi, E. Y. & Graham, R. D. Quantitative trait loci
for phytate in rice grain and their relationship with grain micronutrient content. _Euphytica_ 154, 289–294. https://doi.org/10.1007/s10681-006-9211-7 (2007). Article Google Scholar *
Anandan, A., Rajiv, G., Eswaran, R. & Prakash, M. Genotypic variation and relationships between quality traits and trace elements in traditional and improved rice (_Oryza sativa_ L.)
genotypes. _J. Food Sci._ 76(4), H122–H130. https://doi.org/10.1111/j.1750-3841.2011.02135.x (2011). Article CAS PubMed Google Scholar * Garvin, D. F., Welch, R. M. & Finley, J. W.
Historical shifts in the seed mineral micronutrient concentration of US hard red winter wheat germplasm. _J. Sci. Food Agric._ 86, 2213–2220. https://doi.org/10.1002/jsfa.2601 (2006).
Article CAS Google Scholar * Peleg, Z. _et al._ Quantitative trait loci conferring grain mineral nutrient concentrations in durum wheat × wild emmer wheat RIL population. _Theo. Appl.
Genet._ 119, 353–369. https://doi.org/10.1007/s00122-009-1044-z (2009). Article CAS Google Scholar * Zhang, Y. _et al._ Mineral element concentrations in grains of Chinese wheat
cultivars. _Euphytica_ 174, 303–313. https://doi.org/10.1007/s10681-009-0082-6 (2010). Article Google Scholar * Velu, G., Ortiz-Monasterio, I., Singh, R. P. & Payne, T. Variation for
grain micronutrients in wheat core collections accession of diverse origin. _Asian J. Crop Sci._ 3, 43–48. https://doi.org/10.3923/ajcs.2011.43.48 (2011). Article Google Scholar * Yu, K.
_et al._ Genome-wide association studies reveal novel QTLs, QTL-by environment interactions and their candidate genes for tocopherol content in soybean seed. _Front. Plant Sci._ 13, 1026581.
https://doi.org/10.3389/fpls.2022.1026581 (2022). Article PubMed PubMed Central Google Scholar * Zhang, Y. M., Jia, Z. & Dunwell, J. M. Editorial: The applications of new
multi-locus GWAS methodologies in the genetic dissection of complex traits. _Front. Plant Sci._ 10, 100. https://doi.org/10.3389/fpls.2019.00100 (2019). Article PubMed PubMed Central
Google Scholar * Chang, M., He, L. & Cai, L. An overview of genome-wide association studies. In _Computational Systems Biology Methods in Molecular Biology_ Vol. 1754 (ed. Huang, T.)
(Humana Press, New York, 2018). https://doi.org/10.1007/978-1-4939-7717-8_6. Chapter Google Scholar * He, L. _et al._ Evaluation of genomic prediction for pasmo resistance in flax. _Int.
Mol. Sci._ 20, E359. https://doi.org/10.3390/ijms20020359 (2019). Article CAS Google Scholar * Li, M. _et al._ Enrichment of statistical power for genome-wide association studies. _BMC
Biol._ 12, 73. https://doi.org/10.1186/s12915-014-0073-5 (2014). Article CAS PubMed PubMed Central Google Scholar * Li, C. X. _et al._ Molecular marker assisted breeding and genome
composition analysis of Zhengmai 7698, an elite winter wheat cultivar. _Sci. Rep._ 8, 322. https://doi.org/10.1038/s41598-017-18726-8 (2018). Article ADS CAS PubMed PubMed Central
Google Scholar * Sul, J. H., Martin, L. S. & Eskin, E. Population structure in genetic studies: Confounding factors and mixed models. _PLoS Genet._
https://doi.org/10.1371/journal.pgen.1007309 (2018). Article PubMed PubMed Central Google Scholar * Yuan, Y. _et al._ Genome-wide association mapping and genomic prediction analyses
reveal the genetic architecture of grain yield and flowering time under drought and heat stress conditions in maize. _Front. Plant Sci._ 9, 1919. https://doi.org/10.3389/fpls.2018.01919
(2019). Article PubMed PubMed Central Google Scholar * Chakraborty, P., Biswas, A., Dey, S., Bhattacharjee, T. & Chakrabarty, S. Cytochrome P450 gene families: Role in plant
secondary metabolites production and plant defense. _J. Xenobiot._ 13(3), 402–423. https://doi.org/10.3390/jox13030026 (2023). Article CAS PubMed PubMed Central Google Scholar * Li, L.,
Chang, Z., Pan, Z., Fu, Z. & Wang, X. Modes of heme binding and substrate access for cytochrome P450 CYP74A revealed by crystal structures of allene oxide synthase. _Proc. Nat. Acad.
Sci. U. S. A._ 105(37), 13883–13888. https://doi.org/10.1073/pnas.0804099105 (2008). Article ADS Google Scholar * Sun, K. _et al._ Genome-wide analysis of the cytochrome P450 gene family
involved in salt tolerance in _Gossypium hirsutum_. _Front. Plant Sci._ 12, 685054. https://doi.org/10.3389/fpls.2021.685054 (2021). Article PubMed PubMed Central Google Scholar *
Satyavathi, C. T. _et al._ Stage specific comparative transcriptomic analysis to reveal gene networks regulating iron and zinc content in pearl millet [_Pennisetum glaucum_ (L.) R. Br.].
_Sci. Rep._ 12, 276. https://doi.org/10.1038/s41598-021-04388-0 (2022). Article ADS CAS PubMed PubMed Central Google Scholar * Roda, F. A. _et al._ Rice biofortification with zinc and
selenium: A transcriptomic approach to understand mineral accumulation in flag leaves. _Front. Genet._ 11, 543. https://doi.org/10.3389/fgene.2020.00543 (2020). Article CAS PubMed PubMed
Central Google Scholar * Hochmuth, G. Iron (Fe) nutrition of plants: SL353/SS555, 8/2011. EDIS 2011 (8) https://doi.org/10.32473/edis-ss555-2011 (2011). * Araki, R., Takano, Y., Miyazaki,
H., Ii, H. & An, P. Drought stress alters iron accumulation in _Sorghum bicolor_ seeds. _Environ. Exp. Bot._ 204, 105093. https://doi.org/10.1016/j.envexpbot.2022.105093 (2022). Article
CAS Google Scholar * Shabbir, Z. _et al._ Copper uptake, essentiality, toxicity, detoxification and risk assessment in soil-plant environment. _Chemosphere_ 259, 127436.
https://doi.org/10.1016/j.chemosphere.2020.127436 (2020). Article CAS PubMed Google Scholar * Varshney, R. K. _et al._ Designing future crops: Genomics-assisted breeding comes of age.
_Trends Plant Sci._ 26(6), 631–649. https://doi.org/10.1016/j.tplants.2021.03.010 (2021). Article CAS PubMed Google Scholar * Sehgal, D. _et al._ Haplotype-based, genome-wide association
study reveals stable genomic regions for grain yield in CIMMYT spring bread wheat. _Front. Gen._ https://doi.org/10.3389/fgene.2020.589490 (2020). Article Google Scholar * Bhat, J. A. _et
al._ Identification of superior haplotypes in a diverse natural population for breeding desirable plant height in soybean. _Theor. Appl. Genet._ 135(7), 2407–2422.
https://doi.org/10.1007/s00122-022-04120-0 (2022). Article CAS PubMed PubMed Central Google Scholar * Meuwissen, T. H., Odegard, J., Andersen-Ranberg, I. & Grindflek, E. On the
distance of genetic relationships and the accuracy of genomic prediction in pig breeding. _Genet. Sel. Evol._ 46, 1–8. https://doi.org/10.1186/1297-9686-46-49 (2014). Article Google Scholar
* Zhang, L. M. _et al._ Sweet sorghum originated through selection of _Dry_, a plant-specific NAC transcription factor gene. _Plant Cell._ 30(10), 2286–2307.
https://doi.org/10.1105/tpc.18.00313 (2018). Article CAS PubMed PubMed Central Google Scholar * Lin, Z. _et al._ Parallel domestication of the _Shattering1_ genes in cereals. _Nat
Genet_ 44(6), 720–724. https://doi.org/10.1038/ng.2281 (2012). Article CAS PubMed PubMed Central Google Scholar * Wu, X. _et al._ Genomic footprints of sorghum domestication and
breeding selection for multiple end uses. _Mol. Plant_ 15(3), 537–551. https://doi.org/10.1016/j.molp.2022.01.002 (2022). Article CAS PubMed Google Scholar * Galotta, M. F., &
Roberts, I. N. A method for in vivo determination of subtilase activity in germinating seeds. _J. Cereal Sci,_ 85, 105–110 https://doi.org/10.1016/j.jcs.2018.11.014 (2019). * Schaller, A.
_et al._ From structure to function—a family portrait of plant subtilases. _New Phytolol._ 218(3), 901–915. https://doi.org/10.1111/nph.14582 (2017). Article Google Scholar * Lance, C.
& Rustin, P. The central role of malate in plant metabolism. _Physiol. Vég._ 22, 625–641 (1984). CAS Google Scholar * Martinoia, E. & Rentsch, D. Malate compartmentation—responses
to a complex metabolism. _Annu. Rev. Plant Physiol. Plant Mol. Biol._ 45, 447–467 (1994). Article CAS Google Scholar * Gietl, C. Malate dehydrogenase isoenzymes: Cellular locations and
role in the flow of metabolites between cytoplasm and cell organelles. _Biochem. Biophys. Acta_ 1100, 217–234 (1992). CAS PubMed Google Scholar * Kochian, L. V. Cellular mechanisms of
aluminum toxicity and resistance in plants. _Annu. Rev. Plant Physiol. Plant Mol. Biol._ 46, 237–260 (1995). Article CAS Google Scholar * Ebbisa, A. Mechanisms underlying cereal/legume
intercropping as nature-based biofortification: A review. _Food Prod. Process. Nutr._ 4, 19. https://doi.org/10.1186/s43014-022-00096-y (2022). Article CAS Google Scholar Download
references ACKNOWLEDGEMENTS Niranjan Ravindra Thakur acknowledges the funding support for this research from the International Crops Research Institute for the Semi-Arid Tropics, Patancheru,
India (ICRISAT). The authors thank Dr. Kamalakar Kamble and Mr. Jayakumar Jaganathan for their help in executing and managing the trials, and phenotypic data curation. AUTHOR INFORMATION
AUTHORS AND AFFILIATIONS * International Crops Research Institute for the Semi-Arid Tropics, Patancheru, Telangana, India Niranjan Ravindra Thakur, Sunita Gorthy, AnilKumar Vemula, Damaris
A. Odeny, Pradeep Ruperao, Pramod Ramchandra Sargar & Ephrem Habyarimana * Vasantrao Naik Marathwada Agriculture University, Parbhani, Maharashtra, India Niranjan Ravindra Thakur, Pramod
Ramchandra Sargar, Shivaji Pandurang Mehtre & Hirakant V. Kalpande Authors * Niranjan Ravindra Thakur View author publications You can also search for this author inPubMed Google
Scholar * Sunita Gorthy View author publications You can also search for this author inPubMed Google Scholar * AnilKumar Vemula View author publications You can also search for this author
inPubMed Google Scholar * Damaris A. Odeny View author publications You can also search for this author inPubMed Google Scholar * Pradeep Ruperao View author publications You can also search
for this author inPubMed Google Scholar * Pramod Ramchandra Sargar View author publications You can also search for this author inPubMed Google Scholar * Shivaji Pandurang Mehtre View
author publications You can also search for this author inPubMed Google Scholar * Hirakant V. Kalpande View author publications You can also search for this author inPubMed Google Scholar *
Ephrem Habyarimana View author publications You can also search for this author inPubMed Google Scholar CONTRIBUTIONS N.R.T., S.P.M., H.V.K., and P.R.S. were involved in performing the
experiments. A.V. analyzed the data. P.R. and N.R.T. involved in GWAS. analysis and images and visualization. P.R. and D.A.O. provided analysis tools. P.R. conducted bioinformatics to
identify associated candidate genes. N.R.T. and S.G. wrote the first draft of the manuscript, and D.A.O. and E.H. edited and revised the manuscript. E.H. conceived, designed, supervised the
experiments, project administration, and funding acquisition. All authors contributed to the article and approved the submitted version. CORRESPONDING AUTHOR Correspondence to Ephrem
Habyarimana. ETHICS DECLARATIONS COMPETING INTERESTS The authors declare no competing interests. ADDITIONAL INFORMATION PUBLISHER'S NOTE Springer Nature remains neutral with regard to
jurisdictional claims in published maps and institutional affiliations. SUPPLEMENTARY INFORMATION SUPPLEMENTARY INFORMATION. RIGHTS AND PERMISSIONS OPEN ACCESS This article is licensed under
a Creative Commons Attribution 4.0 International License, which permits use, sharing, adaptation, distribution and reproduction in any medium or format, as long as you give appropriate
credit to the original author(s) and the source, provide a link to the Creative Commons licence, and indicate if changes were made. The images or other third party material in this article
are included in the article's Creative Commons licence, unless indicated otherwise in a credit line to the material. If material is not included in the article's Creative Commons
licence and your intended use is not permitted by statutory regulation or exceeds the permitted use, you will need to obtain permission directly from the copyright holder. To view a copy of
this licence, visit http://creativecommons.org/licenses/by/4.0/. Reprints and permissions ABOUT THIS ARTICLE CITE THIS ARTICLE Thakur, N.R., Gorthy, S., Vemula, A. _et al._ Genome-wide
association study and expression of candidate genes for Fe and Zn concentration in sorghum grains. _Sci Rep_ 14, 12729 (2024). https://doi.org/10.1038/s41598-024-63308-0 Download citation *
Received: 26 December 2023 * Accepted: 27 May 2024 * Published: 03 June 2024 * DOI: https://doi.org/10.1038/s41598-024-63308-0 SHARE THIS ARTICLE Anyone you share the following link with
will be able to read this content: Get shareable link Sorry, a shareable link is not currently available for this article. Copy to clipboard Provided by the Springer Nature SharedIt
content-sharing initiative