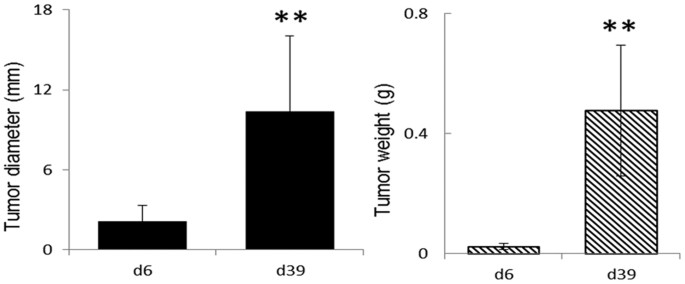
Tumor growth affects the metabonomic phenotypes of multiple mouse non-involved organs in an a549 lung cancer xenograft model
- Select a language for the TTS:
- UK English Female
- UK English Male
- US English Female
- US English Male
- Australian Female
- Australian Male
- Language selected: (auto detect) - EN
Play all audios:

ABSTRACT The effects of tumorigenesis and tumor growth on the non-involved organs remain poorly understood although many research efforts have already been made for understanding the
metabolic phenotypes of various tumors. To better the situation, we systematically analyzed the metabolic phenotypes of multiple non-involved mouse organ tissues (heart, liver, spleen, lung
and kidney) in an A549 lung cancer xenograft model at two different tumor-growth stages using the NMR-based metabonomics approaches. We found that tumor growth caused significant metabonomic
changes in multiple non-involved organ tissues involving numerous metabolic pathways, including glycolysis, TCA cycle and metabolisms of amino acids, fatty acids, choline and nucleic acids.
Amongst these, the common effects are enhanced glycolysis and nucleoside/nucleotide metabolisms. These findings provided essential biochemistry information about the effects of tumor growth
on the non-involved organs. SIMILAR CONTENT BEING VIEWED BY OTHERS FEATURES OF METABOLISM ASSOCIATED MOLECULAR PATTERNS IN PANCREATIC DUCTAL ADENOCARCINOMA Article 06 July 2023 TARGETED
METABOLOMIC PROFILING AS A TOOL FOR DIAGNOSTICS OF PATIENTS WITH NON-SMALL-CELL LUNG CANCER Article Open access 08 July 2023 METABOLIC HETEROGENEITY IN CANCER Article 24 January 2024
INTRODUCTION Cancers collectively remain as a type of most serious diseases globally with high mortality. Even after decades’ efforts, the situation showed no outstanding improvement with
more than 14 million newly diagnosed cancer cases and 8 million mortality in 2012 worldwide1. In United States alone, for instance, there were 1.6 million new cancer cases in 2015 with half
a million cancer-caused deaths2. Amongst all cancers, lung cancer accounted for about 13% of the newly diagnosed cancers but 27% of the cancer-caused mortality2 with non-small cell lung
cancer (NSCLC) accounting for approximately 84% of the lung cancer, half of which are metastatic at time of diagnosis3,4. It is now well known that unique metabolic phenotypes are present
with largely enhanced glycolysis and lactate elevations in solid tumors, which is known as “Warburg effects”5. Choline metabolism involving Kennedy pathway (or cytidine diphosphate choline
cycle) is also characteristically different between malignant tumors and non-cancerous tissues with promoted phosphorylcholine biosynthesis in solid malignant tumors due to different demands
for cell membrane metabolism6. Furthermore, cancer tissues differ considerably from non-cancerous tissues in their metabolism of fatty acid and nucleic acids7,8. The combined such metabolic
alterations have been widely reported in many different cancerous tissues, compared with the adjacent non-involved tissues, including human liver cancer9, colorectal cancer10,11,12, lung
cancer13, thyroid tumor14,15, bladder cancer16 and brain tissues17. It is conceivable that tumorigenesis and tumor growth at a specific organ sites demand more energy and nutrition that can
be mobilized from other non-involved organs. Tumor angiogenesis, which generally occurs when tumor reaches about 1–2 mm in diameter, is essential to meet such demands and requirement of
other essential supplies including hormones, growth factors and proteolytic enzymes for further growth and metastatic dissemination18,19,20. However, the effects of tumorigenesis and tumor
growth on the biochemistry of other non-involved organs remain largely unknown. Such information is conceivably important for understanding not only the effects of tumors on patients as a
whole but also the therapeutic effects of effective treatments. By using the NMR-based metabonomics approaches in this study, we comprehensively analyzed the metabonomic phenotypes of
multiple organs (heart, liver, spleen, lung and kidney) for an A549 lung cancer xenograft mouse model at two tumor growth stages with tumor diameters of about 2 and 10 millimeters. We also
analyzed the metabonomic phenotypes of these tumor tissues and the tumor-growth induced concurrent urinary and serum metabonomic changes as complementary information. The objective of this
work is to define the effects of lung tumor growth on metabolism of the organs which are not directly involved prior to metastasis. RESULTS PHENOTYPES OF A549 XENOGRAFT MICE On day 6 post
inoculation (d6PI) with A549 cells, tumor size in the A549 xenograft nude mice was about 2 mm in diameter with an average weight of about 0.02 g (n = 10) representing pre-angiogenesis tumor
growth. On day 39 post inoculation (d39PI), the tumor sizes (n = 10) were significantly larger than that on d6PI reaching about 10 mm in diameter with an average weight of about 0.48 g (Fig.
1). There were no significant differences in histological features for tissues from heart, liver, spleen, lung and kidney between control and xenograft tumor model mice even on d39PI
(Supplementary Fig. S1). This confirmed that on d39PI tumor reached the post-angiogenesis but pre-metastasis growth stage. No significant differences were detected in animal body weights
between control and tumor groups (Fig. S2). ASSIGNMENTS AND INTERGROUP DIFFERENCES FOR METABOLITES The average 1H NMR spectra of tissue extracts from ten mouse organs (see Supplementary Fig.
S3) showed abundant information of metabolites, which were assigned according to the literature data21,22,23 and confirmed individually with two-dimensional (2D) NMR spectral data24 (see
Supplementary Table S1). These metabolites included mainly amino acids, lipids (glycerides and cholesterol), ketone body (3-hydroxybutyrate), carbohydrates (glycogen, glucose and mannose),
glycolytic metabolites (pyruvate and lactate), TCA cycle intermediates (citrate, succinate and fumarate), bile acids, metabolites of nucleic acids (uridine, uracil, inosine, guanosine,
xanthine and hypoxanthine), formate, acetate, taurine, nicotinamide, some acetyl-glycoproteins together with choline metabolites including choline, phosphorylcholine (PC),
glycerophosphorylcholine (GPC), dimethylamine, trimethylamine (TMA), dimethylglycine, creatine and ethanolamine (see Supplementary Fig. S3). Differential metabograms derived from the
multiple univariate data analysis (MUDA)21 showed that, on d6PI, only a few metabolites in all these organ tissues had significant differences between the tumor and control groups (n = 10,
see Fig. 2). On d39PI, in contrast, many more metabolites showed significant intergroup differences between tumor and control groups (Fig. 2). Signal integrals of the metabolites showing
significant intergroup differences (_p_ < 0.05) were further analyzed and their corresponding _p-_values were tabulated in Table 1. The tumor-growth induced changes of metabolites were
also calculated for both d6PI and d39PI as the ratios of metabolite changes against corresponding control groups (Fig. 3). TUMOR GROWTH INDUCED METABOLIC CHANGES IN HEART TISSUES On d6PI,
there were no significantly differentiated metabolites between control and the tumor groups. On d39PI, however, lactate and alanine levels were significantly higher whereas the levels of
glucose, pyruvate, aspartate, choline, GPC, inosinate and nicotinamide were significantly lower in the tumor group than in controls (Table 1, Fig. 3). Lung tumor growth led to about 25%
level increases for lactate and alanine but more than 65% declines for glucose and inosinate. Tumor growth also induced about 20–35% decreases for the levels of aspartate, pyruvate, choline,
GPC and nicotinamide (Table 1, Fig. 3). TUMOR GROWTH INDUCED METABOLIC CHANGES IN LIVER TISSUES The levels of hepatic GPC and taurine in tumor group showed significant decreases (15–20%) on
d6PI compared to control (Table 1, Fig. 3). On d39PI, in contrast, tumor growth caused much more significant metabolic changes highlighted by level elevations for glucose, lactate, alanine
and TMA but level declines for guanosine, inosinate, uracil, inosine, hypoxanthine, GPC and nicotinamide. Amongst them, tumor growth caused about 85% level increase hepatic TMA and 55%
increase for lactate level with concurrent about 55% decrease for guanosine and inosinate (Fig. 3). The tumor-growth induced level increases for alanine and glucose were about 25–35% whereas
level decreases for GPC, uracil, inosine, hypoxanthine and nicotinamide ranged around 15–35% (Fig. 3). TUMOR GROWTH INDUCED METABOLIC CHANGES IN KIDNEY TISSUES On d6PI, tumor growth only
caused significant reduction (about 10–20%) in the levels of renal alanine, acetate, creatine and hypoxanthine (Table 1, Fig. 3). In contrast, tumor growth for 39 days induced significant
elevations in succinate level (about 35%) together with lactate, uracil and hypoxanthine (20–25%). Tumor-growth for 39 days also caused level reductions for glucose (about 40%) together with
inosine and guanosine (about 10–15%) (Table 1, Fig. 3). TUMOR GROWTH INDUCED METABOLIC CHANGES IN SPLEEN TISSUES On d6PI, the levels of 3-hydroxybutyrate, citrate, inosine, guanosine and
nicotinamide were about 15–25% higher in spleen tissues of tumor group than in controls (Table 1, Fig. 3). On d39PI, tumor growth caused significant elevation of lactate (about 53%) with
concurrent level decline for glucose (about 55%) in spleen. Tumor-growth also resulted in significant decreases (10–30%) in the levels of 3-hydroxybutyrate, citrate, choline, PC, taurine,
inosine and guanosine (Table 1, Fig. 3). TUMOR GROWTH INDUCED METABOLIC CHANGES IN NON-INVOLVED LUNG TISSUES The tumor-growth induced metabolic changes were detected in lung tissue even
though in this xenograft model tumor only grew at mouse armpit without any detectable histological changes in lung tissue. For example, after A549 inoculation for 6 days, the levels of TMA
and inosine in lung tissue of the tumor group were about 28% and 15%, respectively, higher than in controls (Table 1, Fig. 3). In contrast, tumor-growth for 39 days led to significant level
elevations for lactate (about 45%) and alanine (about 20%) but decreases for glucose (about 40%) and for acetate, choline, inosine as well as guanosine (about 10–20%) (Table 1, Fig. 3).
TUMOR GROWTH INDUCED METABOLIC CHANGES IN URINE, SERUM AND TUMOR TISSUE SAMPLES Both urinary and serum metabonomic responses to such tumor growth were mild in both tumor growth stages (Fig.
3). Tumor tissues at two different growth stages showed some significant differences in their choline metabolism (see Supplementary Fig. S4). DISCUSSION Growth of solid tumors often
accompanies with induced angiogenesis when tumor size reaches about 1–2 mm in diameter so as to meet the increased demands for energy, nutrition and other essential supplies such as
hormones, growth factors and proteolytic enzymes for further growth and metastatic dissemination18,19,20. Such demands inevitably require the mobilization of energy, nutrients and other
metabolites from the organs which are not directly involved in tumorigenesis. Therefore, tumor growth at a specific organ site is expected to affect the biochemistry of those non-involved
organs as well even before metastasis. So far, little is known about the details of such effects though these effects are potentially important for understanding systems biology of cancer
pathogenesis, progression and treatments. Xenograft tumor animal models derived from human cancer cells were shown to be useful for studying the metabonomic phenotypic characteristics of the
various corresponding human cancers25,26. Here, we employed a classical A549 xenograft tumor mouse model for lung cancer so as to investigate how tumor-growth affects the metabolic
phenotypes of the non-involved organs. Such xenograft model was readily established via subcutaneous inoculation of A549 cells into the armpit of nude mice and were commonly used for
assessing anti-tumor efficacy of drugs and treatment schedules27. We focused on two time points, namely day 6 and 39 post inoculation (d6PI and d39PI). This is because that on d6PI, tumor
size was about 2mm in diameter (Fig. 1) representing the stage about angiogenesis whereas on d39PI, tumor size reached about 10 mm in diameter but with no detectable metastasis (see
Supplementary Fig. S1) representing more advanced post-angiogenesis but pre-metastatic stages. We found in this work that the growth of xenograft tumor induced substantial metabolic changes
in multiple non-involved organs upon angiogenesis (Fig. 4) though such changes appeared to be limited prior to angiogenesis. Tumor-growth causes significant alterations in glycolysis and TCA
cycle in multiple non-involved organs. Tumor growth for 6 days caused limited metabolic changes with kidney and spleen having more metabolic responses than the rest studied organs.
Elevations of nicotinamide, citrate and 3-hydroxybutyrate in spleen indicated promotion of fatty acid oxidation with nicotinamide mainly used for nicotinamide adenine di-nucleotide (NAD+)
biosynthesis28,29. The decline of creatine in kidney suggested enhancement of energy metabolism with the conversion of phosphocreatine into creatine to produce ATP30. Such notion is also
supported by observed level declines of serum creatine and glucose for these animals (Fig. 3). When reached more advanced post angiogenesis stage (i.e., on d39PI), tumor-growth caused
lactate elevation in all studied organs indicated the enhanced glycolysis which was supported by the concurrent level decline for glucose. Liver tissue showed elevation of glucose in this
case probably due to promoted glycogenolysis as well. The accompanied changes in alanine probably resulted from the same process since pyruvate produced from glycolysis could be converted
into alanine by alanine aminotransferase (ALT)31 and into lactate by lactate dehydrogenase32. This is further supported by significant level declines of serum glucose for the xenograft mice
(Fig. 3). After angiogenesis, tumor growth caused significant changes in nucleic acid metabolism in multiple non-involved organs reflected with significant declines in the levels both purine
and pyrimidine metabolites (hypoxanthine, inosine, inosinate, guanosine and uracil) in liver. This probably resulted from the hugely increased demands of nucleotides for biosynthesis of DNA
and RNA to ensure cancer cell proliferation during the tumor-growth. Such mobilization of metabolites will partially meet the demands for tumor growth with the rests synthesized by cancer
cells themselves via up-regulations of nucleotide biosynthesis using glutamine and glucose as raw materials33,34,35. Such level declines of nucleic acids were less in other organs such as
heart, lung and spleen than in liver with it functioning as the major metabolic organ and supplier of metabolites. It is also obvious that tumor-growth effects on nucleic acid metabolism
vary with organs. Heart tissue only showed level decline in inosinate (i.e., inosine monophosphate) whereas spleen, lung and kidney had level decreases for inosine and guanosine. The
responses of kidney toward xenograft tumor growth (elevation of uracil and hypoxanthine but declines of inosine and guanosine) suggested degradation of nucleosides and oxidative stress for
kidney. This is further supported by concurrent elevation of urinary allantoin (Fig. 3). Moreover, tumor growth induced alterations in choline metabolism in multiple non-involved organs.
Choline metabolism is critically important for membrane biosynthesis and degradation through so-called Kennedy cycle36. On one hand, choline is converted into PC by choline kinases and
further into phosphatidylcholines (PtC) functioning as the major components of cell membrane. On the other hand, GPC derived from PtC (or membrane) degradation can be converted into choline
to feed the biosynthesis of PC and PtC again36. It is now well known that the PC-to-GPC ratio (PGR) is drastically different for cancer cells (PGR > 1) and normal or benign cells (PGR
< 1)6. In general, therefore, tumor growth requires large amount of choline and PC to synthesize membrane PtC for rapid cell proliferation37 from both the _de novo_ biosynthesis of cancer
cells and other parts of body. Our observation of consistent level declines for choline, PC and GPC in these non-involved organs during xenograft tumor growth (Table 1, Fig. 3) suggested
that demands of membrane biosynthesis in xenograft lung tumor growth (Fig. S4) led to efflux of the choline metabolites from liver, heart and spleen. Currently, it is not clear what affects
such efflux-induced losses of metabolites and membrane degradations actually have on the physiological functions of these organs. It is interesting to observe the tumor-growth caused
elevation of TMA in liver and lung tissues. TMA in mammals is largely generated from cholines and carnitines by gut microbiota in gastrointestinal track and then absorbed into liver via
enterohepatic circulation. Volatility of TMA also enables it enter lung tissues through so-called air-blood exchange. Therefore, the aforementioned TMA changes in these non-involved organs
suggested tumor-growth might also affect the gut microbiota functions as well. This notion is further supported by the tumor-growth induced changes of gut microbial metabolites in our mouse
urinary samples such as hippurate and butyrate (Fig. 3). Since urinary hippurate is one of the mammal-microbiota co-metabolites, its changes implies importance of such co-metabolism in tumor
growth. The details of such clearly warrant further investigation and the integrative metabonomics and metagenomics approaches38,39 ought to be feasible, which is currently ongoing. To sum
up, our results indicated that tumor growth had systematic effects on metabolic functions of a number of non-involved organs. Such effects became much more marked during the pre-metastatic
post-angiogenesis stage and on more non-involved organs (e.g., liver, spleen, lung, heart and kidney) and probably microbiota functions as well. Xenograft tumor growth promoted glycolysis
and membrane degradations, altered TCA cycle, lipid metabolism and nucleotides biosynthesis in these non-involved organs with an outstanding shift of metabolites from the non-involved
organs. These findings offered vital information about tumor-growth effects on whole body biochemistry even prior to metastasis which might be of some importance for understanding the
tumorigenesis and progression in the systems level. MATERIALS AND METHODS REAGENTS Dulbecco’s modified Eagle’s medium (DMEM) and trypsin were purchased from Thermo Fisher Scientific Inc.
(Beijing, China). Fetal bovine serum (FBS) was obtained from Hangzhou Sijiqing Company. Sodium penicillin and streptomycin were purchased from North China Pharmaceutical Company (Hebei,
China). NaH2PO4 · 2H2O, K2HPO4 · 3H2O, K2CO3 and methanol (all in analytical grade) were purchased from Sinopharm Chemical Reagent Co. Ltd. (Shanghai, China). Deuterium oxide (D2O, 99.9%D)
and acetyl chloride were obtained from Sigma-Aldrich, Inc. (St. Louis, MO) and sodium 3-(trimethylsilyl) [2,2,3,3-2H4] propionate (TSP) were obtained from Cambridge Isotope Laboratories,
Inc. (Miami, U.S.A.). Phosphate buffer was prepared from NaH2PO4 and K2HPO4 with good low-temperature stability40 containing 0.001% TSP (w/v) and 80% D2O (0.15 M, pH 7.45) and employed as
solvent for NMR analysis of tissue extracts. CELL PREPARATION A549 cells (from human adenocarcinoma alveolar basal epithelial cells) were obtained from China Center for Type Culture
Collection in Wuhan University. A549 cells were cultured in a humidified atmosphere with 5% CO2 and 95% air at 37 °C in a medium containing DMEM, 10% fetal bovine serum, 100 U/mL sodium
penicillin and streptomycin. ANIMAL EXPERIMENTS AND SAMPLE COLLECTIONS All animal experiments were conducted in accordance with the National Guidelines for Experimental Animal Welfare (the
Ministry of Science and Technology, People’s Republic of China, 2006) using a certified SPF facility at the Animal Experiment Center of Hubei Province Cancer Hospital (Wuhan, China). The
animal experiment was approved by the local ethic committee of Hubei Province Cancer Hospital. All experimental protocols were in accordance with guidelines of Wuhan Institute of Physics and
Mathematics, University of Chinese Academy of Sciences. Female athymic nude mice (BALB/c, 3–4 weeks old) were obtained from Beijing Vitalriver Experimental Animal Technical Co., LTD. The
mice were housed in sterile cages under laminar airflow hoods in a local SPF experimental animal facility with a 12 hr light/dark cycle and constant temperature (to about 25 °C) and relative
humidity (to about 50%). All animals were allowed to have free access to normal mouse chow and water. After two weeks acclimatization, mice were inoculated with either A549 cells for the
tumor groups or medium for controls as schematically described in Supplementary Fig. S5. For xenograft tumor models, each nude mouse was inoculated with 0.15 mL suspension of A549 cells (5 ×
107 cells/mL) subcutaneously into its left armpit. Xenografting mice were randomly divided into two different groups (n = 10), namely, early stage (before angiogenesis) and late stage (post
angiogenesis but before metastasis) tumor groups respectively. The former was sacrificed on d6PI whereas the late stage tumor group was completed on d39PI. Two corresponding control groups
(n = 10) was treated in the same manner but with 0.15 mL medium. Animal body weights and food intakes were monitored. On d6PI and d39PI, animals were sacrificed with the tumor sizes obtained
by weighing tumor tissue and measuring tumor diameters _ex vivo_. These and all other non-involved tissue (heart, liver, spleen, lung and kidney) and biofluid (urine and serum) samples were
collected and snap-frozen with liquid nitrogen followed with storage in −80 °C freezer until further analysis. Some tissue samples from two groups (control and tumor group on day 39) were
randomly selected and fixed in 10% formalin for histopathological assessments. HISTOPATHOLOGICAL ASSESSMENT All tissues from the above selected animals were fixed in formalin followed with
staining their micro-sectioned slices with Hematoxylin and Eosin (H&E) methods. All histopathological assessments were conducted by a qualified pathologist microscopically as a paid
service. SAMPLE PREPARATIONS FOR METABONOMIC ANALYSIS Tissue metabolites were extracted as reported previously21,22,23 with some minor modifications. Briefly, each individual tissue sample
(about 50 mg) was weighed and extracted with 600 μL pre-cooled methanol-water mixture (2:1, v/v) using Tissuelyzer II (QIAGEN TissueLyser II, Germany) for 90 s at 20 Hz. The resultant
mixture was subjected to ultrasonication for 3 minutes in an ice both followed with centrifugation (11180 × g, 4 °C) for 10 min to collect the supernatant. This extraction procedure was
further repeated twice and so-obtained three supernatants from each sample were pooled together followed with another centrifugation. Methanol in the pooled supernatants for all samples was
removed _in vacuo_ and the resultant extracts were lyophilized. The freeze-dried extract for each sample was individually dissolved in Na-K phosphate buffer (600 μL, pH 7.45) and 550 μL of
the supernatant was individually transferred into a 5 mm NMR tube for each sample for further NMR analysis. NMR MEASUREMENTS All NMR spectra for tissue extracts were acquired at 298 K on a
Bruker AVANCE III600 MHz NMR spectrometer equipped with an inverse-detection optimized cryogenic probe (Bruker-Biospin, Germany). One-dimensional 1H NMR spectra were acquired for all samples
using the first increment of the gradient selected NOESY pulse sequence (RD−90°−t1−90°−tm−90°−acquisition) with water signal presaturated during both the mixing time (tm) of 80 ms and
recycle delay (RD) of 2 s. The inter-pulse delay t1 was set to about 4 μs and 90° pulse length was adjusted to about 10 μs. 128 transients were recorded into 32k data points with the
spectral width of 20 ppm. Serum and urinary spectra were acquired as previously reported40,41,42. For resonance assignment purposes, a series of 2D NMR spectra were acquired for selected
samples and processed as previously reported28,29. These included 1H−1H correlation spectroscopy (COSY), 1H J-resolved spectroscopy (JRES),1H−1H total correlation spectroscopy (TOCSY),
1H−13C heteronuclear single quantum correlation spectroscopy (HSQC) and 1H−13C heteronuclear multiple bond correlation spectroscopy (HMBC). NMR DATA PROCESSING AND DATA ANALYSIS Free
induction decays (FID) were multiplied by an exponential window function with 1 Hz line broadening prior to Fourier transformation. All spectra were phase- and baseline-corrected manually
and referenced internally to the chemical shift of TSP (δ 0.00) using Topspin software package (V3.0, Bruker-Biospin, Germany). The δ 0.6–9.3 region was bucketed into bins of 0.004 ppm using
AMIX software package (V3.9.2, Bruker-Biospin, Germany). The regions of δ 4.20–5.20 were discarded prior to analysis to eliminate the effects of imperfect water-saturation. All integrated
bins were normalized to the wet weight for tissue samples extracts to represent absolute metabolite quantity in the form of peak-area per mg tissue. All above normalized bins (i.e.,
variables) were subjected to either nonparametric tests or the Student’s t-test as appropriate21 using an in-house developed MATLAB script. Subsequently, differential metabograms were
generated by color-coding all variables (or metabolites) with their corresponding _p_-values in the forms of loadings plots, where the red-colored variables showed significant intergroup
differences (_p_ < 0.05). Characteristic peaks of the metabolites showing significant intergroup differences were also curve-fitted from original NMR spectra using MestReNova (V8.1.0,
Mestrelab Research S. L.) to obtain their peak areas that were subjected to further analysis with the Student’s t-test and Kruskal−Wallis test as appropriate. The ratios of concentration
changes for metabolites showing significant intergroup differences were then calculated as previously reported22,23 in the form of (CT−CCD)/CCD, where CT and CCD represented the metabolite
concentrations in tumor and control groups, respectively. The PC-to-GPC ratio for all samples was individually calculated from the signal integrals of their methyl groups using the same
curve-fitting method. ADDITIONAL INFORMATION HOW TO CITE THIS ARTICLE: Xu, S. _et al._ Tumor growth affects the metabonomic phenotypes of multiple mouse non-involved organs in an A549 lung
cancer xenograft model. _Sci. Rep._ 6, 28057; doi: 10.1038/srep28057 (2016). REFERENCES * Torre, L. A. et al. Global cancer statistics, 2012. Ca-Cancer J. Clin. 65, 87–108 (2015). Article
Google Scholar * Siegel, R. L., Miller, K. D. & Jemal, A. Cancer statistics, 2015. Ca-Cancer J. Clin. 65, 5–29 (2015). Article Google Scholar * Detterbeck, F. C., Boffa, D. J. &
Tanoue, L. T. The new lung cancer staging system. Chest 136, 260–271 (2009). Article Google Scholar * Goulart, B. H. et al. Referral and treatment patterns among patients with stages III
and IV non-small-cell lung cancer. J. Oncol. Pract. 9, 42–50 (2013). Article Google Scholar * Vander Heiden, M. G., Cantley, L. C. & Thompson, C. B. Understanding the warburg effect:
the metabolic requirements of cell proliferation. Science 324, 1029–1033 (2009). Article CAS ADS Google Scholar * Glunde, K., Bhujwalla, Z. M. & Ronen, S. M. Choline metabolism in
malignant transformation. Nat. Rev. Cancer 11, 835–848 (2011). Article CAS Google Scholar * Carracedo, A., Cantley, L. C. & Pandolfi, P. P. Cancer metabolism: fatty acid oxidation in
the limelight. Nat. Rev. Cancer 13, 227–232 (2013). Article CAS Google Scholar * Currie, E., Schulze, A., Zechner, R., Walther, T. C. & Farese, R. V. Cellular fatty acid metabolism
and cancer. Cell Metab. 18, 153–161 (2013). Article CAS Google Scholar * Yang, Y. X. et al. Metabonomic studies of human hepatocellular carcinoma using high-resolution magic-angle
spinning H-1 NMR spectroscopy in conjunction with multivariate data analysis. J. Proteome Res. 6, 2605–2614 (2007). Article CAS Google Scholar * Bertini, I. et al. Metabolomic NMR
fingerprinting to identify and predict survival of patients with metastatic colorectal cancer. Cancer Res. 72, 356–364 (2012). Article CAS Google Scholar * Tian, Y. et al. Tissue
Metabonomic phenotyping for diagnosis and prognosis of human colorectal cancer. Sci. Rep. 6, 20790, doi: 10.1038/srep20790 (2016). Article CAS ADS PubMed PubMed Central Google Scholar
* Mirnezami, R. et al. Rapid diagnosis and staging of colorectal cancer via high-resolution magic angle spinning nuclear magnetic resonance (HR-MAS NMR) spectroscopy of intact tissue
biopsies. Ann. Surg. 259, 1138–1149 (2014). Article Google Scholar * Rocha, C. M. et al. Metabolic profiling of human lung cancer tissue by 1H high resolution magic angle spinning (HRMAS)
NMR spectroscopy. J. Proteome Res. 9, 319–332 (2010). Article CAS Google Scholar * Torregrossa, L. et al. Toward the reliable diagnosis of indeterminate thyroid lesions: a HRMAS NMR-based
metabolomics case of study. J. Proteome Res. 11, 3317–3325 (2012). Article CAS Google Scholar * Tian, Y. et al. Integrative metabonomics as potential method for diagnosis of thyroid
malignancy. Sci. Rep. 5, 14869, doi: 10.1038/srep14869 (2015). Article CAS ADS PubMed PubMed Central Google Scholar * Chan, E. C. Y. et al. Metabonomic profiling of bladder cancer. J.
Proteome Res. 14, 587–602 (2015). Article CAS Google Scholar * Dali-Youcef, N. et al. Gene expression mapping of histone deacetylases and co-factors and correlation with survival time and
H-1-HRMAS metabolomic profile in human gliomas. Sci. Rep. 5, 9087, doi: 10.1038/srep09087 (2015). Article CAS PubMed PubMed Central Google Scholar * Bergers, G. & Benjamin, L. E.
Tumorigenesis and the angiogenic switch. Nat. Rev. Cancer 3, 401–410 (2003). Article CAS Google Scholar * Hicklin, D. J. & Ellis, L. M. Role of the vascular endothelial growth factor
pathway in tumor growth and angiogenesis. J. Clin. Oncol. 23, 1011–1027 (2005). Article CAS Google Scholar * Hanahan, D. & Weinberg, R. A. Hallmarks of cancer: the next generation.
Cell 144, 646–674 (2011). Article CAS Google Scholar * Duan, Y. X. et al. Multiple univariate data analysis reveals the inulin effects on the high-fat-diet induced metabolic alterations
in rat myocardium and testicles in the preobesity state. J. Proteome Res. 12, 3480–3495 (2013). Article CAS Google Scholar * Li, D. et al. Metabonomic changes associated with
atherosclerosis progression for LDLR(−/−) mice. J. Proteome Res. 14, 2237–2254 (2015). Article CAS Google Scholar * Li, N. et al. Antagonist of prostaglandin E2 receptor 4 induces
metabolic alterations in liver of mice. J. Proteome Res. 14, 1566–1573 (2015). Article CAS Google Scholar * Wu, J. F. et al. Metabolic changes reveal the development of schistosomiasis in
mice. Plos Neglect. Trop. Dis. 4, doi: 10.1371/journal.pntd.0000807 (2010). * Seierstad, T. et al. Principal component analysis for the comparison of metabolic profiles from human rectal
cancer biopsies and colorectal xenografts using high-resolution magic angle spinning 1H magnetic resonance spectroscopy. Mol. Cancer 7, 33, doi: 10.1186/1476-4598-7-33 (2008). Article CAS
PubMed PubMed Central Google Scholar * Loftus, N. J. et al. Global metabolite profiling of human colorectal cancer xenografts in mice using HPLC-MS/MS. J. Proteome Res. 12, 2980–2986
(2013). Article CAS Google Scholar * Pan, H., Ma, Z. & Mao, L. Establishment of nude mouse models of patient-derived lung cancer xenograft. Chin. J. Oncol. 36, 571–574 (2014). Google
Scholar * Magni, G. et al. Enzymology of NAD+ homeostasis in man. Cell Mol. Life Sci. 61, 19–34 (2004). Article CAS Google Scholar * Rongvaux, A., Andris, F., Van Gool, F. & Leo, O.
Reconstructing eukaryotic NAD metabolism. Bioessays 25, 683–690 (2003). Article CAS Google Scholar * Bong, S. M. et al. Structural studies of human brain-type creatine kinase complexed
with the ADP-Mg2+-NO3− -creatine transition-state analogue complex. FEBS Lett. 582, 3959–3965 (2008). Article CAS Google Scholar * Adeva-Andany, M. et al. Comprehensive review on lactate
metabolism in human health. Mitochondrion 17, 76–100 (2014). Article CAS Google Scholar * Rogatzki, M. J., Ferguson, B. S., Goodwin, M. L. & Gladden, L. B. Lactate is always the end
product of glycolysis. Front. Neurosci. 9, doi: 10.3389/fnins.2015.00022 (2015). * Tong, X., Zhao, F. & Thompson, C. B. The molecular determinants of de novo nucleotide biosynthesis in
cancer cells. Curr. Opin. Genet. Dev. 19, 32–37 (2009). Article CAS Google Scholar * Sun, L. C. et al. cMyc-mediated activation of serine biosynthesis pathway is critical for cancer
progression under nutrient deprivation conditions. Cell Res. 25, 429–444 (2015). Article CAS ADS Google Scholar * Li, H. D. et al. The metabolic responses to hepatitis B virus infection
shed new light on pathogenesis and targets for treatment. Sci. Rep. 5, 8421, doi: 10.1038/srep08421 (2015). Article CAS PubMed PubMed Central Google Scholar * Fagone, P. &
Jackowski, S. Phosphatidylcholine and the CDP-choline cycle. Biochim. Biophys. Acta. 1831, 523–532 (2013). Article CAS Google Scholar * Awwad, H. M., Geisel, J. & Obeid, R. The role
of choline in prostate cancer. Clin. Biochem. 45, 1548–1553 (2012). Article CAS Google Scholar * Hewezi, T. et al. Arabidopsis spermidine synthase is targeted by an effector protein of
the cyst nematode Heterodera schachtii. Plant Physiol. 152, 968–984 (2010). Article CAS Google Scholar * Lin, H., An, Y., Hao, F., Wang, Y. & Tang, H. Correlations of fecal
metabonomic and microbiomic changes induced by high-fat diet in the pre-obesity state. Sci. Rep. 6, 21618, doi: 10.1038/srep21618 (2016). Article CAS ADS PubMed PubMed Central Google
Scholar * Jiang, L. M., Huang, J., Wang, Y. L. & Tang, H. R. Eliminating the dication-induced intersample chemical-shift variations for NMR-based biofluid metabonomic analysis. Analyst
137, 4209–4219 (2012). Article CAS ADS Google Scholar * Xu, W. X. et al. Streptozotocin-induced dynamic metabonomic changes in rat biofluids. J. Proteome Res. 11, 3423–3435 (2012).
Article CAS Google Scholar * Zhang, L. M. et al. Metabonomic analysis reveals efficient ameliorating effects of acupoint stimulations on the menopause-caused alterations in mammalian
metabolism. Sci. Rep. 4, 3641, doi: 10.1038/srep03641 (2014). Article CAS PubMed PubMed Central Google Scholar Download references ACKNOWLEDGEMENTS We acknowledge the financial supports
from the Ministry of Science and Technology of China (2012CB934004), the National Natural Science Foundation of China (81590950, 21175149, 91439102, 81590953, 21375144 and 21305155). AUTHOR
INFORMATION Author notes * Xu Shan and Tian Yuan contributed equally to this work. AUTHORS AND AFFILIATIONS * Key Laboratory of Pesticide and Chemical Biology, Ministry of Education,
College of Chemistry, Central China Normal University, 430079, Wuhan, China Shan Xu, Nijia Zhang, Dandan Song, Zhengshun Wu & Yanfang Cui * CAS Key Laboratory of Magnetic Resonance in
Biological Systems, State Key Laboratory of Magnetic Resonance and Atomic and Molecular Physics, National Centre for Magnetic Resonance in Wuhan, Wuhan Institute of Physics and Mathematics,
University of Chinese Academy of Sciences, 430071, Wuhan, China Shan Xu, Yuan Tian, Yili Hu & Yulan Wang * Department of Medical Oncology, Hubei Province Cancer Hospital, 430079, Wuhan,
China Sheng Hu * Collaborative Innovation Center for Diagnosis and Treatment of Infectious Diseases, Zhejiang University, 310058, Hangzhou, China Yulan Wang * State Key Laboratory of Genetic
Engineering, Collaborative Innovation Center for Genetics and Development, Ministry of Education Key Laboratory of Contemporary Anthropology, Metabonomics and Systems Biology Laboratory,
School of Life Sciences, Fudan University, 200438, Shanghai, China Huiru Tang Authors * Shan Xu View author publications You can also search for this author inPubMed Google Scholar * Yuan
Tian View author publications You can also search for this author inPubMed Google Scholar * Yili Hu View author publications You can also search for this author inPubMed Google Scholar *
Nijia Zhang View author publications You can also search for this author inPubMed Google Scholar * Sheng Hu View author publications You can also search for this author inPubMed Google
Scholar * Dandan Song View author publications You can also search for this author inPubMed Google Scholar * Zhengshun Wu View author publications You can also search for this author
inPubMed Google Scholar * Yulan Wang View author publications You can also search for this author inPubMed Google Scholar * Yanfang Cui View author publications You can also search for this
author inPubMed Google Scholar * Huiru Tang View author publications You can also search for this author inPubMed Google Scholar CONTRIBUTIONS S.X., S.H., Y.C., D.S. and H.T. conceived and
designed research; S.X., Y.T., Y.H., N.Z. and Z.W. performed experiments; S.X., Y.T., Y.H., H.T. and Y.W. analyzed the data; S.X., Y.T., H.T. and Y.W. wrote the paper. All authors reviewed
the manuscript. ETHICS DECLARATIONS COMPETING INTERESTS The authors declare no competing financial interests. ELECTRONIC SUPPLEMENTARY MATERIAL SUPPLEMENTARY INFORMATION RIGHTS AND
PERMISSIONS This work is licensed under a Creative Commons Attribution 4.0 International License. The images or other third party material in this article are included in the article’s
Creative Commons license, unless indicated otherwise in the credit line; if the material is not included under the Creative Commons license, users will need to obtain permission from the
license holder to reproduce the material. To view a copy of this license, visit http://creativecommons.org/licenses/by/4.0/ Reprints and permissions ABOUT THIS ARTICLE CITE THIS ARTICLE Xu,
S., Tian, Y., Hu, Y. _et al._ Tumor growth affects the metabonomic phenotypes of multiple mouse non-involved organs in an A549 lung cancer xenograft model. _Sci Rep_ 6, 28057 (2016).
https://doi.org/10.1038/srep28057 Download citation * Received: 05 March 2016 * Accepted: 31 May 2016 * Published: 22 June 2016 * DOI: https://doi.org/10.1038/srep28057 SHARE THIS ARTICLE
Anyone you share the following link with will be able to read this content: Get shareable link Sorry, a shareable link is not currently available for this article. Copy to clipboard Provided
by the Springer Nature SharedIt content-sharing initiative