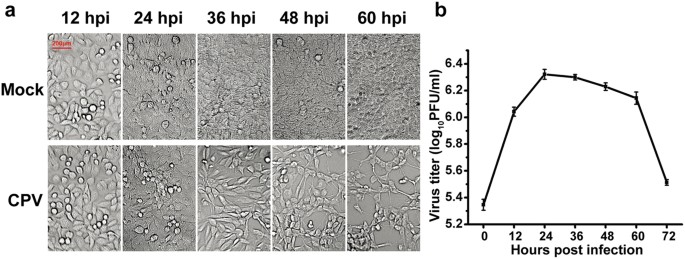
Profiling of host cell response to successive canine parvovirus infection based on kinetic proteomic change identification
- Select a language for the TTS:
- UK English Female
- UK English Male
- US English Female
- US English Male
- Australian Female
- Australian Male
- Language selected: (auto detect) - EN
Play all audios:

ABSTRACT Canine parvovirus (CPV) reproduces by co-opting the resources of host cells, inevitably causing cytotoxic effects to the host cells. Feline kidney F81 cells are sensitive to CPV
infection and show disparate growing statuses at different time points post-infection. This study analysed the response of F81 cells to CPV infection at successive infection time points by
iTRAQ-based quantitative proteomics. Differentially expressed proteins (DEPs) during 60 h of infection and at selected time points post-infection were identified by an analysis of variance
test and a two-tailed unpaired _t_ test, respectively. DEPs with similar quantitative changes were clustered by hierarchical clustering and analysed by gene ontology enrichment, revealing
that 12 h and 60 h post-infection were the optimal times to analyse the autonomous parvovirus replication and apoptosis processes, respectively. Using the MetacoreTM database, 29 DEPs were
enriched in a network involved in p53 regulation. Besides, a significantly enriched pathway suggests that the CPV-induced cytopathic effect was probably due to the deficiency of functional
CFTR caused by CPV infection. This study uncovered the systemic changes in key cellular factors involved in CPV infection and help to understand the molecular mechanisms of the anti-cancer
activity of CPV and the cytopathic effects induced by CPV infection. SIMILAR CONTENT BEING VIEWED BY OTHERS DYNAMIC REMODELLING OF THE HUMAN HOST CELL PROTEOME AND PHOSPHOPROTEOME UPON
ENTEROVIRUS INFECTION Article Open access 28 August 2020 MULTI-OMICS CHARACTERIZATION OF THE MONKEYPOX VIRUS INFECTION Article Open access 08 August 2024 TEMPORAL TRANSCRIPTIONAL PROFILING
OF HOST CELLS INFECTED BY A VETERINARY ALPHAHERPESVIRUS USING NANOPORE SEQUENCING Article Open access 25 January 2025 INTRODUCTION Canine parvovirus (CPV) is a member of autonomous
parvoviruses in the _Parvoviridae_ family. It appears as an icosahedral capsid that encloses a single-strand DNA genome that is 5.2 kb long. CPV replicates without the use of a helper virus
and hence is called autonomous, but it utilises the machinery and components of host cells, including DNA polymerase and RNA polymerase ΙΙ for DNA replication and RNA transcription,
respectively. Consequently, CPV replication is restricted to the S phase of the cell cycle1,2. The CPV genome contains two open reading frames; by alternative splicing, one produces two
mRNAs encoding two non-structural proteins (NS1 and NS2) and the other transcribes two mRNAs encoding two structural proteins (VP1 and VP2)3. The VP1 and VP2 proteins contain the most
important antigenic epitopes, which are targeted by neutralising antibodies. VP2, which represents 90% of the viral capsid, also functions as a viral ligand that determines the viral host
specificity and tissue affinity4. NS1, a pleiotropic phosphoprotein, is thought to be a culprit of apoptosis of CPV-infected cells. For instance, NS1 of CPV-2 has been shown to induce
caspase-dependent and p53-independent apoptosis5. CPV quickly spread worldwide within months of identification and now threatens various species hosts6. CPV infection causes high fatality in
neonatal animals and severe haemorrhagic enteritis in adult dogs7. It is introduced by faecal-oral transmission and it initially infects and replicates in the rapidly dividing cells of
lymphoid tissues, intestinal crypt epithelial cells and precursor cells in the bone marrow. Severe haemorrhagic enteritis increases the risk for viral translocation and coliform septicaemia,
leading to septic shock and ultimately death8. CPV infects cells by binding to the transferrin receptor9 and CPV pathogenicity is thought to be caused by the non-structural proteins of
parvoviruses10. Although infection and replication of parvoviruses kill host cells, the extent of cell death strongly depends on the type of the host cell. For instance, neoplastic cells are
preferentially killed over normal cells11,12. Due to this characteristic, a rodent parvovirus has been used in a phase Ι/ΙΙa clinical trial to prevent tumour recurrence in patients with
glioblastoma multiforma5. Currently, the clinical application of several chemotherapeutic agents is limited because of their severe toxic effects to healthy cells. Anti-cancer therapy using
a virus that can selectively target cancer cells has become a popular approach. CPV is a virus that can be potentially used to treat cancer13. A previous study has shown that CPV2 NS1 can
lead to regression of skin tumours in Wistar rats without producing toxic side effects on healthy cells14. Moreover, the CPV NS1 protein shows anti-tumour activity in a mouse mammary tumour
model and it further stimulates the immune cells to attack the tumour15. Proteomic based approaches have been widely used to develop extensive cellular protein databases that focus on
infection by viruses16,17. However, no such study has been conducted to understand the molecular mechanisms involved in host response to CPV infection. Isobaric tag for relative and absolute
quantitation (iTRAQ) is an _in vitro_ labelling method that has the capability to compare several time points during a single experiment18. The method is considered more sensitive than
difference gel electrophoresis and it has been demonstrated to be effective and accurate in characterising numerous diseases19,20. In this study, we utilised an iTRAQ-labelled mass
spectrometry approach to explore the proteomic changes of F81 cells under different cellular growth behaviour induced by CPV infection. Our findings will help to reveal the biological
processes of different infection stages and systematically understand the host response under CPV infection. RESULTS GENERAL EXPERIMENTAL DESIGN AND OVERVIEW OF THE QUANTITATIVE PROTEOMIC
PROFILE OF F81 CELLS The F81 cells were selected because they efficiently support CPV replication and show specific cytopathic effects (CPEs)21,22,23,24. The cells were infected at a
multiplicity of infection (MOI) of 1 and analysed between 0 and 60 h post-infection (hpi). As shown in Fig. 1a, the mock-infected cells maintained growth without obvious CPEs. In contrast,
CPEs in the infection groups became noticeable at 36 hpi and progressed thereafter and more than half of the cells had become detached at 60 hpi. As shown in Fig. 1b, the viral titre reached
a plateau between 24 hpi and 60 hpi and then rapidly declined. Using the dynamic changes in CPEs and viral titre as a guide, time points between 12 hpi and 60 hpi were selected for
comprehensive proteomic analysis during which the viral titre remained high while the CPEs progressed in the infected cells. Based on a combination of three biological replicates from two
mocks and five infected samples, the iTRAQ analysis identified a total of 3,224 proteins. Proteins were designated as differentially expressed proteins (DEPs) if they had expression
fold-change ratios ≥1.2 or ≤0.833 and the _p_-value was less than 0.05. By two statistical methods, we identified 615 DEPs during the entire infection process by method 1 and 437 DEPs for
all five time points by method 2 (Fig. 2c). As shown in Fig. 2a1,a2, a total of 679 DEPs were identified by combining the two selection methods, which was greater than the number identified
by each of the individual methods. Among the 679 DEPs, 373 proteins were identified by both methods. Among the 437 DEPs identified by method 2, the expression of 85, 102, 95, 209 and 225
proteins was significantly changed at 12, 24, 36, 48 and 60 hpi, respectively (Fig. 2b). As shown in Fig. 2b, only 3 of 437 proteins were identified at all five time points. Moreover, 22,
28, 27, 82 and 86 DEPs were separately identified at the each of the five time points, respectively. These proteins were the key proteins used to study the biological processes at certain
time points. A summary of the 615 quantitatively DEPs obtained by method 1 are shown in Supplementary Table S1. The quantitatively DEPs selected at each time point by method 2 are listed in
Supplementary Tables S2.1–2.5, respectively. The abbreviations of all 679 differentially expressed proteins are listed in Supplementary Table S3; these abbreviations appear in the figures
and their corresponding descriptions. BIOLOGICAL PROCESSES OF DEPS AT 12, 24, 36, 48 AND 60 HPI WERE ENRICHED BY DAVID SOFTWARE To analyse the potential functions of the significantly DEPs
at each time point, we then performed a gene ontology enrichment of biological processes using DAVID software for the proteins obtained by method 2. Generally, gene ontology terms are
enriched by David software without fuzzy heuristic clustering. So, an overwhelming number of terms were generated, which were too difficult to analyse. In order to reduce the redundant
terms, we re-analysed them by “Functional Annotation Clustering,” and only the term with the minimum _p_-value in each cluster was reserved. As a result, 60/79, 57/77, 22/33, 119/166 and
91/135 of the biological process terms at each time point were removed. As shown in Fig. 3a–e, the retained functions of these proteins at each time point are depicted. Enriched functions of
the DEPs at 12 hpi were mainly related to the cell cycle, including regulation of the mitotic phase of the cell cycle. At 24 hpi, numerous proteins involved in RNA processing were enriched.
By 36 hpi, the DEPs were significantly enriched in proteins related to chromatin assembly (_p_ = 1.88E-14). At 48 hpi, enriched functions were related to cellular macromolecular complex
assembly (_p_ = 3.91E-08) and regulation of organelle organisation (_p_ = 2.99E-05). The functional terms in the regulation of apoptosis were enriched at each time point; but at 60 hpi,
proteins involved in the regulation of apoptosis became the largest component (8.51%) of all DEPs. PROTEINS INVOLVED IN THE STIMULUS-RESPONSE AND REGULATION OF APOPTOSIS WERE ENRICHED BY
GENE CLUSTER 3.0 AND DAVID SOFTWARE As shown in Fig. 4a, hierarchical clustering of the _Felis catus_ proteins was generated using the DEPs listed in Supplementary Table S1. The analysis
revealed that all replicates clustered tightly. Interestingly, the DAVID software analysis showed that most proteins in a given cluster were enriched for functions involved in similar
biological processes. As shown in Fig. 4a, most clusters were enriched in the biological processes of energy metabolism, the cell cycle, translation and RNA-related functions. Both cluster A
(Fig. 4b) and cluster B (Fig. 4c) are highlighted. Sixty-nine proteins in cluster A were up-regulated during the whole infection period, especially at 48 hpi and 60 hpi. The DAVID analysis
indicated that the proteins in cluster A were mainly associated with cellular apoptosis, including HMOX1, TOP2A, HSPA9, HSPD1, F2, HBB, SLC25A24, BAK1, VDAC2, PHB, APOH and VIMP. Moreover,
five of these proteins were classified as negative regulators of apoptosis. Furthermore, in cluster B consisting of 35 proteins, 10 are known to be stimulus-responsive, 7 of which are
additionally known to respond to viral infections. For instance, both RAB7A and CD63 are required for the HIV-1 replication cycle25,26. In addition, LSD1, HSPA5 and CAV-1 have been reported
to influence the efficiency of viral infection and replication27,28,29,30,31; NPC1 is the key influencer for Ebola virus to enter cells32; SEC22b can inhibit the release of infectious viral
progeny33; and the down-regulation of CD59 is associated with the development of hepatitis B cytotoxicity34. A NETWORK FOCUSING ON P53 REGULATION AND A PATHWAY INVOLVING CYSTIC FIBROSIS
TRANSMEMBRANE CONDUCTANCE REGULATOR (CFTR) DEGRADATION WERE ENRICHED ACCORDING TO THE METACORETM DATABASE Using MetacoreTM software, 29 DEPs associated with p53 were significantly enriched
(_p_-value = 1.130E-49), including BAK1, VDAC2, HSP60 and Ebp1 (Fig. 5), indicating that CPV infection may trigger biological processes of p53 regulation. The pathways were enriched and
ranked according to their _p_-values by the MetacoreTM database (Supplementary Table S4). These pathways are involved in processes of cell cycle regulation, cytoskeleton remodelling, cell
apoptosis and survival, oxidative phosphorylation and immune response. Notably, eight DEPs were enriched in the pathway involved in the “CFTR folding and maturation” with a _p_-value of
9.398E-06 (Supplementary Fig. S1). The detailed analysis depicted in Fig. 6 indicates that eight DEPs were directly or indirectly associated with the CFTR (Derlin 1, AMFR, HSP70, HSP90,
Hdi-2, UBE1, BAG-2 and SAE1). Among them, Derlin 1 and AMFR were up-regulated during infection, which can directly degrade the abnormal CFTR35,36. ITRAQ-MS DATA WERE VERIFIED BY WESTERN
BLOTTING The verification of DEPs by western blotting was limited by the availability of antibodies to _Felis catus_ proteins. Initial tests selected a set of proteins that were
significantly changed during infection and also reliably cross-reacted with antibodies to the corresponding human protein. As shown in Fig. 7, the western blots confirmed the differential
expression of ribosomal protein S4X (RPS4X), 60S ribosomal protein L7 (RPL7), guanine nucleotide-binding protein-like 3-like protein (GNL3L), laminin subunit gamma-1 (LAMC1), histone H3.1
(H3.1), 60S ribosomal protein L17 (RPL17) and 60S ribosomal protein L10 (RPL10 or QM) detected by iTRAQ. DISCUSSION In this study, we used proteomics to investigate the global profiles of
differentially expressed cellular proteins under CPV infection. The features of our experimental design and data analysis are as follows: 1. The kinetic changes in the expression levels of
cellular proteins along the infection course, consisting of five different time points, were quantified, which represented an improvement over a cross-sectional picture if only a sample from
a single time point was analysed; these results provided us a kinetic view of the changed expression of cellular proteins under the cytopathic insult of CPV infection. 2. Two mocks were
analysed. These two mocks helped to distinguish the natural kinetics of the cellular protein expression and helped to set reasonable standards to screen the DEPs. 3. Three biological
replicates were prepared for each sample to assess inter-experimental variations. 4. The DEPs identified over the whole infection process were determined to be significant by an analysis of
variance test, while those at each of the five time points were determined to be significant by the two-tailed unpaired _t_ test. As expected, a large amount of proteomic data was generated
in this study. Venn diagramming functional annotation, hierarchical clustering and pathway enrichment were utilised to analyse the results. A common analysis strategy in proteomics is to
look for a period in which the changes in the protein expression were the most prominent. However, such a strategy is dissociated from the status of viral infection and the impact on the
infected cells. A different strategy in this study was employed that combined the detected significant changes in DEPs with viral replication and CPEs. In view of the CPEs and the one-step
growth curve shown in Fig. 1b, the viral titre was increased quickly during the first 12 hpi. In addition, the DEPs involved in the mitotic cell cycle were significantly enriched by up to
6.74% of the total DEPs at 12 hpi (Fig. 3). Since the cells had efficient proliferation at 12 hpi, this time point was suitable for studying the cellular proteins involved in autonomous
parvovirus replication. As shown in Fig. 4c, the proteins involved in the stimulus-response were enriched and most of them are reported to respond to viral infection. These proteins can be
potential targets for controlling viral infection. Dynamic changes in the expressed proteins in cluster B were noted. They were initially down-regulated at 12 and 24 hpi, followed by a rapid
return to near basal levels at 36 hpi and slight up-regulation at 48 and 60 hpi. Thus, 12 and 24 hpi were the best time points to study these proteins (Fig. 4). Similarly, 60 hpi was the
optimal time point to analyse the DEPs involved in the regulation of apoptosis because apoptosis-related proteins accounted for the largest percentage at this time point (Fig. 3e) and the
CPEs were the most serious at 60 hpi (Fig. 1b). As shown in Fig. 4b, many proteins related to cell apoptosis were enriched in cluster A and they were up-regulated during the entire infection
period, but most strikingly at 48 and 60 hpi. Thus, we concluded that the late stage of CPV infection was a better time to investigate the CPV-induced F81 cell apoptosis process. As
apoptosis may be the main mechanism utilised by viral oncolytic therapy, these identified DEPs may be useful for detailed studies in the future. The anti-cancer activity of CPV is closely
related to its apoptosis-inducing effect. A previous study has shown that CPV2 NS1 induces apoptosis mainly through the mitochondrial pathway37. Coincidently, we found that BAK1 was
up-regulated at 48 hpi and 60 hpi in this study. BAK is a pro-apoptotic regulator that plays a central role in mitochondrial membrane permeabilisation38. It suppresses tumourigenesis by
mediating apoptosis of tumour cells39. For example, BAK over-expression can effectively induce apoptosis in gastric cancer cells40. Also, elevations in BAK protein levels have been shown to
accelerate apoptosis in murine lymphoid, lung cancer and breast cancer cells41. In addition, VDAC2, which can recruit newly synthesised BAK to the mitochondria to carry out apoptosis42, was
also up-regulated at 48 hpi and 60 hpi in our study. These findings remind us that the anti-cancer activity of CPV may be closely related to the over-expression of BAK1 and the mitochondrial
apoptotic pathway that is mediated by it. Lakshman Santra _et al_. suggest that CPV2 NS1 might be effective against tumours with the p53 mutation43. As a critical effector of apoptosis, BAK
can participate in the apoptotic process without activation of p53. Therefore, over-expression of the _bak_ gene may be a new strategy for the treatment of tumours, especially for tumours
bearing p53 mutations. What’s more, we enriched a panel of 29 proteins that are associated with p53 by MetacoreTM software, as shown in Fig. 5. As p53 is known as an important anti-cancer
protein, these proteins could be related to cancer development. For instance, API5 was enriched in this network and the API5 expression level was reduced in F81 cells at 48 hpi and 60 hpi.
API5 is an apoptosis inhibitory protein that can suppress E2F-dependent apoptosis44. It is frequently up-regulated in tumour cells and functions as a master regulator of immune escape genes
in tumours45. Based on these findings, CPV may be a powerful apoptosis-modulating agent that could down-regulate API5 gene expression. We found that the HSP60 expression level was increased
at 60 hpi. Heat shock proteins (HSPs) are a group of proteins that respond to stress, including heat, hypoxia, irradiation, infection, or toxic agents46. HSP has been shown to influence
apoptosis in tumour cells, regulate p53 function and induce an immune response to tumours47. Increased HSP levels make cells more resistant to apoptosis, but they also can increase immunity
to cancer48. For example, over-expressed HSP60 protein is suggested to promote prostate cancer, colorectal cancer and primary breast cancer; for these cancers, HSP60 inhibitors may be used
as anti-cancer agents49,50,51. Whereas, a loss of HSP60 immunopositivity is related to the development and progression of bronchial cancer in smokers with chronic obstructive pulmonary
disease52. In this experiment, we also identified that the HSP70 expression level was increased at 60 hpi and the HSP90A expression level was decreased at 48 hpi and 60 hpi. As the influence
of the HSP expression changes due to different cancers, the anti-cancer significance of the differential expression of HSPs in CPV-infected cells is complicated and requires further study.
Previous studies have shown that the CPV NS1 protein exhibits anti-tumour activity in mammary tumours and skin tumours13,15, but the complete mechanisms of its anti-cancer activity have not
been revealed. In this study, we first identified a group of cancer-related proteins by a proteomics technique. Some of these proteins were not noted for CPV-induced apoptosis or anti-cancer
function, which would provide a new perspective for studying the anti-cancer mechanisms of CPV. Using MetacoreTM software, we were able to suggest the main pathways related to CPV
infection. Interestingly, a pathway that involved the degradation of CFTR was significantly enriched in our study. CFTR is a transmembrane protein that functions as an ATP-gated chloride
channel53. Deficiency in CFTR can lead to several diseases related to the reduction of chloride secretion and the increase of sodium uptake54. Many studies have suggested that wound healing
function is impaired in CFTR-deficient epithelial cells and that CFTR may be effective in cell repair after injury18,55,56. CPV is normally cytopathic in infected F81 cells. Typical CPEs
appear as a mesh of monolayer cells prompted by detached cells. These results provided us hints that CPV may trigger CFTR deficiency and influence the cell pathological change by reducing
the wound healing of infected cells. In summary, DEPs and their expression kinetics in CPV-infected F81 cells were characterised with iTRAQ analysis. There were dynamic changes in DEPs over
the course of infection. DEPs that are involved in viral defence or apoptosis and are related to cancer may be useful for studying anti-viral and oncolytic therapy. Finally, the identified
DEPs that are involved in folding and maturation of CFTR provide us with a list of candidates for further understanding the pathogenesis of CPV-infected host cells. EXPERIMENTAL PROCEDURES
GENERAL EXPERIMENTAL DESIGN CPV-HLJ-6 (GenBank accession no. KR611475.1) was passaged ten times in F81 cells, resulting in a final stock containing viruses at 105.5 TCID50/mL. For infection,
106 F81 cells were plated in 25 cm2 culture flasks and, at the same time, inoculated by CPV at a MOI of 1. Infected cells were collected at 12, 24, 36, 48 and 60 hpi and uninfected
mock-treated cells were collected at 0 and 48 h after plating. All experiments were repeated in three biological replicates with cells at different passage numbers. PROTEIN EXTRACTION AND
QUANTIFICATION The collected cells were lysed by Protein Extraction Reagent (Thermo Scientific™), containing a protease inhibitor cocktail, for 10 min under gentle shaking conditions. The
samples were centrifuged at 15,000 _g_ for 10 min and the supernatants were collected. The protein concentrations were measured by the Bradford protein assay. ITRAQ LABELLING AND PEPTIDE
FRACTIONATION The iTRAQ labelling was conducted according to the protocol of the iTRAQ reagents (8 plex, Applied Biosystems). Briefly, the protein samples were thermally denatured, reduced
and alkylated prior to trypsin (Promega) digestion for 15 h. The digested samples were labelled with each component of the iTRAQ reagent kit: two mock samples were labelled with 113 and 114;
and five CPV-infected samples collected at 12, 24, 36, 48 and 60 hpi were labelled with 115, 116, 117, 118 and 119, respectively. Three biological replicates were prepared for all samples.
Next, an equal ratio of each set was pooled for analysis. Additionally, to reduce the complexity of the peptide mixtures, reverse-phase chromatography using a RIGOL L-3000 system was applied
for separating the peptides. The labeled peptide mixtures were dissolved in 100 μL of mobile phase A [2% acetonitrile in ddH2O, pH 10] and centrifuged at 14,000 _g_ for 20 min prior to
loading onto the column. Samples were eluted by injection of stepwise gradients of mobile phase B [2% ddH2O in acetonitrile, pH 10], with a flow rate set at 700 μL/min. Each fraction was
eluted for 1.5 min. ANALYSIS BY Q-EXACTIVE MASS SPECTROMETRY The fractionated peptide mixtures were analysed by a Q-Exactive mass spectrometer equipped with an EASY-nLC 1000 System (Thermo
Fisher Scientific). The spray voltage, capillary temperature and declustering potential of the source ionisation parameters were set as 2.1 kV, 250 °C and 100 V, respectively. A Top 20
data-dependent acquisition mode was selected in the mass spectrometer, with automatic switching between MS and MS/MS. Full-scan MS mode (350−1800 _m/z_) was operated at a resolution of
70,000, with an automatic gain control target of 1 × 106 ions and a maximum ion transfer of 60 ms. The precursor ions were fragmented by high-energy collisional dissociation and subjected to
MS/MS scans with the following parameters: resolution, 17,500; automatic gain control, 5 × 106 ions; maximum ion transfer, 70 ms; intensity threshold, 5,000; and normalised collision
energy, 29%. DATABASE SEARCHING AND PROTEIN QUANTITATION All raw data files generated by mass spectrometry were analysed by Proteome Discoverer software 1.3 (Thermo Fisher Scientific) using
the Mascot search engine against the _Felis catus_ protein sequences downloaded from the NCBI reference sequences (Refseq) database (downloaded on March 20, 2015, containing 35,247 entries).
The parameters for database searching were as follows: trypsin was selected as the enzyme; up to two missed cleavages were allowed; the precursor and fragment ion mass tolerance were set to
15 ppm and 20 mmu, respectively; carbamidomethylation on cysteine was set as a fixed modification; oxidation on methionine and iTRAQ 8 plex labels at the N-termini and at lysine side chains
were allowed as dynamic modifications. The strict maximum parsimony principle was applied and only peptide spectrum matches with high or medium confidence were considered for protein
grouping. Ion peaks were integrated based on the most confident centroid with a tolerance of 20 ppm. The quantification was also performed by Proteome Discoverer software 1.3, which can
automatically calculate the relative abundance of iTRAQ-labeled peptides as well as the corresponding proteins. Proteins identified with two or more unique peptides were considered as a
highly confident identification and used for quantification. Also, to ensure the accuracy of quantification, only the proteins whose coefficient of variation of three biological repeats were
less than 20% were identified as DEPs. The protein ratios were calculated from channels (114/113 to 119/113) for all proteins identified in each iTRAQ experiment. Two identical mock
samples, with iTRAQ labels of 113 and 114, were used as references. The fold threshold for changed protein fold-change ratios was set at ±1.20, which covers a 95% quantification area based
on the normal distribution of two mock samples (113 versus 114) in all biological replicates57. Thus, the proteins with fold-change ratios ≥1.20 or ≤0.83 were selected as DEPs. To identify
the DEPs more comprehensively, we used two methods: An analysis of variance test that included a _p_-value < 0.05 and at least one of the fold-change ratios between any time points
passing the threshold was used to identify the DEPs of all groups (Method 1). Furthermore, to clarify the protein alterations of each time points, a two-tailed unpaired _t_ test that
included a _p_-value < 0.05 and a fold-change ratio between a given time point and the mock (114) passing the threshold was used for screening the DEPs at the different time points
(Method 2). BIOINFORMATICS DATA ANALYSIS The majority of the proteins in the _Felis catus_ database are not annotated with biological functions. Nevertheless, because most of the identified
proteins shared high homologies to _Homo sapien_ proteins, we blasted DEPs defined by iTRAQ analysis with the _H. sapien_ protein database in Swissprot. Only the proteins with at least 70%
identity were considered to be matched. The DEPs were submitted to the online software DAVID (http://david.ncifcrf.gov) for enrichment analysis of the gene ontology category “Biological
Process”. Redundant items were reduced by “Functional Annotation Clustering” with medium classification stringency. The terms with the minimum _p_-values in every cluster were then selected
out and reserved. The patterns of differential protein expression were displayed by hierarchical clustering according to the fold-change ratios. The protein relationships were calculated by
average linkage with uncentered correlation using Gene Cluster 3.0. The network analysis of the DEPs was performed by the “Analyze Network Algorithm” provided by MetacoreTM software. The
pathway analysis of the DEPs was performed using the GeneGo pathway maps in the MetacoreTM database (version 6.24; build 67895, Thomson Reuters). In addition, the Pathway Maps tool was used
for pathway enrichment evaluation, in which the _p_-values were calculated based on the hypergeometric distribution with the default background database. WESTERN BLOT ANALYSIS Cells were
harvested and cellular proteins were extracted as mentioned above. Total cellular proteins from different time points were separated by SDS-PAGE and electrotransferred to nitrocellulose
membranes (Whatman). The membranes were blocked with 5% non-fat milk powder dissolved in tris-buffered saline, containing 0.05% Tween-20, for 2 h at room temperature. Primary antibodies (see
below) were diluted 1:500 in blocking buffer and incubated with the membranes overnight at 4 °C. Next, the membranes were incubated with the appropriate secondary antibodies diluted in
blocking buffer at 1:3000 for 2 h at room temperature. Detected proteins were revealed using the ECL Detection Kit (KeyGen Biotech). The following rabbit antibodies were used: Ribosomal
Protein S4X Polyclonal Antibody (YT4135), Ribosomal Protein L17 Polyclonal Antibody (YT4098), QM Polyclonal Antibody (YT3916), Histone H3.1 Polyclonal Antibody (YT2163), Laminin γ-1
Polyclonal Antibody (YT2531), GNL3L Polyclonal Antibody (YT1938), Ribosomal Protein L7 Polyclonal Antibody (YT4118) and β-Actin Polyclonal Antibody YT0099). All the rabbit antibodies were
purchased from ImmunoWay Biotechnology Company. The Peroxidase AffiniPure goat Anti-Rabbit IgG was used as the secondary antibody (Jackson ImmunoResearch Lab.). ADDITIONAL INFORMATION HOW TO
CITE THIS ARTICLE: Zhao, H. _et al_. Profiling of Host Cell Response to Successive Canine Parvovirus Infection Based on Kinetic Proteomic Change Identification. _Sci. Rep._ 6, 29560; doi:
10.1038/srep29560 (2016). REFERENCES * Tattersall, P. & Ward, D. C. Rolling hairpin model for replication of parvovirus and linear chromosomal DNA. Nature 263, 106–109 (1976). Article
CAS ADS Google Scholar * Parrish, C. R. & Kawaoka, Y. The origins of new pandemic viruses: the acquisition of new host ranges by canine parvovirus and influenza A viruses. Annu Rev
Microbiol 59, 553–586, doi: 10.1146/annurev.micro.59.030804.121059 (2005). Article CAS PubMed Google Scholar * Shackelton, L. A., Parrish, C. R., Truyen, U. & Holmes, E. C. High rate
of viral evolution associated with the emergence of carnivore parvovirus. Proc Natl Acad Sci USA 102, 379–384, doi: 10.1073/pnas.0406765102 (2005). Article CAS ADS PubMed Google Scholar
* Organtini, L. J., Allison, A. B., Lukk, T., Parrish, C. R. & Hafenstein, S. Global displacement of canine parvovirus by a host-adapted variant: structural comparison between pandemic
viruses with distinct host ranges. J Virol 89, 1909–1912, doi: 10.1128/JVI.02611-14 (2015). Article CAS PubMed Google Scholar * Nykky, J., Tuusa, J. E., Kirjavainen, S., Vuento, M.
& Gilbert, L. Mechanisms of cell death in canine parvovirus-infected cells provide intuitive insights to developing nanotools for medicine. Int J Nanomedicine 5, 417–428 (2010). CAS
PubMed PubMed Central Google Scholar * Allison, A. B. et al. Host-specific parvovirus evolution in nature is recapitulated by _in vitro_ adaptation to different carnivore species. PLos
Pathog 10, e1004475, doi: 10.1371/journal.ppat.1004475 (2014). Article CAS PubMed PubMed Central Google Scholar * Zhao, H. et al. Typing of Canine Parvovirus Strains Circulating in
North-East China. Transbound Emerg Dis, doi: 10.1111/tbed.12390 (2015). * Zourkas, E., Ward, M. P. & Kelman, M. Canine parvovirus in Australia: A comparative study of reported rural and
urban cases. Vet Microbiol, doi: 10.1016/j.vetmic.2015.10.009 (2015). * Kaelber, J. T. et al. Evolutionary reconstructions of the transferrin receptor of Caniforms supports canine parvovirus
being a re-emerged and not a novel pathogen in dogs. PLos Pathog 8, e1002666, doi: 10.1371/journal.ppat.1002666 (2012). Article CAS PubMed PubMed Central Google Scholar * Gupta, S. K.
et al. Canine parvovirus NS1 induced apoptosis involves mitochondria, accumulation of reactive oxygen species and activation of caspases. Virus Res 213, 46–61, doi:
10.1016/j.virusres.2015.10.019 (2015). Article CAS PubMed Google Scholar * Caillet-Fauquet, P., Perros, M., Brandenburger, A., Spegelaere, P. & Rommelaere, J. Programmed killing of
human cells by means of an inducible clone of parvoviral genes encoding non-structural proteins. EMBO J 9, 2989–2995 (1990). Article CAS Google Scholar * Mousset, S., Ouadrhiri, Y.,
Caillet-Fauquet, P. & Rommelaere, J. The cytotoxicity of the autonomous parvovirus minute virus of mice nonstructural proteins in FR3T3 rat cells depends on oncogene expression. J Virol
68, 6446–6453 (1994). CAS PubMed PubMed Central Google Scholar * Santra, L. et al. Non-Structural protein 1 (NS1) gene of Canine Parvovirus-2 regresses chemically induced skin tumors in
Wistar rats. Res Vet Sci 97, 292–296, doi: 10.1016/j.rvsc.2014.07.024 (2014). Article CAS PubMed Google Scholar * Geletneky, K. et al. Phase I/IIa study of intratumoral/intracerebral or
intravenous/intracerebral administration of Parvovirus H-1 (ParvOryx) in patients with progressive primary or recurrent glioblastoma multiforme: ParvOryx01 protocol. BMC Cancer 12, 99, doi:
10.1186/1471-2407-12-99 (2012). Article PubMed PubMed Central Google Scholar * Gupta, S. K. et al. Canine parvovirus NS1 protein exhibits anti-tumor activity in a mouse mammary tumor
model. Virus Res 213, 289–298, doi: 10.1016/j.virusres.2015.12.017 (2016). Article CAS PubMed Google Scholar * Yoo, Y. H., Yun, J., Yoon, C. N. & Lee, J. S. Chemical proteomic
identification of T-plastin as a novel host cell response factor in HCV infection. Sci Rep 5, 9773, doi: 10.1038/srep09773 (2015). Article CAS PubMed PubMed Central Google Scholar *
Kipper, S. et al. New host factors important for respiratory syncytial virus (RSV) replication revealed by a novel microfluidics screen for interactors of matrix (M) protein. Mol Cell
Proteomics 14, 532–543, doi: 10.1074/mcp.M114.044107 (2015). Article CAS PubMed PubMed Central Google Scholar * Jadaliha, M. et al. Quantitative proteomic analysis of human embryonic
stem cell differentiation by 8-plex iTRAQ labelling. PLos One 7, e38532, doi: 10.1371/journal.pone.0038532 (2012). Article CAS ADS PubMed PubMed Central Google Scholar * Lu, Q. et al.
Two-dimensional liquid chromatography-tandem mass spectrometry coupled with isobaric tags for relative and absolute quantification (iTRAQ) labeling approach revealed first proteome profiles
of pulmonary alveolar macrophages infected with porcine reproductive and respiratory syndrome virus. J Proteome Res 11, 2890–2903, doi: 10.1021/pr201266z (2012). Article CAS ADS PubMed
Google Scholar * Zhang, J., Niu, D., Sui, J., Ching, C. B. & Chen, W. N. Protein profile in hepatitis B virus replicating rat primary hepatocytes and HepG2 cells by iTRAQ-coupled 2-D
LC-MS/MS analysis: Insights on liver angiogenesis. Proteomics 9, 2836–2845, doi: 10.1002/pmic.200800911 (2009). Article CAS PubMed Google Scholar * Wen, J. et al. Soluble form of canine
transferrin receptor inhibits canine parvovirus infection _in vitro_ and _in vivo_. Biomed Res Int 2013, 172479, doi: 10.1155/2013/172479 (2013). Article CAS PubMed PubMed Central Google
Scholar * Yuan, D. et al. Establishment of a rescue system for an autonomous Parvovirus mink enteritis virus. Virus Res 183, 1–5, doi: 10.1016/j.virusres.2014.01.012 (2014). Article CAS
PubMed Google Scholar * Sun, J. Z. et al. MicroRNA profile analysis of a feline kidney cell line before and after infection with mink enteritis virus. Gene 539, 224–229, doi:
10.1016/j.gene.2014.01.074 (2014). Article CAS PubMed Google Scholar * Sun, J. Z. et al. MicroRNA miR-320a and miR-140 inhibit mink enteritis virus infection by repression of its
receptor, feline transferrin receptor. Virol J 11, 210, doi: 10.1186/s12985-014-0210-3 (2014). Article CAS PubMed PubMed Central Google Scholar * Li, G. et al. The dual role of
tetraspanin CD63 in HIV-1 replication. Virol J 11, 23, doi: 10.1186/1743-422X-11-23 (2014). Article CAS PubMed PubMed Central Google Scholar * Caillet, M. et al. Rab7A is required for
efficient production of infectious HIV-1. PLos Pathog 7, e1002347, doi: 10.1371/journal.ppat.1002347 (2011). Article CAS PubMed PubMed Central Google Scholar * Hill, J. M. et al.
Inhibition of LSD1 reduces herpesvirus infection, shedding and recurrence by promoting epigenetic suppression of viral genomes. Sci Transl Med 6, 265ra169, doi: 10.1126/scitranslmed.3010643
(2014). Article CAS PubMed PubMed Central Google Scholar * Liang, Y., Vogel, J. L., Narayanan, A., Peng, H. & Kristie, T. M. Inhibition of the histone demethylase LSD1 blocks
alpha-herpesvirus lytic replication and reactivation from latency. Nat Med 15, 1312–1317, doi: 10.1038/nm.2051 (2009). Article CAS PubMed PubMed Central Google Scholar * Roberts, J. L.
et al. GRP78/Dna K Is a Target for Nexavar/Stivarga/Votrient in the Treatment of Human Malignancies, Viral Infections and Bacterial Diseases. J Cell Physiol 230, 2552–2578, doi:
10.1002/jcp.25014 (2015). Article CAS PubMed PubMed Central Google Scholar * Bohm, K., Sun, L., Thakor, D. & Wirth, M. Caveolin-1 limits human influenza A virus (H1N1) propagation
in mouse embryo-derived fibroblasts. Virology 462-463, 241–253, doi: 10.1016/j.virol.2014.05.028 (2014). Article CAS PubMed Google Scholar * Lo, Y. T., Nadeau, P. E., Lin, S. &
Mergia, A. Establishing Restricted Expression of Caveolin-1 in HIV Infected Cells and Inhibition of Virus Replication. Open Microbiol J 8, 114–121, doi: 10.2174/1874285801408010114 (2014).
Article PubMed PubMed Central Google Scholar * Kuroda, M. et al. Interaction between TIM-1 and NPC1 Is Important for Cellular Entry of Ebola Virus. J Virol 89, 6481–6493, doi:
10.1128/JVI.03156-14 (2015). Article CAS PubMed PubMed Central Google Scholar * Vogels, M. W. et al. Identification of host factors involved in coronavirus replication by quantitative
proteomics analysis. Proteomics 11, 64–80, doi: 10.1002/pmic.201000309 (2011). Article CAS PubMed Google Scholar * Qu, Z. et al. Hepatitis B virus sensitizes hepatocytes to
complement-dependent cytotoxicity through downregulating CD59. Mol Immunol 47, 283–289, doi: 10.1016/j.molimm.2009.09.022 (2009). Article CAS PubMed Google Scholar * Wang, B. et al.
BAP31 interacts with Sec61 translocons and promotes retrotranslocation of CFTRDeltaF508 via the derlin-1 complex. Cell 133, 1080–1092, doi: 10.1016/j.cell.2008.04.042 (2008). Article CAS
PubMed Google Scholar * Ballar, P., Ors, A. U., Yang, H. & Fang, S. Differential regulation of CFTRDeltaF508 degradation by ubiquitin ligases gp78 and Hrd1. Int J Biochem Cell Biol 42,
167–173, doi: 10.1016/j.biocel.2009.10.005 (2010). Article CAS PubMed Google Scholar * Gupta, S. K. et al. Canine parvovirus NS1 induced apoptosis involves mitochondria, accumulation of
reactive oxygen species and activation of caspases. Virus Res 213, 46–61, doi: 10.1016/j.virusres.2015.10.019 (2016). Article CAS PubMed Google Scholar * Cory, S., Huang, D. C. &
Adams, J. M. The Bcl-2 family: roles in cell survival and oncogenesis. Oncogene 22, 8590–8607, doi: 10.1038/sj.onc.1207102 (2003). Article CAS PubMed Google Scholar * Degenhardt, K.,
Chen, G., Lindsten, T. & White, E. BAX and BAK mediate p53-independent suppression of tumorigenesis. Cancer Cell 2, 193–203 (2002). Article CAS Google Scholar * Tong, Q. S., Zheng, L.
D., Wang, L., Liu, J. & Qian, W. BAK overexpression mediates p53-independent apoptosis inducing effects on human gastric cancer cells. BMC Cancer 4, 33, doi: 10.1186/1471-2407-4-33
(2004). Article CAS PubMed PubMed Central Google Scholar * Pataer, A. et al. Adenoviral Bak overexpression mediates caspase-dependent tumor killing. Cancer Res 60, 788–792 (2000). CAS
PubMed Google Scholar * Cartron, P. F., Petit, E., Bellot, G., Oliver, L. & Vallette, F. M. Metaxins 1 and 2, two proteins of the mitochondrial protein sorting and assembly machinery,
are essential for Bak activation during TNF alpha triggered apoptosis. Cell Signal 26, 1928–1934, doi: 10.1016/j.cellsig.2014.04.021 (2014). Article CAS PubMed Google Scholar * Doley, J.
et al. Canine parvovirus type 2a (CPV-2a)-induced apoptosis in MDCK involves both extrinsic and intrinsic pathways. Appl Biochem Biotechnol 172, 497–508, doi: 10.1007/s12010-013-0538-y
(2014). Article CAS PubMed Google Scholar * Morris, E. J. et al. Functional identification of Api5 as a suppressor of E2F-dependent apoptosis _in vivo_. PLos Genet 2, e196, doi:
10.1371/journal.pgen.0020196 (2006). Article CAS PubMed PubMed Central Google Scholar * Noh, K. H. et al. API5 confers tumoral immune escape through FGF2-dependent cell survival
pathway. Cancer Res 74, 3556–3566, doi: 10.1158/0008-5472.CAN-13-3225 (2014). Article CAS PubMed PubMed Central Google Scholar * Lindquist, S. & Craig, E. A. The heat-shock
proteins. Annu Rev Genet 22, 631–677, doi: 10.1146/annurev.ge.22.120188.003215 (1988). Article CAS PubMed Google Scholar * Lebret, T. et al. Heat shock proteins HSP27, HSP60, HSP70 and
HSP90: expression in bladder carcinoma. Cancer 98, 970–977, doi: 10.1002/cncr.11594 (2003). Article CAS PubMed Google Scholar * Ghosh, J. C., Dohi, T., Kang, B. H. & Altieri, D. C.
Hsp60 regulation of tumor cell apoptosis. J Biol Chem 283, 5188–5194, doi: 10.1074/jbc.M705904200 (2008). Article CAS PubMed Google Scholar * Castilla, C. et al. Immunohistochemical
expression of Hsp60 correlates with tumor progression and hormone resistance in prostate cancer. Urology 76, 1017 e1011–1016, doi: 10.1016/j.urology.2010.05.045 (2010). Article Google
Scholar * He, Y. et al. Proteomics-based identification of HSP60 as a tumor-associated antigen in colorectal cancer. Proteomics Clin Appl 1, 336–342, doi: 10.1002/prca.200600718 (2007).
Article CAS PubMed Google Scholar * Desmetz, C. et al. Proteomics-based identification of HSP60 as a tumor-associated antigen in early stage breast cancer and ductal carcinoma _in situ_.
J Proteome Res 7, 3830–3837, doi: 10.1021/pr800130d (2008). Article CAS PubMed Google Scholar * Cappello, F. et al. Hsp60 and Hsp10 down-regulation predicts bronchial epithelial
carcinogenesis in smokers with chronic obstructive pulmonary disease. Cancer 107, 2417–2424, doi: 10.1002/cncr.22265 (2006). Article CAS PubMed Google Scholar * Gadsby, D. C., Vergani,
P. & Csanady, L. The ABC protein turned chloride channel whose failure causes cystic fibrosis. Nature 440, 477–483, doi: 10.1038/nature04712 (2006). Article CAS ADS PubMed PubMed
Central Google Scholar * Liu, X. X. et al. iTRAQ-based quantitative proteomic analysis of the anti-apoptotic effect of hyperin, which is mediated by Mcl-1 and Bid, in H2O2-injured EA.hy926
cells. Int J Mol Med 37, 1083–1090, doi: 10.3892/ijmm.2016.2510 (2016). Article CAS PubMed Google Scholar * Cao, X. et al. iTRAQ-based comparative proteomic analysis of
excretory-secretory proteins of schistosomula and adult worms of Schistosoma japonicum. J Proteomics 138, 30–39, doi: 10.1016/j.jprot.2016.02.015 (2016). Article CAS PubMed Google Scholar
* Li, J. et al. Differential proteomics analysis to identify proteins and pathways associated with male sterility of soybean using iTRAQ-based strategy. J Proteomics 138, 72–82, doi:
10.1016/j.jprot.2016.02.017 (2016). Article CAS PubMed Google Scholar * Unwin, R. D., Griffiths, J. R. & Whetton, A. D. Simultaneous analysis of relative protein expression levels
across multiple samples using iTRAQ isobaric tags with 2D nano LC-MS/MS. Nat Protoc 5, 1574–1582, doi: 10.1038/nprot.2010.123 (2010). Article CAS PubMed Google Scholar Download
references ACKNOWLEDGEMENTS This study was supported by the Guiding Fund of Jilin Provincial Strategic. Adjustment of Economic Structure (No. 2014Y139) and Jilin Provincial Major. Science
and Technology Development Project Fund (No. 20150201006NY). AUTHOR INFORMATION AUTHORS AND AFFILIATIONS * State Key Laboratory for Molecular Biology of Special Economic Animals, Institute
of Special Animal and Plant Sciences, Chinese Academy of Agricultural Sciences, Changchun, 130112, China Hang Zhao, Yuening Cheng, Jianke Wang, Peng Lin, Li Yi, Yaru Sun, Jingqiang Ren,
Mingwei Tong, Zhigang Cao, Jiawei Li, Jinliang Deng & Shipeng Cheng Authors * Hang Zhao View author publications You can also search for this author inPubMed Google Scholar * Yuening
Cheng View author publications You can also search for this author inPubMed Google Scholar * Jianke Wang View author publications You can also search for this author inPubMed Google Scholar
* Peng Lin View author publications You can also search for this author inPubMed Google Scholar * Li Yi View author publications You can also search for this author inPubMed Google Scholar *
Yaru Sun View author publications You can also search for this author inPubMed Google Scholar * Jingqiang Ren View author publications You can also search for this author inPubMed Google
Scholar * Mingwei Tong View author publications You can also search for this author inPubMed Google Scholar * Zhigang Cao View author publications You can also search for this author
inPubMed Google Scholar * Jiawei Li View author publications You can also search for this author inPubMed Google Scholar * Jinliang Deng View author publications You can also search for this
author inPubMed Google Scholar * Shipeng Cheng View author publications You can also search for this author inPubMed Google Scholar CONTRIBUTIONS S.C. and H.Z. designed the study; H.Z.,
Y.C., J.W., P.L. and J.D. performed the experiments; L.Y., Y.S., J.R. and M.T. analysed the data; Z.C. and J.L. prepared the figures and tables; H.Z. wrote the manuscript. ETHICS
DECLARATIONS COMPETING INTERESTS The authors declare no competing financial interests. ELECTRONIC SUPPLEMENTARY MATERIAL SUPPLEMENTARY INFORMATION SUPPLEMENTARY TABLES RIGHTS AND PERMISSIONS
This work is licensed under a Creative Commons Attribution 4.0 International License. The images or other third party material in this article are included in the article’s Creative Commons
license, unless indicated otherwise in the credit line; if the material is not included under the Creative Commons license, users will need to obtain permission from the license holder to
reproduce the material. To view a copy of this license, visit http://creativecommons.org/licenses/by/4.0/ Reprints and permissions ABOUT THIS ARTICLE CITE THIS ARTICLE Zhao, H., Cheng, Y.,
Wang, J. _et al._ Profiling of Host Cell Response to Successive Canine Parvovirus Infection Based on Kinetic Proteomic Change Identification. _Sci Rep_ 6, 29560 (2016).
https://doi.org/10.1038/srep29560 Download citation * Received: 25 January 2016 * Accepted: 20 June 2016 * Published: 13 July 2016 * DOI: https://doi.org/10.1038/srep29560 SHARE THIS ARTICLE
Anyone you share the following link with will be able to read this content: Get shareable link Sorry, a shareable link is not currently available for this article. Copy to clipboard
Provided by the Springer Nature SharedIt content-sharing initiative