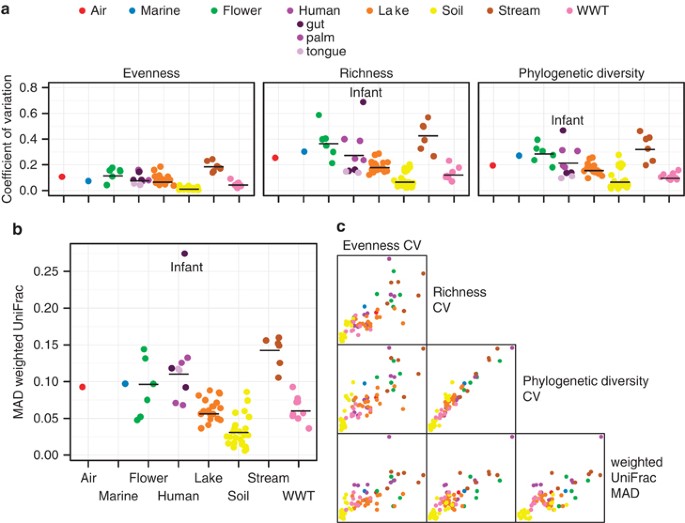
A meta-analysis of changes in bacterial and archaeal communities with time
- Select a language for the TTS:
- UK English Female
- UK English Male
- US English Female
- US English Male
- Australian Female
- Australian Male
- Language selected: (auto detect) - EN
Play all audios:

ABSTRACT Ecologists have long studied the temporal dynamics of plant and animal communities with much less attention paid to the temporal dynamics exhibited by microbial communities. As a
result, we do not know if overarching temporal trends exist for microbial communities or if changes in microbial communities are generally predictable with time. Using microbial time series
assessed via high-throughput sequencing, we conducted a meta-analysis of temporal dynamics in microbial communities, including 76 sites representing air, aquatic, soil, brewery wastewater
treatment, human- and plant-associated microbial biomes. We found that temporal variability in both within- and between-community diversity was consistent among microbial communities from
similar environments. Community structure changed systematically with time in less than half of the cases, and the highest rates of change were observed within ranges of 1 day to 1 month for
all communities examined. Microbial communities exhibited species–time relationships (STRs), which describe the accumulation of new taxa to a community, similar to those observed previously
for plant and animal communities, suggesting that STRs are remarkably consistent across a broad range of taxa. These results highlight that a continued integration of microbial ecology into
the broader field of ecology will provide new insight into the temporal patterns of microbial and ‘macro’-bial communities alike. SIMILAR CONTENT BEING VIEWED BY OTHERS PRIORITY EFFECTS IN
MICROBIOME ASSEMBLY Article 27 August 2021 INTEGRATION OF TIME-SERIES META-OMICS DATA REVEALS HOW MICROBIAL ECOSYSTEMS RESPOND TO DISTURBANCE Article Open access 19 October 2020
META-ANALYSIS OF MULTIDECADAL BIODIVERSITY TRENDS IN EUROPE Article Open access 13 July 2020 INTRODUCTION Understanding how communities are structured in time is a fundamental pursuit in
ecology. There is a long history of research on temporal dynamics in animal and plant communities (for example, Preston, 1960; Holling, 1973; McNaughton, 1977; Pimm, 1984; Ives et al., 2003;
Ives and Carpenter, 2007), and this research has been integral to the development of a wide variety of concepts in ecology. In particular, research on long-term changes in plant and animal
diversity have been instrumental in helping ecologists recognize successional dynamics (for example, Lockwood et al., 1997; Chase, 2003), identify relationships between community stability
and biodiversity (Cottingham et al., 2001; White et al., 2006) and predict how communities may respond to disturbances, including longer-term global changes (for example, Fraterrigo and
Rusak, 2008; Magurran et al., 2010). Though microorganisms are ubiquitous, abundant and have critical roles in ecosystems, far less is known about the temporal dynamics exhibited by
microbial communities relative to those exhibited by communities of larger organisms. A growing collection of site-specific studies in the microbial ecology literature suggests that
microbial communities exhibit a wide range of discernable temporal patterns. For example, patterns of primary succession in an infant gut (Koenig et al., 2011) and on leaf surfaces (Redford
and Fierer, 2009) as well as patterns of recurring seasonality in aquatic systems (Fuhrman et al., 2006; Shade et al., 2007; Eiler et al., 2012; Gilbert et al., 2012) have demonstrated that
some microbial communities change directionally, according to environmental conditions. By contrast, patterns of stability in wastewater treatment systems suggest that some microbial
communities are composed of core members that exhibit minimal temporal variability and rarer taxa that exhibit more pronounced fluctuations in abundance over time (Werner et al., 2011).
Further, in a range of systems, including gut microbiota challenged with an antibiotic (Dethlefsen et al., 2008), lake microbial communities after water column mixing (Jones et al., 2008;
Shade et al., 2012b) and soil communities of denitrifiers and nitrite oxidizers after exposure to increased temperature (Wertz et al., 2007), some microbial communities have the capacity to
recover quickly after disturbance events, either to the pre-disturbance state or to an alternative stable state (Shade et al., 2012a). Overall, these and other time series highlight that
microbial communities, like plant and animal communities, are dynamic and exhibit temporal patterns that can reflect underlying biotic and abiotic processes. Because changes in microbial
community composition are often associated with changes in the functional capabilities of those communities (for example, Bell et al., 2005; Fierer et al., 2007; Strickland et al., 2009),
understanding microbial temporal patterns can be critical for understanding ecosystem processes. Despite this importance, our ability to generalize microbial community dynamics has been
limited by a focus on site- or habitat-specific research. However, the ever-increasing accumulation of 16S rRNA gene sequence data allows for comparison of microbial communities across
habitats. Though microbial communities from different habitats clearly differ in composition (Lozupone and Knight, 2007; Nemergut et al., 2011), it is unknown whether there are common
patterns in the dynamics or variability of microbial communities through time and across habitats. To date, there has been no concerted analysis of microbial temporal dynamics across biomes,
and thus understanding these dynamics and determining their commonalities are key challenges in microbial ecology. Aquatic systems are perhaps the most frequently studied microbial
communities through time, due, in part, to the relatively large number of long-term ecological studies conducted in aquatic systems. As a result, published time series of aquatic microbial
communities have yielded key insights into the drivers of microbial dynamics in marine and freshwater systems. For instance, we know that physicochemical changes in the environment often
drive shifts in aquatic microbial communities, as evident from the observation that marine, lake and river communities frequently exhibit pronounced seasonality (Hofle et al., 1999; Fuhrman
et al., 2006; Shade et al., 2007; Nelson, 2008; Crump et al., 2009; Eiler et al., 2012). Furthermore, there is evidence that phytoplankton and bacterioplankton communities appear to be
synchronous in some systems (Kent et al., 2007), potentially linked by the consumption of phytoplankton-specific exudates by heterotrophic bacterioplankton (Paver and Kent, 2010). These
select examples of aquatic microbial time series (culled from hundreds in the literature), demonstrate the importance of time series analysis for identifying the important biotic and abiotic
drivers of microbial community structure. There are clear challenges to studying microbial communities through time. First, it is often difficult to characterize the micron-scale niches for
microorganisms, and therefore the immediate environment experienced by many microbial communities remains unknown (Brock, 1987). Additionally, the timescales over which the greatest
microbial community changes occur are typically unknown (Shade and Peter et al., 2013). Depending on the habitat, survey efforts may lack the temporal resolution to capture rapid community
changes, particularly in systems with actively growing microbial populations, in which generation times may be on the order of minutes. Finally, surveying microbial diversity can be a
daunting task. Individual samples often harbor hundreds to thousands of individual microbial taxa (Curtis and Sloan, 2004; Schloss and Handelsman, 2007; Quince et al., 2008), and the
majority of microorganisms cannot be identified using traditional culture-based techniques (Pace, 1997; Schloss and Handelsman, 2007). Fortunately, the ongoing development of
culture-independent tools and high-throughput sequencing technologies has made it feasible to describe the temporal dynamics of microbial communities at time scales and resolutions that were
previously unattainable (Gonzalez et al., 2012). We conducted a meta-analysis of newly available time series of microbial communities assessed via high-throughput sequencing of the 16S rRNA
gene, which permits the detailed analysis of microbial community changes through time. Our objective was to characterize temporal dynamics of microbial communities from a suite of habitats,
and when possible, to compare these dynamics with communities of larger organisms. To assess whether temporal patterns were common across both microbial and ‘macro’-bial organisms, we
applied analyses previously applied to temporal patterns in plant and animal communities. We address the following outstanding questions: How variable are microbial communities over time,
and how does this variability compare within habitats? What kinds of temporal patterns are often exhibited by microbial communities, and at what scales are these patterns most apparent? Do
microbial communities exhibit species–time relationships (STRs), and if so, are those relationships similar to those for larger organisms? MATERIALS AND METHODS DATA SETS We compiled
bacterial and archaeal time series from 76 sites, spanning a wide range of study durations and habitats, including aquatic, air, brewery wastewater treatment, soil, plant- and
human-associated communities. Fungal and other eukaryotic communities were not included in the meta-analysis. Each site had a minimum of five observations through time, and sites having a
destructive sampling regime (for example, soils) were concatenated into a single time series. The compiled time series included eight seasonally sampled temperate bog lakes in Wisconsin,
USA, each monitored at two locations: one sample from the upper, mixed layer (epilimnion) and one sample from the lower stratified layer (hypolimnion). These time series included between 1
and 3 years of approximately once or twice weekly observations during the ice-free period (Shade et al., 2008). Additional lake microbial communities were sampled intensively over the month
of a whole-ecosystem disturbance experiment at three depths in the lake (Shade et al., 2012b). A second data set included air from near-surface troposphere samples from a mountaintop
location in Colorado, USA (Bowers et al., 2012). The air communities were sampled continuously for hours over a few days, with samplings occurring approximately every month for about a year.
Nine brewery wastewater treatment communities were sampled once per month for a year, and two of those sites had been previously sampled a few years before (Werner et al., 2011). The
six-year English Channel time series, a coastal marine system, provided our longest series of monthly samples (Caporaso et al., 2012; Gilbert et al., 2012). Flower-associated microbial
communities were sampled from six apple trees over the lifespan of the flowers (1 week), including five time points from before flowers opened until petal fall (Shade et al., 2013). In a
study of human-associated microbiota, the palm, oral and gut microbial communities from two human subjects were collected approximately daily for a year for a male subject, and daily for 6
months for a female subject (Caporaso et al., 2011). Gut microbiota were also sampled from one infant across dietary shifts for the first 2.5 years of life (Koenig et al., 2011).
Agricultural soils at the Kellogg Biological Station, Michigan, USA maintained under different management regimes were sampled monthly for 6 months (Lauber _et al._, 2013) and soils from
National Ecological Observatory Network (NEON) sites in Hawaii and Florida, USA (www.neon.org) were sampled once per month for 3 months. Finally, six freshwater streams in Colorado, USA were
sampled every 1–2 weeks for approximately 1 year (Portillo et al., 2012). Additional details about each data set are provided in Supplementary Table S1 and in the associated references.
Clearly, these sample sets vary widely with respect to their sampling intensity and study duration. However, this is unavoidable if we want to assess temporal dynamics for microbial
communities from diverse environments, and, as has been demonstrated for meta-analyses of plant and animal community dynamics (Nekola and White, 1999; White et al., 2006; Soininen et al.,
2007; Korhonen et al., 2010; White et al., 2010), we can still describe patterns that would not be evident if we were to restrict our analyses to a far more limited set of sample types.
SEQUENCE ANALYSES The microbial communities in each of the 3431 individual samples were characterized by sequencing a portion of the 16S rRNA gene on either the Illumina (San Diego, CA, USA)
or 454 (Branford, CT, USA) platforms. The 16S rRNA gene is widely used for determining the phylogenetic and taxonomic composition of bacterial communities and, in all the cases, the data
were derived from PCR amplification of environmental DNA using primer pairs designed to amplify the gene region from all, or nearly all, known bacterial taxa. A closed reference operational
taxonomic unit (OTU) picking protocol was applied to each data set separately (Caporaso et al., 2012). Briefly, OTUs were assigned based on 97% sequence identity to sequences in the
Greengenes reference database (McDonald et al., 2012) preclustered at 97% identity (http://qiime.org/home_static/dataFiles.html). As we used the same reference-based OTU picking strategy for
all samples, we could directly compare the relative abundances of taxa across samples. Furthermore, we sub-sampled each individual data set such that all samples from a given data set were
compared at an equivalent sequencing depth (Supplementary Table S1). All analyses were performed on the rarefied OTU tables to permit comparisons of patterns in within- and between-community
diversity. Our goal was not to quantify the absolute diversity found in any of the samples: this task is difficult, if not impossible, because individual samples may harbor thousands of
rare taxa (Sogin et al., 2006). However, as recent work has demonstrated (Shaw et al., 2008; Kuczynski et al., 2010a), it is not necessary to characterize absolute diversity in order to
accurately describe changes in within-sample and between-sample diversity within and between habitat types. Pielou’s evenness (Pielou, 1969), richness (number of OTUs) and Faith’s
phylogenetic diversity (Faith, 1992) were used as within-sample (alpha) diversity metrics. Bray–Curtis was used as a taxon-based metric of differences in community composition (beta
diversity), and the dissimilarities were calculated from the rarefied OTU tables in R using the vegan package (Oksanen et al., 2011; R Development Core Team, 2011). QIIME (version 1.2.1,
(Caporaso et al., 2010) was used for constructing weighted and unweighted UniFrac distances. UniFrac is a commonly used phylogenetic distance metric to assess pairwise dissimilarity in
community composition and incorporates information about differences in phylogenetic composition of community members (Lozupone and Knight, 2005; Lozupone et al., 2011), with weighted
UniFrac accounting for differences in the relative abundances of community members. STATISTICAL ANALYSES All analyses were performed using the R environment for statistical computing (R
Development Core Team, 2011), with the aid of the vegan and ggplot2 packages (Wickham, 2009; Oksanen et al., 2011). To compare temporal variability in diversity across habitats having
inherently different diversities, we calculated the coefficient of variation (CV) in within-sample diversity for each community (Equation 1) where σ is the s.d. and _μ_ is the mean. We
calculated median absolute deviation (MAD) to compare variability in between-sample diversity (Equation 2). Finally, we calculated _z_-scores of richness to examine the step-wise variability
of richness through time, across data sets (Equation 3). where _χ_ is the raw value of richness, _μ_ is the mean of the sample, and _σ_ is the s.d. around the mean. In using the coefficient
of variation, median absolute deviation and _z_-scores of richness over time, we compared variability in diversity rather than absolute measures of diversity, which was most appropriate for
comparing communities from different habitats that were assessed using different protocols for 16S rRNA short-read sequencing. To assess whether there were patterns in community structure
that could be described by time between observations, we related community similarity/distance to time elapsed using Mantel tests with Pearson’s correlation on 999 permutations. We evaluated
the decay of community similarity over time (time-decay) using the same methods for calculating decay of community similarity over space (distance-decay; Nekola and White, 1999; Soininen et
al., 2007). Though assessment of distance-decay is common in the literature, it is less common to assess time-decay. To assess time-decay in microbial communities, we used a similar
approach adopted by Korhonen et al. (2010) to the meta-analysis of aquatic community time-decay. A log-linear model was fitted between the change in community structure (assessed by
pair-wise similarities or distances, including Bray–Curtis, UniFrac and unweighted UniFrac) and days elapsed. Community dissimilarities were converted to similarities by subtracting from
one. Similarities were log-transformed. The slope of the log-linear model is a rate of community change, sometimes referred to as turnover (Nekola and White, 1999). For plotting time-decay
examples from each biome, we applied lowess smoothing over windows the length of 5% of the total series. Because time-decay can be sensitive to the duration of the study, we also performed a
simple analysis of how quickly microbial communities change at temporal scales from 1 week to 1 month, from 1 to 6 months, from 6 months to 1 year, from 1 to 2 years, from 2 to 3 years,
from 3 to 4 years, from 4 to 5 years and from 5 to 6 years. These windows encompass the breadth of time series durations included in the meta-analysis. For all pairs of observations within a
community’s time series, a rate of change was calculated by dividing Bray–Curtis dissimilarity by the time between observations. Next, all pairs of observations were partitioned into the
appropriate temporal window (determined by the time between observations) and an average rate of change for each window was calculated. The global average rate of change was summarized
across sites from the same habitat (reported in Supplementary Table S2). STRs for each site were constructed in R by calculating richness using the moving window approach of White et al.
(2006). This approach involves partitioning a time series into as many window subsets as possible given the number of observations and fitting the STR model (the power-law relationship
between time and richness) at each window. For example, a 250-time point series could divided into one 250-point window, two 249-point windows, and so on. The power function, rather than the
lognormal function, was used so that our results would be directly comparable with the results from communities of larger organisms reported in White et al. (2006). There is some debate
regarding which function is most appropriate for describing the STR (McGill, 2003; White et al., 2006), but White et al. (2006) found that the log and power functions produced identical
patterns. We compared STR patterns in microbial communities with patterns for communities of larger organisms. However, microbial communities are widely considered to be more diverse than
communities of larger organisms, and it remains difficult to make a direct comparison, as discussed at length previously (Fierer and Lennon, 2011). There are caveats to the data sets used
here that are worth highlighting. First, high-throughput sequencing of 16S rRNA genes can introduce biases in the determination of microbial diversity; these limitations have been described
elsewhere (for example, Kunin et al., 2010; Haas et al., 2011; Quince et al., 2011; Soergel et al., 2012). Second, because sequencing depth varied across the sample sets (Supplementary Table
S1), it is difficult to directly compare the temporal dynamics of individual taxa across the data sets (particularly taxa that are relatively rare). For this reason, we have focused our
meta-analyses entirely on the overarching patterns in within- and between-sample diversity, which should be reasonably robust to differences in sequencing depth (Kuczynski et al., 2010b);
however, we also tested whether inter-biome differences were a byproduct of differences in sequencing depth. Third, we picked OTUs using a closed-reference database protocol to compare data
sets generated using different primers that target different variable regions of the 16S rRNA gene (as done in Caporaso _et al._, 2011). Although this method allowed us to directly compare
data sets using the same reference phylogeny, any sequences not matching the well-curated database were discarded from the analyses. Therefore, communities that had better representation of
taxa in the database (for example, human-associated communities) had a higher proportion of taxon assignments than, for instance, flower communities, where a larger percentage of the
sequences lack a close match to those found in the database. However, we found no relationship between the proportion of sequences that matched the reference database and patterns of
temporal variability, suggesting that frequency of matches to the reference database did not bias overall patterns. RESULTS AND DISCUSSION TEMPORAL VARIABILITY IN WITHIN-SAMPLE DIVERSITY We
used coefficients of variation calculated over each site’s time series to compare the variability in community evenness (equitability of representation of taxa), richness (number of taxa)
and phylogenetic diversity (breadth of lineages). Variability in these diversity metrics was generally consistent across sites representing the same habitat (Figure 1a). Evenness exhibited
less variability than richness or phylogenetic diversity over time. This is likely due to the exceedingly uneven nature of microbial communities (for example, Dethlefsen et al., 2008; Quince
et al., 2008), where there are few very prevalent community members and a relatively large number of rare members (a ‘long tail’ distribution). Variability in community structure (beta
diversity), measured as the median absolute deviation in weighted UniFrac distances, exhibited similar patterns as the variability in other diversity metrics (Figure 1b). Supplementary
Figure S1 additionally provides for each time series a visualization of every time point’s deviation from the mean richness. Variability in evenness, richness, phylogenetic diversity and
community structure were all highly correlated (Figure 1c), demonstrating that both phylogenetic (phylogenetic diversity and UniFrac) and taxonomic (evenness and richness) approaches to
community diversity revealed similar overarching patterns in temporal variability across sites. This was surprising because we did not necessarily expect phylogenetic metrics, such as
phylogenetic diversity, and taxonomic metrics, such as richness, to vary consistently with one another in time. This result suggests that the diversity metric used to investigate temporal
variability may not matter, allowing robust comparisons of microbial diversity measured in different ways across different studies. Furthermore, differences in temporal variability across
sites and habitats were not likely an artifact of differences in survey effort (the number of sequences per sample) or an artifact of our ability to identify taxa against the reference
database (Figure 2, Pearson’s correlation, all _P_-values >0.10). These results suggest that there are often clear differences in the temporal variability in both within- and
between-sample diversity within bacterial communities from different environment types. Overall, soil and brewery wastewater treatment communities were consistently less variable than other
community types (Figure 1). Though we cannot know _a priori_ the drivers governing the observed rates of change, we can generate reasonable hypotheses based on knowledge of the biology and
ecology of a microbial habitat. For example, we may expect a relatively low degree of variability in soil communities for two reasons. First, soil environmental conditions may simply be less
variable at the timescale of the included studies (6 months) than some of the other habitats considered in this study. Second, soil communities contain a large proportion of dormant
organisms (Lennon and Jones, 2011) and because our sequencing approach could not discriminate between active and inactive members, the communities may appear to change relatively little over
the given time scale. Soils also have high spatial heterogeneity, which can mask changes in local communities with time because of high community variability across micro-sites. However,
brewery wastewater treatment communities may vary minimally for different reasons. Wastewater treatment facilities have been engineered to perform a function (for example, nutrient removal
Curtis and Sloan, 2006; Martín et al., 2006; Harris et al., 2012). Thus, a wastewater treatment system may represent an environmental filter for microorganisms that survive in wastewater
treatment processes while system performance is maintained. However, operating conditions, such as feeding rate, and other environmental variables (including temperature) change over time in
wastewater treatment facilities, as was true for the environmental conditions in the system studied here (Werner et al., 2011). Therefore, these communities may have low variability simply
because of an environmental filter, rather than because of dormancy or invariant environmental conditions. Stream bacterioplankton communities, the infant gut community, flower communities
and human palm communities were highly variable in diversity (Figure 1). Again, we can generate hypotheses as to the drivers of this variability based on the knowledge of the microbial
habitat. Streams in the sub-alpine region studied here are flow-through systems with pronounced physicochemical instability. They experience frequent and rapid shifts in environmental
conditions as well as shifts in bacterial inputs into the stream channel (from sediment, soil and biofilms) that are likely driven by pulse flashes in hydrological conditions (Portillo et
al., 2012). Human palm sites were previously noted to be highly variable (Fierer et al., 2008; Caporaso et al., 2011), and this was attributed to frequent exposure to bacterial inocula from
a diverse array of touched surfaces and a high degree of temporal heterogeneity in environmental conditions (likely driven, in part, by the disturbances associated with washing events). In
this way, palm and stream communities both experience environmental conditions that can vary considerably over time. The time series that represent cases of microbial primary succession, the
infant gut and flower surfaces, also exhibited a high degree of temporal variability in diversity. In both cases, there was microbial colonization of a sterile (or nearly sterile) habitat
and rapid replacement of members through time as the environment is altered (Fierer et al., 2010). Thus, just as primary succession in plant communities can often lead to rapid changes in
diversity and community composition over time, we would also expect a high degree of variability in diversity for microbial communities undergoing succession. PREDICTABILITY IN MICROBIAL
COMMUNITY CHANGES OVER TIME AND ACROSS TEMPORAL SCALES We next asked whether communities sampled close together in time were, on average, more similar in composition than communities sampled
further apart. Approximately 26–40% of the 76 sites had community changes that were correlated with time over the entirety of study duration (Figure 3). Specifically, there were 34
significant relationships using Bray–Curtis, 26 relationships for unweighted UniFrac and 21 relationships for weighted UniFrac out of the 76 sites. Thus, in less than half of the sites did
we find that samples collected at shorter intervals harbored communities that were consistently more similar than those collected at longer intervals. However, there was at least one site
from each biome that exhibited community changes that were significantly correlated with time (Figure 3). Overall, these results suggest that temporal variability is not necessarily
predictable solely by considering the time between sampling events. Those communities that consistently exhibited temporal dependence included air, marine, human and lake communities. The
air, marine and lake community time series were sampled over a duration that included seasonal changes, and thus temporal trends are expected for these sites (Shade et al., 2008; Bowers et
al., 2012; Gilbert et al., 2012). Further, human-associated microbial communities sampled consecutively over time have been shown to be more similar than those sampled more distantly in time
(Costello et al., 2009), which is consistent with our detection of a temporal trend for these communities. These results suggest that overall temporal signals exist for communities from
certain habitats and that, for communities from these habitats, the relationship between community structure and time is insensitive to the choice of metric used for evaluating community
structure (Figure 3). Several habitats had no or few communities that exhibited correlations between time and community structure (Figure 3). For example, depending on the metric used,
anywhere from five to none of the 25 soil time series exhibited significant temporal dependence (Figure 3). For soil communities, inter-annual changes would not be evident in this data set,
as the study durations were ⩽6 months. Thus, it may be that changes in soil communities are not correlated with time at the time scale included but that such relationships could become
evident if we had time series extending across full years or multiple years. It is also not surprising that the structure of brewery wastewater treatment communities was often not correlated
with time, as any changes in operation would not necessarily be related to time. For the flower communities, the taxonomic metric, Bray–Curtis, detected a significant temporal signal
(Figure 3a) where the phylogenetic metrics did not (Figures 3b and c), likely because closely related taxa were replacing one another (Shade et al., 2013), a change to which a phlyogenetic
metric would be less sensitive. Only one of the stream communities was correlated with time, and this correlation was evident only when using the weighted UniFrac distance metric (Figure
3c). Because environmental conditions in the sampled streams are flashy with irregular pulse distances (Portillo et al., 2012), changes in stream communities may not be correlated with time
but rather with the occurrence of these infrequent events. Plant and animal communities often (but not always) become less similar with increasing time or geographical distance, a phenomenon
known as similarity-decay (Nekola and White, 1999). To determine whether there was decay of microbial community structure over time (time-decay), we fit log-linear models to community
similarity over differences in time between sample collections (Nekola and White, 1999; Korhonen et al., 2010; Figure 4). The rate of temporal change in community structure is the slope of
the model, and this slope is a measure of community turnover. A community that is not changing over time would have a slope of zero. More negative, steeper slopes indicate a faster rate of
change than less negative slopes. In Figure 4, very gradual time-decay is evident, even for microbial communities that exhibit seasonality, such as the coastal marine and lake sites, as well
as for sites that have high temporal variability, such as the stream community. Overall, 31–40% of microbial communities exhibited significant time-decay in community similarity
(Supplementary Figure S2, _P_<0.05). Slope was not correlated with richness (all _P_>0.05 for all similarity metrics), suggesting that differences in the number of taxa detected in
individual samples did not influence outcomes. Slopes were barely negative in most cases. However, one flower (Gala 2), one soil (HI_R12) and one brewery wastewater treatment (E1) had slopes
between −0.02 and −0.03. Aside from these exceptions, the consistency of microbial time-decay suggests that community turnover is generally quite slow. Time-decay can be sensitive to a
study’s duration. Therefore, we asked at what temporal scale communities changed the most quickly for the study duration and sampling intensities of observations that were available to us.
To do this, we calculated a simple rate of change by dividing pair-wise Bray–Curtis dissimilarity by the time between observations and then partitioned observations into nine broad temporal
ranges, from 1 day to 6 years (Supplementary Figure S3), representing the breadth of the available time series. This analysis also showed a consistent trend of slower change with longer
durations of time, and additionally reveals gaps in the currently available time series, and points to the temporal resolutions that are yet unknown for certain habitats (Supplementary Table
S2). Notably, just because microbial community turnover is very gradual at long study durations does not mean that there is no change occurring in these communities. Rather, it suggests
that there are not drastic or new changes occurring, such as the addition of new or phylogenetically distinct taxa. Also, it is possible that some of the communities may be changing around a
‘baseline’ of normal variability, following the conceptual model of ball and urn for alternative stable states (Beisner et al., 2003). STRS STRs describe the accumulation of richness in a
community, over increasingly longer durations (for example, Preston, 1960). The exponent of the STR provides an indication of the rate at which new taxa are observed in a community over
time; the higher the exponent the more new taxa are introduced over time. Depending on the scale of the study and the community of interest, STRs can be explained by sampling, ecological or
evolutionary effects (Preston, 1960; White et al., 2006, 2010). Over short-time periods, incomplete sampling effort (duration or intensity) often drives STRs, but evolutionary processes such
as speciation and extinction become more important for STRs over longer-time periods. Between these extremes, ecological processes such as meta-community dynamics are important for STR,
including dispersal of transient tourists into a regional species pool (Magurran and Henderson, 2003). Though the STR spatial equivalent, the species–area relationship, remains actively
investigated in microbial ecology (for example, Horner-Devine et al., 2004; Fierer and Jackson, 2006; Green and Bohannan, 2006; Martiny et al., 2006; Woodcock et al., 2006; Bell, 2010), STRs
are less often documented for microorganisms. However, STRs have been reported for communities from diverse environments, such as on leaf surfaces (Redford and Fierer, 2009), in streams
(Portillo et al., 2012) and in bioreactors (Van Der Gast et al., 2008), hinting that STRs may generally apply to microbial communities. We found that all communities had significant STRs
(_P_<0.05, Figure 5a). Microbial STRs were not related to study duration or sequencing depth (Pearson’s correlation _P_=0.81 and 0.36, respectively), suggesting limited, if any, influence
of sampling. However, our analysis does not distinguish stochastic processes (for example, random presence and absence of taxa) from deterministic properties in driving the STR, and both of
these likely contribute. There was a very consistent taxa–time relationship across microbial communities, ranging from 0.24 to 0.61. Communities had comparable STR exponents within biomes,
again highlighting the within-biome consistency. Furthermore, differences in the STR exponents across biomes could be explained by known ecological attributes of the communities. For
example, the air and stream communities, with high flow-through and heterogeneity, had high STR. The infant gut and flower primary succession communities also had high STR. The soil
communities had the lowest STR exponents, demonstrating that despite their high levels of diversity (for example, Elshahed et al., 2008), the taxa present at a given location do not change
appreciably over time (a pattern also noted in Fierer and Jackson, 2006). These results are consistent with the general trends in diversity over time as seen in Figure 1 and suggest that
fluctuating abiotic conditions drive higher microbial STRs. These environmental fluctuations potentially allow for time series to effectively encompass a wider range of microbial niches
(Shurin, 2007). We next asked whether STRs could be distinguished at the phylum level. For each community, we calculated STRs for common phyla observed within each habitat (Actinobacteria,
Bacteroidetes, Firmicutes, Proteobacteria and Verrucomicrobia), at each site. We found a range of STRs at the phylum-level, but the exponents had comparable means across phyla (Figure 5b).
This suggests that microbial phyla do not have inherently different rates of replacement but that the local ecology drives the observed STR within a community. One exception to this may be
the Firmicutes, which had a slightly higher mean STR and wider upper range than the other phyla. We speculate that this pattern may be a product of many taxa affiliated with Firmicutes being
capable of sporulation (Onyenwoke et al., 2004) and may either have higher rates of dispersal into communities or are able to persist in communities in very low abundances when conditions
are unfavorable and bloom when conditions become favorable. However, taxa affiliated with other phyla are also capable of persisting in dormant states, and more evidence is needed to address
this hypothesis. It can be difficult to directly compare STR patterns of microbial communities with communities of larger organisms. First, most microbes probably have generation times
shorter than those of larger organisms, and the microbial time series investigated here likely included far more microbial generations than comparable study durations of plant and animal
taxa. In addition, species definitions as applied to microbes are distinct from how plant and animal species are typically defined (Stackebrandt et al., 2002; Gevers et al., 2005). Finally,
microbial communities are often more diverse than communities of larger organisms, with individual samples harboring hundreds to thousands of taxa as compared with the tens of taxa reported
in White et al., (2006). However, despite these caveats, the range of STRs for microbial communities was the same as that reported in White et al., (2006) meta-analysis of STR for larger
organisms (Figures 5a and c). On average, STR exponents are higher for microorganisms than for larger organisms, and the exponents were less variable within communities. Though White et al.
(2006) found a relationship between STRs and richness in their meta-analysis, we found no such relationship for microbial communities (_P_=0.19), suggesting that microbial community STRs are
better explained by changes in environmental conditions than by local richness. However, the slightly higher average STR for microbial communities over macrobial communities may be due to
the generally higher richness of microbial communities. Random changes in the occurrences of these community members may partly be driven by the stochastic process of drift (Vellend, 2010),
which could also contribute to the STR. Nonetheless, the overall consistency in STRs across microbial and macrobial communities is striking, suggesting that this may be an ecological pattern
that is ubiquitous across scales, phyla and habitats. CONCLUSIONS AND FUTURE DIRECTIONS From this meta-analysis, we gain a preliminary understanding of how temporal patterns in microbial
communities compare with each other and with those of communities of larger organisms. There was consistent variability in diversity and STRs across communities from similar habitats. This
consistency can be leveraged when making logistical decisions about sampling regimes and for understanding baseline levels of temporal variability. Within a habitat, these temporal patterns
may represent the equilibrium from which disturbance events may alter microbial dynamics. This information is important for identifying when a microbial community is experiencing a
disturbance, anticipating how quickly a community may recover from such events, and determining if and when a community has recovered post-disturbance (Allison and Martiny, 2008; Shade et
al., 2012a; Shade and Peter et al., 2013). A challenge in conducting this meta-analysis was the availability of directly comparable microbial time series collected across habitats. The same
protocols and standards of quality across data sets were necessary for this undertaking, and though there are 76 microbial communities that span 8 distinct microbial biomes, there are
obviously many habitats that were not represented in the meta-analysis simply because data were not available. Further, some of the available time series were limited in duration or sampling
intensity, and therefore, it cannot be determined whether temporal patterns were not discovered because they truly do not exist or because of a limitation in the sampling effort. For
example, we may observe seasonality in soil microbial communities sampled over 6 years instead of over 6 months. With the increased availability of high-throughput sequencing, the number of
higher-resolution, longer-term time series will only increase. For example, the Earth Microbiome Project is leading a concerted effort to collect and curate high-quality microbial community
sequencing data and corresponding contextual data from diverse environments (Gilbert et al., 2010). Placing microbial community dynamics within the rich context of environmental dynamics
will provide insight into the key drivers of those communities, help to explain discrepancies in patterns across communities from similar habitats, and allow ecologists to begin predicting
microbial dynamics. Efforts like these will help to build theory for microbial ecology, advancing beyond system-specific observations (Prosser et al., 2007). Additional discussion about
challenges with sampling microbial communities in time (including defining a microbial community, sampling the same community longitudinally and accounting for micro-scale spatial
heterogeneity) is available as a Supplementary Discussion. This meta-analysis highlights that microbial communities, like plant and animal communities, are variable with time; that microbial
temporal dynamics are dependent on the habitat type in question; and, furthermore, that microbial temporal dynamics are often predictable. Perhaps more importantly, this work demonstrates
the utility of time course analyses in microbial ecology. Likewise, this meta-analysis highlights that microbial communities represent a useful system for studying temporal dynamics in
communities, dynamics that would be very difficult to explore in plant and animal communities where generation times are often far longer. For example, it is often easier to execute
disturbance experiments with microbial communities than with plant and animal communities, and microbial responses can be detected over relatively short-time periods (for example, Dethlefsen
et al., 2008; Shade et al., 2012b). These kinds of directed, _in situ_ experiments with microbial communities may provide key empirical validation (or invalidation) of theoretical
paradigms. Just as comparing the spatial patterns exhibited by microorganisms versus larger organisms has yielded interesting findings that have allowed us test paradigms in biogeography
(for example, Horner-Devine et al., 2004; Fuhrman et al., 2008; King et al., 2010), paradigms almost wholly derived from research on plant and animal communities, we can use microbial
communities to build a more comprehensive understanding of time–biodiversity relationships. The continued integration of microorganisms into the broader field of ecology will clearly be
advantageous for both ‘macro’-bial and microbial ecologists, providing rich insight into the common forces that shape patterns of distribution and diversity for organisms of all sizes.
REFERENCES * Allison SD, Martiny JBH . (2008). Resistance, resilience, and redundancy in microbial communities. _Proc Natl Acad Sci USA_ 105: 11512–11519. Article CAS PubMed PubMed
Central Google Scholar * Beisner B, Haydon D, Cuddington K . (2003). Alternative stable states in ecology. _Front Ecol Environ_ 1: 376–382. Article Google Scholar * Bell T . (2010).
Experimental tests of the bacterial distance-decay relationship. _ISME J_ 4: 1357–1365. Article PubMed Google Scholar * Bell T, Newman JA, Silverman BW, Turner SL, Lilley AK . (2005). The
contribution of species richness and composition to bacterial services. _Nature_ 436: 1157–1160. Article CAS PubMed Google Scholar * Bowers RM, McCubbin IB, Hallar AG, Fierer N .
(2012). Seasonal variability in airborne bacterial communities at a high-elevation site. _Atmos Environ_ 50: 41–49. Article CAS Google Scholar * Brock TD . (1987). The study of
microorganisms _in situ:_ progress and problems. In M Fletcher, TRG Gray, JG Jones (Eds.),. _Forty-first symposium of the Society for General Microbiology._ University of St. Andrews:
Cambridge University Press. * Caporaso JG, Kuczynski J, Stombaugh J, Bittinger K, Bushman FD, Costello EK _et al_ (2010). QIIME allows analysis of high-throughput community sequencing data.
_Nat Methods_ 7: 335–336. Article CAS PubMed PubMed Central Google Scholar * Caporaso JG, Lauber CL, Costello EK, Berg-Lyons D, Gonzalez A, Stombaugh J _et al_ (2011). Moving pictures
of the human microbiome. _Genome Biol_ 12: R50. Article PubMed PubMed Central Google Scholar * Caporaso JG, Paszkiewicz K, Field D, Knight R, Gilbert JA . (2012). The Western English
Channel contains a persistent microbial seed bank. _ISME J_ 6: 1089–1093. Article CAS PubMed Google Scholar * Chase JM . (2003). Community assembly: when should history matter? _Oecol_
136: 489–498. Article Google Scholar * Costello EK, Lauber CL, Hamady M, Fierer N, Gordon JI, Knight R . (2009). Bacterial community variation in human body habitats across space and time.
_Science_ 326: 1694. Article CAS PubMed PubMed Central Google Scholar * Cottingham KL, Brown BL, Lennon JT . (2001). Biodiversity may regulate the temporal variability of ecological
systems. _Ecol Lett_ 4: 72–85. Article Google Scholar * Crump BC, Peterson BJ, Raymond PA, Amon RMW, Rinehart A, McClelland JW _et al_ (2009). Circumpolar synchrony in big river
bacterioplankton. _Proc Natl Acad Sci USA_ 106: 21208–21212. Article CAS PubMed PubMed Central Google Scholar * Curtis TP, Sloan WT . (2004). Prokaryotic diversity and its limits:
microbial community structure in nature and implications for microbial ecology. _Curr Opin Microbiol_ 7: 221–226. Article PubMed Google Scholar * Curtis TP, Sloan WT . (2006). Towards the
design of diversity: stochastic models for community assembly in wastewater treatment plants. _Water Sci Technol_ 54: 227–236. Article CAS PubMed Google Scholar * Dethlefsen L, Huse S,
Sogin ML, Relman DA . (2008). The pervasive effects of an antibiotic on the human gut microbiota, as revealed by deep 16S rRNA sequencing. _PLoS Biol_ 6: e280. Article PubMed PubMed
Central Google Scholar * Eiler A, Heinrich F, Bertilsson S . (2012). Coherent dynamics and association networks among lake bacterioplankton taxa. _ISME J_ 6: 330–342. Article CAS PubMed
Google Scholar * Elshahed MS, Youssef NH, Spain AM, Sheik C, Najar FZ, Sukharnikov LO _et al_ (2008). Novelty and uniqueness patterns of rare members of the soil biosphere. _Appl Environ
Microbiol_ 74: 5422–5428. Article CAS PubMed PubMed Central Google Scholar * Faith DP . (1992). Conservation evaluation and phylogenetic diversity. _Biol Conserv_ 61: 1–10. Article
Google Scholar * Fierer N, Bradford MA, Jackson RB . (2007). Toward an ecological classification of soil bacteria. _Ecology_ 88: 1354–1364. Article PubMed Google Scholar * Fierer N,
Hamady M, Lauber CL, Knight R . (2008). The influence of sex, handedness, and washing on the diversity of hand surface bacteria. _Proc Natl Acad Sci USA_ 105: 17994. Article CAS PubMed
PubMed Central Google Scholar * Fierer N, Jackson RB . (2006). The diversity and biogeography of soil bacterial communities. _Proc Natl Acad Sci USA_ 103: 626–631. Article CAS PubMed
PubMed Central Google Scholar * Fierer N, Nemergut D, Knight R, Craine JM . (2010). Changes through time: integrating microorganisms into the study of succession. _Res Microbiol_ 161:
635–642. Article PubMed Google Scholar * Fierer N, Lennon J . (2011). The generation and maintenance of diversity in microbial communities. _Am J Bot_ 98: 439–448. Article PubMed Google
Scholar * Fraterrigo JM, Rusak JA . (2008). Disturbance-driven changes in the variability of ecological patterns and processes. _Ecol Lett_ 11: 756–770. Article PubMed Google Scholar *
Fuhrman JA, Hewson I, Schwalbach MS, Steele JA, Brown MV, Naeem S . (2006). Annually reoccurring bacterial communities are predictable from ocean conditions. _Proc Natl Acad Sci USA_ 103:
13104–13109. CAS PubMed PubMed Central Google Scholar * Fuhrman JA, Steele JA, Hewson I, Schwalbach MS, Brown MV, Green JL _et al_ (2008). A latitudinal diversity gradient in planktonic
marine bacteria. _Proc Natl Acad Sci USA_ 105: 7774. Article CAS PubMed PubMed Central Google Scholar * Gevers D, Cohan FM, Lawrence JG, Spratt BG, Coenye T, Feil EJ _et al_ (2005).
Re-evaluating prokaryotic species. _Nat Rev Microbiol_ 3: 733–739. Article CAS PubMed Google Scholar * Gilbert JA, Meyer F, Antonopoulos D, Balaji P, Brown CT, Desai N _et al_ (2010).
Meeting report: the terabase metagenomics workshop and the vision of an Earth Microbiome project. _Stand Genomic Sci_ 3: 243. Article PubMed PubMed Central Google Scholar * Gilbert JA,
Steele JA, Caporaso JG, Steinbrück L, Reeder J, Temperton B _et al_ (2012). Defining seasonal marine microbial community dynamics. _ISME J_ 6: 298–308. Article CAS PubMed Google Scholar
* Gonzalez A, King A, Robeson MS, Song SJ, Shade A, Metcalf JL _et al_ (2012). Characterizing microbial communities through space and time. _Curr Opin Biotechnol_ 23: 431–436. Article CAS
PubMed Google Scholar * Green J, Bohannan BJM . (2006). Spatial scaling of microbial biodiversity. _Trends Ecol Evol_ 21: 501–507. Article PubMed Google Scholar * Haas BJ, Gevers D,
Earl AM, Feldgarden M, Ward DV, Giannoukos G _et al_ (2011). Chimeric 16S rRNA sequence formation and detection in Sanger and 454-pyrosequenced PCR amplicons. _Genome Res_ 21: 494–504.
Article CAS PubMed PubMed Central Google Scholar * Harris J, Baptista J, Curtis T, Nelson A, Pawlett M, Ritz K _et al_ (2012). Engineering difference: matrix design determines community
composition in wastewater treatment systems. _Ecol Eng_ 40: 183–188. Article Google Scholar * Hofle MG, Haas H, Dominik K . (1999). Seasonal dynamics of bacterioplankton community
structure in a eutrophic lake as determined by 5S rRNA analysis. _Appl Environ Microbiol_ 65: 3164–3174. CAS PubMed PubMed Central Google Scholar * Holling CS . (1973). Resilience and
stability of ecological systems. _Annu Rev Ecol Syst_ 4: 1–23. Article Google Scholar * Horner-Devine MC, Lage M, Hughes JB, Bohannan BJM . (2004). A taxa-area relationship for bacteria.
_Nature_ 432: 750–753. Article CAS PubMed Google Scholar * Ives AR, Carpenter SR . (2007). Stability and diversity of ecosystems. _Science_ 317: 58–62. Article CAS PubMed Google
Scholar * Ives AR, Dennis B, Cottingham K, Carpenter SR . (2003). Estimating community stability and ecological interactions from time-series data. _Ecol Monogr_ 73: 301–330. Google Scholar
* Jones SE, Chiu CY, Kratz TK, Wu JT, Shade A, McMahon KD . (2008). Typhoons initiate predictable change in aquatic bacterial communities. _Limnol Oceanogr_ 53: 1319–1326. Article Google
Scholar * Kent AD, Yannarell AC, Rusak JA, Triplett EW, McMahon KD . (2007). Synchrony in aquatic microbial community dynamics. _ISME J_ 1: 38–47. CAS PubMed Google Scholar * King AJ,
Freeman KR, McCormick KF, Lynch RC, Lozupone C, Knight R _et al_ (2010). Biogeography and habitat modelling of high-alpine bacteria. _Nat Commun_ 1: 53. Article PubMed Google Scholar *
Koenig JE, Spor A, Scalfone N, Fricker AD, Stombaugh J, Knight R _et al_ (2011). Succession of microbial consortia in the developing infant gut microbiome. _Proc Natl Acad Sci USA_ 108:
4578. Article CAS PubMed Google Scholar * Korhonen JJ, Soininen J, Hillebrand H . (2010). A quantitative analysis of temporal turnover in aquatic species assemblages across ecosystems.
_Ecology_ 91: 508–517. Article PubMed Google Scholar * Kuczynski J, Costello EK, Nemergut DR, Zaneveld J, Lauber CL, Knights D _et al_ (2010a). Direct sequencing of the human microbiome
readily reveals community differences. _Genome Biol_ 11: 210. Article PubMed PubMed Central Google Scholar * Kuczynski J, Liu Z, Lozupone C, McDonald D, Fierer N, Knight R . (2010b).
Microbial community resemblance methods differ in their ability to detect biologically relevant patterns. _Nat Methods_ 7: 813–819. Article CAS PubMed PubMed Central Google Scholar *
Kunin V, Engelbrektson A, Ochman H, Hugenholtz P . (2010). Wrinkles in the rare biosphere: pyrosequencing errors can lead to artificial inflation of diversity estimates. _Environ Microbiol_
12: 118–123. Article CAS PubMed Google Scholar * Lauber CL, Ramirez KS, Aanderud Z, Lennon J, Fierer N . (2013). Temporal variability in soil microbial communities across land-use types.
_ISME J_ e-pub ahead of print 4 April 2013; doi: 10.1038/ismej.2013.50. * Lennon JT, Jones SE . (2011). Microbial seed banks: the ecological and evolutionary implications of dormancy. _Nat
Rev Microbiol_ 9: 119–130. Article CAS PubMed Google Scholar * Lockwood JL, Powell RD, Nott MP, Pimm SL . (1997). Assembling ecological communities in time and space. _Oikos_ 80:
549–553. Google Scholar * Lozupone C, Knight R . (2005). UniFrac: a new phylogenetic method for comparing microbial communities. _Appl Environ Microbiol_ 71: 8228–8235. Article CAS PubMed
PubMed Central Google Scholar * Lozupone C, Lladser ME, Knights D, Stombaugh J, Knight R . (2011). UniFrac: an effective distance metric for microbial community comparison. _ISME J_ 5:
169–172. Article PubMed Google Scholar * Lozupone CA, Knight R . (2007). Global patterns in bacterial diversity. _Proc Natl Acad Sci USA_ 104: 11436–11440. CAS PubMed PubMed Central
Google Scholar * Magurran AE, Baillie SR, Buckland ST, Dick JMP, Elston DA, Scott EM _et al_ (2010). Long-term datasets in biodiversity research and monitoring: assessing change in
ecological communities through time. _Trends Ecol Evol_ 25: 574–582. Article PubMed Google Scholar * Magurran AE, Henderson PA . (2003). Explaining the excess of rare species in natural
species abundance distributions. _Nature_ 422: 714–716. Article CAS PubMed Google Scholar * Martiny JBH, Bohannan BJM, Brown JH, Colwell RK, Fuhrman JA, Green JL _et al_ (2006).
Microbial biogeography: putting microorganisms on the map. _Nat Rev Microbiol_ 4: 102–112. CAS PubMed Google Scholar * Martín HG, Ivanova N, Kunin V, Warnecke F, Barry KW, McHardy AC _et
al_ (2006). Metagenomic analysis of two enhanced biological phosphorus removal (EBPR) sludge communities. _Nat Biotechnol_ 24: 1263–1269. Article Google Scholar * McDonald D, Price MN,
Goodrich J, Nawrocki EP, DeSantis TZ, Probst A _et al_ (2012). An improved Greengenes taxonomy with explicit ranks for ecological and evolutionary analyses of bacteria and archaea. _ISME J_
6: 610–618. Article CAS PubMed Google Scholar * McGill B . (2003). Strong and weak tests of macroecological theory. _Oikos_ 102: 679–685. Article Google Scholar * McNaughton SJ .
(1977). Diversity and stability of ecological communities: a comment on the role of empiricism in ecology. _Am Nat_, 515–525. * Nekola JC, White PS . (1999). The distance decay of similarity
in biogeography and ecology. _J Biogeogr_ 26: 867–878. Article Google Scholar * Nelson CE . (2008). Phenology of high-elevation pelagic bacteria: the roles of meteorologic variability,
catchment inputs and thermal stratification in structuring communities. _ISME J_ 3: 13–30. Article PubMed Google Scholar * Nemergut DR, Costello EK, Hamady M, Lozupone C, Jiang L, Schmidt
SK _et al_ (2011). Global patterns in the biogeography of bacterial taxa. _Environ Microbiol_ 13: 135–144. Article PubMed PubMed Central Google Scholar * Oksanen JF, Blanchet G, Kindt
R, Legendre P, Minchin PR, O’Hara R _et al_ (2011), vegan: Community Ecology Package, R package version 2.0, 2nd edn.. * Onyenwoke RU, Brill JA, Farahi K, Wiegel J . (2004). Sporulation
genes in members of the low G+C Gram-type-positive phylogenetic branch (Firmicutes). _Arch Microbiol_ 182: 182–192. Article CAS PubMed Google Scholar * Pace NR . (1997). A molecular view
of microbial diversity and the biosphere. _Science_ 276: 734–740. Article CAS PubMed Google Scholar * Paver S, Kent A . (2010). Temporal patterns in glycolate-utilizing bacterial
community composition correlate with phytoplankton population dynamics in humic lakes. _Microb Ecol_ 60: 406–418. Article PubMed Google Scholar * Pielou EC . (1969) _An Introduction to
Mathematical Ecology_. Wiley-Interscience: New York, NY, USA. Google Scholar * Pimm SL . (1984). The complexity and stability of ecosystems. _Nature_ 307: 321–326. Article Google Scholar
* Portillo MC, Anderson SP, Fierer N . (2012). Temporal variability in the diversity and composition of stream bacterioplankton communities. _Environ Microbiol_ 14: 2417–2428. Article
PubMed Google Scholar * Preston F . (1960). Time and space and the variation of species. _Ecology_ 41: 611–627. Article Google Scholar * Prosser JI, Bohannan BJM, Curtis TP, Ellis RJ,
Firestone MK, Freckleton RP _et al_ (2007). The role of ecological theory in microbial ecology. _Nat Rev Microbiol_ 5: 384–392. Article CAS PubMed Google Scholar * Quince C, Curtis TP,
Sloan WT . (2008). The rational exploration of microbial diversity. _ISME J_ 2: 997–1006. Article CAS PubMed Google Scholar * Quince C, Lanzen A, Davenport RJ, Turnbaugh PJ . (2011).
Removing noise from pyrosequenced amplicons. _BMC Bioinformatics_ 12: 38. Article PubMed PubMed Central Google Scholar * R Development Core Team (2011) _R: A Language and Environment for
Statistical Computing_. R Foundation for Statistical Computing: Vienna, Austria. * Redford AJ, Fierer N . (2009). Bacterial succession on the leaf surface: a novel system for studying
successional dynamics. _Microb Ecol_ 58: 189–198. Article PubMed Google Scholar * Schloss PD, Handelsman J . (2007). The last word: books as a statistical metaphor for microbial
communities. _Annu Rev Microbiol_ 61: 23–34. Article CAS PubMed Google Scholar * Shade A, Jones SE, McMahon KD . (2008). The influence of habitat heterogeneity on freshwater bacterial
community composition and dynamics. _Environ Microbiol_ 10: 1057–1067. Article CAS PubMed Google Scholar * Shade A, Kent AD, Jones SE, Newton RJ, Triplett EW, McMahon KD . (2007).
Interannual dynamics and phenology of bacterial communities in a eutrophic lake. _Limnol Oceanogr_ 52: 487–494. Article CAS Google Scholar * Shade A, McManus PS, Handelsman J . (2013).
Unexpected diversity during community succession in the apple flower microbiome. _mBio_ 4: e00602–e00612. Article PubMed PubMed Central Google Scholar * Shade A, Peter H, Allison SD,
Baho D, Berga M, Buergmann H _et al_ (2012a). Fundamentals of microbial community resistance and resilience. _Front Microbiol_ 3: 417. Article PubMed PubMed Central Google Scholar *
Shade A, Peter H, Allison SD, Baho DL, Berga M, Bürgmann H _et al_ (2013). Fundamentals of microbial community resistance and resilience. _Front Microbiol_ 3: 417. Google Scholar * Shade A,
Read JS, Youngblut ND, Fierer N, Knight R, Kratz TK _et al_ (2012b). Lake microbial communities are resilient after a whole-ecosystem disturbance. _ISME J_ 6: 2153–2167. Article CAS
PubMed PubMed Central Google Scholar * Shaw AK, Halpern AL, Beeson K, Tran B, Venter JC, Martiny JBH . (2008). It’s all relative: ranking the diversity of aquatic bacterial communities.
_Environ Microbiol_ 10: 2200–2210. Article PubMed Google Scholar * Shurin JB . (2007). How is diversity related to species turnover through time? _Oikos_ 116: 957–965. Article Google
Scholar * Soergel DA, Dey N, Knight R, Brenner SE . (2012). Selection of primers for optimal taxonomic classification of environmental 16S rRNA gene sequences. _ISME J_ 6: 1440–1444.
Article CAS PubMed PubMed Central Google Scholar * Sogin ML, Morrison HG, Huber JA, Welch DM, Huse SM, Neal PR _et al_ (2006). Microbial diversity in the deep sea and the underexplored
‘rare biosphere’. _Proc Natl Acad Sci USA_ 103: 12115–12120. Article CAS PubMed PubMed Central Google Scholar * Soininen J, McDonald R, Hillebrand H . (2007). The distance decay of
similarity in ecological communities. _Ecography_ 30: 3–12. Article Google Scholar * Stackebrandt E, Frederiksen W, Garrity GM, Grimont PAD, Kampfer P, Maiden MCJ _et al_ (2002). Report of
the ad hoc committee for the re-evaluation of the species definition in bacteriology. _Int J Syst Evol Microbiol_ 52: 1043–1047. CAS PubMed Google Scholar * Strickland MS, Lauber C,
Fierer N, Bradford MA . (2009). Testing the functional significance of microbial community composition. _Ecology_ 90: 441–451. Article PubMed Google Scholar * Van Der Gast CJ, Ager D,
Lilley AK . (2008). Temporal scaling of bacterial taxa is influenced by both stochastic and deterministic ecological factors. _Environ Microbiol_ 10: 1411–1418. Article PubMed Google
Scholar * Vellend M . (2010). Conceptual synthesis in community ecology. _Q Rev Biol_ 85: 183–205. Article PubMed Google Scholar * Werner JJ, Knights D, Garcia ML, Scalfone NB, Smith S,
Yarasheski K _et al_ (2011). Bacterial community structures are unique and resilient in full-scale bioenergy systems. _Proc Natl Acad Sci USA_ 108: 4158–4163. Article CAS PubMed PubMed
Central Google Scholar * Wertz S, Degrange V, Prosser JI, Poly F, Commeaux C, Guillaumaud N _et al_ (2007). Decline of soil microbial diversity does not influence the resistance and
resilience of key soil microbial functional groups following a model disturbance. _Environ Microbiol_ 9: 2211–2219. Article PubMed Google Scholar * White EP, Adler PB, Lauenroth WK, Gill
RA, Greenberg D, Kaufman DM _et al_ (2006). A comparison of the species–time relationship across ecosystems and taxonomic groups. _Oikos_ 112: 185–195. Article Google Scholar * White EP,
Ernest S, Adler PB, Hurlbert AH, Lyons SK . (2010). Integrating spatial and temporal approaches to understanding species richness. _Philos Trans R Soc Lond B Biol Sci_ 365: 3633–3643.
Article PubMed PubMed Central Google Scholar * Wickham H . (2009) _ggplot2: Eelegant Graphics for Data Analysis_. Springer: New York, NY, USA. Book Google Scholar * Woodcock S, Curtis
TP, Head IM, Lunn M, Sloan WT . (2006). Taxa-area relationships for microbes: the unsampled and the unseen. _Ecol Lett_ 9: 805–812. Article PubMed Google Scholar Download references
ACKNOWLEDGEMENTS This work would not have been possible without the generosity and collaborative spirit of the many primary authors of the data sets included in the meta-analysis. AS is a
Gordon and Betty Moore Foundation Fellow of the Life Sciences Research Foundation. We thank Petr Keil for insightful discussions. We thank Jack Gilbert for the Western English Channel
dataset, and Jack Gilbert and Trina McMahon for the lakes dataset. NF was supported by funding from the National Science Foundation and the US Department of Agriculture. RK was supported, in
part, by the Howard Hughes Medical Institute and the National Institutes of Health. AUTHOR INFORMATION AUTHORS AND AFFILIATIONS * Department of Molecular, Cellular and Developmental
Biology, Yale University, New Haven, CT, USA Ashley Shade & Jo Handelsman * Department of Computer Science, Northern Arizona University, Flagstaff, AZ, USA J Gregory Caporaso * Argonne
National Laboratory, Argonne, IL, USA J Gregory Caporaso * Department of Chemistry and Biochemistry and Biofrontiers Institute, University of Colorado, Boulder, CO, USA Rob Knight * Howard
Hughes Medical Institute, Boulder, CO, USA Rob Knight * Cooperative Institute for Research in Environmental Sciences, University of Colorado, Boulder, CO, USA Noah Fierer * Department of
Ecology and Evolutionary Biology, University of Colorado, Boulder, CO, USA Noah Fierer Authors * Ashley Shade View author publications You can also search for this author inPubMed Google
Scholar * J Gregory Caporaso View author publications You can also search for this author inPubMed Google Scholar * Jo Handelsman View author publications You can also search for this author
inPubMed Google Scholar * Rob Knight View author publications You can also search for this author inPubMed Google Scholar * Noah Fierer View author publications You can also search for this
author inPubMed Google Scholar CORRESPONDING AUTHOR Correspondence to Noah Fierer. ETHICS DECLARATIONS COMPETING INTERESTS The authors declare no conflict of interest. ADDITIONAL
INFORMATION Supplementary Information accompanies this paper on The ISME Journal website SUPPLEMENTARY INFORMATION SUPPLEMENTARY DISCUSSION (PDF 92 KB) SUPPLEMENTARY TABLES 1 AND 2 (DOC 212
KB) SUPPLEMENTARY FIGURE 1 (JPG 683 KB) SUPPLEMENTARY FIGURE 2 (PDF 16 KB) SUPPLEMENTARY FIGURE 3 (JPG 214 KB) SUPPLEMENTARY INFORMATION (DOC 73 KB) RIGHTS AND PERMISSIONS This work is
licensed under a Creative Commons Attribution-NonCommercial-ShareAlike 3.0 Unported License. To view a copy of this license, visit http://creativecommons.org/licenses/by-nc-sa/3.0/ Reprints
and permissions ABOUT THIS ARTICLE CITE THIS ARTICLE Shade, A., Gregory Caporaso, J., Handelsman, J. _et al._ A meta-analysis of changes in bacterial and archaeal communities with time.
_ISME J_ 7, 1493–1506 (2013). https://doi.org/10.1038/ismej.2013.54 Download citation * Received: 26 November 2012 * Revised: 22 February 2013 * Accepted: 26 February 2013 * Published: 11
April 2013 * Issue Date: August 2013 * DOI: https://doi.org/10.1038/ismej.2013.54 SHARE THIS ARTICLE Anyone you share the following link with will be able to read this content: Get shareable
link Sorry, a shareable link is not currently available for this article. Copy to clipboard Provided by the Springer Nature SharedIt content-sharing initiative KEYWORDS * similarity-decay *
species–time relationship * beta diversity * 16S rRNA * turnover * high-throughput sequencing