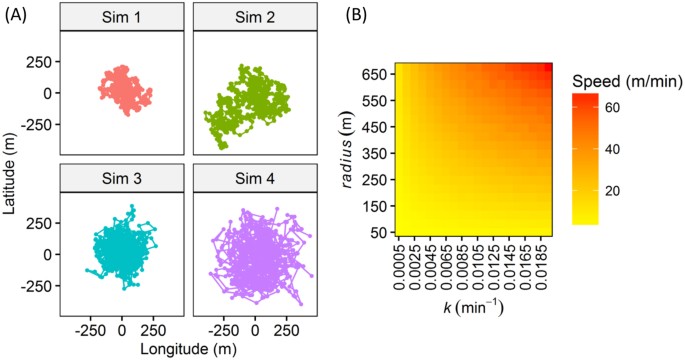
Fast and behavior-selective exploitation of a marine fish targeted by anglers
- Select a language for the TTS:
- UK English Female
- UK English Male
- US English Female
- US English Male
- Australian Female
- Australian Male
- Language selected: (auto detect) - EN
Play all audios:

ABSTRACT Harvesting of wild-living animals is often intensive and may selectively target heritable behavioral traits. We studied the exploitation dynamics and the vulnerability consequences
of individual heterogeneity in movement-related behaviors in free-ranging pearly razorfish (_Xyrichthys novacula_). Using underwater-video recording, we firstly document a fast and high
exploitation rate of about 60% of the adult population removed in just few days after the opening of the season. Subsequently, we tagged a sample of individuals with acoustic transmitters
and studied whether behavioral traits were significant predictors of the vulnerability to angling. Tagged individuals revealed repeatable behaviors in several home range-related traits,
suggesting the presence of spatial behavioral types. The individuals surviving the experimental fishery showed only localized and low-intensity movement patterns. Our study provides new
insights for understanding the harvesting pressures and selective properties acting on behavioral traits of recreational fishing. Many fish stocks around the globe are today predominantly
exploited by recreational fisheries. The fisheries-induced change in fish behavior described here may be therefore widespread, and has the potential to alter food-webs, profitability of the
fisheries and to affect stock assessment by eroding catchability in the long-term. SIMILAR CONTENT BEING VIEWED BY OTHERS MOVEMENTS AND SPATIAL DISTRIBUTION OF AN ENDANGERED FISH (_SCIAENA
UMBRA_) WITHIN A MARINE PROTECTED AREA Article Open access 07 February 2024 MOVEMENT BEHAVIOR OF SWORDFISH PROVISIONS CONNECTIVITY BETWEEN THE TEMPERATE AND TROPICAL SOUTHWEST PACIFIC OCEAN
Article Open access 21 July 2023 SEASONAL INFLUENCE ON THE BATHYMETRIC DISTRIBUTION OF AN ENDANGERED FISH WITHIN A MARINE PROTECTED AREA Article Open access 25 June 2021 INTRODUCTION Humans
exploit wild-living animals by way of fishing and hunting since the origin of our species1. Because fish feature high in our society’s demand for food and recreation, many fish stocks show
signs of overexploitation2, although many assessed stocks are beginning to recover or have already recovered in response to the implementation of proper management3. Most freshwater and some
coastal fish stocks in developed countries are today predominantly exploited by recreational fisheries4. The role of recreational fishing in the global fishing crisis is now considered
non-negligible5. However, the general lack of monitoring and assessment programs in recreational fisheries in many countries of the world imposes constraints on our ability to estimate
exploitation rates6,7. This is potentially problematic because knowledge of current exploitation rate relative to the sustainable fishing mortality rate and the relation of current to
unexploited spawning stock biomass are both crucial reference points, whose knowledge is essential for ensuring sustainable capture fisheries8. In addition to the extraction of biomass,
exploitation affects fish populations also by way of trait-selective harvesting9. There is now substantial evidence in the context of commercial fisheries that intensive and/or
size-selective harvesting selects for fast life-histories10,11. Less evidence for fisheries-induced evolution (FIE) in recreational fisheries exist, but models12, observational studies13,14,
and experiments15 have all demonstrated that fishing with hooks and lures can also generate similarly strong selection gradients than those observed in commercial fisheries. These findings
are not surprising given that annual exploitation rates in recreational angling can be as high as 80%16. Although the economic consequences of FIE for fisheries may be not be severe when
fisheries are managed sustainably17, the evolutionary consequences of recreational fishing will reduce the recovery ability of exploited stocks and the attractiveness of local fisheries to
anglers and business18. Identifying the traits that render fish vulnerable to harvest are therefore of importance for ensuring sustainable exploitation10. Vulnerability is a complex process
in recreational fisheries. Behavioral traits have been suggested to play a major role, either due to selection directly acting on such traits or due to indirect selection responses emerging
from correlation with life-history traits19,20. The argument underlying this assumption is that catching a fish with baited hooks, artificial lures, gill nets or traps strongly depends on
the behavior-driven encounter probability and the active decision of a fish to attack or ingest the bait/lure or enter the trap or gill net19. Hence, individual heterogeneity in relation to
behavioral traits such as boldness, space use, refuge seeking, energy acquisition (e.g., swimming activity) or aggression should play a major role in the vulnerability of fish to
recreational fishing gear21, but few empirical studies on this topic exist so far22. Moreover, the scant evidence of behavior-selective fishing that exist has generally been generated from
experimental settings in laboratories or in ponds (see ref. 23 for exception). Laboratory-based behavioral assays will rarely represent how the very same individuals behave in the wild24,
and so far due to the difficulty of tracking fishes over long periods of time in the wild, only a few papers have documented consistent individual heterogeneity in fish behavior in
free-ranging individuals targeted by fishers23,25,26. With the development of fine-scale aquatic telemetry and the development of novel statistical tools applied to movement data such as
state-space models (SSM), ecologists have now a powerful tool for studying individual behavioral heterogeneity _in situ_27 and how it correlates with the vulnerability of exploited wildlife
and fishes23,28. One of the behavioral traits that we can measure in the field using positional data is the home range, i.e., the area used by an animal to fulfil its normal activities29. An
individual’s home range is the outcome of a complex interplay of the environment and the intrinsic personality traits of the animals. Many animals develop home range and these can be highly
predictable at the individual level but strongly vary among individuals25. The process behind a home range development is Brownian motion (i.e., movement according to random stimuli), but
with a general tendency to remain around a specific area of interest (e.g., the center of the home range or a refuge, Fig. 130). Any correlation between vulnerability and fish home range
could have important ecological and evolutionary consequences by affecting the distribution of individuals and the spatial overlap of predators and prey20,31,32. Harvested animals in systems
where encounters with the human predator mainly determines the probability of capture are expected to display smaller home range areas and reduced exploration rates in response to
exploitation in agreement with the recently proposed “timidity syndrome”22. Empirical evidence demonstrating these predictions are however both scant and inconsistent. While two studies28,33
demonstrated that hunters selects against elk, _Cervus elaphus_ and pheasants, _Phasianus colchicus_ that exhibit large home ranges, other recent work23,34 failed to find a relationship
between fishing vulnerability and home range extension in Atlantic cod, _Gadus morhua_ and the European lobster, _Homarus gammarus_, respectively. Simulation models have also shown the
specific spatial patterns of harvesting by humans shapes the consistency and direction of selection gradients on home ranges21. By contrast, a consistent negative selection on how fast the
individual explores the home range was uncovered in theoretical models where encounters strongly shape vulnerability21. There is a need to validate these predictions to understand the
mechanisms behind the behavioral dimension of vulnerability. The objectives of our work were two-fold. First, we sought to estimate the exploitation rate induced by recreational anglers on
pearly razorfish _Xyrichtys novacula_ (Linnaeus, 1758) using a before-after-impact-control (BACI) design based on underwater video cameras. Second, we used a novel SSM model applied to
acoustic tracking data and investigated the individual heterogeneity in behavior and whether the home range behavior shown by individual fish was repeatable, hence representing a personality
trait25. We then tested which individuals were harvested after the opening of the fishing season within a few days using a survival approach, and whether vulnerability to capture was
related to the home range behavior. RESULTS RECREATIONAL HARVESTING DYNAMICS OF PEARLY RAZORFISH The GLMM fitted to _n_Max as proxy of abundance of pearly razorfish demonstrated a
significant (_p_ < 0.05) interaction effect of area (no-take vs. exploited) × period (before vs. after the seasonal opening of the fishery, Fig. 2). The sign of the interaction effect
indicated that the relative abundance of pearly razorfish strongly declined due to harvesting compared to the temporal trend in the unfished control area (Fig. 2). In general, the relative
abundance of fishes present in the no-take control (ntMPA) area was much greater than in the exploited area (pMPA) as would be expected from an exploited equilibrium (Fig. 2). The abundance
increased from the before to the after period in ntMPA due to natural fluctuations from a mean ± s.e. [CI] of 3.5 ± 0.16 [2.59, 4.85] individuals before to a value of 3.7 ± 0.16 [2.74, 5.06]
individuals, but these differences in abundance were non-significant (Fig. 2). By contrast, the exploited area experienced a significant decrease in relative abundance from 1.05 ± 0.16
[0.77, 1.43] individuals to 0.47 ± 0.23 [0.29, 0.75] individuals after exploitation (Fig. 2). The dispersion of the residuals of the abundance model was estimated as 1.1. Thus, no evidence
for over-dispersion was observed, suggesting a proper fit of the data using the Poisson distribution. Considering the slight temporal fluctuations in fish abundance observed in the control
site and the significant decrease observed in the exploited population, we estimated a very rapid and intense exploitation rate of 57% [13.9, 79.2] over just three weeks in the pearly
razorfish fishery. INDIVIDUAL HETEROGENEITY AND REPEATABILITY OF HOME RANGE BEHAVIOR The Bayesian SSM applied to the acoustic tracking detections (Fig. 3) revealed that tagged fish varied
widely in their home range-related behaviors and distributed their home ranges across the entire array of omnidirectional receivers (Table 1). The means and s.d. for the home range-related
behavioral traits were 217 ± 100 m for the variable _radius_ and 0.006 ± 0.005 min−1 for the variable exploration rate (_k_). Overall, the acoustic tracking revealed strong among-individual
differences in both mechanistic descriptors of home range suggesting high individual heterogeneity in home range behavior in our tagged sample of pearly razorfish. _Radius_ and _k_ were
uncorrelated and therefore provided independent measures of the intensity (_k_) and spatial extent (_radius_) of the home range behavior (Supplementary Material Fig. SM1). The estimated
repeatabilities (_R_) to assess the consistency of individual heterogeneity in home range behavior over time in the five chosen daily-based behavioral traits were generally high (Fig. 4). We
found latitude (_R__2012_ = 0.97[0.93, 0.99] and _R__2013_ = 0.93[0.82, 0.97]) and longitude (_R__2012_ = 0.96[0.92, 0.99] and _R__2013_ = 0.92[0.82, 0.97]) of the center of the activity to
be highly repeatable in both study years, with _R_ values close to 1. This indicated strong among-individual differences in the location of the center of the home range and in activity over
the study periods in both years (Fig. 4 and Table 2). The daily space utilization approximated by the bivariate fixed-kernel utilization distributions (50% and 95% KUD in km2) was also
repeatable, although values were slightly lower for the fish tagged in 2013 in relation to the 95% KUD: 50% KUD (_R__2012_ = 0.78[0.61, 0.94] and _R_2013 = 0.81[0.57–0.91]) and 95% KUD
(_R__2012_ = 0.85[0.65, 0.95] and _R__2013_ = 0.28[0.1, 0.56]). These results again indicated consistent differences in the spatial extent of the activity spaces across the two tracking
periods (Fig. 4 and Table 2). Finally, the _Rs_ of the daily distance travelled also indicated strong among-individual differences in movement activity in both study years (_R__2012_ =
0.93[0.8, 0.96] and _R__2013_ = 0.74[0.55, 0.89]). These results overall suggested that the specific home range behavior shown by individual pearly razorfish can be interpreted as a
repeatable behavioral trait, either being an outcome of personality or in the case of swimming activity a personality trait _per se_, thus demonstrating the existence of spatial behavioral
types (Fig. 4). Conventional daily metrics of behavior of the pearly razor fish just described were highly positively correlated with the _radius_ and _k_ obtained by the mechanistic
SSM-based home range approach (Supplementary Material Figs SM1 and SM2). Therefore, any result of the vulnerability analysis and posterior analysis of the selection gradients (see below)
using the parameters of the mechanistic home range model described above can be extrapolated to these conventional behavioral metrics. BEHAVIORAL DETERMINANTS OF VULNERABILITY IN AN
EXPLOITED ENVIRONMENT In line with the results obtained in the BACI experiment using underwater video cameras, a large proportion of the tagged and acoustically tracked fish (68%) were
harvested during a monitoring period of just 9 days after the opening of the harvesting season. After the AIC-reduction of the Cox model (see AIC-based ranking of all Cox models in
Supplementary Material Table SM1), the only variables retained were the home range-related behavioral traits, _radius_ and _k_, and both of them statistically affected the survivorship of
fish (Table 3). Neither the spatial location of the home range, year, sex nor two-order interactions explained enough variance to be retained by the model (Supplementary Material Table SM1).
The partial-effects survivorship plots show how, for example, the individuals characterized by a large value of the exploration intensity _k_ were quickly removed from the fishery leaving
behind fishes with smaller values for _k_ (Fig. 5). Similarly, the individuals with large _radius_ were quickly removed from the fishery (Fig. 5), overall suggesting that individual with
larger swimming speed according to Fig. 1 were selectively harvested. No other variables were related to the survivorship of the tagged individuals, including gender/size, suggesting that
vulnerability in a fished environment of our tagged sample of pearly razorfish was predominantly driven by home range and generally movement-related behavioral traits. NORMALIZED SELECTION
STRENGTH ON BEHAVIORAL TRAITS The trait-specific mean-standardized selection gradients (_S__μ_) were negative in all cases, collectively suggesting a reduced space use and smaller activity
levels of the remaining individuals of our tagged sample after the harvesting peak. Specifically, _S__μ_ was estimated as −1.43 for the parameter _radius_ and −0.52 for the parameter
exploration tendency _k_. Hence, selection by fishing was more strongly acting on the spatial extent compared to selection on exploration tendency. _S__μ_ for the latitude and longitude of
the center of the home range were also negative with values of −0.16 and −0.32, respectively. These findings collectively indicated recreational fishing-induced could favor selection towards
individuals characterized by small home range and reduced exploration intensity with small swimming speeds. DISCUSSION Our work is one of the first assessments of the largely overlooked
behavioral dimension of fish vulnerability to fishing gear directly measured in the wild10. Two of our findings are of particular importance. First, using fishery-independent methods we
documented a fast and remarkably high recreational fishing mortality that removed nearly 60% of all available adult pearly razorfish population over a very confined temporal scale of just a
few days. Second, our work is among the first to report a behavioral-mediated explanation for fish vulnerability acting on repeatable personality traits in the wild. Because the
vulnerability to harvest was found independent of gender, and by the same token body size, our work supports theoretical19 and empirical laboratory evidence in crayfish, _Cherax
destructor_35 that the mechanistic basis for vulnerability to passive fishing gear such as lures, hooks or traps is predominantly behavior-based rather than size-based. In fact, although
positive size-selective vulnerability to angling has been commonly reported in the recreational fisheries literature16, we propose that the underlying mechanisms of vulnerability to fishing
in many stocks will often be strongly or even exclusively behavior-based19. There is the perception that recreational fishing is less efficient, and hence potentially has smaller extractive
potential, compared to industrial marine fisheries36. However, due to cumulative effects in many coastal and freshwater stocks, recreational angling today is the major or single source of
fisheries mortality in some coastal stocks37,38. In our study area we estimated the exploitation rate induced by anglers using fishery-independent data (underwater cameras) and a BACI
design. Although exploitation rates could be directly estimated by mark and recapture studies observing tag returns of marked fishes by fishers39, estimating exploited biomass or abundance
and associated reference points using fishery-dependent data notoriously difficult and costly40. In addition, conventional stock assessment approaches based on fishery-dependent data are
prone to biases41,42. Our novel camera-based method is less sensitive to biases expected from fishery-dependent data (e.g., gear-selectivity, underreporting). Many coastal fish species are
characterized by moving in confined home ranges and they form discrete meta-populations43. In these particular cases, underwater video cameras applied to exploited sites before and after a
fishing peak could be easily implemented as a monitoring tool, especially in those coastal and inland areas intensively exploited by anglers with a lack of information on landings or tag
return. Our method to assess the change in abundance due to fishing determined that in just a couple of days the abundance of pearly razorfish was reduced by close to 60%. In our fishery
almost all annual fishing effort on the pearly razorfish in the study site is confined to a very limited temporal scale (Fig. 2). Hence, the “instantaneous” exploitation rates we estimated
for the pearly razorfish can be considered annual mortality rates for this species. The annual exploitation rate reported here ranges among the highest ever reported for marine recreational
fisheries16, and the total mortality of about 60% realized over just a few days mirrors the entire annual fishing mortality estimated for Atlantic cod exploited by anglers in southern
Norway44. Although we have no estimate of _F__MSY_ at our disposal, the sharp abundance decline in the exploited site after only few days of fishing relative to the control site suggests the
population could be close to experiencing recruitment overfishing, which often occurs when the spawning stock is reduced to below 35% of the virgin stock45. The exploitation rate is,
however, only one component assessing the sustainability of the fish stock, and one has to also provide data on the way recruitment happens in the focal fishery from spawners thriving
elsewhere along the coast8. It is our hope that our work stimulates the development of assessments and related population dynamics models that take into account the fast and high
exploitation rate caused by anglers obtained here. It is only then that we can finally conclude whether the fast and high exploitation rate documented here is sustainable or not. We found
that angler-induced exploitation of pearly razorfish is not only fast and intensive, but may also be non-random with respect to behavioral traits as revealed by our vulnerability experiment.
The major limitation of our work is that we do have an unbiased sample of movement behaviors of the population of razorfish. Instead, our vulnerability analysis was based on a subset of
fish behavioral types, given that all of the tagged fishes were captured by angling in the first place. No gear is entirely unselective for behavioral traits, which is why it is impossible
to create a random sample of behavioral types. Nevertheless, it is very likely that the behavioral types we studied represented a subset of the random population. Accordingly, the selection
gradients we estimated on behavioral traits are unlikely to represent the selection pressures at the population level. Despite this limitation, the selection portion of our study provides
strong evidence for the mechanistic basis of angling vulnerability, suggesting that corresponding selection pressures are likely to exist in nature. Moreover, we measured individual
heterogeneity of behavioral traits and vulnerability to harvest in the wild, adding realism to the study findings. All of the existing studies on the relationships of behavioral traits and
vulnerability to capture have either been entirely conducted in the laboratory35 or in semi-natural ponds46 or have tested wild-captured fish for personality in laboratory settings47,48,49.
However, there is an increasing evidence that laboratory-assayed behavior is not consistent with what the very same animals do in the wild and hence there is a need for testing relationships
among behavior and fitness in a natural contexts24. This fact highlights the relevance of our findings as they are closer to the reality of the system. We specifically have found that
movement parameters characterizing the extension of the home range and the exploration rate were the only predictors of the survivorship of the tracked individuals after the opening of the
fishery. Collectively, individuals with small foraging areas and exploration rates and with low average swimming speeds survived the harvesting season. This pattern was consistent across the
two years and independent of size/gender. Moreover, we found high repeatability of the daily home range-related behaviors. There is growing evidence about consistent (i.e., context-and
time-independent) individual heterogeneity in behavioral traits leading to personality traits that form behavioral types50. Our study, however, has revealed values of _R_s for some daily
movement metrics (daily space use, center of activity and travelled distance) that were higher than those reported in previous telemetry studies in the wild23,25. The limited body size of
the pearly razorfish required the use of very small acoustic tags (pico-tags), with a life-span of only a few days (Table 1). Moreover, acoustic tracking and in general any telemetry studies
are constrained to the limited sample size due to the high cost of the technology. Therefore, it is possible that our _Rs_ values were overestimated due to the limitations in the duration
of tracking and the potential temporal autocorrelation of the behaviors measured at daily scales51 and we thus have to be cautions with over-interpreting the exceedingly high _R_s. However,
a meta-analysis on animal personality reported average _R_ of 0.37 among 98 species across several taxa52, which were estimated by on average 4.4 repeated observations of behaviors. The data
in our work exceed in most of the cases these average values. We therefore feel that it is a safe conclusion that home range behavior is an expression of the underlying personality of the
pearly razorfish in agreement with other recent findings in other species23,25, conforming spatial behavioral types that are linked to the vulnerability of the individual to be harvested. We
concluded from our previous simulation work21 that consistent selection for low activity phenotypes should be expected across fishing styles, while the selection gradient on home range size
could range from positive to negative depending on the fishery and the inherent stochastic properties of fish movement. In this previous theoretical paper we simulated among-individual
variability according to a mean and s.d. of 200 ± 20 m of the size of the home range. Our new empirical data in the pearly razorfish suggests that this variability is actually much higher
(217 ± 100 m of the size of the home range) defining a much broader gradient of spatial behavioral types. The large behavioral gradient, and the fact that larger space uses increase the
spatial overlap between the fish and fishers and thus the encounter probability among them just by chance is likely the cause of why we found a negative selection gradient acting on spatial
extension of the home range in our fishery system. We also found that individuals characterized by a large exploration metric (_k_) were more quickly harvested from the fishery, generating
an estimate of a negative selection gradient on _k_, in agreement with our previous theoretical work21. The selection gradients that we estimated for _radius_ and _k_ was found to be high
compared with published values for a range of traits under natural selection, despite our sample being biased towards angled fish53. Our results therefore suggest the potential for
recreational fishing favoring individuals with low swimming speeds and contrived space, which could conform part of the timidity syndrome at population level recently described for exploited
systems22. A recent meta-analysis suggests that about 52% behavioral variation across taxa is attributable to additive genetic variation54. Our work therefore demonstrates that the
potential for FIE of behavioral traits certainly exists. Further work should therefore move forward exploring whether the population is actually responding this selective properties of
recreational fishing and individuals of the population are decreasing their space use while accounting for plastic responses. Until population-level data are available, we can only speculate
about a number of potential consequences of our findings. Recent work in largemouth bass, _Micropterus salmoides_ protected from fishing also shows that exploited bass are more timid, which
could have been caused by both evolution and learning similar to the case in pearly razorfish55. If pearly razorfish become increasingly difficult to capture, this behaviorally-related
mechanism will lower population-level catchability, which affects stock assessments and angling quality42. Moreover, behavioral traits such as exploration are usually positively correlated
with as growth rates and thus increased timidity and contrived space use could contribute to the downsizing of adults13. Fortunately, the quickly diminishing fishing effort on the pearly
razorfish after just three weeks of angling is evidence of a somewhat self-regulating fishery where anglers leave as abundance of vulnerable fish and catch rates drop. This
behavioral-mediated dynamic interplay of the pearly razorfish and anglers should be studied further in detail, in particular as relates to the replenishment dynamics of the adult stock
across years, which could contribute to the fishery being sustainable even at the high exploitation rates reported here. Considering the growing intensity by which many freshwater and marine
systems are being exploited by recreational gears38, we propose that the selective exploitation on movement behavioral traits may be widespread and can impact many different aspects of
ecosystem functioning and ecosystems services provided by wildlife and fishes. METHODS Methods were carried out in accordance with approved guidelines. Specifically, all experimental
protocols, including fish capturing and tagging, were approved by the authority responsible for marine natural resources of the study area (Fisheries Department of the Balearic Island),
through a permit to the CONFLICT Project (ref: CGL2008-00958) and to the REC2 Project (ref: CTM2011-23835). STUDY CASE: THE RECREATIONAL FISHERY OF THE PEARLY RAZORFISH We studied the pearly
razorfish population harvested in a local fishery located in the Balearic Islands (Fig. 2 and see ref. 56). The pearly razorfish is the main target of the fishery, it is very popular in the
Mediterranean, and the fishery is based on hook-and-line gear baited with natural baits (usually live shrimp). The fishery is located within a marine protected area (MPA) where two main
areas with different levels of protection are stipulated (Fig. 1): a no-take MPA (ntMPA) where all fishing activities are prohibited and a partial MPA (pMPA) where fishing for the pearly
razorfish is permitted outside a closed season (Fig. 2). All pearly razorfish fisheries of the Balearic Islands remain closed from April, 1 to August, 31 according to local legislation. This
fact not only creates a temporally clustered socially-related harvesting boost (Fig. 2), but also allows observing fish density and fish behavior before, across the exploitation phase and
after the fishery opening in both the fished and protected control area. The peculiar habitat configuration of the fishery strongly limits the dispersal of adult fishes (who do not cross
extended seagrass beds, Fig. 2), and the management of the fishery offered us a unique opportunity for testing our hypotheses. ESTIMATION OF EXPLOITATION RATE We randomly defined 49 sampling
points within the MPA of Palma Bay (n = 11 in ntMPA and n = 38 in the pMPA) (Fig. 2). We used a fishery-independent method for assessing the abundance of the pearly razorfish just before
and after the harvesting peak using a custom device to which a baited underwater video camera (Model GoPro Inc., © 2014) was attached. The video camera was deployed for a minimum of 30 min
in each of the sampling points, and we used _n_Max as proxy of real abundance, which is defined as the maximum number of individuals observed in a single frame during the viewing interval57.
We fitted a Poisson-GLMM in the R-package58, where _n_MAX was regressed on the interaction effect (the effect if interest) of site (no-take vs. exploited) × period (before vs. after) with
sampling site ID considered as random effect (over-dispersion was checked). We discarded spatial pseudo-replication of the sampling points by fitting the univariate Moran’s I
autocorrelation. The estimated interaction effect was then used to calculate the exploitation rate while controlling for the environmental-related changes in the abundance by comparing
exploited and control (baseline) sites. INDIVIDUAL HETEROGENEITY IN THE MOVEMENT BEHAVIOR OF THE PEARLY RAZORFISH We used the mechanistic approach proposed by ref. 30 to assess the
individual heterogeneity of home range-related behaviors in the wild based on the integration of a movement model (a random walk defined by an Ornstein–Uhlenbeck process) into a Bayesian SSM
using acoustic individual tracking data. According to the SSM proposed by ref. 30, four mechanistic behavioral parameters that lead the establishment of home range areas were estimated at
the individual level: the size of the circular home range (_radius_) in meters as a surrogate of the total foraging area and activity space, the harmonic force (exploration rate _k_ in
min−1) that represents how strongly a fish is attracted toward the center of its home range, i.e., how fast and intense the home range is explored, and the latitude and longitude of the
center of the home range. Figure 1 shows four fish trajectories simulated from our movement model to visualize how individual heterogeneity in _radius_ and _k_ affects the fish trajectory
and the average daily swimming speed. To feed the SSM with field data, we carried out a standard acoustic tracking experiment in the study area (Fig. 2). In August 2012 and 2013 before the
opening of the harvesting season wild pearly razorfish were captured by angling, tagged using tiny acoustic tags of the model Pico-Tag-2 (Sonotronics©) and tracked using an array of
omni-directional receivers (Table 1, Figs 2 and 3). The details of the tagging procedures are explained in ref. 56. The only practically possible, non-invasive method to capture live pearly
razorfish is hook-and-line. Therefore, the estimation of the individual heterogeneity in the movement behavior, as well as the study of the relationship between behavior and vulnerability
(see below) was based on angled fish. The main aim of our study was to provide evidence for spatial behavioral types and its relationship with vulnerability. Although the variability of
behavioral types was likely biased towards fish that are all in general vulnerable to angling gear, our approach was reasonable to study the mechanistic behavioral basis of vulnerability in
the wild. However, results on this portion of the study cannot be up-scaled to the whole population. After cleaning the data-base following the decision criteria tree for differentiating
among false and spurious detections proposed by ref. 56, the data was composed of 22 daily time-series of acoustic detections of individual fish (n = 11 fish in 2012 and n = 11 fish in 2013)
tagged before the opening of the fishery and tracked across the exploitation peak after the opening of the fishery (Fig. 3). The temporal series of acoustic detections were fitted to a SSM
according to ref. 30 to estimate the four home range movement parameters (_radius_, _k_, latitude and longitude) at the individual level. Three Markov chain Monte Carlo (MCMC) were run
assuming minimal prior knowledge30, and the convergence of the MCMC chains of all parameters was assessed by visual inspection of the plots of the iterations and tested using the
Gelman-Rubin Statistic59. The posterior distribution of each movement parameter was used to characterize each pearly razorfish behaviorally (i.e., parameters were used as traits).
REPEATABILITY OF MOVEMENT BEHAVIOR The repeatability score (_R_) assesses the degree of consistency of behaviors shown by individuals over time60. _R_ represents the phenotypic variation
attributable to individual heterogeneity and is often used to characterized animal personalities; it can be estimated as: where is the between-individual variance and is the
within-individual variance for a given behavioral trait. Our mechanistic approach to the home range behavior summarized the behavior of fish in relation to four movement parameters, which
was useful for testing our vulnerability hypothesis. However, this approach did not provide repeated measures for studying how consistent and repeatable the four behaviors were over time. To
obtain alternative repeated measures and estimate _R_, we calculated three conventional home range-based metrics on a daily basis using positional data similar to ref. 25. We first
calculated the daily space use defined as the area (in km2) using the 95% and 50% KUD in the same way as ref. 56. Secondly, we calculated the latitude and the longitude of the daily activity
for each fish as the average of the latitude and the longitude used data for each day. Thirdly, we estimated the total distance travelled (in m per day) using the summation of the Euclidean
distances between all two consecutive positions. MCMC-GLMM was used to decompose the raw phenotypic variance of each single behavioral metric into between- and within-individual variances,
and _R_ was computed following equation 1 using the library _MCMCglmm_ of the R-package51. The distribution of the residuals was inspected for proper fit of the data, and a transformation
(natural logarithm) was applied in the cases were the normality in the residuals was strongly deviating from a normal distribution. BEHAVIORAL DETERMINANTS OF VULNERABILITY IN A FISHED
ENVIRONMENT To examine whether home range behavior and other traits (e.g., body size or gender) were correlated with vulnerability (i.e., mortality in an exploited environment), a survival
model was fitted to the survivorship data of the tagged fish. Survival of each tagged fish was evaluated across/during the intensive harvesting period of 9 days, which covered the first two
weekends after the opening of the fishery that concentrated most of the fishing pressure of the year according to the effort dynamics of the fishery (see Figs 2 and 3 and Table 1). Moreover,
this monitoring period was chosen to keep the overall monitoring period from before to after fishing to within 23 days to avoid reaching the life-span of the small acoustic tags that we
used56. We assumed that the individual was harvested when the particular animal did no longer follow the normal diel behavior defined in ref. 56. No tag loss was observed in the 22 fish
according to the characteristic flat pattern of acoustic detections generated by tag loss described in our study area56. Any disappearing signal rendered harvest the most likely outcome for
the individual as the last position (likely location of fish when was captured) was clearly within the array of omni-directional receivers for all individuals that we tracked (see
Supplementary Material Fig. SM3). Note that the potential for tagging mortality was diagnosed following the decision criteria for acoustic tracking developed by ref. 56, and natural
mortality is highly unlikely during for this short time scale. For all of these reasons, it was reasonable to assume that the fish that were lost during the sampled period of 9 days were
harvested by anglers. We fitted a Cox- regression model to the binary (harvested or not) and continuous (survival days) response data using the _survival_ library of the R-package. The full
model included the individual mechanistic movement-related behavioral traits (_radius_, _k_ and the latitude and longitude of the center of the home range), the study year, gender and all of
the second order interaction with gender. Fish length was strongly correlated with gender as the pearly razorfish is a protogynous hermaphrodite, and we selected gender to be incorporated
in the Cox model. The coefficients of the Cox regression were estimated for the minimally adequate model ranked by the Akaike information criterion (AIC). The predicted survivorship
probabilities over the exploitation phase were plotted using the function _survfit_ of the R-package for significant effects. SELECTION GRADIENTS ON BEHAVIORAL TRAITS We calculated the
mean-standardized selection gradient (_S__μ_) acting on each movement trait according to ref. 61 as follows: where _S_ is the selection gradient defined as the difference between the
phenotypic mean of all tagged individuals and the mean of survivals, and _μ_P and are the mean and the variance of the movement trait of all tagged individuals prior to the harvesting
impact21. Note that _S__μ_ is a clean measure of selection strength and allows ranking the strength of selection acting on each of the various behavioral traits independent of the trait’s
mean and variance61. _S__μ_ can be interpreted as an elasticity of fitness to trait change61. For example, an _S__μ_ = 0.5 means that doubling the trait value elevates fitness by 50%.
ADDITIONAL INFORMATION HOW TO CITE THIS ARTICLE: Alós, J. _et al_. Fast and behavior-selective exploitation of a marine fish targeted by anglers. _Sci. Rep._ 6, 38093; doi: 10.1038/srep38093
(2016). PUBLISHER'S NOTE: Springer Nature remains neutral with regard to jurisdictional claims in published maps and institutional affiliations. REFERENCES * Hilborn, R. & Stokes,
K. Defining overfished stocks: have we lost the plot? Fisheries 35, 113–120, doi: 10.1577/1548-8446-35.3.113 (2010). Article Google Scholar * Worm, B. et al. Rebuilding global fisheries.
Science 325, 578–585, doi: 10.1126/science.1173146 (2009). Article CAS ADS PubMed Google Scholar * Costello, C. et al. Global fishery prospects under contrasting management regimes.
Proc. Natl. Acad. Sci. USA 113, 5125–5129, Online version, doi: 10.1073/pnas.1520420113 (2016). Article CAS ADS Google Scholar * Arlinghaus, R., Mehner, T. & Cowx, I. G. Reconciling
traditional inland fisheries management and sustainability in industrialized countries, with emphasis on Europe. Fish Fish. 3, 261–316, doi: 10.1046/j.1467-2979.2002.00102.x (2002). Article
Google Scholar * Cooke, S. J. & Cowx, I. G. The role of recreational fishing in global fish crises. Bioscience 54, 857–859 (2004). Article Google Scholar * Cooke, S. J. et al. Where
the waters meet: sharing ideas and experiences between inland and marine realms to promote sustainable fisheries management. Can. J. Fish. Aquat. Sci. 71, 1593–1601, doi:
10.1139/cjfas-2014-0176 (2014). Article Google Scholar * Arlinghaus, R. et al. Recommendations for the future of recreational fisheries to prepare the social-ecological system to cope with
change. Fish. Manag. Ecol. 23, 177–186, doi: 10.1111/fme.12191 (2016). Article Google Scholar * Hilborn, R. & Walters, C. Quantitative fisheries stock assessment: choice, dynamics and
uncertainty. (Chapman and Hall Inc., New York, 1992). * Allendorf, F. W. & Hard, J. J. Human-induced evolution caused by unnatural selection through harvest of wild animals. Proc. Natl.
Acad. Sci. USA 106, 9987–9994 (2009). Article CAS ADS Google Scholar * Heino, M., Pauli, B. D. & Dieckmann, U. Fisheries-induced evolution. An. Rev. Ecol. Evol. Syst. 46, 461–48
(2015). Article Google Scholar * Jørgensen, C. et al. Managing evolving fish stocks. Science 318, 1247–1248, doi: 10.1126/science.1148089 (2007). Article PubMed Google Scholar *
Matsumura, S., Arlinghaus, R. & Dieckmann, U. Assessing evolutionary consequences of size-selective recreational fishing on multiple life-history traits, with an application to northern
pike (_Esox lucius_). Evol. Ecol. 25, 711–735 (2011). Article Google Scholar * Alós, J. et al. Selective exploitation of spatially structured coastal fish populations by recreational
anglers may lead to evolutionary downsizing of adults. Mar. Ecol. Prog. Ser. 503, 219–233, doi: 10.3354/meps10745 (2014). Article ADS Google Scholar * Hessenauer, J.-M. et al. Differences
in the metabolic rates of exploited and unexploited fish oopulations: a signature of recreational fisheries induced evolution? PLoS ONE 10, e0128336, doi: 10.1371/journal.pone.0128336
(2015). Article CAS PubMed PubMed Central Google Scholar * Philipp, D. P. et al. Selection for vulnerability to angling in largemouth bass. Trans. Am. Fish. Soc. 138, 189–199 (2009).
Article Google Scholar * Lewin, W. C., Arlinghaus, R. & Mehner, T. Documented and potential biological impacts of recreational fishing: insights for management and conservation. Rev.
Fish. Sci. 14, 305–367 (2006). Article Google Scholar * Eikeset, A. M., Richter, A., Dunlop, E. S., Dieckmann, U. & Stenseth, N. C. Economic repercussions of fisheries-induced
evolution. Proc. Natl. Acad. Sci. USA 110, 12259–12264, doi: 10.1073/pnas.1212593110 (2013). Article ADS PubMed Google Scholar * Sutter, D. A. H. et al. Recreational fishing selectively
captures individuals with the highest fitness potential. Proc. Natl. Acad. Sci. USA 109, 20960–20965 (2012). Article CAS ADS Google Scholar * Uusi-Heikkilä, S., Wolter, C., Klefoth, T.
& Arlinghaus, R. A behavioral perspective on fishing-induced evolution. Trends Ecol. Evol. 23, 419–421 (2008). Article Google Scholar * Arlinghaus, R. et al. Passive gear-induced
timidity syndrome in wild fish populations and its potential ecological and managerial implications. Fish Fish., Published online, doi: 10.1111/faf.12176 (2016). * Alós, J., Palmer, M. &
Arlinghaus, R. Consistent selection towards low activity phenotypes when catchability depends on encounters among human predators and fish. PLoS ONE 7, e48030 (2012). Article ADS Google
Scholar * Arlinghaus, R. et al. Consumptive tourism causes timidity, rather than boldness, syndromes: a response to Geffroy _et al_. Trends Ecol. Evol. 31, 92–94, doi:
10.1016/j.tree.2015.11.008 (2016). Article PubMed Google Scholar * Olsen, E. M., Heupel, M. R., Simpfendorfer, C. A. & Moland, E. Harvest selection on Atlantic cod behavioral traits:
implications for spatial management. Ecol. Evol. 2, 1549–1562 (2012). Article Google Scholar * Niemelä, P. T. & Dingemanse, N. J. Artificial environments and the study of ‘adaptive’
personalities. Trends Ecol. Evol. 29, 245–247, doi: 10.1016/j.tree.2014.02.007 (2014). Article PubMed Google Scholar * Harrison, P. M. et al. Personality-dependent spatial ecology occurs
independently from dispersal in wild burbot (_Lota lota_). Behav. Ecol. 26, 483–49 (2014). Article Google Scholar * Nakayama, S., Laskowski, K. L., Klefoth, T. & Arlinghaus, R.
Between- and within-individual variation in activity increases with water temperature in wild perch. Behav. Ecol. in press, doi: 10.1093/beheco/arw090 (2016). * Hussey, N. E. et al. Aquatic
animal telemetry: a panoramic window into the underwater world. Science 348, 1255642–1255652 (2015). Article Google Scholar * Ciuti, S. et al. Human selection of elk behavioural traits in
a landscape of fear. Proc. R. Soc. Lond. B Biol. Sci. 279, 4407–4416, doi: 10.1098/rspb.2012.1483 (2012). Article Google Scholar * Burt, W. H. Territoriality and home range concepts as
applied to mammals. J. Mammal. 24, 346–352, doi: 10.2307/1374834 (1943). Article Google Scholar * Alós, J., Palmer, M., Balle, S. & Arlinghaus, R. Bayesian state-space modelling of
conventional acoustic tracking provides accurate descriptors of home range behavior in a small-bodied coastal fish species. PLoS ONE 11, e0154089, doi: 10.1371/journal.pone.0154089 (2016).
Article CAS PubMed PubMed Central Google Scholar * Boerger, L., Dalziel, B. D. & Fryxell, J. M. Are there general mechanisms of animal home range behaviour? A review and prospects
for future research. Ecol. Lett. 11, 637–650, doi: 10.1111/j.1461-0248.2008.01182.x (2008). Article Google Scholar * Villegas-Ríos, D., Moland, E. & Olsen, E. M. Potential of
contemporary evolution to erode fishery benefits from marine reserves. Fish Fish., online version, doi: 10.1111/faf.12188 (2016). * Madden, J. R. & Whiteside, M. A. Selection on
behavioural traits during ‘unselective’ harvesting means that shy pheasants better survive a hunting season. Anim. Behav. 87, 129–135, doi: 10.1016/j.anbehav.2013.10.021 (2014). Article
Google Scholar * Wiig, J. R., Moland, E., Haugen, T. O. & Olsen, E. M. Spatially structured interactions between lobsters and lobster fishers in a coastal habitat: fine-scale behaviour
and survival estimated from acoustic telemetry. Can. J. Fish. Aquat. Sci. 70, 1468–1476, doi: 10.1139/cjfas-2013-0209 (2013). Article Google Scholar * Biro, P. A. & Sampson, P. Fishing
directly selects on growth rate via behaviour: implications of growth-selection that is independent of size. Proc. R. Soc. Lond. B Biol. Sci. 282, 0962–8452 (2015). Article Google Scholar
* Cooke, S. J. & Cowx, I. G. Contrasting recreational and commercial fishing: searching for common issues to promote unified conservation of fisheries resources and aquatic
environments. Biol. Conserv. 128, 93–108 (2006). Article Google Scholar * Coleman, F. C., Figueira, W. F., Ueland, J. S. & Crowder, L. B. The impact of United States recreational
fisheries on marine fish populations. Science 305, 1958–1960 (2004). Article CAS ADS Google Scholar * Arlinghaus, R., Tillner, R. & Bork, M. Explaining participation rates in
recreational fishing across industrialised countries. Fish. Manag. Ecol. 22, 45–55, doi: 10.1111/fme.12075 (2015). Article Google Scholar * Fernández-Chacón, A., Moland, E., Espeland, S.
H., Kleiven, A. R. & Olsen, E. M. Causes of mortality in depleted populations of Atlantic cod estimated from multi-event modelling of mark–recapture and recovery data. Can. J. Fish.
Aquat. Sci. online version, doi: 10.1139/cjfas-2015-0313 (2016). * van Poorten, B. T., Walters, C. J. & Ward, H. G. M. Predicting changes in the catchability coefficient through effort
sorting as less skilled fishers exit the fishery during stock declines. Fish. Res. 183, 379–384, doi: http://dx.doi.org/10.1016/j.fishres.2016.06.023 (2016). Article Google Scholar *
Pauly, D., Hilborn, R. & Branch, T. A. Fisheries: does catch reflect abundance? Nature 494, 303–306 (2013). Article CAS ADS Google Scholar * Alós, J., Palmer, M., Trías, P.,
Díaz-Gil, C. & Arlinghaus, R. Recreational angling intensity correlates with alteration of vulnerability to fishing in a carnivorous coastal fish species. Can. J. Fish. Aquat. Sci. 72,
217–225, doi: 10.1139/cjfas-2014-0183 (2015). Article Google Scholar * Botsford, L. W. et al. Connectivity, sustainability, and yield: bridging the gap between conventional fisheries
management and marine protected areas. Rev. Fish Biol. Fish. 19, 69–95 (2009). Article Google Scholar * Kleiven, A. R. et al. Harvest pressure on coastal Atlantic cod _(Gadus morhua)_ from
recreational fishing relative to commercial fishing assessed from tag-recovery data.. PLoS ONE 11, e0149595, doi: 10.1371/journal.pone.0149595 (2016). Article CAS PubMed PubMed Central
Google Scholar * Mace, P. M. Relationships between common biological reference points used as thresholds and targets of fisheries management strategies. Can. J. Fish. Aquat. Sci. 51,
110–122, doi: 10.1139/f94-013 (1994). Article Google Scholar * Klefoth, T., Pieterek, T. & Arlinghaus, R. Impacts of domestication on angling vulnerability of common carp (_Cyprinus
carpio_ L.): the role of learning, foraging behaviour and food preferences. Fish. Manag. Ecol. 20, 174–186 (2013). Article Google Scholar * Härkönen, L., Hyvärinen, P., Paappanen, J. &
Vainikka, A. Explorative behavior increases vulnerability to angling in hatchery-reared brown trout (_Salmo trutta_). Can. J. Fish. Aquat. Sci. 71, 1900–1909, doi: 10.1139/cjfas-2014-0221
(2014). Article Google Scholar * Kekäläinen, J., Podgorniak, T., Puolakka, T., Hyvärinen, P. & Vainikka, A. Individually assessed boldness predicts _Perca fluviatilis_ behaviour in
shoals, but is not associated with the capture order or angling method. J. Fish Biol. 85, 1603–1616, doi: 10.1111/jfb.12516 (2014). Article PubMed Google Scholar * Wilson, A. D. M.,
Brownscombe, J. W., Sullivan, B., Jain-Schlaepfer, S. & Cooke, S. J. Does angling technique selectively target fishes based on their behavioural type? PLoS ONE 10, e0135848, doi:
10.1371/journal.pone.0135848 (2015). Article CAS PubMed PubMed Central Google Scholar * Conrad, J. L., Weinersmith, K. L., Brodin, T., Saltz, J. B. & Sih, A. Behavioural syndromes
in fishes: a review with implications for ecology and fisheries management. J. Fish Biol. 78, 395–435 (2011). Article CAS Google Scholar * Dingemanse, N. J. & Dochtermann, N. A.
Quantifying individual variation in behaviour: mixed-effect modelling approaches. J. Anim. Ecol. 82, 39–54, doi: 10.1111/1365-2656.12013 (2013). Article PubMed Google Scholar * Bell, A.
M., Hankison, S. J. & Laskowski, K. L. The repeatability of behaviour: a meta-analysis. Anim. Behav. 77, 771–783, doi: http://dx.doi.org/10.1016/j.anbehav.2008.12.022 (2009). Article
Google Scholar * Hereford, J., Hansen, T. F. & Houle, D. Comparing strengths of directional slection: how strong is strong? Evolution 58, 2133–2143, doi:
10.1111/j.0014-3820.2004.tb01592.x (2004). Article PubMed Google Scholar * Dochtermann, N. A., Schwab, T. & Sih, A. The contribution of additive genetic variation to personality
variation: heritability of personality. Proc. R. Soc. Lond. B Biol. Sci. 282, 0962–8452, doi: 10.1098/rspb.2014.2201 (2015). Article Google Scholar * Hessenauer, J.-M., Vokoun, J., Davis,
J., Jacobs, R. & O’Donnell, E. Loss of naivety to angling at different rates in fished and unfished populations of largemouth bass. Trans. Am. Fish. Soc. 145, 1068–1076, doi:
10.1080/00028487.2016.1194894 (2016). Article Google Scholar * Alós, J., Cabanellas-Reboredo, M. & Lowerre-Barbieri, S. Diel behaviour and habitat utilisation by the pearly razorfish
during the spawning season. Mar. Ecol. Prog. Ser. 460, 207–220, doi: 10.3354/meps09755 (2012). Article ADS Google Scholar * Schobernd, Z. H., Bacheler, N. M. & Conn, P. B. Examining
the utility of alternative video monitoring metrics for indexing reef fish abundance. Can. J. Fish. Aquat. Sci. 71, 464–471, doi: 10.1139/cjfas-2013-0086 (2013). Article Google Scholar * R
Core Team. R: a language and environment for statistical Computing. R Foundation for Statistical Computing, Vienna, Austria. URL https://www.R-project.org/. (2015). * Plummer, M., Best, N.,
Cowles, K. & Vines, K. CODA: convergence diagnosis and output analysis for MCMC. R News 6, 5 (2006). Google Scholar * Nakagawa, S. & Schielzeth, H. Repeatability for Gaussian and
non-Gaussian data: a practical guide for biologists. Biol. Rev. 85, 935–956 (2010). PubMed Google Scholar * Matsumura, S., Arlinghaus, R. & dieckmann, U. Standardizing selection
strengths to study selection in the wild: A critical comparison and suggestions for the future. Bioscience 62, 1039–1054 (2012). Article Google Scholar Download references ACKNOWLEDGEMENTS
This study was funded through a Marie Curie Post-Doc grant to JA (FP7-PEOPLE-2012-IEF, grant no. 327160. The study was also funded by the B-Types project funded through Leibniz Competition
(grant No. SAW-2013-IGB-2) to RA.). JA received additional funding from a Juan de la Cierva Post-doc grant (grant No. FJCI-2014-21239) and MP from the research project PHENOFISH (grant no.
CTM2015-69126-C2-1-R), CONFLICT (grant No. CGL2008-00958) and REC2 (grant no. CTM2011-23835) funded by the Spanish Ministry of Economy and Competiveness. We especially thank Toni Grau and
the staff of the _Direcció General de Pesca (Govern de les Balear)_ for supporting and authoring this study in the MPA of Palma Bay and researchers involved in the field for their help. We
thank two anonymous reviewers for their excellent feedback. AUTHOR INFORMATION AUTHORS AND AFFILIATIONS * Department of Biology and Ecology of Fishes, Leibniz-Institute of Freshwater Ecology
and Inland Fisheries, Müggelseedamm 310, Berlin, 12587, Germany Josep Alós & Robert Arlinghaus * Instituto Mediterráneo de Estudios Avanzados, IMEDEA (CSIC-UIB)., C/Miquel Marqués 21,
Esporles, 07190, Illes Balears, Spain Josep Alós, Miquel Palmer & Rosario Rosselló * Division of Integrative Fisheries Management, Faculty of Life Sciences and Integrative Research
Institute for the Transformation of Human-Environmental Systems (IRI THESys), Humboldt-Universität zu Berlin, Invalidenstrasse 42, Berlin, 10155, Germany Robert Arlinghaus Authors * Josep
Alós View author publications You can also search for this author inPubMed Google Scholar * Miquel Palmer View author publications You can also search for this author inPubMed Google Scholar
* Rosario Rosselló View author publications You can also search for this author inPubMed Google Scholar * Robert Arlinghaus View author publications You can also search for this author
inPubMed Google Scholar CONTRIBUTIONS J.A. and R.R. conducted the field work and collected the data. J.A., M.P. and R.A. analyzed the data. J.A., M.P. and R.A. wrote and modified the
manuscript. All authors reviewed the manuscript. ETHICS DECLARATIONS COMPETING INTERESTS The authors declare no competing financial interests. ELECTRONIC SUPPLEMENTARY MATERIAL SUPPLEMENTARY
INFORMATION RIGHTS AND PERMISSIONS This work is licensed under a Creative Commons Attribution 4.0 International License. The images or other third party material in this article are
included in the article’s Creative Commons license, unless indicated otherwise in the credit line; if the material is not included under the Creative Commons license, users will need to
obtain permission from the license holder to reproduce the material. To view a copy of this license, visit http://creativecommons.org/licenses/by/4.0/ Reprints and permissions ABOUT THIS
ARTICLE CITE THIS ARTICLE Alós, J., Palmer, M., Rosselló, R. _et al._ Fast and behavior-selective exploitation of a marine fish targeted by anglers. _Sci Rep_ 6, 38093 (2016).
https://doi.org/10.1038/srep38093 Download citation * Received: 15 April 2016 * Accepted: 04 November 2016 * Published: 06 December 2016 * DOI: https://doi.org/10.1038/srep38093 SHARE THIS
ARTICLE Anyone you share the following link with will be able to read this content: Get shareable link Sorry, a shareable link is not currently available for this article. Copy to clipboard
Provided by the Springer Nature SharedIt content-sharing initiative