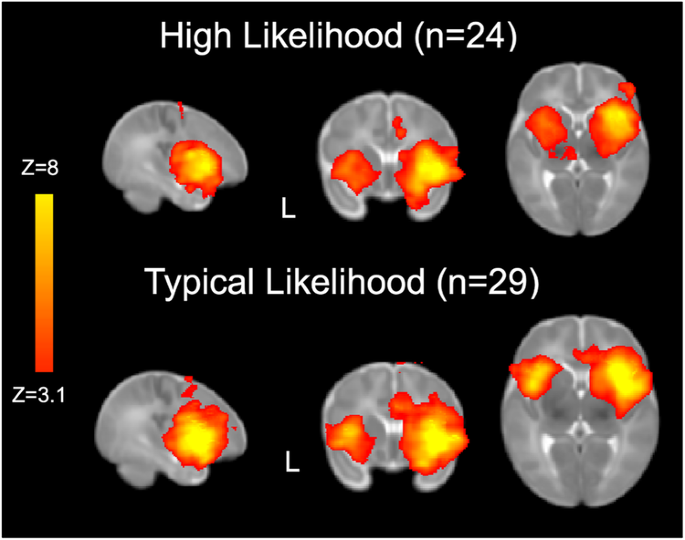
Salience network connectivity is altered in 6-week-old infants at heightened likelihood for developing autism
- Select a language for the TTS:
- UK English Female
- UK English Male
- US English Female
- US English Male
- Australian Female
- Australian Male
- Language selected: (auto detect) - EN
Play all audios:

ABSTRACT Converging evidence implicates disrupted brain connectivity in autism spectrum disorder (ASD); however, the mechanisms linking altered connectivity early in development to the
emergence of ASD symptomatology remain poorly understood. Here we examined whether atypicalities in the Salience Network – an early-emerging neural network involved in orienting attention to
the most salient aspects of one’s internal and external environment – may predict the development of ASD symptoms such as reduced social attention and atypical sensory processing.
Six-week-old infants at high likelihood of developing ASD based on family history exhibited stronger Salience Network connectivity with sensorimotor regions; infants at typical likelihood of
developing ASD demonstrated stronger Salience Network connectivity with prefrontal regions involved in social attention. Infants with higher connectivity with sensorimotor regions had lower
connectivity with prefrontal regions, suggesting a direct tradeoff between attention to basic sensory versus socially-relevant information. Early alterations in Salience Network
connectivity predicted subsequent ASD symptomatology, providing a plausible mechanistic account for the unfolding of atypical developmental trajectories associated with vulnerability to ASD.
SIMILAR CONTENT BEING VIEWED BY OTHERS CONNECTIVITY ALTERATIONS IN AUTISM REFLECT FUNCTIONAL IDIOSYNCRASY Article Open access 15 September 2021 THALAMOCORTICAL CONNECTIVITY IS ASSOCIATED
WITH AUTISM SYMPTOMS IN HIGH-FUNCTIONING ADULTS WITH AUTISM AND TYPICALLY DEVELOPING ADULTS Article Open access 03 February 2021 IMBALANCED SOCIAL-COMMUNICATIVE AND RESTRICTED REPETITIVE
BEHAVIOR SUBTYPES OF AUTISM SPECTRUM DISORDER EXHIBIT DIFFERENT NEURAL CIRCUITRY Article Open access 14 May 2021 INTRODUCTION Shortly after birth, newborns display systematic preferences for
faces1, voices2, and biological motion3. These early social-orienting mechanisms are foundational for normative social development4. However, the salience of socially-relevant information
appears disrupted in autism spectrum disorders (ASD). Infants who develop ASD show altered developmental trajectories5 characterized by reduced attention to social information6,7 and
heightened awareness of non-social sensory input8. Atypicalities in social versus nonsocial attention are broadly recognized as a marker of increased likelihood for ASD9 and likely
contribute to the emergence of social impairments and the restrictive and repetitive behaviors characteristic of ASD7,10. The neurobiological mechanisms underlying these early attentional
abnormalities that give rise to autism-related symptoms remains largely unknown. Examining early brain connectivity offers a promising lens for investigation11,12. Indeed, most genes
associated with increased likelihood for ASD impact synapse formation and function, presenting a biological pathway for ASD that converges on brain connectivity13,14. Neuroimaging studies
have consistently implicated atypical brain network dynamics in ASD15,16, with recent evidence demonstrating that 6-month-olds who later develop ASD already exhibit systematic differences in
whole-brain functional connectivity17. Thus, early deviations in brain network connectivity may provide a biomarker of ASD likelihood prior to the emergence of behavioral symptoms18. One
early emerging functional brain network19 of particular interest in understanding ASD symptomatology is the Salience Network (SN)20, which is believed to be integral in guiding attention to
the most salient interoceptive and exteroceptive stimuli20,21. Altered Salience Network connectivity can discriminate children with ASD from neurotypical controls with high classification
accuracy22, and is associated with symptoms of restrictive/repetitive behaviors22, including atypical sensory processing23, which may highlight a potential neural mechanism underlying
increased perceived salience of low-level, perceptual contingencies in the environment at the expense of higher-level social information24. The Salience Network has been identified in
neonates19,25,26, and while not yet fully mature19, even in its early forms, it is thought to play a key role in influencing early brain development27. Furthermore, hypoconnectivity within
Salience Network hubs has recently been reported in a small sample of neonates at increased likelihood for developing ASD based on family history28. In addition to these consistent findings
of atypical Salience Network connectivity in ASD, behavioral evidence also implicates this network in the emergence of ASD symptomatology in early infancy. Faces represent a highly salient
class of stimuli for typically-developing infants29 and normative patterns of early Salience Network connectivity may support the initial attentional bias toward faces as well as subsequent
developmental increases in visual attention to faces in the first year30. Conversely, early disruptions in Salience Network connectivity may iteratively derail processes that typically
reinforce the perceived salience of faces31 by conferring heightened salience to lower-level non-social stimuli24. Indeed, initial overt symptom-based markers of ASD suggest deviations in
processes that typically guide social communicative development, including attention to faces5 and speech. Potential atypicalities in Salience Network connectivity may thus contribute to the
emergence of characteristic features of ASD (i.e., social communicative impairments and altered sensitivity to sensory stimuli). To test this model, we evaluated Salience Network
connectivity in 6-week-old infants, at high (HL) and typical (TL) likelihood of developing ASD, based on family history, and its association with subsequent behavioral ASD symptom-based
markers, including atypicalities in visual social attention to faces, communicative development, and sensory processing. Between 4 and 8 weeks, early social-orienting behaviors transition
from being under reflexive subcortical control to experience-dependent cortical control32,33. Thus, examining Salience Network connectivity during this period may reveal altered development
of social attention and provide a mechanistic account for the ontogeny of ASD symptomatology. We hypothesized that HL infants would show Salience Network hyperconnectivity with sensorimotor
regions22,23 and that patterns of Salience Network connectivity would predict individual trajectories of social attention, communicative development, and sensory sensitivities. This study
prospectively evaluated 53 HL and TL infants. HL infants had at least one older sibling with an ASD diagnosis (_N_ = 24) whereas TL infants (_N_ = 29) had no family history of ASD or any
other developmental disorders. Infants underwent resting-state functional magnetic resonance imaging (rs-fMRI) at 6 weeks of age during natural sleep to examine Salience Network
connectivity. Infants’ eye movements were tracked at 3-, 6-, 9-, and 12-months of age while they viewed video stimuli of naturalistic social interactions (i.e., excerpts from _Charlie Brown_
and _Sesame Street_34) to capture developmental trajectories in visual social attention to faces. We examined core autism symptomatology with the Autism Observation Scale for Infants (AOSI)
and nonverbal social-communication with the Early Social Communication Scale (ESCS) at 12 months (see Table 1), as well as sensory sensitivity with the Infant/Toddler Sensory Profile (ITSP)
from 6- to 12-months. RESULTS AND DISCUSSION WITHIN- AND BETWEEN-GROUP SALIENCE NETWORK CONNECTIVITY Consistent with prior work, we used a right anterior insula (rAI) seed to characterize
Salience Network connectivity across the whole brain23,35 (Fig. 1). We first examined between-group differences in Salience Network connectivity based on the likelihood of developing ASD
(Fig. 2). Compared to TL infants, HL infants showed stronger connectivity between the hub of the Salience Network (i.e., the rAI) and sensorimotor regions, including bilateral precentral
gyrus and left postcentral gyrus, thalamus, hippocampus, caudate and putamen (Fig. 2a). In contrast, compared to TL infants, HL infants showed weaker connectivity between the rAI and
prefrontal regions associated with higher-order processing and attentional control, including right inferior and middle frontal gyri, and anterior cingulate (Fig. 2b). Importantly, we found
an inverse relationship in Salience Network connectivity with sensorimotor and prefrontal regions across all participants (_r_ = _−0.43, p_ = 0.001, 95% CI: 0.18–0.63) such that infants
exhibiting the strongest Salience Network connectivity with sensorimotor regions also showed the weakest connectivity with higher-order prefrontal regions (Fig. 2c). This direct tradeoff
between neural resources allocated towards sensorimotor processing versus social attention could thus explain the co-emergence of both core sensory and social ASD symptoms. SALIENCE NETWORK
CONNECTIVITY AND DEVELOPMENTAL TRAJECTORIES IN ATTENTION TO FACES This inverse pattern of connectivity between the Salience Network and social vs. sensorimotor regions indicates an early
neural mechanism that may lead to divergent developmental outcomes for HL infants. To then directly examine the downstream, developmental effects of Salience Network connectivity on visual
social attention to faces, which has been shown to be attenuated in HL infants6, we evaluated whether early Salience Network connectivity predicts individual trajectories in attention to
faces from 3- to 12-months. We measured percent looking time to faces from the eye-tracking data (Fig. 3a) and used a Bayesian hierarchical linear model to estimate each infant’s rate of
increased attention to faces from 3- to 12-months. This estimate was then used as a covariate of interest in our analysis of Salience Network connectivity. HL infants did not show any
significant associations between 6-week Salience Network connectivity and subsequent trajectories in attention to faces. In TL infants, however, greater connectivity between the two major
hubs of the SN – rAI and the anterior cingulate cortex20 – and right lateral orbitofrontal cortex at 6 weeks predicted greater increases in attention to faces across the first postnatal year
(Fig. 3b, c). Importantly, these frontal regions predicting increased social attention overlapped with those exhibiting greater Salience Network connectivity in TL infants relative to HL
infants. Early social behavior, including orienting to faces and preferential attention to biological motion, is highly phylogenetically-conserved3 and genetically constrained36. Similarly,
developmental changes in Salience Network connectivity during the first postnatal year appears to be strongly influenced by genetic effects, more so than environmental factors37. In light of
our findings, strong Salience Network connectivity with frontal regions at 6 weeks may thus contribute to the early perceived salience of faces in typical development and thereafter support
normative gains in social attention through iterative processes that further consolidate brain-behavior connections. SALIENCE NETWORK CONNECTIVITY AND LATER SOCIAL- AND SENSORY-PROCESSING
DEVELOPMENT We next examined the relation between Salience Network connectivity and standardized measures of communicative development (ESCS), ASD-related symptoms (AOSI), and sensory
processing atypicalities (ITSP). In TL infants, greater connectivity between the Salience Network hub (i.e., rAI) and both prefrontal (i.e., right inferior frontal gyrus, middle frontal
gyrus, and orbital frontal cortex) and subcortical regions associated with reward and learning (i.e., bilateral caudate, pallidum, and nucleus accumbens) predicted higher rates of initiating
joint attention – nonverbal communicative behaviors associated with better language functioning and social competence38– at 12 months (Fig. 4a). Salience Network connectivity at 6 weeks was
not related to response to joint attention in either group. This finding provides further evidence that normative patterns of Salience Network connectivity in early infancy support
attention to socially relevant stimuli, thereby scaffolding the development of social communication skills. The lack of similar relationships between Salience Network connectivity and later
social communication skills in the HL group could reflect the overall reduced Salience Network connectivity with frontal cortex at 6 weeks in this group. In other words, it is possible that
this group shows delayed development of the early neural precursors of social communication, but further longitudinal investigations of connectivity across the first year of life are
necessary to determine whether this is a delayed or atypical trajectory. Distinct patterns of 6-week Salience Network connectivity also predicted ASD-associated symptoms in HL infants at
6–12 months (Fig. 4b, c). Greater Salience Network connectivity with regions associated with primary auditory and sensory processing (right superior temporal gyrus and thalamus) predicted
higher parental ratings of sensory hypersensitivity at 6–12 months. This finding is consistent with observed associations between Salience Network hyperconnectivity and sensory
over-responsivity in older children with ASD23. HL infants may be predisposed to attend to extraneous sensory inputs quite early in development at the expense of socially relevant
information. Furthermore, stronger connectivity between the Salience Network hub and regions involved in implicit learning and reward processing (left putamen, inferior frontal gyrus,
caudate, amygdala), and memory (left hippocampus) predicted fewer ASD symptoms on the AOSI at 12 months. Better integration between the Salience Network and both reward and emotional systems
(e.g., regions associated with the social brain39) may be protective for social cognitive development in HL infants; conversely, reduced Salience Network connectivity with these social
brain regions in early infancy may negatively impact social learning. Our data reveal dissociable patterns of Salience Network connectivity between 6-week-old TL and HL infants with
cascading effects on brain-behavioral processes that may underlie both normative and atypical social communicative development. Detectable differences in functional connectivity in early
infancy corroborate cytoarchitectural and genetic data implicating prenatal neural development as a neurobiological factor that increases the likelihood for ASD40. The observed
hyperconnectivity between the hub of the Salience Network and sensorimotor regions in HL infants likely represents a developmental vulnerability that, given the heterogeneity of
developmental trajectories associated with a family history of ASD, may be broadly associated with suboptimal outcomes. The observed tradeoff in functional connectivity with these areas
versus higher-order prefrontal regions highlight that ASD-associated brain development manifests as differences of degree, not kind. Taken together, these results provide clear empirical
support for recent theoretical frameworks positing that initial deviations in attentional biases and/or sensorimotor processing may lead to the emergence of ASD-related behaviors by altering
the experience-dependent brain changes that typically guide social development41. Furthermore, our findings demonstrate that atypical functional brain connectivity is present as early as 6
weeks of age, long before detectable differences in behavior are observed in infants and toddlers at high likelihood for developing ASD. This difference is notable because it suggests a
protracted prodromal period for known ASD-associated symptoms to emerge, including early social difficulties, attentional difficulties, language delays, and socioemotional problems. While
specific patterns of Salience Network connectivity within the HL and TL infants predicted distinct levels of social functioning and sensory processing, HL and TL infants exhibited comparable
behavioral profiles at 12 months, consistent with prior behavioral studies10. By affecting attentional biases, initial differences in brain connectivity may become compounded over
development through individual experiences, highlighting the role of experience in shaping brain-behavior relationships and the opportunity to intervene to prevent the crystallization of
atypical social communicative development. Examining early functional brain connectivity can inform the impact of genetic and environmental factors on subsequent development by highlighting
the intermediary neural networks that ultimately underlie behavior. For instance, recent work has suggested that prenatal exposure to maternal inflammatory markers negatively influence early
Salience Network connectivity in infants without a family history of ASD25, which suggests an intricate interplay between genetic and environmental factors in fine-tuning brain-behavior
connections and contributing to the individual variability in early social orienting behaviors. While a primary aim of the current study was to elucidate the role of Salience Network
connectivity in conferring vulnerability to ASD, infants with a family history of ASD are also more likely to exhibit atypical development, including subclinical ASD symptomatology (i.e.,
broader autism phenotype), speech/language delays, global developmental delay, and Attention Deficit Hyperactivity Disorder (ADHD) during school-age years42. Thus, the clinical implications
of our findings extend beyond the likelihood of an ASD diagnosis, providing a testable model for examining how early Salience Network connectivity may be related to other suboptimal
developmental outcomes that are associated with a family history of ASD. Although our modest sample size and the single timepoint for evaluating Salience Network connectivity are limitations
in the current study, the overall results strongly suggest that atypical patterns of Salience Network connectivity may reflect a developmental vulnerability. This is a possibility that
should be examined in large-scale longitudinal studies that heavily sample brain and behavioral measures during the first postnatal years. In sum, our findings demonstrate that aberrant
patterns of functional brain connectivity can be detected in infants at high likelihood for developing ASD shortly after birth, and that these alterations predict ASD-related symptomatology
a year later. Identifying atypical brain connectivity in infancy may ultimately pave the way for early interventions that can effectively redirect attention to socially relevant inputs
and/or reduce distress caused by aversive sensory inputs and thus promote optimal developmental outcomes. METHODS PARTICIPANTS Participants in this study were enrolled as part of a
longitudinal project examining early brain-based markers of ASD during the first year, with data collected between December 2012 through November 2017. The Institutional Review Board (IRB)
at the University of California, Los Angeles, approved all protocols associated with the project, and all enrolled participants had informed consent provided by their parent/legal guardian.
All procedures complied with ethical regulations for vulnerable populations. Infants were assigned to ASD-likelihood cohorts based on family history: high-likelihood infants (HL) had at
least one older sibling with a clinical ASD diagnosis whereas typical-likelihood infants (TL) had no family history of ASD or any other developmental disorder. Prior research showed that the
recurrence likelihood for developing ASD is approximately 20% in HL infants43. High and typical likelihood families were broadly recruited in Los Angeles County through IRB-approved fliers
posted in pediatrician offices, newspapers, magazines, community-based events, and the Center for Autism Research and Treatment at the University of California, Los Angeles. Additional
recruitment strategies included IRB-approved messages aired on Pandora and local radio stations, and word-of-mouth. This ensured a broad sampling. The sample size was based on prior
resting-state fMRI studies in infants. Based on effect sizes derived from these prior studies (e.g., Liu et al. and Damaraju et al.), a minimum sample size of 23 per group was sufficient to
detect significant effects. Exclusionary criteria for both groups included: 1) indication of genetic or neurological conditions associated with ASD likelihood (e.g., fragile X syndrome,
epilepsy, tuberous sclerosis), 2) significant perinatal insult or chronic medical conditions impacting development, 3) severe visual, hearing, or motor impairment, 4) non-English speaking
parents, and 5) contraindication for MRI (e.g., metal implants). All participants were enrolled in the study prior to 6 weeks of age. HL and TL infants were matched by gender (Mann–Whitney
_U_ = 306, Z = 0.87 _p_ = 0.38, _r_ = 0.12), and birth weight (_t_(51) = 0.43, _p_ = 0.67, Cohen’s _d_ = 0.12), as well as ethnicity and family socio-economic status (race: Mann–Whitney _U_
= 341.5_, p_ = 0.88; household income: Mann–Whitney _U_ = 282.5, _Z_ = 1.21 _p_ = 0.23, _r_ = 0.166). Given the diverse demographic of Los Angeles County, the research sample was
representative of the broader population of infants with and without familial history of ASD. HL and TL infants did not differ on cognitive development at 12 months according to the Mullen
Scales of Early Learning—Early Learning Composite (HLELC Mean=106.67, SD = 17.24; TLELC Mean = 110.22, SD = 11.83; _t_(49) = 0.87, _p_ = 0.39, Cohen’s _d_ = 0.24; see Table 1). A total of 53
infants (_N_ = 24 HL, _N_ = 29 TL) underwent functional magnetic resonance imaging (fMRI) during natural sleep at approximately 6 weeks of age (HLage Mean = 6.63 weeks, SD = 1.37 weeks;
TLage Mean = 6.65 weeks, SD = 1.09 weeks, _t_(51) = 0.062, _p_ = 0.95, Cohen’s _d_ = 0.02). A subset of 51 infants (_N_ = 24 HL, _N_ = 27 TL) provided data from behavioral measures of social
and cognitive development at 12 months (2 TL infants dropped out of the study). Of the 53 infants, 50 infants (_N_ = 24 HL; 26 TL) provided longitudinal eye-tracking data at 3-, 6-, 9-, and
12-months of age. An additional 3 infants participated in the study but were not included in the analyses due to excessive head motion during scanning and/or scanner artifacts. No
statistical methods were used to pre-determine sample size, but our sample size is larger than what has been reported in previous publications evaluating resting-state connectivity in early
infancy44,45,46. HL infants were all later-born children; the TL infants in our sample included both first-born (_N_ = 16) and later-born children (_N_ = 13). We did not observe differences
in overall connectivity strength within the SN between first- and later-born TL infants (t(27) = 1.07, _p_ = 0.318, Cohen’s d = 0.379), nor did we observe differences in behavioral measures
of cognitive and social development at 12 months (_t’_s < 1.90, _p_’s > 0.06, Cohen’s _d_ < 0.53). Therefore, we do not believe birth order would have had an effect on the findings
presented here. BEHAVIORAL MEASURES Infants were administered a battery of behavioral assessments during the first postnatal year to measure socio-communicative, cognitive, and sensory
development (see Table 1). At 12 months, infants’ developmental level was assessed with the Mullen Scales of Early Learning47, nonverbal social communicative behaviors (i.e., rates of
initiating of and responding to joint attention cues—IJA and RJA respectively) with the Early Social Communication Scales (ESCS48), and early signs of ASD symptomatology with the Autism
Observation Scale for Infants (AOSI49). Approximately 20% of the total ESCS sample was double coded for reliability. Coders were trained undergraduate research assistants who were blind to
ASD family status and other study variables. Intraclass correlations (ICC; absolute agreement, single measures) indicated good reliability for IJA (ICC = 0.96) and RJA (ICC = 0.89).
Behavioral measures were administered blind to the infant’s ASD likelihood status. Parents also completed the Infant/Toddler Sensory Profile50, a standardized questionnaire tracking their
child’s sensitivity to sensory inputs and sensory-related difficulties, at 6, 9, and 12 months; the average raw score on the Sensory Sensitivity quadrant was calculated and used as a general
metric of sensitivity to visual, auditory, and tactile stimuli. EYE-TRACKING PROTOCOL Infants were eye-tracked at the 3-, 6-, 9- and 12-month visits while they were presented with two,
2-mintue full audiovisual video segments taken from a cartoon and live-action video; these video stimuli have been previously used in studies on visual social attention to faces in
typically-developing infants34,51. Infants sat on their caregiver’s lap during the eye-tracking procedure at approximately 60 cm from the 65-cm video display monitor. Caregivers were
explicitly instructed not to distract their infant’s attention from the screen during stimuli presentation. Point-of-gaze data were collected using a Tobii T60XL eye-tracker at 60 Hz with a
spatial accuracy of approximately 0.5° accuracy. Eye-movements (e.g., fixations, blinks, and saccades) were detected using the accompanying Tobii software. Infants’ point-of-gaze were
calibrated using a 5-point calibration scheme prior to data collection. The calibration scheme was repeated until an infant’s point-of-gaze was within 1° of the center of the target and
repeated between the two trials. The video stimuli were presented only after the calibration criterion had been reached. Individual trials were removed from analyses due to failure to
initially calibrate the infant’s eyes to the eye-tracking system (_N_trials = 9) or failure to track an infant’s eyes because of excessive movement or fussiness (_N_trials = 31). Video
frames were 8-bit color images and 720 by 480 pixels in resolution. Each frame was hand-traced for areas of interest, which were demarcated as a box encompassing each character’s face as in
the prior studies of typical development using the same stimuli34,51. Fixations that fell within areas of interest were identified using software written in MATLAB (MathWorks, Inc; Natick,
MA). The primary dependent measure was percent of fixations that were directed at the face areas of interest. EYE-TRACKING STATISTICAL ANALYSIS Our primary interest from modeling the
eye-tracking data was to estimate each infant’s rate of change in attention to faces from 3 to 12 months, such that individual developmental trajectories in social attention could be
analyzed as a function of 6-week Salience Network connectivity. Longitudinal changes in percent fixation to faces were analyzed with a Bayesian hierarchical linear model in R (rstanarm
package), which uses a Markov chain Monte Carlo simulation to draw a posterior distribution (e.g., a range of probable values for a variable given the data). This aspect of the Bayesian
framework allows for greater precision in estimating parameters than by the frequentist approach52,53. Attention to faces was operationalized as percent fixations to characters’ faces in
each video stimulus. Developmental trajectories were modeled as the linear and quadratic effects of age (i.e., age and age2, respectively). ASD-likelihood group (HL versus TL) and video
stimulus type (_Charlie Brown_ versus _Sesame Street_) were modeled as fixed effects; age and intercept were modeled as random effects to account for individual differences and correlated
repeated measures at 3, 6, 9, and 12 months. The inclusion of the change of slope (i.e., the quadratic term age2) aimed to capture the change in growth rate from 3 to 12 months. Student-t
distributions were used as priors for the regression coefficients and standard deviation, and a Gaussian function was used as the identity link function. The model in equation form is:
$${{{{{\rm{Level}}}}}}1:{{{{{\rm{Fixatio}}}}}}{{{{{{\rm{n}}}}}}}_{{{{{{\rm{ij}}}}}}}={{{{{\rm{intercep}}}}}}{{{{{{\rm{t}}}}}}}_{0{{{{{\rm{j}}}}}}}+{{{{{{\rm{B}}}}}}}_{1{{{{{\rm{j}}}}}}}{({{{{{\rm{Age}}}}}})}_{{{{{{\rm{ij}}}}}}}+{{{{{{\rm{B}}}}}}}_{2{{{{{\rm{j}}}}}}}{({{{{{\rm{Age}}}}}})}^{2}{{{{{\rm{ij}}}}}}+{{{{{{\rm{error}}}}}}}_{{{{{{\rm{ij}}}}}}}$$
$${{{{{\rm{Level}}}}}}2:{{{{{{\rm{Intercept}}}}}}}_{0{{{{{\rm{j}}}}}}}={\Upsilon }_{00}+{\Upsilon }_{01}({{{{{\rm{Likelihood\; Status}}}}}})+{\Upsilon }_{02}({{{{{\rm{Stimulus\;
Type}}}}}})+{{{{{{\rm{error}}}}}}}_{0{{{{{\rm{j}}}}}}}$$ $${{{{{\rm{Level}}}}}}2:{{{{{\rm{B}}}}}}1{{{{{\rm{j}}}}}}={\Upsilon }_{10}+{\Upsilon }_{11}({{{{{\rm{Likelihood\;
Status}}}}}})+{\Upsilon }_{12}({{{{{\rm{Stimulus\; Type}}}}}})+{{{{{{\rm{error}}}}}}}_{1{{{{{\rm{j}}}}}}}$$ $${{{{{\rm{Level}}}}}}2:{{{{{{\rm{B}}}}}}}_{2{{{{{\rm{j}}}}}}}={\Upsilon
}_{20}+{\Upsilon }_{21}({{{{{\rm{Likelihood\; Status}}}}}})+{\Upsilon }_{22}({{{{{\rm{Stim\; Type}}}}}})+{{{{{{\rm{error}}}}}}}_{2{{{{{\rm{j}}}}}}}$$ Pareto k diagnostic values indicated a
good model fit (all pareto k estimates were less that 0.7). Diagnostics regarding model fit and visualization of posterior distributions were verified with the shinystan package in R.
Statistical significance was evaluated using two-tailed tests. As expected, there was a significant linear effect of age such that attention to faces increased with age across all
participants (95% CI: [1.73 9.39]). The rate of change over time decreased across all infants indicating a quadratic trajectory in face-looking (95% CI: [−0.47 −0.01]). There was also a main
effect of ASD-likelihood group such that HL infants overall attended less to faces than TL infants (95% CI: [−40.91 −3.66]). Using the coef function in R, we extracted each infant’s
estimate of developmental increase in face-looking from 3 to 12 months (i.e., each infant’s beta coefficient for the age term in the model). These estimates were then used as a covariate of
interest in a model of Salience Network connectivity. MRI DATA ACQUISITION MRI data were acquired on a 3T Siemens Tim Trio scanner using a 12-channel head coil during natural sleep. Parents
were instructed to put their infant to sleep using their normal bedtime routine. After the infant was asleep and swaddled, silicon earplugs were placed over the infant’s ear canal, and mini
earmuffs were fitted over the entire outer ears. Infants were then placed on a custom-made bed that fit inside the scanner’s head coil and secured on the scanner bed with a Velcro strap. To
minimize movement, a weighted blanket was used and foam pads were positioned around each infant’s head. A localizer scan was used for graphic prescription. Structural matched bandwidth
T2-weighted high-resolution echo planar images are acquired co-planar to the functional scans to ensure identical distortion characteristics to the fMRI scans (TR = 5000 ms, TE = 34 ms,
matrix size 128×128, FOV = 192 mm, 34 slices, 1.5 mm in-plane resolution, with 4-mm-thick axial slices). Resting-state data were collected during an 8-min rs-fMRI scan (TR = 2000 ms, TE = 28
ms, matrix size 64 × 64, FOV = 192 mm, 34 slices, 3 mm in-plane resolution, with 4mm-thick axial slices). FMRI DATA PREPROCESSING Functional imaging data were preprocessed and analyzed
using FSL version 5.0.8 (fMRIB’s Software Library54. Functional images were co-registered to the subject’s corresponding T2-weighted high-resolution anatomical scan, registered to an infant
brain template55 using 12-parameter affine transformations, and spatially smoothed (Gaussian kernel of 6 mm FWHM) to increase signal-to-noise ratio. ICA-AROMA was used to detect and remove
motion artifacts from the data56. This is a validated procedure that uses probabilistic independent component analysis to automatically detect participant-specific motion-related independent
components while preserving signal of interest57. ICA-AROMA was selected over other motion denoising methods, such as deleting individual motion-contaminated volume (e.g., scrubbing)58 to
effectively control for motion while maximizing data from the full scan. HL and TL infants did not differ in the average number of noise components identified by ICA-AROMA [HL: Mean = 27.25,
SD = 9.43; TL: Mean = 27.72, SD = 11.37; _t(51)_ = 0.16, _p_ = 0.87, Cohen’s _d_ = 0.05]; the number of noise components detected were comparable to that reported by Pruim and colleagues
(23.1 components)56. HL and TL infants also did not differ on average relative motion prior to the denoising with ICA-AROMA (HL: Mean = 0.10 mm, SD = 0.07 mm; TL: Mean = 0.23 mm, SD = 0.82
mm; _t(51)_ = 0.81_, p_ = 0.42, Cohen’s _d_ = 0.22). Data were then band-pass filtered (0.01–0.1 Hz). Nuisance regressors (e.g., mean cerebrospinal fluid, white matter, and global time
series) from the bandpass filtered data were calculate and then regressed out of the filtered data to further remove noise. Given continuous debate in the field as per the pros and cons of
implementing global signal regression59, group-level analyses were also completed without regressing global signal or its derivatives as nuisance variables; none of our main findings were
affected by this change, including the inverse pattern of connectivity between frontal and sensorimotor regions (_r_ = −0.428, _p_ = 0.001, 95% CI: [−0.626 −0.178]). FMRI STATISTICAL
ANALYSES Resting-state fMRI analyses were conducted with FSL fMRI Expert Analysis Tool (FEAT, version 6.0 www.fmrib.ox.ac.uk/fsl/60). Whole brain connectivity within the Salience Network was
examined using a right anterior insula (rAI) seed35 that was derived from an anatomical parcellation of the right insula from a neonatal template. We identified the center of gravity of the
right insula and created an anatomical mask composed of voxels anterior to the midline55. The center of gravity for our rAI seed (X = 23, Y = 5, Z = 1) corresponds to meta-analytic
definitions of rAI61. Region-of-interest (ROI) time-series from each infant’s processed residuals in standard space were extracted and correlated with every other voxel in the brain to
generate Salience Network functional connectivity maps. Individual Salience Network maps were converted into z-statistic maps using Fischer’s r-to-z transformation. At the group level, we
modeled a 2-sample mixed-effects design, at _Z_ > 3.1 with cluster correction for multiple comparisons at _p_ < 0.05, using FSL FLAME (FMRIB’s Local Analysis of Mixed Effects State)
Stage 1 + 2. Statistical significance was evaluated using two-tailed tests. For the between-group comparisons and regression analyses (see below), significance was assessed voxel-wise at _p_
< 0.05, controlling for multiple comparisons using cluster-level correction estimated by AFNI’s 3dClustSim with 10,000 iterations at initial cluster forming thresholds of both _p_ <
0.01 (_Z_ = 2.3) and _p_ < 0.001 (_Z_ = 3.1), a mixed-model spatial autocorrelation function, and a joint [HL + TL] Salience Network connectivity map. Family income and gestational age
were examined as potential covariates; as none contributed significantly they were excluded from the final analyses. Regression analyses with estimates of increases in face-looking from 3 to
12 months, AOSI, ESCS, and Infant/Toddler Sensory Profile were restricted to (i.e., masked by) the joint [HL + TL] Salience Network connectivity maps (see Table 2). Parameter estimates of
connectivity strength for between-group comparisons were extracted from significant clusters using FMRIB and evaluated using Pearson correlation. ADDITIONAL CONTROL ANALYSES To address the
specificity of our findings to the Salience Network, additional analyses were conducted using posterior cingulate cortex, bilateral dorsolateral prefrontal cortex, and bilateral
pre/postcentral gyri seeds to separately evaluate connectivity in the default mode network, the fronto-parietal attentional network, and sensorimotor networks, respectively, following the
dame data-analytic procedures. Atypicalities in functional connectivity in the default mode network and fronto-parietal networks have been observed in youth and adults with ASD, thus serving
as good control networks to examine potential developmental origins of disrupted network connectivity in individuals with ASD15,62,63. However, the default mode and fronto-parietal networks
undergo substantive development during the first year whereas the somatosentory network is present at birth64. Accordingly, examining this network would allow to further evaluate whether
the observed between-group differences in network connectivity between HL and TL infants are specific to the Salience Network or are more broadly reflective of disruptions in early
functional brain organization65. These additional seed-based connectivity analyses were implemented according to the same procedures as used for the Salience Network, as follows:
Anatomically-defined seeds were used to identify the default mode network, fronto-parietal network, and sensorimotor networks for whole-brain connectivity analyses. The 5-mm seeds for these
three networks were the precuneus (Montreal Neurological Institute coordinates; MNI: [−1, −30, 12]66; bilateral dorsolateral prefrontal cortex (MNI: [+/−30, 11, 25]), and bilateral pre- and
post-central gyri (MNI: [+/−28, −11, 32]), respectively. FSLMATHS54 was used to identify the center of gravity (i.e., coordinates in MNI space) for the precuneus and pre-/post-central gyri
based on the parcellation available for the infant anatomical atlas55 However, because the atlas does not include a separate dorsolateral prefrontal cortex region of interest, the
meta-analytic database Neurosynth (https://neurosynth.org)61 was used to identify the MNI coordinates for the dlPFC seed. As shown in Supplementary Fig. 1, we did not observe any significant
between-group differences in connectivity across these three additional networks, attesting to the specificity of our findings of early atypicalities in SN connectivity in infants at high
familial risk for ASD. EQUIVALENCE TESTS To further qualify the non-significant between-group differences in connectivity within these three additional control networks, we conducted
equivalence tests using the TOSTER package in R67. First, we used the FSL command fslmeants to separately extract parameter estimates of connectivity for HL and TL groups, from the
respective within-group connectivity maps for each of these three control networks (DMN, frontoparietal, and sensorimotor networks). While effect sizes are not commonly reported in the
functional connectivity literature, we calculated the standardized effect size associated with a Z score ≥3.1 and our sample size, which can be interpreted as the critical effect size. We
used this value as the smallest effect size of interest (SESOI). Using this value, an equivalence test can reject effect sizes falling outside that bound. In this case, the critical effect
size was _d_ = 0.855. The TOST (two one-sided test) procedure for Student’s equivalence test for independent samples, with equivalence bounds of ΔL = –0.855 and ΔU = 0.855, revealed that the
effects observed were statistically equivalent across HL and TL groups for both the frontoparietal network (t(51) = −1.861, _p_ = 0.0342) and sensorimotor network (t(51) = −2.183, _p_ =
0.0168). Despite a trend in the same direction, this was not the case for the default mode network (t(51) = −1.286, _p_ = 0.102), warranting further examination of this network in future
studies. STATISTICS AND REPRODUCIBILITY Detailed explanations for statistical approaches used in all analyses (i.e., eye tracking, within- and between-group functional connectivity,
correlations between functional connectivity and behavior, and control connectivity analyses) are provided in each of the relevant sections above. Our experiments were not replicated, but a
description of power and effect size is described in the Participants section, and effect sizes and/or confidence intervals are provided for each analysis, as relevant. REPORTING SUMMARY
Further information on research design is available in the Nature Portfolio Reporting Summary linked to this article. DATA AVAILABILITY The raw data that support the findings of this paper
are available via the National Institute of Mental Health Data Archive (NDA; https://nda.nih.gov/)69. Dataset identifier: 10.15154/qx4v-t626. The source data for Figs. 2c, 3a, 3c, and 4 are
provided in Supplementary Data 1. CODE AVAILABILITY Eye movements and coding of fixation data were conducted with software written in MATLAB (MathWorks), available from Zenodo at this link:
https://zenodo.org/record/840370168. REFERENCES * Morton, J. & Johnson, M. H. CONSPEC and CONLERN: a two-process theory of infant face recognition. _Psychol. Rev._ 98, 164–181 (1991).
Article CAS PubMed Google Scholar * DeCasper, A. & Fifer, W. Of human bonding: newborns prefer their mothers’ voices. _Science_ 208, 1174–1176 (1980). Article CAS PubMed Google
Scholar * Simion, F., Regolin, L. & Bulf, H. A predisposition for biological motion in the newborn baby. _Proc. Natl Acad. Sci._ 105, 809–813 (2008). Article CAS PubMed PubMed
Central Google Scholar * Grossmann, T. & Johnson, M. H. The development of the social brain in human infancy. _Eur. J. Neurosci._ 25, 909–919 (2007). Article PubMed Google Scholar *
Jones, W. & Klin, A. Attention to eyes is present but in decline in 2-6-month-old infants later diagnosed with autism. _Nature_ 504, 427–431 (2013). Article CAS PubMed PubMed Central
Google Scholar * Chawarska, K., Macari, S. L. & Shic, F. Decreased spontaneous attention to social scenes in 6-month-old infants later diagnosed with autism spectrum disorders. _Biol.
Psychiatry_ 74, 195–203 (2013). Article PubMed PubMed Central Google Scholar * Shic, F., Macari, S. & Chawarska, K. Speech disturbs face scanning in 6-month-old infants who develop
autism spectrum disorder. _Biol. Psychiatry_ 75, 231–237 (2014). Article PubMed Google Scholar * Thye, M. D., Bednarz, H. M., Herringshaw, A. J., Sartin, E. B. & Kana, R. K. The
impact of atypical sensory processing on social impairments in autism spectrum disorder. _Dev. Cogn. Neurosci_. https://doi.org/10.1016/j.dcn.2017.04.010 (2017). * Tager-Flusberg, H. The
origins of social impairments in autism spectrum disorder: Studies of infants at risk. _Neural Netw._ 23, 1072–1076 (2010). Article PubMed PubMed Central Google Scholar * Zwaigenbaum,
L., Bryson, S. & Garon, N. Early identification of autism spectrum disorders. _Behav. Brain Res._ 251, 133–146 (2013). Article PubMed Google Scholar * Zhang, H., Shen, D. & Lin,
W. Resting-state Functional MRI Studies on Infant Brains: a Decade of Gap-Filling Efforts. _NeuroImage_ 185, 664–684 (2019). Article PubMed Google Scholar * Ciarrusta, J. et al. Emerging
functional connectivity differences in newborn infants vulnerable to autism spectrum disorders. _Transl. Psychiatry_ 10, 1–10 (2020). Article Google Scholar * Voineagu, I. et al.
Transcriptomic analysis of autistic brain reveals convergent molecular pathology. _Nature_ 474, 380–384 (2011). Article CAS PubMed PubMed Central Google Scholar * Gandal, M. J. et al.
Transcriptome-wide isoform-level dysregulation in ASD, schizophrenia, and bipolar disorder. _Science_ 362, 1–31 (2018). Article Google Scholar * Hernandez, L. M., Rudie, J. D., Green, S.
A., Bookheimer, S. & Dapretto, M. Neural Signatures of Autism Spectrum Disorders: Insights into Brain Network Dynamics. _Neuropsychopharmacology_ 40, 171–189 (2015). Article PubMed
Google Scholar * Hull, J. V. et al. Resting-State Functional Connectivity in Autism Spectrum Disorders: A Review. _Front. Psychiatry_ 7, 205 (2016). Article PubMed Google Scholar *
Emerson, R. W. et al. Functional neuroimaging of high-risk 6-month-old infants predicts a diagnosis of autism at 24 months of age. _Sci. Transl. Med._ 9, 1–19 (2017). Article Google Scholar
* Girault, J. B. & Piven, J. The Neurodevelopment of Autism from Infancy Through Toddlerhood. _Neuroimaging Clin. N. Am._ 30, 97–114 (2020). Article PubMed Google Scholar * Gao, W.,
Alcauter, S., Smith, J. K., Gilmore, J. H. & Lin, W. Development of human brain cortical network architecture during infancy. _Brain Struct. Funct_. 1–14,
https://doi.org/10.1007/s00429-014-0710-3 (2014). * Uddin, L. Q. Salience processing and insular cortical function and dysfunction. _Nat. Rev. Neurosci._ 16, 55–61 (2014). Article PubMed
Google Scholar * Uddin, L. Q., Nomi, J. S., Hébert-Seropian, B., Ghaziri, J. & Boucher, O. Structure and Function of the Human Insula. _J. Clin. Neurophysiol. Publ. Am.
Electroencephalogr. Soc._ 34, 300–306 (2017). Google Scholar * Uddin, L. & Supekar, K. Salience Network-Based Classification and Prediciton of Symptom Severity in Children with Autism.
_JAMA_ 70, 869–879 (2013). Google Scholar * Green, S. A., Hernandez, L., Bookheimer, S. Y. & Dapretto, M. Salience Network Connectivity in Autism Is Related to Brain and Behavioral
Markers of Sensory Overresponsivity. _J. Am. Acad. Child Adolesc. Psychiatry_ 55, 618–626 (2016). Article PubMed PubMed Central Google Scholar * Klin, A., Lin, D. J., Gorrindo, P.,
Ramsay, G. & Jones, W. Two-year-olds with autism orient to non-social contingencies rather than biological motion. _Nature_ 459, 257–261 (2009). Article CAS PubMed PubMed Central
Google Scholar * Spann, M. N., Monk, C., Scheinost, D. & Peterson, B. S. Maternal immune activation during the third trimester is associated with neonatal functional connectivity of the
salience network and fetal to toddler behavior. _J. Neurosci_. 2272–17, https://doi.org/10.1523/JNEUROSCI.2272-17.2018 (2018). * Scheinost, D. et al. Developmental trajectories of the
default mode, executive control, and salience networks from the third trimester through the newborn period. Preprint at https://doi.org/10.1101/2022.09.27.509687 (2022). * Gao, W., Lin, W.,
Grewen, K. & Gilmore, J. H. Functional Connectivity of the Infant Human Brain: Plastic and Modifiable. _Neurosci. Rev. J. Bringing Neurobiol. Neurol. Psychiatry_ 23, 169–184 (2017).
Google Scholar * Scheinost, D. et al. Hypoconnectivity between anterior insula and amygdala associates with future vulnerabilities in social development in a neurodiverse sample of
neonates. _Sci. Rep._ 12, 1–10 (2022). Article Google Scholar * Valenza, E., Simion, F., Cassia, V. M. & Umiltà, C. Face preference at birth. _J. Exp. Psychol._ 22, 892 (1996). CAS
Google Scholar * Tsang, T., Johnson, S., Jeste, S. & Dapretto, M. Social complexity and the early social environment affect visual social attention to faces. _Autism Res_. 1–13,
https://doi.org/10.1002/aur.2060 (2019). * Chawarska, K. & Shic, F. Looking But Not Seeing: Atypical Visual Scanning and Recognition of Faces in 2 and 4-Year-Old Children with Autism
Spectrum Disorder. _J. Autism Dev. Disord._ 39, 1663–1672 (2009). Article PubMed PubMed Central Google Scholar * Johnson, M. Cortical Maturation and the Development of Visual Attention
in Early Infancy. _J. Cogn. Neurosci._ 2, 81–95 (1990). Article CAS PubMed Google Scholar * Shultz, S., Klin, A. & Jones, W. Neonatal Transitions in Social Behavior and Their
Implications for Autism. _Trends Cogn. Sci._ 22, 452–469 (2018). Article PubMed PubMed Central Google Scholar * Frank, M. C., Amso, D. & Johnson, S. P. Visual search and attention to
faces during early infancy. _J. Exp. Child Psychol._ 118, 13–26 (2014). Article PubMed Google Scholar * Seeley, W. W. et al. Dissociable Intrinsic Connectivity Networks for Salience
Processing and Executive Control. _J. Neurosci._ 27, 2349–2356 (2007). Article CAS PubMed PubMed Central Google Scholar * Klin, A. et al. Infant viewing of social scenes is under
genetic control and is atypical in autism. _Nature_ 547, 340–344 (2017). Article PubMed PubMed Central Google Scholar * Gao, W. et al. Intersubject Variability of and Genetic Effects on
the Brain’s Functional Connectivity during Infancy. _J. Neurosci. J. Soc. Neurosci._ 34, 11288–11296 (2014). Article CAS Google Scholar * Mundy, P. & Newell, L. Attention, Joint
Attention, and Social Cognition. _Curr. Dir. Psychol. Sci._ 16, 269–274 (2007). Article PubMed PubMed Central Google Scholar * Insel, T. R. & Fernald, R. D. How the brain processes
social information: searching for the social brain. _Annu. Rev. Neurosci._ 27, 697–722 (2004). Article CAS PubMed Google Scholar * Stoner, R. et al. Patches of Disorganization in the
Neocortex of Children with Autism. _N. Engl. J. Med._ 370, 1209–1219 (2014). Article CAS PubMed PubMed Central Google Scholar * Piven, J., Elison, J. T. & Zylka, M. J. Toward a
conceptual framework for early brain and behavior development in autism. _Mol. Psychiatry Mol. Psychiatry_ 22, 1–10 (2017). Google Scholar * Miller, M. et al. School-age outcomes of infants
at risk for autism spectrum disorder. _Autism Res._ 9, 632–642 (2015). Article PubMed PubMed Central Google Scholar * Ozonoff, S. et al. Recurrence risk for autism spectrum disorders: a
Baby Siblings Research Consortium study. _Pediatrics_ 128, e488–e495 (2011). Article PubMed PubMed Central Google Scholar * Damaraju, E. et al. Functional connectivity in the developing
brain: A longitudinal study from 4 to 9months of age. _NeuroImage_ 84, 169–180 (2014). Article CAS PubMed Google Scholar * Fransson, P., Aden, U., Blennow, M. & Lagercrantz, H. The
functional architecture of the infant brain as revealed by resting-state fMRI. _Cereb. Cortex N. Y. N._ 21, 145–154 (2011). Article Google Scholar * Liu, W. C., Flax, J. F., Guise, K. G.,
Sukul, V. & Benasich, A. A. Functional connectivity of the sensorimotor area in naturally sleeping infants. _Brain Res._ 1223, 42–49 (2008). Article CAS PubMed Google Scholar *
Mullen, E. _Mullen Scales of Early Learning_ (AGS, 1995). * Mundy, P., Delgado, C. & Block, J. _Early social communication scales (ESCS)_ (Coral Gables FL, 2003). * Bryson, S. E.,
Zwaigenbaum, L., McDermott, C., Rombough, V. & Brian, J. The Autism Observation Scale for Infants: scale development and reliability data. _J. Autism Dev. Disord._ 38, 731–738 (2008).
Article PubMed Google Scholar * Dunn, W. & Daniels, D. B. Development of the Infant / Toddler Sensory Profile. _OTJR_ 27–41, https://doi.org/10.1177/105381510202500104 (2000). *
Frank, M. C., Vul, E. & Johnson, S. P. Development of infants’ attention to faces during the first year. _Cognition_ 110, 160–170 (2009). Article PubMed Google Scholar * Kruschke, J.
K. & Liddell, T. M. The Bayesian New Statistics: Hypothesis testing, estimation, meta-analysis, and power analysis from a Bayesian perspective. _Psychon. Bull. Rev._ 13, 1–28 (2017).
Google Scholar * Kruschke, J. K. & Vanpaemel, W. Bayesian estimation in hierarchical models. In _The Oxford Handbook of Computational and Mathematical Psychology_ (eds. Busemeyer, J.
R., Wang, Z., Townsend, J. T. & Eidels, A.) 279–299 (Oxford University Press, Oxford, UK, 2015). * Jenkinson, M., Beckmann, C. F., Behrens, T. E. J., Woolrich, M. W. & Smith, S. M.
Fsl. _NeuroImage_ 62, 782–790 (2012). Article PubMed Google Scholar * Shi, F. et al. Infant brain atlases from neonates to 1- and 2-year-olds. _PLoS One_ 6, e18746 (2011). * Pruim, R. H.
R. et al. ICA-AROMA: A robust ICA-based strategy for removing motion artifacts from fMRI data. _NeuroImage_ 112, 267–277 (2015). Article PubMed Google Scholar * Pruim, R. H. R., Mennes,
M., Buitelaar, J. K. & Beckmann, C. F. Evaluation of ICA-AROMA and alternative strategies for motion artifact removal in resting state fMRI. _NeuroImage_ 112, 278–287 (2015). Article
PubMed Google Scholar * Power, J. D., Barnes, K. A., Snyder, A. Z., Schlaggar, B. L. & Petersen, S. E. Spurious but systematic correlations in functional connectivity MRI networks
arise from subject motion. _NeuroImage_ 59, 2142–2154 (2012). Article PubMed Google Scholar * Murphy, K. & Fox, M. D. Towards a consensus regarding global signal regression for
resting state functional connectivity MRI. _NeuroImage_ 154, 169–173 (2017). Article PubMed Google Scholar * Woolrich, M. W. et al. Bayesian analysis of neuroimaging data in FSL.
_NeuroImage_ 45, S173–S186 (2009). Article PubMed Google Scholar * Yarkoni, T. et al. Large-scale automated synthesis of human functional neuroimaging data. _Nat. Methods_ 8, 665–670
(2012). Article Google Scholar * Weng, S.-J. et al. Alterations of resting state functional connectivity in the default network in adolescents with autism spectrum disorders. _Brain Res._
1313, 202–214 (2010). Article CAS PubMed Google Scholar * Uddin, L. Q., Supekar, K. & Menon, V. Reconceptualizing functional brain connectivity in autism from a developmental
perspective. _Front. Hum. Neurosci._ 7, 458 (2013). Article PubMed PubMed Central Google Scholar * Gao, W. et al. Temporal and spatial evolution of brain network topology during the
first two years of life. _PLoS One_ 6, e25278 (2011). * Geschwind, D. H. & Levitt, P. Autism spectrum disorders: developmental disconnection syndromes. _Curr. Opin. Neurobiol._ 17,
103–111 (2007). Article CAS PubMed Google Scholar * Fox, M. D. & Raichle, M. E. Spontaneous fluctuations in brain activity observed with functional magnetic resonance imaging. _Nat.
Rev. Neurosci._ 8, 700–711 (2007). Article CAS PubMed Google Scholar * Lakens, D., Scheel, A. M. & Isager, P. M. Equivalence Testing for Psychological Research: A Tutorial. _Adv.
Methods Pract. Psychol. Sci._ 1, 259–269 (2018). Article Google Scholar * Tsang, T., et al. Salience Netowrk Connectivity is Altered in 6-Week-Old Infants at Heightened Likelihood for
Developing Autism. https://doi.org/10.5281/zenodo.8403701 (2023). * Tsang, T., et al. Altered Salience Network Connectivity in 6-Week-Old Infants at Risk for Autism (NDA 2338) [Data Set].
NIMH Data Archive. https://doi.org/10.15154/qx4v-t626 (2023). Download references ACKNOWLEDGEMENTS This work was supported by grants from National Institute of Child Health and Human
Development (NICHD P50 HD055784, F31HD090937). The content is solely the responsibility of the authors and does not necessarily represent the official views of the National Institutes of
Health. The authors are grateful for the generous support from the Brain Mapping Medical Research Organization, Brain Mapping Support Foundation, Pierson-Lovelace Foundation, Ahmanson
Foundation, Capital Group Companies Charitable Foundation, William M. and Linda R. Dietel Philanthropic Fund, and Northstar Fund. The authors also wish to thank the infants and their
families for their time and participation in the study, Carolyn Ponting and Rosemary McCarron for their assistance in project coordination and data collection, Megan Banchik for her
assistance in data quality assurance, and the Child and Adult Neurodevelopmental Clinic for their contribution in behavioral characterization of the sample.Data and used in the preparation
of this manuscript has been submitted to the National Institute of Mental Health (NIMH) Data Archive (NDA). NDA is a collective informatics system created by the National Institutes of
Health to provide a national resource to support and accelerate research in mental health. Dataset identifier: 10.15154/qx4v-t626. This manuscript reflects the views of the authors and may
not reflect the opinions or views of the NIH. AUTHOR INFORMATION AUTHORS AND AFFILIATIONS * Meta, Menlo Park, CA, USA Tawny Tsang * Ahmanson-Lovelace Brain Mapping Center, University of
California, Los Angeles, Los Angeles, CA, USA Shulamite A. Green & Mirella Dapretto * Department of Psychiatry and Biobehavioral Sciences, University of California, Los Angeles, Los
Angeles, CA, USA Shulamite A. Green, Susan Y. Bookheimer & Mirella Dapretto * Semel Institute of Neuroscience and Human Behavior, David Geffen School of Medicine, University of
California, Los Angeles, Los Angeles, CA, USA Shulamite A. Green, Susan Y. Bookheimer & Mirella Dapretto * Center for Cognitive Neuroscience, University of California, Los Angeles, Los
Angeles, CA, USA Shulamite A. Green & Susan Y. Bookheimer * Cedars Sinai Hospital, Los Angeles, CA, USA Janelle Liu * Keck School of Medicine, University of Southern California, Los
Angeles, CA, USA Katherine Lawrence * Children’s Hospital Los Angeles, USC Keck School of Medicine, Los Angeles, CA, USA Shafali Jeste Authors * Tawny Tsang View author publications You can
also search for this author inPubMed Google Scholar * Shulamite A. Green View author publications You can also search for this author inPubMed Google Scholar * Janelle Liu View author
publications You can also search for this author inPubMed Google Scholar * Katherine Lawrence View author publications You can also search for this author inPubMed Google Scholar * Shafali
Jeste View author publications You can also search for this author inPubMed Google Scholar * Susan Y. Bookheimer View author publications You can also search for this author inPubMed Google
Scholar * Mirella Dapretto View author publications You can also search for this author inPubMed Google Scholar CONTRIBUTIONS T.T. and M.D. developed the initial aims and design of the
study. J.L. and T.T. contributed to the data acquisition and statistical analyses. K.L assisted in the processing and analyses of the data. T.T., S.G., S.J., S.Y.B. and M.D. interpreted the
findings and contributed to the manuscript. T.T. wrote the initial draft of the manuscript and all authors revised and commented on drafts of the manuscript. CORRESPONDING AUTHOR
Correspondence to Mirella Dapretto. ETHICS DECLARATIONS COMPETING INTERESTS The authors declare no competing interests. PEER REVIEW PEER REVIEW INFORMATION _Communications Biology_ thanks
Xiujuan Geng and the other, anonymous, reviewer(s) for their contribution to the peer review of this work. Primary Handling Editors: Sahba Besharati and George Inglis. ADDITIONAL INFORMATION
PUBLISHER’S NOTE Springer Nature remains neutral with regard to jurisdictional claims in published maps and institutional affiliations. SUPPLEMENTARY INFORMATION SUPPLEMENTARY INFORMATION
DESCRIPTION OF ADDITIONAL SUPPLEMENTARY FILES SUPPLEMENTARY DATA 1 REPORTING SUMMARY RIGHTS AND PERMISSIONS OPEN ACCESS This article is licensed under a Creative Commons Attribution 4.0
International License, which permits use, sharing, adaptation, distribution and reproduction in any medium or format, as long as you give appropriate credit to the original author(s) and the
source, provide a link to the Creative Commons licence, and indicate if changes were made. The images or other third party material in this article are included in the article’s Creative
Commons licence, unless indicated otherwise in a credit line to the material. If material is not included in the article’s Creative Commons licence and your intended use is not permitted by
statutory regulation or exceeds the permitted use, you will need to obtain permission directly from the copyright holder. To view a copy of this licence, visit
http://creativecommons.org/licenses/by/4.0/. Reprints and permissions ABOUT THIS ARTICLE CITE THIS ARTICLE Tsang, T., Green, S.A., Liu, J. _et al._ Salience network connectivity is altered
in 6-week-old infants at heightened likelihood for developing autism. _Commun Biol_ 7, 485 (2024). https://doi.org/10.1038/s42003-024-06016-9 Download citation * Received: 20 March 2023 *
Accepted: 06 March 2024 * Published: 22 April 2024 * DOI: https://doi.org/10.1038/s42003-024-06016-9 SHARE THIS ARTICLE Anyone you share the following link with will be able to read this
content: Get shareable link Sorry, a shareable link is not currently available for this article. Copy to clipboard Provided by the Springer Nature SharedIt content-sharing initiative