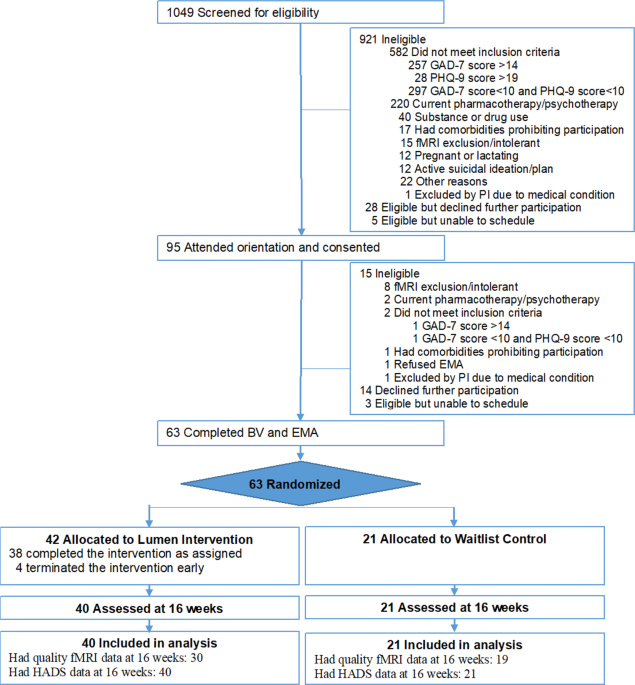
Effects of a virtual voice-based coach delivering problem-solving treatment on emotional distress and brain function: a pilot rct in depression and anxiety
- Select a language for the TTS:
- UK English Female
- UK English Male
- US English Female
- US English Male
- Australian Female
- Australian Male
- Language selected: (auto detect) - EN
Play all audios:

ABSTRACT Consumer-based voice assistants have the ability to deliver evidence-based treatment, but their therapeutic potential is largely unknown. In a pilot trial of a virtual voice-based
coach, Lumen, delivering problem-solving treatment, adults with mild-to-moderate depression and/or anxiety were randomized to the Lumen intervention (_n_ = 42) or waitlist control (_n_ =
21). The main outcomes included changes in neural measures of emotional reactivity and cognitive control, and Hospital Anxiety and Depression Scale [HADS] symptom scores over 16 weeks.
Participants were 37.8 years (SD = 12.4), 68% women, 25% Black, 24% Latino, and 11% Asian. Activation of the right dlPFC (neural region of interest in cognitive control) decreased in the
intervention group but increased in the control group, with an effect size meeting the prespecified threshold for a meaningful effect (Cohen’s _d_ = 0.3). Between-group differences in the
change in activation of the left dlPFC and bilateral amygdala were observed, but were of smaller magnitude (_d_ = 0.2). Change in right dlPFC activation was also meaningfully associated (_r_
≥ 0.4) with changes in self-reported problem-solving ability and avoidance in the intervention. Lumen intervention also led to decreased HADS depression, anxiety, and overall psychological
distress scores, with medium effect sizes (Cohen’s _d_ = 0.49, 0.51, and 0.55, respectively), compared with the waitlist control group. This pilot trial showed promising effects of a novel
digital mental health intervention on cognitive control using neuroimaging and depression and anxiety symptoms, providing foundational evidence for a future confirmatory study. SIMILAR
CONTENT BEING VIEWED BY OTHERS ONE-YEAR RANDOMIZED TRIAL COMPARING VIRTUAL REALITY-ASSISTED THERAPY TO COGNITIVE–BEHAVIORAL THERAPY FOR PATIENTS WITH TREATMENT-RESISTANT SCHIZOPHRENIA
Article Open access 12 February 2021 A DIGITAL INTERVENTION TARGETING COGNITIVE CONTROL NETWORK DYSFUNCTION IN MIDDLE AGE AND OLDER ADULTS WITH MAJOR DEPRESSION Article Open access 04 May
2021 PROTOCOL FOR EVALUATION OF ITEST, A NOVEL BLENDED INTERVENTION TO ENHANCE INTROSPECTIVE ACCURACY IN PSYCHOTIC DISORDERS Article Open access 14 February 2025 INTRODUCTION The prevalence
of depression in the United States has increased multiple fold to approximately 32% during the COVID-19 pandemic [1]. Correspondingly, >40 million adults (~19%) have anxiety disorders
[2], often with co-morbid depressive symptoms. Efficacious psychotherapies such as problem-solving treatment (PST) exist [3], and in-person PST is a proven intervention for treating both
depression and anxiety, which often manifest as comorbid conditions [4,5,6]. However, access to such therapies is affected by shortages in mental healthcare professionals and high
out-of-pocket costs. Digital mental health applications offer viable solutions [7, 8]; consumer-based voice assistants that leverage artificial intelligence to deliver psychotherapy is a
nascent and underexplored area with considerable potential for behavioral counseling and to promote emotional well-being [9, 10]. With the integration of voice assistants in mobile devices,
their use is pervasive; recent reports have highlighted that nearly a third of search queries rely on voice input [11]. However, their use in healthcare delivery is limited [10], with
current research largely focusing on information seeking activities on topics including healthy lifestyle [12, 13], medication names [14], and mental health [15, 16]. Although prototypes of
voice-based applications for behavioral assessment and counseling have been developed (e.g., [17, 18]), high-quality clinical research on their therapeutic potential is currently lacking.
Relying on user-centered design principles, and aligned with the treatment fidelity of PST, we developed a virtual voice-based coach, Lumen, that delivers PST for patients with
mild-to-moderate depression and/or anxiety [19, 20]. We conducted a pilot randomized clinical trial (RCT) to obtain initial evidence on the effects of PST delivery using Lumen on brain
function and clinical outcomes, as is consistent with a theory-driven, mechanism-focused approach to treatment evaluation. The primary objectives were to assess the magnitude of treatment
effects on: (a) the activation of a priori neural targets involved in emotional reactivity and cognitive control, the two core theoretical constructs for PST, and (b) depression and anxiety
symptoms. We also assessed associations between neural targets and self-reported surveys of emotional reactivity and cognitive control. METHOD The Institutional Review Board for the
University of Illinois Chicago (UIC) approved the study. All participants provided written consent. The study was registered on ClinicalTrials.gov (NCT# 04524104). PARTICIPANTS Enrollment
followed a multi-step process (Fig. 1). Participants were recruited between April 5, 2021, and October 7, 2021, from the outpatient care clinics at the University of Illinois Hospital and
Health Sciences System (UI Health) and employee email listservs at UIC, a minority-serving institution. Adults were deemed eligible if they had a Patient Health Questionnaire-9 (PHQ-9) score
of 10–19 and/or a Generalized Anxiety Disorder Scale (GAD-7) score of 10–14, without serious medical or psychiatric comorbidities or other exclusions (see Supplementary Material, Section A;
also see full protocol in Supplementary Material, Section H). Participants were asked to self-identify their race and ethnicity based on fixed categories to comply with National Institutes
of Health’s reporting requirements. Participants were compensated for this study. As part of this study, participants made two visits for neuroimaging (baseline [visit 1], and at 16 weeks
[visit 2]). Upon completion of visit 1, all participants received $50 in compensation. At visit 2, participants in the Lumen intervention arm could choose to receive $100 in compensation or
keep the iPad with their access to Lumen deactivated. For those in the waitlist control arm, at visit 2, participants could choose to receive $100 in compensation or choose to attend a Lumen
orientation session and receive a Lumen PST-enabled iPad (which they could keep in lieu of the $100 compensation). RANDOMIZATION AND MASKING Participants were randomly assigned in a 2:1
ratio to receive the Lumen intervention or to be in a waitlist control group using a validated online system [21] based on Pocock’s covariate-adaptive minimization [22]. The 2:1 allocation
allowed more participants to receive the Lumen intervention without substantially reducing statistical power [23]. Pocock’s minimization method was used to achieve better-than-chance
marginal balance across multiple baseline characteristics: age, sex, race/ethnicity, education, PHQ-9 score, GAD-7 score, and Digital Health Literacy [24]. Investigators, the safety monitor,
outcome assessors, and data analysts were blinded to participants’ treatment assignment. LUMEN Lumen is a virtual voice-based coach developed on Amazon’s Alexa platform. Lumen delivers an
evidence-based PST program [5, 6] consisting of eight sessions (four weekly, followed by four biweekly sessions) for patients with mild-to-moderate depression and/or anxiety. PST is
patient-driven, where the coach acts as a guide to identify a problem, set a goal, brainstorm solutions, choose a solution, develop an action plan, and to implement and evaluate the plan
[25]. This stepwise approach makes PST appropriate for therapy delivery using a virtual voice-based coach. Lumen was designed through an iterative user-centered process that involved
software developers, interaction designers, psychiatrists, PST experts, and behavioral scientists. Several iterations of the prototype were internally tested; a fully functional prototype
underwent feasibility and usability testing with 26 users [19]. The design was driven by two key principles: (a) aligning participants’ voice-based interaction with Lumen similar to the
cognitive processes of human communicative interactions [26], and (b) configuring the content of the interactions with the principles and process of evidence-based PST. Towards this end, the
Lumen architecture included multiple, interacting components that managed voice-based therapy delivery (a conversation manager), and ascertaining persistence and consistency across the
eight therapy sessions (a context manager; see additional information in Supplementary Material sections B, C, and D; Figure S1, Table S1, and Table S2). For this study, Lumen was integrated
within the Alexa app on an iPad provided to all participants. Lumen participants attended an in-person orientation session with a trained health coach where they received their iPad,
intervention workbook, and completed a tutorial on how to interact with Lumen. Participants were instructed to begin their PST right away, within 1 week of their orientation session and the
health coach helped schedule their 4 weekly and the following 4 biweekly PST sessions. Within 3 days of their first scheduled PST session, the health coach called participants to inquire
about any technical issues and helped troubleshoot these issues (if any). Participants received reminder text messages about their upcoming and overdue (if any) PST sessions. Participants
with overdue sessions, even after their reminders, were called by the health coach and encouraged to complete their outstanding session(s). Participants also had the opportunity to reach out
to the health coach if they faced any issues as part of their study. For each session, participants instantiated Lumen PST through the Alexa app with a “Launch Lumen Coach” voice
instruction and completed their assigned PST sessions. A typical Lumen session lasted ~12 min. Between sessions, participants completed surveys and ecological momentary assessments (EMAs,
see Supplementary Materials, Section D, Table S2). WAITLIST CONTROL Participants in the waitlist control arm received automated text messages to complete surveys and EMAs at intervals
similar to the intervention arm. These participants could choose to receive a Lumen-enabled iPad after their end-of-study assessments at 16 weeks. NEURAL TARGET MEASURES Blinded outcome
assessors conducted standardized assessments at baseline and 16 weeks. Task-based functional magnetic resonance imaging (fMRI) data were collected utilizing previously-established
standardized fMRI sequences and parameters [27, 28] that inform transdiagnostic phenotypes of neural circuit dysfunction for depression and anxiety. These fMRI methods, including facial
expressions task and Go-NoGo tasks, have been standardized in previous work designed for application to precision psychiatry and target engagement studies [29, 30]. A brief description of
these tasks are provided below, and additional details can be found in the Supplementary Materials (Section E). FACIAL EXPRESSIONS TASK A standardized set of 3D evoked facial expression
stimuli was presented in pseudorandom order, with 5 repeated blocks of 8 stimuli per block for sad, fear, anger, and happy relative to neutral blocks [29]. Participants were instructed to
continuously view the faces and were informed beforehand that they would be asked post-scan questions about the faces they were viewing. To assess amygdala activation for the negative affect
circuit, our analysis focused on threatening faces only, given our prior research showing threat-related amygdala activation mediating the effect of in-person PST on depression and
problem-solving outcomes [31]. Threat stimuli included a combination of fear and anger stimuli relative to neutral blocks. During the conscious viewing condition, each face was presented for
500 ms, with an interstimulus interval of 750 ms. To elicit the negative affect circuit in response to non-conscious threat stimuli, the same fear and anger stimuli were presented in a
backward-masking design to prevent awareness. In this non-conscious condition, face stimuli were presented for 10 ms followed immediately by a neutral face mask stimulus for 150 ms, and with
a stimulus onset asynchrony of 1250 ms to match that of the conscious condition [32]. GO-NOGO TASK For the Go-NoGo paradigm, the ‘Go’ and ‘NoGo’ stimuli were presented for 500 ms each with
an interstimulus interval of 750 ms. The Go-NoGo paradigm allowed for event-related analysis and is used to assess impulsivity (automatically generated ‘Go’ responses) versus inhibition
(‘NoGo’ responses). In the ‘Go’ trials, participants were asked to press a button on GREEN stimuli as quickly as possible (with the word “press” displayed in green); in the ‘NoGo’ trials,
participants should withhold button presses on RED stimuli (with the word “press” displayed in red). The probability of ‘NoGo’ stimuli was 0.33. A total of 180 ‘Go’ and 60 ‘NoGo’ stimuli
were presented in a pseudorandom order with a constraint to ensure that ‘NoGo’ stimuli were not repeated more than 3 times in a row. Reaction times and number of errors on task were used to
evaluate task performance [29]. Informed by previous findings [6, 31] identifying neural targets engaged by in-person PST, the primary target regions of interest (ROIs) were the amygdala
(bilaterally) representing a key node in the negative affect circuit, and the dorsal lateral prefrontal cortex (dlPFC) (bilaterally), a key node in the cognitive control circuit. The
negative affect circuit was engaged by the viewing of threat faces in the non-conscious viewing condition. The cognitive control circuit was engaged using the Go/No-go task. Person-level
activation of the ROIs for each contrast of interest for each task (e.g., threat versus neutral faces, no-go versus go) was derived in a manner consistent with the methods used in prior
studies [27]. CLINICAL OUTCOME MEASURES On the Hospital Anxiety and Depression Scale (HADS) [33, 34], depression and anxiety symptom scores ranged from 0 to 21, with 0–7 indicating normal;
8–10 indicating borderline abnormal (borderline); and 11–21 indicating abnormal (case). HADS total scores were computed as the sum of depression and anxiety scores, indicating overall
psychological distress. SELF-REPORTED MEASURES Validated self-report surveys of PST theory-based constructs of emotion (affect, worry) and cognition (problem-solving, dysfunctional
attitudes) were also completed at baseline and 16 weeks. The Positive and Negative Affect Schedule (PANAS) assessed positive and negative affect [35], with scores ranging from 10 to 50 and
higher scores representing higher levels of positive or negative affect. Worry was measured using the Penn State Worry Questionnaire (PSWQ), with a higher total score indicating more worry
(range 16–80) [36]. The Social Problem-solving Index-Revised Short Form (SPSI-R:S) assessed total problem-solving ability, with the higher score indicating more productive problem-solving
skills, and 5 subscales including problem orientation (positive, negative) and problem-solving styles (rational, impulsive/careless, and avoidant) [37]. Each subscale was scored by summing
the respective 5 items (each from 0 to 4), and the total problem-solving ability score ranged from 0 to 20 by averaging the subscale scores. Dysfunctional Attitudes Scale (DAS) measured the
presence and intensity of dysfunctional attitudes, with higher scores indicating more dysfunctional attitudes (range 40–280) [38]. STATISTICAL ANALYSIS The intervention vs. control effects
on changes in neural targets and self-reported measures of emotional reactivity and cognitive control from baseline to 16 weeks were assessed using _t_ tests. Correlations of changes in
neural targets with changes in self-reported measures were estimated using Pearson’s correlation tests. The intervention vs. control effects on changes in HADS scores from baseline to 16
weeks were tested using ordinary least square regression with adjustment of baseline values of the outcome measure. Each model included all participants with follow-up data on the outcome at
16 weeks, and participants were analyzed based on the group to which they were assigned. Moderation analysis used the same models as above plus the main effect of each potential effect
modifier (e.g., sex) and its interaction with the group; the latter, if significant, rejected the null hypothesis of no moderation. Model-adjusted between-treatment mean differences with 95%
confidence intervals (CIs) for the overall sample and the subgroups defined by the effect modifiers were reported. Cohen’s d was calculated by the mean difference between the two groups
divided by the pooled standard deviation. Given that this study was a pilot RCT, the primary purpose was to establish a reliable signal regarding the impact of Lumen on neural targets and
clinical outcomes that would be promising enough to warrant further research. Towards this end, we used Cohen’s _d_ ≥ 0.3 to define the meaningful mean difference between the intervention
and control groups in neural target and symptom changes from baseline to 16 weeks. Moreover, our approach to data reporting and interpretation regarding the intervention effects on neural
targets and symptom outcomes was focused on the magnitude and precision (95% CI) of the effect estimates, and not on _p_-values [5]. Similarly, we were not focused on smaller correlations
(Pearson’s _r_ < 0.4) between the neural targets and self-reported measures as it would have limited clinical relevance. All analyses were conducted using SAS, version 9.4 (SAS Institute
Inc., Cary, North Carolina). SAMPLE SIZE CALCULATION The sample size of this pilot RCT was calculated using a confidence interval approach. To obtain a precision interval with a standardized
half-width of 0.50 (akin to a medium effect) with 90% assurance, we had planned a sample size of 60 (_n__Treatment_ = 40, _n__Control_ = 20), assuming ≥85% retention at 16 weeks. A
precision interval approach was used where we defined that, compared with the waitlist control group, the intervention group will demonstrate a meaningful improvement in outcomes (in both
neural targets and symptoms) if the standardized between-group mean difference was at least Cohen’s d = 0.3 in favor of intervention. At this effect size, the upper limit of the precision
interval overlaps with _d_ = 0.8 (large effect) given a standardized half-width of 0.5 with 90% assurance that the interval contains the true mean difference based on power analysis. For the
correlation of change in neural targets with change in self-reported measures, a sample size of 51 (i.e., 60 × 85%) would be sufficient to detect a coefficient of r = 0.4 with 80% power and
2-sided α = 0.05. RESULTS SAMPLE CHARACTERISTICS AND RETENTION Of 1049 individuals who completed screening, 936 were ineligible and 50 declined or were unable to participate (Fig. 1).
Randomized participants included 42 in the intervention and 21 in the waitlist control. Participants had a mean age of 37.8 years (SD = 12.4), 68% were women, 25% were Black, 24% were
Latino, 59% had a high school or college (1 to 4+ years) education, and 51% had an annual income less than $55,000 (Table 1). On average, participants had moderate depression (mean PHQ-9
Score=12.8 [SD = 3.1]) and anxiety (mean GAD-7 Score=9.7 [SD = 2.7]), and borderline abnormal HADS depression scores (mean HADS depression = 7.3 [SD = 3.0]), and abnormal HADS anxiety scores
(mean HADS anxiety Score=10.6 [SD = 3.3]). Participants had a total HADS score of 17.9 [SD = 5.2]. Based on PHQ-9 and GAD-7 categories of symptom severity, a majority of the 63 participants
had moderate to moderately severe depression or moderate anxiety, and 29 had both. All 63 participants had complete baseline data, and 61 (97%) were assessed at 16 weeks. Of the 42 Lumen
participants, 38 (90.5%) completed at least 4 PST sessions, and 34 (81.0%) completed all 8 PST sessions. INTERVENTION EFFECT ON ACTIVATION OF NEURAL TARGETS Between-group mean differences in
changes in the primary ROIs (i.e., activation decrease in the control group > intervention in L. amygdala, activation decrease in intervention group > control group) engaged in the
non-conscious threat stimuli (i.e., emotional reactivity) from baseline to 16 weeks did not meet the Cohen’s d = 0.3 threshold. There was, however, a meaningful change in a primary cognitive
control target: the activation of both the right and left dlPFC decreased in the intervention from baseline to 16 weeks compared with the control, while the between-group mean difference in
activation of the right dlPFC (−0.20 [95%CI: −0.61, 0.22]) met Cohen’s d = 0.3 (Table 2). INTERVENTION EFFECT ON CLINICAL OUTCOMES At 16 weeks, intervention participants had greater
improvements in their HADS depression, anxiety and total scores compared with control participants, with a medium effect size. Model-adjusted between-group mean difference was −1.33 (95%CI:
−3.26, 0.60; Cohen’s d = 0.49) for the HADS depression score, −1.58 (95%CI: −3.82, 0.66; Cohen’s d = 0.51) for the HADS anxiety score, and −2.89 (95%CI: −6.76, 0.99; Cohen’s d = 0.55) for
the HADS total score (Table 3). The treatment effect on the HADS depression score was significantly moderated by sex (_p_ = 0.048), education (_p_ = 0.048), and digital health literacy score
(_p_ = 0.03). The Lumen group had consistently greater improvements than the control group in HADS depression, anxiety, and total scores than control participants among women (between-group
mean difference [95% CI]: −2.5 [−4.8, −0.3], −2.2 [−4.9, 0.5], −4.7 [−9.3, −0.2], respectively), non-White (−2.4 [−4.7, −0.0], −2.7 [−5.4, 0.0], −5.1 [−9.8, −0.4], respectively), and those
with college or less education (−2.9 [−5.6, −0.2], −3.1 [−6.3, 0.1], −6.0 [−11.4, −0.6], respectively) at 16 weeks (Fig. 2). In addition, participants with lower digital health literacy
scores had a greater mean decrease in their HADS depression score in the Lumen vs. control group (Supplementary Material, Section F, Figure S2). INTERVENTION EFFECT ON SELF-REPORTS Each of
the self-reports associated with emotional reactivity (positive affect score, negative affect score, Penn State worry questionnaire) showed improvement in the intervention and control groups
from baseline to 16 weeks, but did not show meaningful between-group differences (all Cohen’s d < 0.3). Similarly, all self-reports associated with cognitive control showed improvement
over time, with the rational problem-solving (1.43 [95%CI: −1.25, 4.1], Cohen’s d = 0.3) and impulsive/careless raw scores (−1.47, [95%CI: −3.57, 0.63], Cohen’s d = 0.4) having meaningful
differences in improvement in the intervention group compared with the control (Table 2). ASSOCIATION OF NEURAL TARGETS AND SELF-REPORTS In the intervention group, an increase in the
activation of the right dlPFC was positively correlated with an increased self-reported SPSI-R score indicating improved problem-solving ability (_r_ = 0.4, _p_ = 0.02), and negatively
associated with the self-reported avoidant score indicating reduced avoidance (_r_ = −0.5, _p_ = 0.01), from baseline to 16 weeks. In the waitlist control group, the right dlPFC was
negatively correlated with dysfunctional attitudes score (_r_ = −0.5, _p_ = 0.04). Moreover, associations were in the opposite direction in the control group for several of the other
considered scales, although they were not statistically significant: negative correlation with the self-reported SPSI-R score (_r_ = −0.5, _p_ = 0.050), and positive correlation with the
self-reported avoidant score (_r_ = 0.3, _p_ = 0.20) (see Supplementary Materials, Section G, Tables S3 and S4; Figure S3). DISCUSSION A virtual voice-based coach intervention showed
meaningful changes in a subset of select neural targets, with a decrease in the activation of the primary neural target related to cognitive control—the right dlPFC—in the intervention group
compared with the control. Related self-reported rational problem-solving and impulsive/carelessness scores also showed meaningful improvements with Lumen. The change in the right dlPFC
activation was also correlated positively with self-reported problem-solving ability scores and negatively with the avoidance scores in the Lumen group. Moreover, participants in the Lumen
intervention group showed improvements in both depression and anxiety symptoms as well as total psychological distress at 16 weeks, compared with the waitlist control group. The
between-group differences in the HADS-D and HADS-A scores were consistently greater than clinically important differences defined as 1.5–1.7 in prior studies [39, 40]. These treatment
effects were moderated by participant sex, race/ethnicity, and educational status. These findings offer a consistent signal and support the pragmatic viability of Lumen as a promising
digital intervention to address mild-to-moderate depression and/or anxiety. To the best of our knowledge, this is the first clinical trial of a virtual voice-based coach for behavioral
therapy, coupled with neuroimaging, that was delivered using a consumer-based voice platform (i.e., Amazon’s Alexa). The demonstration of neural target engagement for cognitive control and
improved clinical outcomes is promising and offers considerable opportunity for delivering PST. Moreover, more than 80% of the Lumen participants completed all 8 PST sessions, suggesting
high feasibility and acceptability among a highly diverse group of participants. The neural mechanistic findings supplement the validated self-report clinical outcomes, and may help
elucidate the theory-based therapeutic underpinnings of this novel form of PST delivery. Meaningful decrease in the activation of right dlPFC in the Lumen group is consistent with other
studies suggesting that right hemisphere hyperactivation is associated with depressive disorders [41]. Furthermore, the right dlPFC is frequently used as a target for low-frequency
inhibitory repetitive transcranial magnetic stimulation, suggesting that a reduction of activity in this region has an antidepressant effect [42]. The association of right dlPFC activation
with increased problem-solving skills and decreased avoidant scores in the intervention group may represent efficient processing by a key node involved in the cognitive control circuit. This
finding, in combination with our prior work from the ENGAGE-2 study [6], suggests that the malleability of dlPFC activity may serve as a prognostic biomarker for identifying patients who
would likely respond to this type of intervention. The presence of moderators in our models suggests that women, minorities, those college or less educated or those with lower digital health
literacy may likely benefit more from using Lumen. Among marginalized groups with lower resources and limited access to mental health services, Lumen offers a potential resource for easy
and on-demand access. This is especially the case, given the significant proliferation of mobile phones with voice applications. A recent qualitative study [43] found similar results,
showing the potential benefits and opportunities for using virtual technology for health management among Black men, further emphasizing the role of digital tools among minority populations.
There is considerable published literature and on-going research on the use of text-based applications (“chatbots”) for mental health support [44, 45]; however, a recent meta-analysis
showed that text-based chatbots had mixed results [46]. In this context, our study using a virtual voice-based coach offers a novel mental health therapy delivery mechanism. The absence of
full-fledged RCTs of voice-based applications is likely due to the challenges high-quality natural language understanding on these platforms. However, it must be stated that these platforms
have had greater success in directed and specific conversations utilizing the extensive modern machine learning and natural language processing algorithms. As such, Lumen was designed to
string together multiple short, directed conversations (e.g., “what is your goal?”) that reflect the therapeutic approach underlying the delivery of PST [19]. This study has several
limitations. First, this was a pilot RCT with a small study sample, among those with mild-to-moderate depression and/or anxiety, increasing the probability of false discovery and failure to
detect uncommon problems or adverse events. Nonetheless, this study provides the foundational evidence for a planned confirmatory study (NCT05603923). Second, task-based neuroimaging studies
have had varying within-subjects reliability, which may have reduced the capacity to detect changes in certain neural targets. Future studies will also complement data-driven approaches
such as whole-brain network analysis. Third, the comparison group did not receive any treatment, and it remains unclear how Lumen differs from PST delivered by human coaches. Fourth, we
considered several moderator variables in our analysis; given the small sample size the findings should be considered preliminary. Finally, although education and digital literacy were
moderating variables, the overall sample was well-educated and digitally literate. In summary, this pilot RCT provides preliminary evidence that a virtual voice-based coach may represent an
alternative option of PST delivery for managing mild-to-moderate depression and anxiety. This innovative approach may reduce barriers to mental health care access, particularly for
vulnerable populations. DATA AVAILABILITY Data used in the preparation of this manuscript will be submitted to the National Institute of Mental Health (NIMH) Data Archive (NDA). NDA is a
collaborative informatics system created by the National Institutes of Health to provide a national resource to support and accelerate research in mental health. Those wishing to use this
data can contact the corresponding author for the dataset identifier and make a request to the NIMH (visit https://nda.nih.gov/). This manuscript reflects the views of the authors and may
not reflect the opinions or views of the NIH. CHANGE HISTORY * _ 04 JULY 2023 A Correction to this paper has been published: https://doi.org/10.1038/s41398-023-02544-w _ REFERENCES * Ettman
CK, Cohen GH, Abdalla SM, Sampson L, Trinquart L, Castrucci BC, et al. Persistent depressive symptoms during COVID-19: a national, population-representative, longitudinal study of US adults.
The Lancet Regional Health-Americas. 2022;5:100091. Article PubMed Google Scholar * National Alliance on Mental Illness. Anxiety Disorders [cited 2002 April 29]. Available from:
https://www.nami.org/About-Mental-Illness/Mental-Health-Conditions/Anxiety-Disorders. * Bell AC, D’Zurilla TJ. Problem-solving therapy for depression: a meta-analysis. Clin Psychol Rev.
2009;29:348–53. Article PubMed Google Scholar * Ma J, Rosas LG, Lv N, Xiao L, Snowden MB, Venditti EM, et al. Effect of integrated behavioral weight loss treatment and problem-solving
therapy on body mass index and depressive symptoms among patients with obesity and depression: the RAINBOW randomized clinical trial. JAMA. 2019;321:869–79. Article PubMed PubMed Central
Google Scholar * Harrington D, D’Agostino RB, Sr, Gatsonis C, Hogan JW, Hunter DJ, Normand ST, et al. New guidelines for statistical reporting in the journal. N Engl J Med. 2019;381:285–6.
https://doi.org/10.1056/NEJMe1906559. Article PubMed Google Scholar * Lv N, Ajilore OA, Xiao L, Venditti EM, Lavori PW, Gerber BS, et al. Mediating effects of neural targets on
depression, weight and anxiety outcomes of an integrated collaborative care intervention: the ENGAGE-2 mechanistic pilot RCT. Biological Psychiatry: Global Open Science. 2022. * Torous J,
Myrick KJ, Rauseo-Ricupero N, Firth J. Digital mental health and COVID-19: using technology today to accelerate the curve on access and quality tomorrow. JMIR Mental Health. 2020;7:e18848.
Article PubMed PubMed Central Google Scholar * Miner AS, Shah N, Bullock KD, Arnow BA, Bailenson J, Hancock J. Key considerations for incorporating conversational AI in psychotherapy.
Front Psychiatry. 2019;10:746. Article PubMed PubMed Central Google Scholar * Sezgin E, Militello LK, Huang Y, Lin S. A scoping review of patient-facing, behavioral health interventions
with voice assistant technology targeting self-management and healthy lifestyle behaviors. Trans Behav Med. 2020;10:606–28. Article Google Scholar * Steinhubl SR, Topol EJ. Now we’re
talking: bringing a voice to digital medicine. Lancet. 2018;392:627. Article Google Scholar * [cited 2021 April 1]. Available from:
https://voicebot.ai/2019/07/09/new-data-on-voice-assistant-seo-is-a-wake-up-call-for-brands/. * Boyd M, Wilson N. Just ask Siri? A pilot study comparing smartphone digital assistants and
laptop Google searches for smoking cessation advice. PLoS ONE. 2018;13:e0194811. Article PubMed PubMed Central Google Scholar * Kocaballi AB, Quiroz JC, Rezazadegan D, Berkovsky S,
Magrabi F, Coiera E, et al. Responses of conversational agents to health and lifestyle prompts: investigation of appropriateness and presentation structures. Journal of medical Internet
research. 2020;22:e15823. Article PubMed PubMed Central Google Scholar * Palanica A, Thommandram A, Lee A, Li M, Fossat Y. Do you understand the words that are comin outta my mouth?
Voice assistant comprehension of medication names. NPJ Digital Medicine. 2019;2:1–6. Article Google Scholar * Miner AS, Milstein A, Schueller S, Hegde R, Mangurian C, Linos E.
Smartphone-based conversational agents and responses to questions about mental health, interpersonal violence, and physical health. JAMA internal medicine. 2016;176:619–25. Article PubMed
PubMed Central Google Scholar * Nobles AL, Leas EC, Caputi TL, Zhu S-H, Strathdee SA, Ayers JW. Responses to addiction help-seeking from Alexa, Siri, Google Assistant, Cortana, and Bixby
intelligent virtual assistants. NPJ Digital Med. 2020;3:1–3. Article Google Scholar * Ismail HO, Moses AR, Tadrus M, Mohamed EA, Jones LS. Feasibility of use of a smart speaker to
administer snellen visual acuity examinations in a clinical setting. JAMA Netw Open. 2020;3:e2013908-e. Article Google Scholar * Li J, Maharjan B, Xie B, Tao C. A personalized voice-based
diet assistant for caregivers of alzheimer disease and related dementias: system development and validation. J Med Int Res. 2020;22:e19897. Google Scholar * Kannampallil TG, Ronneberg CR,
Wittels N, Kumar V, Lv N, Smyth JM, et al. Design and formative evaluation of a voice-based virtual coach for problem-solving treatment: observational study. JMIR Formative Res.
2022;6:e38092. Article Google Scholar * Kannampallil T, Ronneberg CR, Wittels NE, Kumar V, Lv N, Smyth JM, et al. Design and formative evaluation of a virtual voice-based coach for
problem-solving treatment: observational study. JMIR Formative Res. 2022;6:e38092. Article Google Scholar * Xiao L, Huang Q, Yank V, Ma J. An easily accessible Web-based minimization
random allocation system for clinical trials. J Med Internet Res. 2013;15:e139 https://doi.org/10.2196/jmir.2392. Article PubMed PubMed Central Google Scholar * Scott NW, McPherson GC,
Ramsay CR, Campbell MK. The method of minimization for allocation to clinical trials. a review. Control Clin Trials. 2002;23:662–74. https://doi.org/10.1016/s0197-2456(02)00242-8. Article
PubMed Google Scholar * Meinert CL. Clinical Trials Design, Conduct, and Analysis. New York, NY: Oxford University Press; 1986. * Van Der Vaart R, Drossaert C. Development of the digital
health literacy instrument: measuring a broad spectrum of health 1.0 and health 2.0 skills. J Med Internet Res. 2017;19:e27. Article PubMed PubMed Central Google Scholar * Nezu AM, Nezu
CM, D’Zurilla T. Problem-solving therapy: A treatment manual: springer publishing company; 2012. * Kannampallil T, Smyth JM, Jones S, Payne PR, Ma J. Cognitive plausibility in voice-based AI
health counselors. NPJ digital medicine. 2020;3:1–4. Article Google Scholar * Goldstein-Piekarski AN, Ball TM, Samara Z, Staveland BR, Keller AS, Fleming SL, et al. Mapping neural circuit
biotypes to symptoms and behavioral dimensions of depression and anxiety. Biol Psychiatry. 2022;91:561–71. Article PubMed Google Scholar * Williams LM, Pines A, Goldstein-Piekarski AN,
Rosas LG, Kullar M, Sacchet MD, et al. The ENGAGE study: Integrating neuroimaging, virtual reality and smartphone sensing to understand self-regulation for managing depression and obesity in
a precision medicine model. Behav Res Ther. 2018;101:58–70. https://doi.org/10.1016/j.brat.2017.09.012. Article PubMed Google Scholar * Korgaonkar MS, Grieve SM, Etkin A, Koslow SH,
Williams LM. Using standardized fMRI protocols to identify patterns of prefrontal circuit dysregulation that are common and specific to cognitive and emotional tasks in major depressive
disorder: first wave results from the iSPOT-D study. Neuropsychopharmacology. 2013;38:863–71. https://doi.org/10.1038/npp.2012.252. Article PubMed PubMed Central Google Scholar * Tozzi
L, Goldstein-Piekarski AN, Korgaonkar MS, Williams LM. Connectivity of the cognitive control network during response inhibition as a predictive and response biomarker in major depression:
evidence from a randomized clinical trial. Biological Psychiatry. 2020;87:462–72. Article CAS PubMed Google Scholar * Goldstein-Piekarski AN, Wielgosz J, Xiao L, Stetz P, Correa CG,
Chang SE, et al. Early changes in neural circuit function engaged by negative emotion and modified by behavioural intervention are associated with depression and problem-solving outcomes: A
report from the ENGAGE randomized controlled trial. EBioMedicine. 2021;67:103387. https://doi.org/10.1016/j.ebiom.2021.103387. Article PubMed PubMed Central Google Scholar * Williams LM,
Korgaonkar MS, Song YC, Paton R, Eagles S, Goldstein-Piekarski A, et al. Amygdala reactivity to emotional faces in the prediction of general and medication-specific responses to
antidepressant treatment in the randomized iSPOT-D trial. Neuropsychopharmacology. 2015;40:2398–408. https://doi.org/10.1038/npp.2015.89. Article CAS PubMed PubMed Central Google Scholar
* Snaith R, Zigmond A. The Hospital Anxiety and Depression Scale manual. Windsor, Berkshire (UK): Nfer-Nelson; 1994. * Zigmond AS, Snaith RP. The hospital anxiety and depression scale.
Acta Psychiatrica Scand. 1983;67:361–70. Article CAS Google Scholar * Watson D, Clark LA, Tellegen A. Development and validation of brief measures of positive and negative affect: the
PANAS scales. J Person Soc Psychol. 1988;54:1063–70. https://doi.org/10.1037/0022-3514.54.6.1063. Article CAS Google Scholar * Meyer TJ, Miller ML, Metzger RL, Borkovec TD. Development
and validation of the Penn state worry questionnaire. Behav Res Ther. 1990;28:487–95. https://doi.org/10.1016/0005-7967(90)90135-6. Article CAS PubMed Google Scholar * D’Zurilla T, Nezu
A, Maydeu-Olivares A. Manual for the Social Problem-Solving Inventory-Revised. North Tonawanda, NY: Multi-Health Systems; 2002. * Weissman AN. The Dysfunctional Attitude Scale: A Validation
Study. Publicly Accessible Penn Dissertations. 1182: University of Pennsylvania; 1979. * Puhan MA, Frey M, Büchi S, Schünemann HJ. The minimal important difference of the hospital anxiety
and depression scale in patients with chronic obstructive pulmonary disease. Health Quality Life Outcomes. 2008;6:1–6. Article Google Scholar * Lemay KR, Tulloch HE, Pipe AL, Reed JL.
Establishing the minimal clinically important difference for the hospital anxiety and depression scale in patients with cardiovascular disease. J Cardiopulmonary Rehab Prev. 2019;39:E6–E11.
Article Google Scholar * Hecht D. Depression and the hyperactive right-hemisphere. Neurosci Res. 2010;68:77–87. Article PubMed Google Scholar * Brunoni AR, Chaimani A, Moffa AH, Razza
LB, Gattaz WF, Daskalakis ZJ, et al. Repetitive transcranial magnetic stimulation for the acute treatment of major depressive episodes: a systematic review with network meta-analysis. JAMA
Psychiatry. 2017;74:143–52. Article PubMed Google Scholar * Kramer J, Yinusa-Nyahkoon L, Olafsson S, Penti B, Woodhams E, Bickmore T, et al. Black men’s experiences with health care:
individuals’ accounts of challenges, suggestions for change, and the potential utility of virtual agent technology to assist black men with health management. Qualitative Health Res.
2021;31:1772–85. Article Google Scholar * Laranjo L, Dunn AG, Tong HL, Kocaballi AB, Chen J, Bashir R, et al. Conversational agents in healthcare: a systematic review. J Am Med Inform
Assoc. 2018;25:1248–58. Article PubMed PubMed Central Google Scholar * Vaidyam AN, Wisniewski H, Halamka JD, Kashavan MS, Torous JB. Chatbots and conversational agents in mental health:
a review of the psychiatric landscape. Can J Psychiatry. 2019;64:456–64. Article PubMed PubMed Central Google Scholar * Abd-Alrazaq AA, Rababeh A, Alajlani M, Bewick BM, Househ M.
Effectiveness and safety of using chatbots to improve mental health: systematic review and meta-analysis. J Med Internet Res. 2020;22:e16021. Article PubMed PubMed Central Google Scholar
Download references AUTHOR INFORMATION Author notes * These authors contributed equally: Thomas Kannampallil, Olusola A. Ajilore. AUTHORS AND AFFILIATIONS * Department of Anesthesiology,
Washington University School of Medicine, St Louis, MO, USA Thomas Kannampallil * Institute for Informatics, Washington University School of Medicine, St Louis, MO, USA Thomas Kannampallil *
Department of Psychiatry, University of Illinois at Chicago, Chicago, IL, USA Olusola A. Ajilore & Aifeng Zhang * Department of Medicine, University of Illinois at Chicago, Chicago, IL,
USA Nan Lv, Nancy E. Wittels, Corina R. Ronneberg, Vikas Kumar, Susanth Dosala, Amruta Barve, Kevin C. Tan, Kevin P. Cao, Charmi R. Patel, Emily A. Kringle & Jun Ma * Department of
Biobehavioral Health, The Pennsylvania State University, University Park, PA, USA Joshua M. Smyth & Jillian A. Johnson * Department of Epidemiology and Population Health, Stanford
University, Stanford, USA Lan Xiao * Department of Population & Quantitative Health Sciences, University of Massachusetts Medical School, Worcester, MA, USA Ben S. Gerber Authors *
Thomas Kannampallil View author publications You can also search for this author inPubMed Google Scholar * Olusola A. Ajilore View author publications You can also search for this author
inPubMed Google Scholar * Nan Lv View author publications You can also search for this author inPubMed Google Scholar * Joshua M. Smyth View author publications You can also search for this
author inPubMed Google Scholar * Nancy E. Wittels View author publications You can also search for this author inPubMed Google Scholar * Corina R. Ronneberg View author publications You can
also search for this author inPubMed Google Scholar * Vikas Kumar View author publications You can also search for this author inPubMed Google Scholar * Lan Xiao View author publications You
can also search for this author inPubMed Google Scholar * Susanth Dosala View author publications You can also search for this author inPubMed Google Scholar * Amruta Barve View author
publications You can also search for this author inPubMed Google Scholar * Aifeng Zhang View author publications You can also search for this author inPubMed Google Scholar * Kevin C. Tan
View author publications You can also search for this author inPubMed Google Scholar * Kevin P. Cao View author publications You can also search for this author inPubMed Google Scholar *
Charmi R. Patel View author publications You can also search for this author inPubMed Google Scholar * Ben S. Gerber View author publications You can also search for this author inPubMed
Google Scholar * Jillian A. Johnson View author publications You can also search for this author inPubMed Google Scholar * Emily A. Kringle View author publications You can also search for
this author inPubMed Google Scholar * Jun Ma View author publications You can also search for this author inPubMed Google Scholar CONTRIBUTIONS JM had full access to all the data in the
study and takes responsibility for the integrity of the data and the accuracy of the data analysis. NL, LX, and Zhang have accessed and verified the underlying data. Concept and design: JM,
TK, OAA, JMS. Acquisition, analysis, or interpretation of data: All authors. Drafting of the manuscript: Kannampallil, OAA, JMS, NL, JM. Critical revision of the manuscript for important
intellectual content: All authors. Statistical analysis: LX, NL. Obtained funding: JM, OAA. Administrative, technical, or material support: JM, LX, OAA, AZ. Supervision: JM, OAA.
CORRESPONDING AUTHOR Correspondence to Jun Ma. ETHICS DECLARATIONS COMPETING INTERESTS JM is a paid scientific consultant for Health Mentor, Inc. (San Jose, CA). OAA is the co-founder of
Keywise AI and serves on the advisory boards of Blueprint Health and Embodied Labs. TK is a paid consultant for Pfizer, Inc, outside of this work. The other authors report no conflicts of
interest. ADDITIONAL INFORMATION PUBLISHER’S NOTE Springer Nature remains neutral with regard to jurisdictional claims in published maps and institutional affiliations. SUPPLEMENTARY
INFORMATION SUPPLEMENTAL MATERIAL RIGHTS AND PERMISSIONS OPEN ACCESS This article is licensed under a Creative Commons Attribution 4.0 International License, which permits use, sharing,
adaptation, distribution and reproduction in any medium or format, as long as you give appropriate credit to the original author(s) and the source, provide a link to the Creative Commons
license, and indicate if changes were made. The images or other third party material in this article are included in the article’s Creative Commons license, unless indicated otherwise in a
credit line to the material. If material is not included in the article’s Creative Commons license and your intended use is not permitted by statutory regulation or exceeds the permitted
use, you will need to obtain permission directly from the copyright holder. To view a copy of this license, visit http://creativecommons.org/licenses/by/4.0/. Reprints and permissions ABOUT
THIS ARTICLE CITE THIS ARTICLE Kannampallil, T., Ajilore, O.A., Lv, N. _et al._ Effects of a virtual voice-based coach delivering problem-solving treatment on emotional distress and brain
function: a pilot RCT in depression and anxiety. _Transl Psychiatry_ 13, 166 (2023). https://doi.org/10.1038/s41398-023-02462-x Download citation * Received: 06 October 2022 * Revised: 14
April 2023 * Accepted: 26 April 2023 * Published: 12 May 2023 * DOI: https://doi.org/10.1038/s41398-023-02462-x SHARE THIS ARTICLE Anyone you share the following link with will be able to
read this content: Get shareable link Sorry, a shareable link is not currently available for this article. Copy to clipboard Provided by the Springer Nature SharedIt content-sharing
initiative